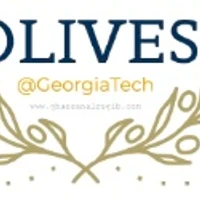
Image
imagewidth (px) 504
504
| B1
float64 0
1
⌀ | B2
float64 0
1
⌀ | B3
float64 0
1
⌀ | B4
float64 0
1
⌀ | B5
float64 0
1
⌀ | B6
float64 0
1
⌀ | BCVA
float64 40
100
⌀ | CST
float64 210
616
⌀ | Eye_ID
float64 18
83
⌀ | Patient_ID
float64 57
256
|
---|---|---|---|---|---|---|---|---|---|---|
1 | 0 | 1 | 1 | 0 | 1 | 82 | 326 | 18 | 247 |
|
1 | 0 | 1 | 1 | 0 | 1 | 97 | 275 | 58 | 58 |
|
1 | 0 | 1 | 1 | 0 | 0 | 82 | 326 | 18 | 247 |
|
1 | 0 | 1 | 1 | 0 | 0 | 97 | 275 | 58 | 58 |
|
1 | 0 | 1 | 0 | 0 | 0 | 82 | 326 | 18 | 247 |
|
1 | 0 | 1 | 0 | 0 | 0 | 97 | 275 | 58 | 58 |
|
1 | 0 | 1 | 1 | 0 | 0 | 82 | 326 | 18 | 247 |
|
1 | 0 | 1 | 1 | 0 | 0 | 97 | 275 | 58 | 58 |
|
1 | 0 | 1 | 0 | 0 | 0 | 82 | 326 | 18 | 247 |
|
1 | 0 | 1 | 0 | 0 | 0 | 97 | 275 | 58 | 58 |
|
1 | 0 | 1 | 1 | 0 | 0 | 82 | 326 | 18 | 247 |
|
1 | 0 | 1 | 1 | 0 | 0 | 97 | 275 | 58 | 58 |
|
1 | 0 | 1 | 1 | 0 | 0 | 82 | 326 | 18 | 247 |
|
1 | 0 | 1 | 1 | 0 | 0 | 97 | 275 | 58 | 58 |
|
1 | 0 | 1 | 1 | 0 | 1 | 82 | 326 | 18 | 247 |
|
1 | 0 | 1 | 1 | 0 | 1 | 97 | 275 | 58 | 58 |
|
1 | 0 | 1 | 1 | 0 | 0 | 82 | 326 | 18 | 247 |
|
1 | 0 | 1 | 1 | 0 | 0 | 97 | 275 | 58 | 58 |
|
1 | 0 | 1 | 1 | 0 | 0 | 82 | 326 | 18 | 247 |
|
1 | 0 | 1 | 1 | 0 | 0 | 97 | 275 | 58 | 58 |
|
1 | 0 | 1 | 1 | 0 | 1 | 82 | 326 | 18 | 247 |
|
1 | 0 | 1 | 1 | 0 | 1 | 97 | 275 | 58 | 58 |
|
1 | 0 | 1 | 1 | 0 | 0 | 82 | 326 | 18 | 247 |
|
1 | 0 | 1 | 1 | 0 | 0 | 97 | 275 | 58 | 58 |
|
0 | 0 | 1 | 1 | 0 | 0 | 82 | 326 | 18 | 247 |
|
0 | 0 | 1 | 1 | 0 | 0 | 97 | 275 | 58 | 58 |
|
0 | 0 | 1 | 1 | 0 | 0 | 82 | 326 | 18 | 247 |
|
0 | 0 | 1 | 1 | 0 | 0 | 97 | 275 | 58 | 58 |
|
1 | 0 | 1 | 1 | 0 | 0 | 82 | 326 | 18 | 247 |
|
1 | 0 | 1 | 1 | 0 | 0 | 97 | 275 | 58 | 58 |
|
1 | 0 | 1 | 1 | 0 | 0 | 82 | 326 | 18 | 247 |
|
1 | 0 | 1 | 1 | 0 | 0 | 97 | 275 | 58 | 58 |
|
1 | 0 | 1 | 1 | 0 | 0 | 82 | 326 | 18 | 247 |
|
1 | 0 | 1 | 1 | 0 | 0 | 97 | 275 | 58 | 58 |
|
0 | 0 | 1 | 1 | 0 | 0 | 82 | 326 | 18 | 247 |
|
0 | 0 | 1 | 1 | 0 | 0 | 97 | 275 | 58 | 58 |
|
0 | 0 | 1 | 1 | 0 | 0 | 82 | 326 | 18 | 247 |
|
0 | 0 | 1 | 1 | 0 | 0 | 97 | 275 | 58 | 58 |
|
1 | 0 | 1 | 1 | 0 | 0 | 82 | 326 | 18 | 247 |
|
1 | 0 | 1 | 1 | 0 | 0 | 97 | 275 | 58 | 58 |
|
1 | 0 | 1 | 1 | 0 | 0 | 82 | 326 | 18 | 247 |
|
1 | 0 | 1 | 1 | 0 | 0 | 97 | 275 | 58 | 58 |
