diff --git a/ packages.txt b/ packages.txt
new file mode 100644
index 0000000000000000000000000000000000000000..7d6f62c7bcd8ed4790678aa6f35ef71093919e62
--- /dev/null
+++ b/ packages.txt
@@ -0,0 +1,2 @@
+bzip2
+cmake
\ No newline at end of file
diff --git a/.gitattributes b/.gitattributes
new file mode 100644
index 0000000000000000000000000000000000000000..c7d9f3332a950355d5a77d85000f05e6f45435ea
--- /dev/null
+++ b/.gitattributes
@@ -0,0 +1,34 @@
+*.7z filter=lfs diff=lfs merge=lfs -text
+*.arrow filter=lfs diff=lfs merge=lfs -text
+*.bin filter=lfs diff=lfs merge=lfs -text
+*.bz2 filter=lfs diff=lfs merge=lfs -text
+*.ckpt filter=lfs diff=lfs merge=lfs -text
+*.ftz filter=lfs diff=lfs merge=lfs -text
+*.gz filter=lfs diff=lfs merge=lfs -text
+*.h5 filter=lfs diff=lfs merge=lfs -text
+*.joblib filter=lfs diff=lfs merge=lfs -text
+*.lfs.* filter=lfs diff=lfs merge=lfs -text
+*.mlmodel filter=lfs diff=lfs merge=lfs -text
+*.model filter=lfs diff=lfs merge=lfs -text
+*.msgpack filter=lfs diff=lfs merge=lfs -text
+*.npy filter=lfs diff=lfs merge=lfs -text
+*.npz filter=lfs diff=lfs merge=lfs -text
+*.onnx filter=lfs diff=lfs merge=lfs -text
+*.ot filter=lfs diff=lfs merge=lfs -text
+*.parquet filter=lfs diff=lfs merge=lfs -text
+*.pb filter=lfs diff=lfs merge=lfs -text
+*.pickle filter=lfs diff=lfs merge=lfs -text
+*.pkl filter=lfs diff=lfs merge=lfs -text
+*.pt filter=lfs diff=lfs merge=lfs -text
+*.pth filter=lfs diff=lfs merge=lfs -text
+*.rar filter=lfs diff=lfs merge=lfs -text
+*.safetensors filter=lfs diff=lfs merge=lfs -text
+saved_model/**/* filter=lfs diff=lfs merge=lfs -text
+*.tar.* filter=lfs diff=lfs merge=lfs -text
+*.tflite filter=lfs diff=lfs merge=lfs -text
+*.tgz filter=lfs diff=lfs merge=lfs -text
+*.wasm filter=lfs diff=lfs merge=lfs -text
+*.xz filter=lfs diff=lfs merge=lfs -text
+*.zip filter=lfs diff=lfs merge=lfs -text
+*.zst filter=lfs diff=lfs merge=lfs -text
+*tfevents* filter=lfs diff=lfs merge=lfs -text
diff --git a/README.md b/README.md
new file mode 100644
index 0000000000000000000000000000000000000000..857ac88fafe1e144537ae70a321e6cfee7b144a2
--- /dev/null
+++ b/README.md
@@ -0,0 +1,10 @@
+---
+title: StyleGANEX
+sdk: gradio
+emoji: 🐨
+colorFrom: pink
+colorTo: yellow
+app_file: app.py
+pinned: false
+duplicated_from: PKUWilliamYang/StyleGANEX
+---
diff --git a/app.py b/app.py
new file mode 100644
index 0000000000000000000000000000000000000000..ecbf1001f0427cef59b76b004c7d89a7e4c3c68d
--- /dev/null
+++ b/app.py
@@ -0,0 +1,112 @@
+from __future__ import annotations
+
+import argparse
+import pathlib
+import torch
+import gradio as gr
+
+from webUI.app_task import *
+from webUI.styleganex_model import Model
+
+def parse_args() -> argparse.Namespace:
+ parser = argparse.ArgumentParser()
+ parser.add_argument('--device', type=str, default='cpu')
+ parser.add_argument('--theme', type=str)
+ parser.add_argument('--share', action='store_true')
+ parser.add_argument('--port', type=int)
+ parser.add_argument('--disable-queue',
+ dest='enable_queue',
+ action='store_false')
+ return parser.parse_args()
+
+DESCRIPTION = '''
+
+
+ Face Manipulation with StyleGANEX
+
+
For faster inference without waiting in queue, you may duplicate the space and upgrade to GPU in settings.
+
+
+
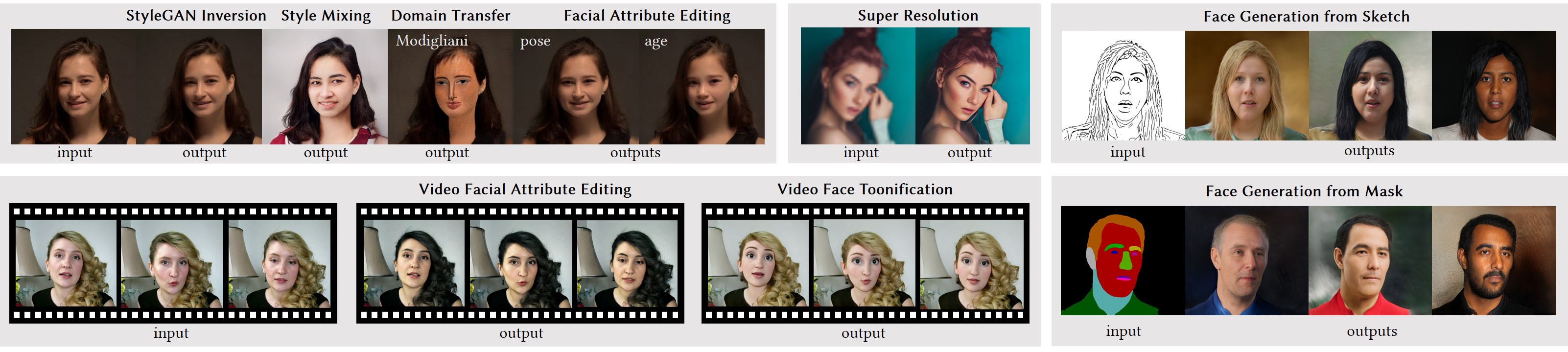
+
+'''
+ARTICLE = r"""
+If StyleGANEX is helpful, please help to ⭐ the Github Repo. Thanks!
+[](https://github.com/williamyang1991/StyleGANEX)
+---
+📝 **Citation**
+If our work is useful for your research, please consider citing:
+```bibtex
+@article{yang2023styleganex,
+ title = {StyleGANEX: StyleGAN-Based Manipulation Beyond Cropped Aligned Faces},
+ author = {Yang, Shuai and Jiang, Liming and Liu, Ziwei and and Loy, Chen Change},
+ journal = {arXiv preprint arXiv:2303.06146},
+ year={2023},
+}
+```
+📋 **License**
+This project is licensed under S-Lab License 1.0.
+Redistribution and use for non-commercial purposes should follow this license.
+
+📧 **Contact**
+If you have any questions, please feel free to reach me out at williamyang@pku.edu.cn.
