File size: 4,027 Bytes
3c544de d3be91c 3c544de d3be91c 3c544de d3be91c 78f84b0 d3be91c 5c7faf2 d3be91c 5c7faf2 d3be91c |
1 2 3 4 5 6 7 8 9 10 11 12 13 14 15 16 17 18 19 20 21 22 23 24 25 26 27 28 29 30 31 32 33 34 35 36 37 38 39 40 41 42 43 44 45 46 47 48 49 50 51 52 53 54 55 56 57 58 59 60 61 62 63 64 65 66 67 68 69 70 71 72 73 74 75 76 77 78 79 80 81 82 83 84 85 86 87 88 89 90 91 92 |
---
language:
- pt
thumbnail: Portuguese T5 for the Legal Domain
tags:
- transformers
license: mit
pipeline_tag: summarization
---
[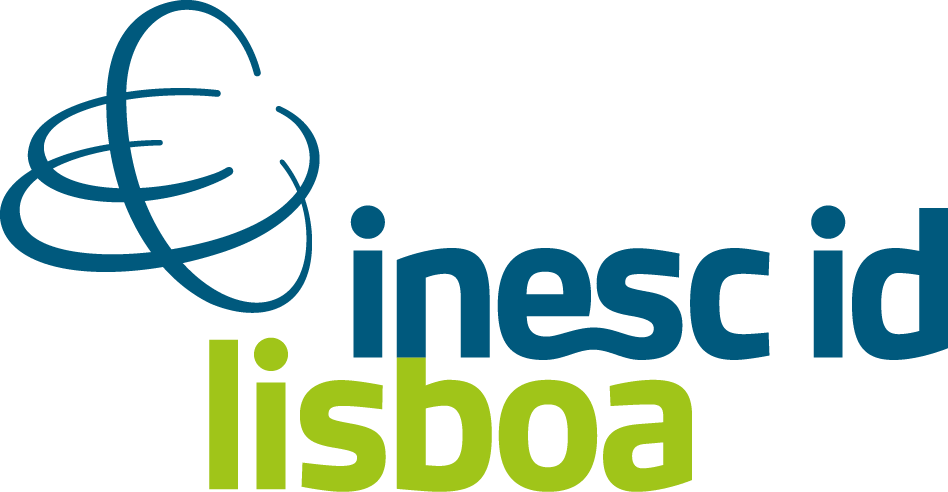](https://www.inesc-id.pt/projects/PR07005/)
[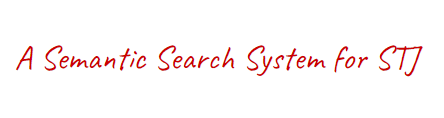](https://rufimelo99.github.io/SemanticSearchSystemForSTJ/)
Work developed as part of [Project IRIS](https://www.inesc-id.pt/projects/PR07005/).
Thesis: [A Semantic Search System for Supremo Tribunal de Justiça](https://rufimelo99.github.io/SemanticSearchSystemForSTJ/)
# stjiris/t5-portuguese-legal-summarization
T5 Model fine-tuned over “unicamp-dl/ptt5-base-portuguese-vocab” t5 model.
We utilized various jurisprudence and its summary to train this model.
## Usage (HuggingFace transformers)
```python
# name of folder principal
from transformers import T5Tokenizer, T5ForConditionalGeneration
model_checkpoint = "stjiris/t5-portuguese-legal-summarization"
t5_model = T5ForConditionalGeneration.from_pretrained(model_checkpoint)
t5_tokenizer = T5Tokenizer.from_pretrained(model_checkpoint)
preprocess_text = "These are some big words and text and words and text, again, that we want to summarize"
t5_prepared_Text = "summarize: "+preprocess_text
#print ("original text preprocessed: \n", preprocess_text)
tokenized_text = t5_tokenizer.encode(t5_prepared_Text, return_tensors="pt").to(device)
# summmarize
summary_ids = t5_model.generate(tokenized_text,
num_beams=4,
no_repeat_ngram_size=2,
min_length=512,
max_length=1024,
early_stopping=True)
output = t5_tokenizer.decode(summary_ids[0], skip_special_tokens=True)
print ("\n\nSummarized text: \n",output)
```
## Citing & Authors
### Contributions
[@rufimelo99](https://github.com/rufimelo99)
If you use this work, please cite:
```bibtex
@InProceedings{MeloSemantic,
author="Melo, Rui
and Santos, Pedro A.
and Dias, Jo{\~a}o",
editor="Moniz, Nuno
and Vale, Zita
and Cascalho, Jos{\'e}
and Silva, Catarina
and Sebasti{\~a}o, Raquel",
title="A Semantic Search System for the Supremo Tribunal de Justi{\c{c}}a",
booktitle="Progress in Artificial Intelligence",
year="2023",
publisher="Springer Nature Switzerland",
address="Cham",
pages="142--154",
abstract="Many information retrieval systems use lexical approaches to retrieve information. Such approaches have multiple limitations, and these constraints are exacerbated when tied to specific domains, such as the legal one. Large language models, such as BERT, deeply understand a language and may overcome the limitations of older methodologies, such as BM25. This work investigated and developed a prototype of a Semantic Search System to assist the Supremo Tribunal de Justi{\c{c}}a (Portuguese Supreme Court of Justice) in its decision-making process. We built a Semantic Search System that uses specially trained BERT models (Legal-BERTimbau variants) and a Hybrid Search System that incorporates both lexical and semantic techniques by combining the capabilities of BM25 and the potential of Legal-BERTimbau. In this context, we obtained a {\$}{\$}335{\backslash}{\%}{\$}{\$}335{\%}increase on the discovery metric when compared to BM25 for the first query result. This work also provides information on the most relevant techniques for training a Large Language Model adapted to Portuguese jurisprudence and introduces a new technique of Metadata Knowledge Distillation.",
isbn="978-3-031-49011-8"
}
@article{ptt5_2020,
title={PTT5: Pretraining and validating the T5 model on Brazilian Portuguese data},
author={Carmo, Diedre and Piau, Marcos and Campiotti, Israel and Nogueira, Rodrigo and Lotufo, Roberto},
journal={arXiv preprint arXiv:2008.09144},
year={2020}
}
``` |