|
1 | 0 | 1 | 1 | 0 | 0 | 82 | 326 | 18 | 247 |
|
1 | 0 | 1 | 1 | 0 | 0 | 97 | 275 | 58 | 58 |
|
1 | 0 | 1 | 1 | 0 | 0 | 82 | 326 | 18 | 247 |
|
1 | 0 | 1 | 1 | 0 | 0 | 97 | 275 | 58 | 58 |
|
1 | 0 | 1 | 1 | 0 | 0 | 82 | 326 | 18 | 247 |
|
1 | 0 | 1 | 1 | 0 | 0 | 97 | 275 | 58 | 58 |
|
1 | 0 | 1 | 1 | 0 | 0 | 82 | 326 | 18 | 247 |
|
1 | 0 | 1 | 1 | 0 | 0 | 97 | 275 | 58 | 58 |
|
1 | 0 | 1 | 1 | 0 | 1 | 82 | 326 | 18 | 247 |
|
1 | 0 | 1 | 1 | 0 | 1 | 97 | 275 | 58 | 58 |
|
1 | 0 | 1 | 1 | 0 | 1 | 82 | 326 | 18 | 247 |
|
1 | 0 | 1 | 1 | 0 | 1 | 97 | 275 | 58 | 58 |
|
1 | 0 | 1 | 1 | 0 | 1 | 82 | 326 | 18 | 247 |
|
1 | 0 | 1 | 1 | 0 | 1 | 97 | 275 | 58 | 58 |
|
1 | 0 | 1 | 1 | 0 | 1 | 82 | 326 | 18 | 247 |
|
1 | 0 | 1 | 1 | 0 | 1 | 97 | 275 | 58 | 58 |
|
1 | 0 | 1 | 1 | 0 | 1 | 82 | 326 | 18 | 247 |
|
1 | 0 | 1 | 1 | 0 | 1 | 97 | 275 | 58 | 58 |
|
1 | 0 | 1 | 0 | 0 | 1 | 82 | 326 | 18 | 247 |
|
1 | 0 | 1 | 0 | 0 | 1 | 97 | 275 | 58 | 58 |
|
1 | 0 | 1 | 1 | 0 | 1 | 82 | 326 | 18 | 247 |
|
1 | 0 | 1 | 1 | 0 | 1 | 97 | 275 | 58 | 58 |
|
1 | 0 | 1 | 0 | 0 | 1 | 82 | 326 | 18 | 247 |
|
1 | 0 | 1 | 0 | 0 | 1 | 97 | 275 | 58 | 58 |
|
1 | 0 | 1 | 0 | 0 | 0 | 82 | 326 | 18 | 247 |
|
1 | 0 | 1 | 0 | 0 | 0 | 97 | 275 | 58 | 58 |
|
1 | 0 | 1 | 1 | 0 | 0 | 82 | 326 | 18 | 247 |
|
1 | 0 | 1 | 1 | 0 | 0 | 97 | 275 | 58 | 58 |
|
1 | 0 | 1 | 0 | 0 | 0 | 82 | 326 | 18 | 247 |
|
1 | 0 | 1 | 0 | 0 | 0 | 97 | 275 | 58 | 58 |
|
1 | 0 | 1 | 0 | 0 | 0 | 82 | 326 | 18 | 247 |
|
1 | 0 | 1 | 0 | 0 | 0 | 97 | 275 | 58 | 58 |
|
1 | 0 | 1 | 0 | 0 | 0 | 82 | 326 | 18 | 247 |
|
1 | 0 | 1 | 0 | 0 | 0 | 97 | 275 | 58 | 58 |
|
0 | 0 | 1 | 0 | 0 | 0 | 82 | 326 | 18 | 247 |
|
0 | 0 | 1 | 0 | 0 | 0 | 97 | 275 | 58 | 58 |
|
0 | 1 | 0 | 0 | 0 | 0 | 82 | 326 | 18 | 247 |
|
0 | 1 | 0 | 0 | 0 | 0 | 97 | 275 | 58 | 58 |
|
0 | 1 | 0 | 0 | 0 | 0 | 82 | 326 | 18 | 247 |
|
0 | 1 | 0 | 0 | 0 | 0 | 97 | 275 | 58 | 58 |
|
0 | 1 | 0 | 0 | 0 | 0 | 82 | 326 | 18 | 247 |
|
0 | 1 | 0 | 0 | 0 | 0 | 97 | 275 | 58 | 58 |
|
0 | 1 | 0 | 0 | 0 | 0 | 82 | 326 | 18 | 247 |
|
0 | 1 | 0 | 0 | 0 | 0 | 97 | 275 | 58 | 58 |
|
0 | 1 | 0 | 0 | 0 | 0 | 82 | 326 | 18 | 247 |
|
0 | 1 | 0 | 0 | 0 | 0 | 97 | 275 | 58 | 58 |
|
1 | 0 | 1 | 1 | 0 | 0 | 82 | 326 | 18 | 247 |
|
1 | 0 | 1 | 1 | 0 | 0 | 97 | 275 | 58 | 58 |
|