+"""
+
+FOOTER = ''
+
+def main():
+ args = parse_args()
+ args.device = 'cuda' if torch.cuda.is_available() else 'cpu'
+ print('*** Now using %s.'%(args.device))
+ model = Model(device=args.device)
+
+
+ torch.hub.download_url_to_file('https://raw.githubusercontent.com/williamyang1991/StyleGANEX/main/data/234_sketch.jpg',
+ '234_sketch.jpg')
+ torch.hub.download_url_to_file('https://github.com/williamyang1991/StyleGANEX/raw/main/output/ILip77SbmOE_inversion.pt',
+ 'ILip77SbmOE_inversion.pt')
+ torch.hub.download_url_to_file('https://raw.githubusercontent.com/williamyang1991/StyleGANEX/main/data/ILip77SbmOE.png',
+ 'ILip77SbmOE.png')
+ torch.hub.download_url_to_file('https://raw.githubusercontent.com/williamyang1991/StyleGANEX/main/data/ILip77SbmOE_mask.png',
+ 'ILip77SbmOE_mask.png')
+ torch.hub.download_url_to_file('https://raw.githubusercontent.com/williamyang1991/StyleGANEX/main/data/pexels-daniel-xavier-1239291.jpg',
+ 'pexels-daniel-xavier-1239291.jpg')
+ torch.hub.download_url_to_file('https://github.com/williamyang1991/StyleGANEX/raw/main/data/529_2.mp4',
+ '529_2.mp4')
+ torch.hub.download_url_to_file('https://github.com/williamyang1991/StyleGANEX/raw/main/data/684.mp4',
+ '684.mp4')
+ torch.hub.download_url_to_file('https://github.com/williamyang1991/StyleGANEX/raw/main/data/pexels-anthony-shkraba-production-8136210.mp4',
+ 'pexels-anthony-shkraba-production-8136210.mp4')
+
+
+ with gr.Blocks(css='style.css') as demo:
+ gr.Markdown(DESCRIPTION)
+ with gr.Tabs():
+ with gr.TabItem('Inversion for Editing'):
+ create_demo_inversion(model.process_inversion, allow_optimization=False)
+ with gr.TabItem('Image Face Toonify'):
+ create_demo_toonify(model.process_toonify)
+ with gr.TabItem('Video Face Toonify'):
+ create_demo_vtoonify(model.process_vtoonify, max_frame_num=12)
+ with gr.TabItem('Image Face Editing'):
+ create_demo_editing(model.process_editing)
+ with gr.TabItem('Video Face Editing'):
+ create_demo_vediting(model.process_vediting, max_frame_num=12)
+ with gr.TabItem('Sketch2Face'):
+ create_demo_s2f(model.process_s2f)
+ with gr.TabItem('Mask2Face'):
+ create_demo_m2f(model.process_m2f)
+ with gr.TabItem('SR'):
+ create_demo_sr(model.process_sr)
+ gr.Markdown(ARTICLE)
+ gr.Markdown(FOOTER)
+
+ demo.launch(
+ enable_queue=args.enable_queue,
+ server_port=args.port,
+ share=args.share,
+ )
+
+if __name__ == '__main__':
+ main()
+
diff --git a/configs/__init__.py b/configs/__init__.py
new file mode 100644
index 0000000000000000000000000000000000000000..e69de29bb2d1d6434b8b29ae775ad8c2e48c5391
diff --git a/configs/data_configs.py b/configs/data_configs.py
new file mode 100644
index 0000000000000000000000000000000000000000..7624ed6ccb0054030afafe0cf049cf210129b812
--- /dev/null
+++ b/configs/data_configs.py
@@ -0,0 +1,48 @@
+from configs import transforms_config
+from configs.paths_config import dataset_paths
+
+
+DATASETS = {
+ 'ffhq_encode': {
+ 'transforms': transforms_config.EncodeTransforms,
+ 'train_source_root': dataset_paths['ffhq'],
+ 'train_target_root': dataset_paths['ffhq'],
+ 'test_source_root': dataset_paths['ffhq_test'],
+ 'test_target_root': dataset_paths['ffhq_test'],
+ },
+ 'ffhq_sketch_to_face': {
+ 'transforms': transforms_config.SketchToImageTransforms,
+ 'train_source_root': dataset_paths['ffhq_train_sketch'],
+ 'train_target_root': dataset_paths['ffhq'],
+ 'test_source_root': dataset_paths['ffhq_test_sketch'],
+ 'test_target_root': dataset_paths['ffhq_test'],
+ },
+ 'ffhq_seg_to_face': {
+ 'transforms': transforms_config.SegToImageTransforms,
+ 'train_source_root': dataset_paths['ffhq_train_segmentation'],
+ 'train_target_root': dataset_paths['ffhq'],
+ 'test_source_root': dataset_paths['ffhq_test_segmentation'],
+ 'test_target_root': dataset_paths['ffhq_test'],
+ },
+ 'ffhq_super_resolution': {
+ 'transforms': transforms_config.SuperResTransforms,
+ 'train_source_root': dataset_paths['ffhq'],
+ 'train_target_root': dataset_paths['ffhq1280'],
+ 'test_source_root': dataset_paths['ffhq_test'],
+ 'test_target_root': dataset_paths['ffhq1280_test'],
+ },
+ 'toonify': {
+ 'transforms': transforms_config.ToonifyTransforms,
+ 'train_source_root': dataset_paths['toonify_in'],
+ 'train_target_root': dataset_paths['toonify_out'],
+ 'test_source_root': dataset_paths['toonify_test_in'],
+ 'test_target_root': dataset_paths['toonify_test_out'],
+ },
+ 'ffhq_edit': {
+ 'transforms': transforms_config.EditingTransforms,
+ 'train_source_root': dataset_paths['ffhq'],
+ 'train_target_root': dataset_paths['ffhq'],
+ 'test_source_root': dataset_paths['ffhq_test'],
+ 'test_target_root': dataset_paths['ffhq_test'],
+ },
+}
diff --git a/configs/dataset_config.yml b/configs/dataset_config.yml
new file mode 100644
index 0000000000000000000000000000000000000000..f7addabb39ff776e4b899a2e41080ff242e7ae01
--- /dev/null
+++ b/configs/dataset_config.yml
@@ -0,0 +1,60 @@
+# dataset and data loader settings
+datasets:
+ train:
+ name: FFHQ
+ type: FFHQDegradationDataset
+ # dataroot_gt: datasets/ffhq/ffhq_512.lmdb
+ dataroot_gt: ../../../../share/shuaiyang/ffhq/realign1280x1280test/
+ io_backend:
+ # type: lmdb
+ type: disk
+
+ use_hflip: true
+ mean: [0.5, 0.5, 0.5]
+ std: [0.5, 0.5, 0.5]
+ out_size: 1280
+ scale: 4
+
+ blur_kernel_size: 41
+ kernel_list: ['iso', 'aniso']
+ kernel_prob: [0.5, 0.5]
+ blur_sigma: [0.1, 10]
+ downsample_range: [4, 40]
+ noise_range: [0, 20]
+ jpeg_range: [60, 100]
+
+ # color jitter and gray
+ #color_jitter_prob: 0.3
+ #color_jitter_shift: 20
+ #color_jitter_pt_prob: 0.3
+ #gray_prob: 0.01
+
+ # If you do not want colorization, please set
+ color_jitter_prob: ~
+ color_jitter_pt_prob: ~
+ gray_prob: 0.01
+ gt_gray: True
+
+ crop_components: true
+ component_path: ./pretrained_models/FFHQ_eye_mouth_landmarks_512.pth
+ eye_enlarge_ratio: 1.4
+
+ # data loader
+ use_shuffle: true
+ num_worker_per_gpu: 6
+ batch_size_per_gpu: 4
+ dataset_enlarge_ratio: 1
+ prefetch_mode: ~
+
+ val:
+ # Please modify accordingly to use your own validation
+ # Or comment the val block if do not need validation during training
+ name: validation
+ type: PairedImageDataset
+ dataroot_lq: datasets/faces/validation/input
+ dataroot_gt: datasets/faces/validation/reference
+ io_backend:
+ type: disk
+ mean: [0.5, 0.5, 0.5]
+ std: [0.5, 0.5, 0.5]
+ scale: 1
diff --git a/configs/paths_config.py b/configs/paths_config.py
new file mode 100644
index 0000000000000000000000000000000000000000..2d5d7e14859e90ecd4927946f2881247628fddba
--- /dev/null
+++ b/configs/paths_config.py
@@ -0,0 +1,25 @@
+dataset_paths = {
+ 'ffhq': 'data/train/ffhq/realign320x320/',
+ 'ffhq_test': 'data/train/ffhq/realign320x320test/',
+ 'ffhq1280': 'data/train/ffhq/realign1280x1280/',
+ 'ffhq1280_test': 'data/train/ffhq/realign1280x1280test/',
+ 'ffhq_train_sketch': 'data/train/ffhq/realign640x640sketch/',
+ 'ffhq_test_sketch': 'data/train/ffhq/realign640x640sketchtest/',
+ 'ffhq_train_segmentation': 'data/train/ffhq/realign320x320mask/',
+ 'ffhq_test_segmentation': 'data/train/ffhq/realign320x320masktest/',
+ 'toonify_in': 'data/train/pixar/trainA/',
+ 'toonify_out': 'data/train/pixar/trainB/',
+ 'toonify_test_in': 'data/train/pixar/testA/',
+ 'toonify_test_out': 'data/train/testB/',
+}
+
+model_paths = {
+ 'stylegan_ffhq': 'pretrained_models/stylegan2-ffhq-config-f.pt',
+ 'ir_se50': 'pretrained_models/model_ir_se50.pth',
+ 'circular_face': 'pretrained_models/CurricularFace_Backbone.pth',
+ 'mtcnn_pnet': 'pretrained_models/mtcnn/pnet.npy',
+ 'mtcnn_rnet': 'pretrained_models/mtcnn/rnet.npy',
+ 'mtcnn_onet': 'pretrained_models/mtcnn/onet.npy',
+ 'shape_predictor': 'shape_predictor_68_face_landmarks.dat',
+ 'moco': 'pretrained_models/moco_v2_800ep_pretrain.pth.tar'
+}
diff --git a/configs/transforms_config.py b/configs/transforms_config.py
new file mode 100644
index 0000000000000000000000000000000000000000..0af0404f4f59c79e5f672205031470bdab013622
--- /dev/null
+++ b/configs/transforms_config.py
@@ -0,0 +1,242 @@
+from abc import abstractmethod
+import torchvision.transforms as transforms
+from datasets import augmentations
+
+
+class TransformsConfig(object):
+
+ def __init__(self, opts):
+ self.opts = opts
+
+ @abstractmethod
+ def get_transforms(self):
+ pass
+
+
+class EncodeTransforms(TransformsConfig):
+
+ def __init__(self, opts):
+ super(EncodeTransforms, self).__init__(opts)
+
+ def get_transforms(self):
+ transforms_dict = {
+ 'transform_gt_train': transforms.Compose([
+ transforms.Resize((320, 320)),
+ transforms.RandomHorizontalFlip(0.5),
+ transforms.ToTensor(),
+ transforms.Normalize([0.5, 0.5, 0.5], [0.5, 0.5, 0.5])]),
+ 'transform_source': None,
+ 'transform_test': transforms.Compose([
+ transforms.Resize((320, 320)),
+ transforms.ToTensor(),
+ transforms.Normalize([0.5, 0.5, 0.5], [0.5, 0.5, 0.5])]),
+ 'transform_inference': transforms.Compose([
+ transforms.Resize((320, 320)),
+ transforms.ToTensor(),
+ transforms.Normalize([0.5, 0.5, 0.5], [0.5, 0.5, 0.5])])
+ }
+ return transforms_dict
+
+
+class FrontalizationTransforms(TransformsConfig):
+
+ def __init__(self, opts):
+ super(FrontalizationTransforms, self).__init__(opts)
+
+ def get_transforms(self):
+ transforms_dict = {
+ 'transform_gt_train': transforms.Compose([
+ transforms.Resize((256, 256)),
+ transforms.RandomHorizontalFlip(0.5),
+ transforms.ToTensor(),
+ transforms.Normalize([0.5, 0.5, 0.5], [0.5, 0.5, 0.5])]),
+ 'transform_source': transforms.Compose([
+ transforms.Resize((256, 256)),
+ transforms.RandomHorizontalFlip(0.5),
+ transforms.ToTensor(),
+ transforms.Normalize([0.5, 0.5, 0.5], [0.5, 0.5, 0.5])]),
+ 'transform_test': transforms.Compose([
+ transforms.Resize((256, 256)),
+ transforms.ToTensor(),
+ transforms.Normalize([0.5, 0.5, 0.5], [0.5, 0.5, 0.5])]),
+ 'transform_inference': transforms.Compose([
+ transforms.Resize((256, 256)),
+ transforms.ToTensor(),
+ transforms.Normalize([0.5, 0.5, 0.5], [0.5, 0.5, 0.5])])
+ }
+ return transforms_dict
+
+
+class SketchToImageTransforms(TransformsConfig):
+
+ def __init__(self, opts):
+ super(SketchToImageTransforms, self).__init__(opts)
+
+ def get_transforms(self):
+ transforms_dict = {
+ 'transform_gt_train': transforms.Compose([
+ transforms.Resize((320, 320)),
+ transforms.ToTensor(),
+ transforms.Normalize([0.5, 0.5, 0.5], [0.5, 0.5, 0.5])]),
+ 'transform_source': transforms.Compose([
+ transforms.Resize((320, 320)),
+ transforms.ToTensor()]),
+ 'transform_test': transforms.Compose([
+ transforms.Resize((320, 320)),
+ transforms.ToTensor(),
+ transforms.Normalize([0.5, 0.5, 0.5], [0.5, 0.5, 0.5])]),
+ 'transform_inference': transforms.Compose([
+ transforms.Resize((320, 320)),
+ transforms.ToTensor()]),
+ }
+ return transforms_dict
+
+
+class SegToImageTransforms(TransformsConfig):
+
+ def __init__(self, opts):
+ super(SegToImageTransforms, self).__init__(opts)
+
+ def get_transforms(self):
+ transforms_dict = {
+ 'transform_gt_train': transforms.Compose([
+ transforms.Resize((320, 320)),
+ transforms.ToTensor(),
+ transforms.Normalize([0.5, 0.5, 0.5], [0.5, 0.5, 0.5])]),
+ 'transform_source': transforms.Compose([
+ transforms.Resize((320, 320)),
+ augmentations.ToOneHot(self.opts.label_nc),
+ transforms.ToTensor()]),
+ 'transform_test': transforms.Compose([
+ transforms.Resize((320, 320)),
+ transforms.ToTensor(),
+ transforms.Normalize([0.5, 0.5, 0.5], [0.5, 0.5, 0.5])]),
+ 'transform_inference': transforms.Compose([
+ transforms.Resize((320, 320)),
+ augmentations.ToOneHot(self.opts.label_nc),
+ transforms.ToTensor()])
+ }
+ return transforms_dict
+
+
+class SuperResTransforms(TransformsConfig):
+
+ def __init__(self, opts):
+ super(SuperResTransforms, self).__init__(opts)
+
+ def get_transforms(self):
+ if self.opts.resize_factors is None:
+ self.opts.resize_factors = '1,2,4,8,16,32'
+ factors = [int(f) for f in self.opts.resize_factors.split(",")]
+ print("Performing down-sampling with factors: {}".format(factors))
+ transforms_dict = {
+ 'transform_gt_train': transforms.Compose([
+ transforms.Resize((1280, 1280)),
+ transforms.ToTensor(),
+ transforms.Normalize([0.5, 0.5, 0.5], [0.5, 0.5, 0.5])]),
+ 'transform_source': transforms.Compose([
+ transforms.Resize((320, 320)),
+ augmentations.BilinearResize(factors=factors),
+ transforms.Resize((320, 320)),
+ transforms.ToTensor(),
+ transforms.Normalize([0.5, 0.5, 0.5], [0.5, 0.5, 0.5])]),
+ 'transform_test': transforms.Compose([
+ transforms.Resize((1280, 1280)),
+ transforms.ToTensor(),
+ transforms.Normalize([0.5, 0.5, 0.5], [0.5, 0.5, 0.5])]),
+ 'transform_inference': transforms.Compose([
+ transforms.Resize((320, 320)),
+ augmentations.BilinearResize(factors=factors),
+ transforms.Resize((320, 320)),
+ transforms.ToTensor(),
+ transforms.Normalize([0.5, 0.5, 0.5], [0.5, 0.5, 0.5])])
+ }
+ return transforms_dict
+
+
+class SuperResTransforms_320(TransformsConfig):
+
+ def __init__(self, opts):
+ super(SuperResTransforms_320, self).__init__(opts)
+
+ def get_transforms(self):