1 | 0 | 1 | 0 | 0 | 0 | 82 | 326 | 18 | 247 |
|
1 | 0 | 1 | 0 | 0 | 0 | 97 | 275 | 58 | 58 |
|
0 | 0 | 1 | 0 | 0 | 0 | 82 | 326 | 18 | 247 |
|
0 | 0 | 1 | 0 | 0 | 0 | 97 | 275 | 58 | 58 |
|
1 | 0 | 1 | 0 | 0 | 1 | 82 | 326 | 18 | 247 |
|
1 | 0 | 1 | 0 | 0 | 1 | 97 | 275 | 58 | 58 |
|
1 | 0 | 1 | 0 | 0 | 1 | 82 | 326 | 18 | 247 |
|
1 | 0 | 1 | 0 | 0 | 1 | 97 | 275 | 58 | 58 |
|
null | null | null | null | null | null | 97 | 270 | 58 | 58 |
|
null | null | null | null | null | null | 97 | 270 | 58 | 58 |
OLIVES_Dataset
Abstract
Clinical diagnosis of the eye is performed over multifarious data modalities including scalar clinical labels, vectorized biomarkers, two-dimensional fundus images, and three-dimensional Optical Coherence Tomography (OCT) scans. While the clinical labels, fundus images and OCT scans are instrumental measurements, the vectorized biomarkers are interpreted attributes from the other measurements. Clinical practitioners use all these data modalities for diagnosing and treating eye diseases like Diabetic Retinopathy (DR) or Diabetic Macular Edema (DME). Enabling usage of machine learning algorithms within the ophthalmic medical domain requires research into the relationships and interactions between these relevant data modalities. Existing datasets are limited in that: ($i$) they view the problem as disease prediction without assessing biomarkers, and ($ii$) they do not consider the explicit relationship among all four data modalities over the treatment period. In this paper, we introduce the Ophthalmic Labels for Investigating Visual Eye Semantics (OLIVES) dataset that addresses the above limitations. This is the first OCT and fundus dataset that includes clinical labels, biomarker labels, and time-series patient treatment information from associated clinical trials. The dataset consists of $1268$ fundus eye images each with $49$ OCT scans, and $16$ biomarkers, along with $3$ clinical labels and a disease diagnosis of DR or DME. In total, there are 96 eyes' data averaged over a period of at least two years with each eye treated for an average of 66 weeks and 7 injections. OLIVES dataset has advantages in other fields of machine learning research including self-supervised learning as it provides alternate augmentation schemes that are medically grounded.