+ if self.opts.resize_factors is None:
+ self.opts.resize_factors = '1,2,4,8,16,32'
+ factors = [int(f) for f in self.opts.resize_factors.split(",")]
+ print("Performing down-sampling with factors: {}".format(factors))
+ transforms_dict = {
+ 'transform_gt_train': transforms.Compose([
+ transforms.Resize((320, 320)),
+ transforms.ToTensor(),
+ transforms.Normalize([0.5, 0.5, 0.5], [0.5, 0.5, 0.5])]),
+ 'transform_source': transforms.Compose([
+ transforms.Resize((320, 320)),
+ augmentations.BilinearResize(factors=factors),
+ transforms.Resize((320, 320)),
+ transforms.ToTensor(),
+ transforms.Normalize([0.5, 0.5, 0.5], [0.5, 0.5, 0.5])]),
+ 'transform_test': transforms.Compose([
+ transforms.Resize((320, 320)),
+ transforms.ToTensor(),
+ transforms.Normalize([0.5, 0.5, 0.5], [0.5, 0.5, 0.5])]),
+ 'transform_inference': transforms.Compose([
+ transforms.Resize((320, 320)),
+ augmentations.BilinearResize(factors=factors),
+ transforms.Resize((320, 320)),
+ transforms.ToTensor(),
+ transforms.Normalize([0.5, 0.5, 0.5], [0.5, 0.5, 0.5])])
+ }
+ return transforms_dict
+
+
+class ToonifyTransforms(TransformsConfig):
+
+ def __init__(self, opts):
+ super(ToonifyTransforms, self).__init__(opts)
+
+ def get_transforms(self):
+ transforms_dict = {
+ 'transform_gt_train': transforms.Compose([
+ transforms.Resize((1024, 1024)),
+ transforms.ToTensor(),
+ transforms.Normalize([0.5, 0.5, 0.5], [0.5, 0.5, 0.5])]),
+ 'transform_source': transforms.Compose([
+ transforms.Resize((256, 256)),
+ transforms.ToTensor(),
+ transforms.Normalize([0.5, 0.5, 0.5], [0.5, 0.5, 0.5])]),
+ 'transform_test': transforms.Compose([
+ transforms.Resize((1024, 1024)),
+ transforms.ToTensor(),
+ transforms.Normalize([0.5, 0.5, 0.5], [0.5, 0.5, 0.5])]),
+ 'transform_inference': transforms.Compose([
+ transforms.Resize((256, 256)),
+ transforms.ToTensor(),
+ transforms.Normalize([0.5, 0.5, 0.5], [0.5, 0.5, 0.5])])
+ }
+ return transforms_dict
+
+class EditingTransforms(TransformsConfig):
+
+ def __init__(self, opts):
+ super(EditingTransforms, self).__init__(opts)
+
+ def get_transforms(self):
+ transforms_dict = {
+ 'transform_gt_train': transforms.Compose([
+ transforms.Resize((1280, 1280)),
+ transforms.ToTensor(),
+ transforms.Normalize([0.5, 0.5, 0.5], [0.5, 0.5, 0.5])]),
+ 'transform_source': transforms.Compose([
+ transforms.Resize((320, 320)),
+ transforms.ToTensor(),
+ transforms.Normalize([0.5, 0.5, 0.5], [0.5, 0.5, 0.5])]),
+ 'transform_test': transforms.Compose([
+ transforms.Resize((1280, 1280)),
+ transforms.ToTensor(),
+ transforms.Normalize([0.5, 0.5, 0.5], [0.5, 0.5, 0.5])]),
+ 'transform_inference': transforms.Compose([
+ transforms.Resize((320, 320)),
+ transforms.ToTensor(),
+ transforms.Normalize([0.5, 0.5, 0.5], [0.5, 0.5, 0.5])])
+ }
+ return transforms_dict
\ No newline at end of file
diff --git a/datasets/__init__.py b/datasets/__init__.py
new file mode 100644
index 0000000000000000000000000000000000000000..e69de29bb2d1d6434b8b29ae775ad8c2e48c5391
diff --git a/datasets/augmentations.py b/datasets/augmentations.py
new file mode 100644
index 0000000000000000000000000000000000000000..2e0507f155fa32a463b9bd4b2f50099fd1866df0
--- /dev/null
+++ b/datasets/augmentations.py
@@ -0,0 +1,110 @@
+import numpy as np
+import torch
+from torch import nn
+from torch.nn import functional as F
+from torchvision import transforms
+
+
+class ToOneHot(object):
+ """ Convert the input PIL image to a one-hot torch tensor """
+ def __init__(self, n_classes=None):
+ self.n_classes = n_classes
+
+ def onehot_initialization(self, a):
+ if self.n_classes is None:
+ self.n_classes = len(np.unique(a))
+ out = np.zeros(a.shape + (self.n_classes, ), dtype=int)
+ out[self.__all_idx(a, axis=2)] = 1
+ return out
+
+ def __all_idx(self, idx, axis):
+ grid = np.ogrid[tuple(map(slice, idx.shape))]
+ grid.insert(axis, idx)
+ return tuple(grid)
+
+ def __call__(self, img):
+ img = np.array(img)
+ one_hot = self.onehot_initialization(img)
+ return one_hot
+
+
+class BilinearResize(object):
+ def __init__(self, factors=[1, 2, 4, 8, 16, 32]):
+ self.factors = factors
+
+ def __call__(self, image):
+ factor = np.random.choice(self.factors, size=1)[0]
+ D = BicubicDownSample(factor=factor, cuda=False)
+ img_tensor = transforms.ToTensor()(image).unsqueeze(0)
+ img_tensor_lr = D(img_tensor)[0].clamp(0, 1)
+ img_low_res = transforms.ToPILImage()(img_tensor_lr)
+ return img_low_res
+
+
+class BicubicDownSample(nn.Module):
+ def bicubic_kernel(self, x, a=-0.50):
+ """
+ This equation is exactly copied from the website below:
+ https://clouard.users.greyc.fr/Pantheon/experiments/rescaling/index-en.html#bicubic
+ """
+ abs_x = torch.abs(x)
+ if abs_x <= 1.:
+ return (a + 2.) * torch.pow(abs_x, 3.) - (a + 3.) * torch.pow(abs_x, 2.) + 1
+ elif 1. < abs_x < 2.:
+ return a * torch.pow(abs_x, 3) - 5. * a * torch.pow(abs_x, 2.) + 8. * a * abs_x - 4. * a
+ else:
+ return 0.0
+
+ def __init__(self, factor=4, cuda=True, padding='reflect'):
+ super().__init__()
+ self.factor = factor
+ size = factor * 4
+ k = torch.tensor([self.bicubic_kernel((i - torch.floor(torch.tensor(size / 2)) + 0.5) / factor)
+ for i in range(size)], dtype=torch.float32)
+ k = k / torch.sum(k)
+ k1 = torch.reshape(k, shape=(1, 1, size, 1))
+ self.k1 = torch.cat([k1, k1, k1], dim=0)
+ k2 = torch.reshape(k, shape=(1, 1, 1, size))
+ self.k2 = torch.cat([k2, k2, k2], dim=0)
+ self.cuda = '.cuda' if cuda else ''
+ self.padding = padding
+ for param in self.parameters():
+ param.requires_grad = False
+
+ def forward(self, x, nhwc=False, clip_round=False, byte_output=False):
+ filter_height = self.factor * 4
+ filter_width = self.factor * 4
+ stride = self.factor
+
+ pad_along_height = max(filter_height - stride, 0)
+ pad_along_width = max(filter_width - stride, 0)
+ filters1 = self.k1.type('torch{}.FloatTensor'.format(self.cuda))
+ filters2 = self.k2.type('torch{}.FloatTensor'.format(self.cuda))
+
+ # compute actual padding values for each side
+ pad_top = pad_along_height // 2
+ pad_bottom = pad_along_height - pad_top
+ pad_left = pad_along_width // 2
+ pad_right = pad_along_width - pad_left
+
+ # apply mirror padding
+ if nhwc:
+ x = torch.transpose(torch.transpose(x, 2, 3), 1, 2) # NHWC to NCHW
+
+ # downscaling performed by 1-d convolution
+ x = F.pad(x, (0, 0, pad_top, pad_bottom), self.padding)
+ x = F.conv2d(input=x, weight=filters1, stride=(stride, 1), groups=3)
+ if clip_round:
+ x = torch.clamp(torch.round(x), 0.0, 255.)
+
+ x = F.pad(x, (pad_left, pad_right, 0, 0), self.padding)
+ x = F.conv2d(input=x, weight=filters2, stride=(1, stride), groups=3)
+ if clip_round:
+ x = torch.clamp(torch.round(x), 0.0, 255.)
+
+ if nhwc:
+ x = torch.transpose(torch.transpose(x, 1, 3), 1, 2)
+ if byte_output:
+ return x.type('torch.ByteTensor'.format(self.cuda))
+ else:
+ return x
diff --git a/datasets/ffhq_degradation_dataset.py b/datasets/ffhq_degradation_dataset.py
new file mode 100644
index 0000000000000000000000000000000000000000..b43ff6b1d82c1c491900f119a62f259ac4294b61
--- /dev/null
+++ b/datasets/ffhq_degradation_dataset.py
@@ -0,0 +1,235 @@
+import cv2
+import math
+import numpy as np
+import os.path as osp
+import torch
+import torch.utils.data as data
+from basicsr.data import degradations as degradations
+from basicsr.data.data_util import paths_from_folder
+from basicsr.data.transforms import augment
+from basicsr.utils import FileClient, get_root_logger, imfrombytes, img2tensor
+from basicsr.utils.registry import DATASET_REGISTRY
+from torchvision.transforms.functional import (adjust_brightness, adjust_contrast, adjust_hue, adjust_saturation,
+ normalize)
+
+
+@DATASET_REGISTRY.register()
+class FFHQDegradationDataset(data.Dataset):
+ """FFHQ dataset for GFPGAN.
+ It reads high resolution images, and then generate low-quality (LQ) images on-the-fly.
+ Args:
+ opt (dict): Config for train datasets. It contains the following keys:
+ dataroot_gt (str): Data root path for gt.
+ io_backend (dict): IO backend type and other kwarg.
+ mean (list | tuple): Image mean.
+ std (list | tuple): Image std.
+ use_hflip (bool): Whether to horizontally flip.
+ Please see more options in the codes.
+ """
+
+ def __init__(self, opt):
+ super(FFHQDegradationDataset, self).__init__()
+ self.opt = opt
+ # file client (io backend)
+ self.file_client = None
+ self.io_backend_opt = opt['io_backend']
+
+ self.gt_folder = opt['dataroot_gt']
+ self.mean = opt['mean']
+ self.std = opt['std']
+ self.out_size = opt['out_size']
+
+ self.crop_components = opt.get('crop_components', False) # facial components
+ self.eye_enlarge_ratio = opt.get('eye_enlarge_ratio', 1) # whether enlarge eye regions
+
+ if self.crop_components:
+ # load component list from a pre-process pth files
+ self.components_list = torch.load(opt.get('component_path'))
+
+ # file client (lmdb io backend)
+ if self.io_backend_opt['type'] == 'lmdb':
+ self.io_backend_opt['db_paths'] = self.gt_folder
+ if not self.gt_folder.endswith('.lmdb'):
+ raise ValueError(f"'dataroot_gt' should end with '.lmdb', but received {self.gt_folder}")
+ with open(osp.join(self.gt_folder, 'meta_info.txt')) as fin:
+ self.paths = [line.split('.')[0] for line in fin]
+ else:
+ # disk backend: scan file list from a folder
+ self.paths = paths_from_folder(self.gt_folder)
+
+ # degradation configurations
+ self.blur_kernel_size = opt['blur_kernel_size']
+ self.kernel_list = opt['kernel_list']
+ self.kernel_prob = opt['kernel_prob']
+ self.blur_sigma = opt['blur_sigma']
+ self.downsample_range = opt['downsample_range']
+ self.noise_range = opt['noise_range']
+ self.jpeg_range = opt['jpeg_range']
+
+ # color jitter
+ self.color_jitter_prob = opt.get('color_jitter_prob')
+ self.color_jitter_pt_prob = opt.get('color_jitter_pt_prob')
+ self.color_jitter_shift = opt.get('color_jitter_shift', 20)
+ # to gray
+ self.gray_prob = opt.get('gray_prob')
+
+ logger = get_root_logger()
+ logger.info(f'Blur: blur_kernel_size {self.blur_kernel_size}, sigma: [{", ".join(map(str, self.blur_sigma))}]')
+ logger.info(f'Downsample: downsample_range [{", ".join(map(str, self.downsample_range))}]')
+ logger.info(f'Noise: [{", ".join(map(str, self.noise_range))}]')
+ logger.info(f'JPEG compression: [{", ".join(map(str, self.jpeg_range))}]')
+
+ if self.color_jitter_prob is not None:
+ logger.info(f'Use random color jitter. Prob: {self.color_jitter_prob}, shift: {self.color_jitter_shift}')
+ if self.gray_prob is not None:
+ logger.info(f'Use random gray. Prob: {self.gray_prob}')
+ self.color_jitter_shift /= 255.
+
+ @staticmethod
+ def color_jitter(img, shift):
+ """jitter color: randomly jitter the RGB values, in numpy formats"""
+ jitter_val = np.random.uniform(-shift, shift, 3).astype(np.float32)
+ img = img + jitter_val
+ img = np.clip(img, 0, 1)
+ return img
+
+ @staticmethod
+ def color_jitter_pt(img, brightness, contrast, saturation, hue):
+ """jitter color: randomly jitter the brightness, contrast, saturation, and hue, in torch Tensor formats"""
+ fn_idx = torch.randperm(4)
+ for fn_id in fn_idx:
+ if fn_id == 0 and brightness is not None:
+ brightness_factor = torch.tensor(1.0).uniform_(brightness[0], brightness[1]).item()
+ img = adjust_brightness(img, brightness_factor)
+
+ if fn_id == 1 and contrast is not None:
+ contrast_factor = torch.tensor(1.0).uniform_(contrast[0], contrast[1]).item()
+ img = adjust_contrast(img, contrast_factor)
+
+ if fn_id == 2 and saturation is not None:
+ saturation_factor = torch.tensor(1.0).uniform_(saturation[0], saturation[1]).item()
+ img = adjust_saturation(img, saturation_factor)
+
+ if fn_id == 3 and hue is not None:
+ hue_factor = torch.tensor(1.0).uniform_(hue[0], hue[1]).item()
+ img = adjust_hue(img, hue_factor)
+ return img
+
+ def get_component_coordinates(self, index, status):
+ """Get facial component (left_eye, right_eye, mouth) coordinates from a pre-loaded pth file"""
+ components_bbox = self.components_list[f'{index:08d}']
+ if status[0]: # hflip
+ # exchange right and left eye
+ tmp = components_bbox['left_eye']
+ components_bbox['left_eye'] = components_bbox['right_eye']
+ components_bbox['right_eye'] = tmp
+ # modify the width coordinate
+ components_bbox['left_eye'][0] = self.out_size - components_bbox['left_eye'][0]
+ components_bbox['right_eye'][0] = self.out_size - components_bbox['right_eye'][0]
+ components_bbox['mouth'][0] = self.out_size - components_bbox['mouth'][0]
+
+ # get coordinates
+ locations = []
+ for part in ['left_eye', 'right_eye', 'mouth']:
+ mean = components_bbox[part][0:2]
+ mean[0] = mean[0] * 2 + 128 ########
+ mean[1] = mean[1] * 2 + 128 ########
+ half_len = components_bbox[part][2] * 2 ########
+ if 'eye' in part:
+ half_len *= self.eye_enlarge_ratio
+ loc = np.hstack((mean - half_len + 1, mean + half_len))
+ loc = torch.from_numpy(loc).float()
+ locations.append(loc)
+ return locations
+
+ def __getitem__(self, index):
+ if self.file_client is None:
+ self.file_client = FileClient(self.io_backend_opt.pop('type'), **self.io_backend_opt)
+
+ # load gt image
+ # Shape: (h, w, c); channel order: BGR; image range: [0, 1], float32.