Subsets
There are 3 subsets included in this dataset:
- Disease Classification (
disease_classification
)
Disease Classification
This subset contains information regarding 78,000+ OCT scans obtained from a series of visits patients performed. In terms of labels, there are:
Image
: An image of the OCT scanBCVA
: Best Central Visual AcuityCST
: Central Subfield ThicknessPatient ID
: A value to help distinguish different patientsDisease Label
: A value of0
for DR (Diabetic Retinopathy) and1
for DME (Diabetic Macular Edema)
This information can be used to classify the disease. In addition, the first and last visit of a patient included extra biomarker information. This can be summarized into these 16 mostly-boolean labels:
Scan (n/49)
: The scan number out of the 49 scans taken for each patientAtrophy / thinning of retinal layer
Disruption of EZ
: Disruption of Ellipsoid ZoneDRIL
: Disruption of Retinal Inner LayersIR hemorrhages
: Intraretinal hemorrhagesIR HRF
: Intraretinal Hyperreflective FociPartially attached vitreous face
Fully attached vitreous face
Preretinal tissue/hemorrhage
Vitreous debris
VMT
: Vitreomacular TractionDRT/ME
: Diffuse Retinal Thickening or Macular EdemaFluid (IRF)
: Intraretinal FluidFluid (SRF)
: Subretinal FluidDisruption of RPE
: Disruption of Retinal Pigment EpitheliumPED (serous)
: Pigment Epithelial DetachmentSHRM
: Subretinal Hyperreflective MaterialEye_ID
: A value to help distinguish different eye scans
Data Download
Sample code to download the disease classification dataset:
from datasets import load_dataset
from torch.utils.data import DataLoader
olives = load_dataset('gOLIVES/OLIVES_Dataset', 'disease_classification', split = 'train')
# Covert into a Format Usable by Pytorch
olives = olives.with_format("torch")
dataloader = DataLoader(olives, batch_size=4)
for batch in dataloader:
print(batch)
# Example to get the VMT Biomarker of the first image in the dataset.
print(olives[0]['VMT'])
Known Issues
- Patient ID #79 has missing
BCVA
andCST
for most visits except the first and last visit as the biomarker information is present - Certain visits for patients seem to have the exact same scans as a previous visit. For instance Patient ID #61 has identical images in W8 and their next visit in W12.
Links
Associated Website: https://alregib.ece.gatech.edu/
Citations
If you find the work useful, please include the following citation in your work:
@inproceedings{prabhushankarolives2022,
title={OLIVES Dataset: Ophthalmic Labels for Investigating Visual Eye Semantics},
author={Prabhushankar, Mohit and Kokilepersaud, Kiran and Logan, Yash-yee and Trejo Corona, Stephanie and AlRegib, Ghassan and Wykoff, Charles},
booktitle={Proceedings of the Neural Information Processing Systems Track on Datasets and Benchmarks 2 (NeurIPS Datasets and Benchmarks 2022)},
year={2022}
}
- Downloads last month
- 284
Models trained or fine-tuned on gOLIVES/OLIVES_Dataset
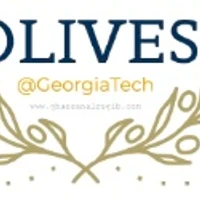