+ gt_path = self.paths[index]
+ img_bytes = self.file_client.get(gt_path)
+ img_gt = imfrombytes(img_bytes, float32=True)
+
+ # random horizontal flip
+ img_gt, status = augment(img_gt, hflip=self.opt['use_hflip'], rotation=False, return_status=True)
+ h, w, _ = img_gt.shape
+
+ # get facial component coordinates
+ if self.crop_components:
+ locations = self.get_component_coordinates(index, status)
+ loc_left_eye, loc_right_eye, loc_mouth = locations
+
+ # ------------------------ generate lq image ------------------------ #
+ # blur
+ kernel = degradations.random_mixed_kernels(
+ self.kernel_list,
+ self.kernel_prob,
+ self.blur_kernel_size,
+ self.blur_sigma,
+ self.blur_sigma, [-math.pi, math.pi],
+ noise_range=None)
+ img_lq = cv2.filter2D(img_gt, -1, kernel)
+ # downsample
+ scale = np.random.uniform(self.downsample_range[0], self.downsample_range[1])
+ img_lq = cv2.resize(img_lq, (int(w // scale), int(h // scale)), interpolation=cv2.INTER_LINEAR)
+ # noise
+ if self.noise_range is not None:
+ img_lq = degradations.random_add_gaussian_noise(img_lq, self.noise_range)
+ # jpeg compression
+ if self.jpeg_range is not None:
+ img_lq = degradations.random_add_jpg_compression(img_lq, self.jpeg_range)
+
+ # resize to original size
+ img_lq = cv2.resize(img_lq, (int(w // self.opt['scale']), int(h // self.opt['scale'])), interpolation=cv2.INTER_LINEAR)
+
+ # random color jitter (only for lq)
+ if self.color_jitter_prob is not None and (np.random.uniform() < self.color_jitter_prob):
+ img_lq = self.color_jitter(img_lq, self.color_jitter_shift)
+ # random to gray (only for lq)
+ if self.gray_prob and np.random.uniform() < self.gray_prob:
+ img_lq = cv2.cvtColor(img_lq, cv2.COLOR_BGR2GRAY)
+ img_lq = np.tile(img_lq[:, :, None], [1, 1, 3])
+ if self.opt.get('gt_gray'): # whether convert GT to gray images
+ img_gt = cv2.cvtColor(img_gt, cv2.COLOR_BGR2GRAY)
+ img_gt = np.tile(img_gt[:, :, None], [1, 1, 3]) # repeat the color channels
+
+ # BGR to RGB, HWC to CHW, numpy to tensor
+ #img_gt, img_lq = img2tensor([img_gt, img_lq], bgr2rgb=True, float32=True)
+ img_gt = img2tensor(img_gt, bgr2rgb=True, float32=True)
+ img_lq = img2tensor(img_lq, bgr2rgb=True, float32=True)
+
+ # random color jitter (pytorch version) (only for lq)
+ if self.color_jitter_pt_prob is not None and (np.random.uniform() < self.color_jitter_pt_prob):
+ brightness = self.opt.get('brightness', (0.5, 1.5))
+ contrast = self.opt.get('contrast', (0.5, 1.5))
+ saturation = self.opt.get('saturation', (0, 1.5))
+ hue = self.opt.get('hue', (-0.1, 0.1))
+ img_lq = self.color_jitter_pt(img_lq, brightness, contrast, saturation, hue)
+
+ # round and clip
+ img_lq = torch.clamp((img_lq * 255.0).round(), 0, 255) / 255.
+
+ # normalize
+ normalize(img_gt, self.mean, self.std, inplace=True)
+ normalize(img_lq, self.mean, self.std, inplace=True)
+
+ '''
+ if self.crop_components:
+ return_dict = {
+ 'lq': img_lq,
+ 'gt': img_gt,
+ 'gt_path': gt_path,
+ 'loc_left_eye': loc_left_eye,
+ 'loc_right_eye': loc_right_eye,
+ 'loc_mouth': loc_mouth
+ }
+ return return_dict
+ else:
+ return {'lq': img_lq, 'gt': img_gt, 'gt_path': gt_path}
+ '''
+ return img_lq, img_gt
+
+ def __len__(self):
+ return len(self.paths)
\ No newline at end of file
diff --git a/datasets/gt_res_dataset.py b/datasets/gt_res_dataset.py
new file mode 100644
index 0000000000000000000000000000000000000000..8892efabcfad7b902c5d49e4b496001241e7ed99
--- /dev/null
+++ b/datasets/gt_res_dataset.py
@@ -0,0 +1,32 @@
+#!/usr/bin/python
+# encoding: utf-8
+import os
+from torch.utils.data import Dataset
+from PIL import Image
+
+
+class GTResDataset(Dataset):
+
+ def __init__(self, root_path, gt_dir=None, transform=None, transform_train=None):
+ self.pairs = []
+ for f in os.listdir(root_path):
+ image_path = os.path.join(root_path, f)
+ gt_path = os.path.join(gt_dir, f)
+ if f.endswith(".jpg") or f.endswith(".png"):
+ self.pairs.append([image_path, gt_path.replace('.png', '.jpg'), None])
+ self.transform = transform
+ self.transform_train = transform_train
+
+ def __len__(self):
+ return len(self.pairs)
+
+ def __getitem__(self, index):
+ from_path, to_path, _ = self.pairs[index]
+ from_im = Image.open(from_path).convert('RGB')
+ to_im = Image.open(to_path).convert('RGB')
+
+ if self.transform:
+ to_im = self.transform(to_im)
+ from_im = self.transform(from_im)
+
+ return from_im, to_im
diff --git a/datasets/images_dataset.py b/datasets/images_dataset.py
new file mode 100644
index 0000000000000000000000000000000000000000..62bb3e3eb85f3841696bac02fa5fb217488a43cd
--- /dev/null
+++ b/datasets/images_dataset.py
@@ -0,0 +1,33 @@
+from torch.utils.data import Dataset
+from PIL import Image
+from utils import data_utils
+
+
+class ImagesDataset(Dataset):
+
+ def __init__(self, source_root, target_root, opts, target_transform=None, source_transform=None):
+ self.source_paths = sorted(data_utils.make_dataset(source_root))
+ self.target_paths = sorted(data_utils.make_dataset(target_root))
+ self.source_transform = source_transform
+ self.target_transform = target_transform
+ self.opts = opts
+
+ def __len__(self):
+ return len(self.source_paths)
+
+ def __getitem__(self, index):
+ from_path = self.source_paths[index]
+ from_im = Image.open(from_path)
+ from_im = from_im.convert('RGB') if self.opts.label_nc == 0 else from_im.convert('L')
+
+ to_path = self.target_paths[index]
+ to_im = Image.open(to_path).convert('RGB')
+ if self.target_transform:
+ to_im = self.target_transform(to_im)
+
+ if self.source_transform:
+ from_im = self.source_transform(from_im)
+ else:
+ from_im = to_im
+
+ return from_im, to_im
diff --git a/datasets/inference_dataset.py b/datasets/inference_dataset.py
new file mode 100644
index 0000000000000000000000000000000000000000..de457349b0726932176f21814c61e34f15955bb7
--- /dev/null
+++ b/datasets/inference_dataset.py
@@ -0,0 +1,22 @@
+from torch.utils.data import Dataset
+from PIL import Image
+from utils import data_utils
+
+
+class InferenceDataset(Dataset):
+
+ def __init__(self, root, opts, transform=None):
+ self.paths = sorted(data_utils.make_dataset(root))
+ self.transform = transform
+ self.opts = opts
+
+ def __len__(self):
+ return len(self.paths)
+
+ def __getitem__(self, index):
+ from_path = self.paths[index]
+ from_im = Image.open(from_path)
+ from_im = from_im.convert('RGB') if self.opts.label_nc == 0 else from_im.convert('L')
+ if self.transform:
+ from_im = self.transform(from_im)
+ return from_im
diff --git a/latent_optimization.py b/latent_optimization.py
new file mode 100644
index 0000000000000000000000000000000000000000..a29a5cbd1e31ed14f95f37601a2b6956bb7de803
--- /dev/null
+++ b/latent_optimization.py
@@ -0,0 +1,107 @@
+import models.stylegan2.lpips as lpips
+from torch import autograd, optim
+from torchvision import transforms, utils
+from tqdm import tqdm
+import torch
+from scripts.align_all_parallel import align_face
+from utils.inference_utils import noise_regularize, noise_normalize_, get_lr, latent_noise, visualize
+
+def latent_optimization(frame, pspex, landmarkpredictor, step=500, device='cuda'):
+ percept = lpips.PerceptualLoss(
+ model="net-lin", net="vgg", use_gpu=device.startswith("cuda")
+ )
+
+ transform = transforms.Compose([
+ transforms.ToTensor(),
+ transforms.Normalize(mean=[0.5, 0.5, 0.5],std=[0.5,0.5,0.5]),
+ ])
+
+ with torch.no_grad():
+
+ noise_sample = torch.randn(1000, 512, device=device)
+ latent_out = pspex.decoder.style(noise_sample)
+ latent_mean = latent_out.mean(0)
+ latent_std = ((latent_out - latent_mean).pow(2).sum() / 1000) ** 0.5
+
+ y = transform(frame).unsqueeze(dim=0).to(device)
+ I_ = align_face(frame, landmarkpredictor)
+ I_ = transform(I_).unsqueeze(dim=0).to(device)
+ wplus = pspex.encoder(I_) + pspex.latent_avg.unsqueeze(0)
+ _, f = pspex.encoder(y, return_feat=True)
+ latent_in = wplus.detach().clone()
+ feat = [f[0].detach().clone(), f[1].detach().clone()]
+
+
+
+ # wplus and f to optimize
+ latent_in.requires_grad = True
+ feat[0].requires_grad = True
+ feat[1].requires_grad = True
+
+ noises_single = pspex.decoder.make_noise()
+ basic_height, basic_width = int(y.shape[2]*32/256), int(y.shape[3]*32/256)
+ noises = []
+ for noise in noises_single:
+ noises.append(noise.new_empty(y.shape[0], 1, max(basic_height, int(y.shape[2]*noise.shape[2]/256)),
+ max(basic_width, int(y.shape[3]*noise.shape[2]/256))).normal_())
+ for noise in noises:
+ noise.requires_grad = True
+
+ init_lr=0.05
+ optimizer = optim.Adam(feat + noises, lr=init_lr)
+ optimizer2 = optim.Adam([latent_in], lr=init_lr)
+ noise_weight = 0.05 * 0.2
+
+ pbar = tqdm(range(step))
+ latent_path = []
+
+ for i in pbar:
+ t = i / step
+ lr = get_lr(t, init_lr)
+ optimizer.param_groups[0]["lr"] = lr
+ optimizer2.param_groups[0]["lr"] = get_lr(t, init_lr)
+
+ noise_strength = latent_std * noise_weight * max(0, 1 - t / 0.75) ** 2
+ latent_n = latent_noise(latent_in, noise_strength.item())
+
+ y_hat, _ = pspex.decoder([latent_n], input_is_latent=True, randomize_noise=False,
+ first_layer_feature=feat, noise=noises)
+
+
+ batch, channel, height, width = y_hat.shape
+
+ if height > y.shape[2]:
+ factor = height // y.shape[2]
+
+ y_hat = y_hat.reshape(
+ batch, channel, height // factor, factor, width // factor, factor
+ )
+ y_hat = y_hat.mean([3, 5])
+
+ p_loss = percept(y_hat, y).sum()
+ n_loss = noise_regularize(noises) * 1e3
+
+ loss = p_loss + n_loss
+
+ optimizer.zero_grad()
+ optimizer2.zero_grad()
+ loss.backward()
+ optimizer.step()
+ optimizer2.step()
+
+ noise_normalize_(noises)
+
+ ''' for visualization
+ if (i + 1) % 100 == 0 or i == 0:
+ viz = torch.cat((y_hat,y,y_hat-y), dim=3)
+ visualize(torch.clamp(viz[0].cpu(),-1,1), 60)
+ '''
+
+ pbar.set_description(
+ (
+ f"perceptual: {p_loss.item():.4f}; noise regularize: {n_loss.item():.4f};"
+ f" lr: {lr:.4f}"
+ )
+ )
+
+ return latent_n, feat, noises, wplus, f
\ No newline at end of file
diff --git a/models/__init__.py b/models/__init__.py
new file mode 100644
index 0000000000000000000000000000000000000000..e69de29bb2d1d6434b8b29ae775ad8c2e48c5391
diff --git a/models/bisenet/LICENSE b/models/bisenet/LICENSE
new file mode 100644
index 0000000000000000000000000000000000000000..bfae0b0c29f885a118e382b445b6eaeca0d3b3e6
--- /dev/null
+++ b/models/bisenet/LICENSE
@@ -0,0 +1,21 @@
+MIT License
+
+Copyright (c) 2019 zll
+
+Permission is hereby granted, free of charge, to any person obtaining a copy
+of this software and associated documentation files (the "Software"), to deal
+in the Software without restriction, including without limitation the rights
+to use, copy, modify, merge, publish, distribute, sublicense, and/or sell
+copies of the Software, and to permit persons to whom the Software is
+furnished to do so, subject to the following conditions:
+
+The above copyright notice and this permission notice shall be included in all
+copies or substantial portions of the Software.
+
+THE SOFTWARE IS PROVIDED "AS IS", WITHOUT WARRANTY OF ANY KIND, EXPRESS OR
+IMPLIED, INCLUDING BUT NOT LIMITED TO THE WARRANTIES OF MERCHANTABILITY,
+FITNESS FOR A PARTICULAR PURPOSE AND NONINFRINGEMENT. IN NO EVENT SHALL THE
+AUTHORS OR COPYRIGHT HOLDERS BE LIABLE FOR ANY CLAIM, DAMAGES OR OTHER
+LIABILITY, WHETHER IN AN ACTION OF CONTRACT, TORT OR OTHERWISE, ARISING FROM,
+OUT OF OR IN CONNECTION WITH THE SOFTWARE OR THE USE OR OTHER DEALINGS IN THE
+SOFTWARE.
diff --git a/models/bisenet/README.md b/models/bisenet/README.md
new file mode 100644
index 0000000000000000000000000000000000000000..849d55e2789c8852e01707d1ff755dc74e63a7f5
--- /dev/null
+++ b/models/bisenet/README.md
@@ -0,0 +1,68 @@
+# face-parsing.PyTorch
+
+
+
+
+
+
+
+### Contents
+- [Training](#training)
+- [Demo](#Demo)
+- [References](#references)
+
+## Training
+
+1. Prepare training data:
+ -- download [CelebAMask-HQ dataset](https://github.com/switchablenorms/CelebAMask-HQ)
+
+ -- change file path in the `prepropess_data.py` and run
+```Shell
+python prepropess_data.py
+```
+
+2. Train the model using CelebAMask-HQ dataset:
+Just run the train script:
+```
+ $ CUDA_VISIBLE_DEVICES=0,1 python -m torch.distributed.launch --nproc_per_node=2 train.py
+```
+
+If you do not wish to train the model, you can download [our pre-trained model](https://drive.google.com/open?id=154JgKpzCPW82qINcVieuPH3fZ2e0P812) and save it in `res/cp`.
+
+
+## Demo
+1. Evaluate the trained model using:
+```Shell
+# evaluate using GPU
+python test.py
+```
+
+## Face makeup using parsing maps
+[**face-makeup.PyTorch**](https://github.com/zllrunning/face-makeup.PyTorch)
+
+
+
+ |
+Hair |
+Lip |
+
+
+
+
+Original Input |
+ |
+ |
+
+
+
+
+Color |
+ |
+ |
+
+
+
+
+
+## References
+- [BiSeNet](https://github.com/CoinCheung/BiSeNet)
\ No newline at end of file
diff --git a/models/bisenet/model.py b/models/bisenet/model.py
new file mode 100644
index 0000000000000000000000000000000000000000..1d2a16ca7533c7b92c600c4dddb89f5f68191d4f
--- /dev/null
+++ b/models/bisenet/model.py
@@ -0,0 +1,283 @@
+#!/usr/bin/python
+# -*- encoding: utf-8 -*-
+
+
+import torch
+import torch.nn as nn
+import torch.nn.functional as F
+import torchvision
+
+from models.bisenet.resnet import Resnet18
+# from modules.bn import InPlaceABNSync as BatchNorm2d
+
+
+class ConvBNReLU(nn.Module):
+ def __init__(self, in_chan, out_chan, ks=3, stride=1, padding=1, *args, **kwargs):
+ super(ConvBNReLU, self).__init__()
+ self.conv = nn.Conv2d(in_chan,
+ out_chan,
+ kernel_size = ks,
+ stride = stride,
+ padding = padding,
+ bias = False)
+ self.bn = nn.BatchNorm2d(out_chan)
+ self.init_weight()
+
+ def forward(self, x):
+ x = self.conv(x)
+ x = F.relu(self.bn(x))
+ return x
+
+ def init_weight(self):
+ for ly in self.children():
+ if isinstance(ly, nn.Conv2d):
+ nn.init.kaiming_normal_(ly.weight, a=1)
+ if not ly.bias is None: nn.init.constant_(ly.bias, 0)
+
+class BiSeNetOutput(nn.Module):
+ def __init__(self, in_chan, mid_chan, n_classes, *args, **kwargs):
+ super(BiSeNetOutput, self).__init__()
+ self.conv = ConvBNReLU(in_chan, mid_chan, ks=3, stride=1, padding=1)
+ self.conv_out = nn.Conv2d(mid_chan, n_classes, kernel_size=1, bias=False)
+ self.init_weight()
+
+ def forward(self, x):
+ x = self.conv(x)
+ x = self.conv_out(x)
+ return x
+
+ def init_weight(self):
+ for ly in self.children():
+ if isinstance(ly, nn.Conv2d):
+ nn.init.kaiming_normal_(ly.weight, a=1)
+ if not ly.bias is None: nn.init.constant_(ly.bias, 0)
+
+ def get_params(self):
+ wd_params, nowd_params = [], []
+ for name, module in self.named_modules():
+ if isinstance(module, nn.Linear) or isinstance(module, nn.Conv2d):
+ wd_params.append(module.weight)
+ if not module.bias is None:
+ nowd_params.append(module.bias)
+ elif isinstance(module, nn.BatchNorm2d):
+ nowd_params += list(module.parameters())
+ return wd_params, nowd_params
+
+
+class AttentionRefinementModule(nn.Module):
+ def __init__(self, in_chan, out_chan, *args, **kwargs):
+ super(AttentionRefinementModule, self).__init__()
+ self.conv = ConvBNReLU(in_chan, out_chan, ks=3, stride=1, padding=1)
+ self.conv_atten = nn.Conv2d(out_chan, out_chan, kernel_size= 1, bias=False)
+ self.bn_atten = nn.BatchNorm2d(out_chan)
+ self.sigmoid_atten = nn.Sigmoid()
+ self.init_weight()
+
+ def forward(self, x):
+ feat = self.conv(x)
+ atten = F.avg_pool2d(feat, feat.size()[2:])
+ atten = self.conv_atten(atten)
+ atten = self.bn_atten(atten)
+ atten = self.sigmoid_atten(atten)
+ out = torch.mul(feat, atten)
+ return out
+
+ def init_weight(self):
+ for ly in self.children():
+ if isinstance(ly, nn.Conv2d):
+ nn.init.kaiming_normal_(ly.weight, a=1)
+ if not ly.bias is None: nn.init.constant_(ly.bias, 0)
+
+
+class ContextPath(nn.Module):
+ def __init__(self, *args, **kwargs):
+ super(ContextPath, self).__init__()
+ self.resnet = Resnet18()
+ self.arm16 = AttentionRefinementModule(256, 128)
+ self.arm32 = AttentionRefinementModule(512, 128)
+ self.conv_head32 = ConvBNReLU(128, 128, ks=3, stride=1, padding=1)
+ self.conv_head16 = ConvBNReLU(128, 128, ks=3, stride=1, padding=1)
+ self.conv_avg = ConvBNReLU(512, 128, ks=1, stride=1, padding=0)
+
+ self.init_weight()
+
+ def forward(self, x):
+ H0, W0 = x.size()[2:]
+ feat8, feat16, feat32 = self.resnet(x)
+ H8, W8 = feat8.size()[2:]
+ H16, W16 = feat16.size()[2:]
+ H32, W32 = feat32.size()[2:]
+
+ avg = F.avg_pool2d(feat32, feat32.size()[2:])
+ avg = self.conv_avg(avg)
+ avg_up = F.interpolate(avg, (H32, W32), mode='nearest')
+
+ feat32_arm = self.arm32(feat32)
+ feat32_sum = feat32_arm + avg_up
+ feat32_up = F.interpolate(feat32_sum, (H16, W16), mode='nearest')
+ feat32_up = self.conv_head32(feat32_up)
+
+ feat16_arm = self.arm16(feat16)
+ feat16_sum = feat16_arm + feat32_up
+ feat16_up = F.interpolate(feat16_sum, (H8, W8), mode='nearest')
+ feat16_up = self.conv_head16(feat16_up)
+
+ return feat8, feat16_up, feat32_up # x8, x8, x16
+
+ def init_weight(self):
+ for ly in self.children():
+ if isinstance(ly, nn.Conv2d):
+ nn.init.kaiming_normal_(ly.weight, a=1)
+ if not ly.bias is None: nn.init.constant_(ly.bias, 0)
+
+ def get_params(self):
+ wd_params, nowd_params = [], []
+ for name, module in self.named_modules():
+ if isinstance(module, (nn.Linear, nn.Conv2d)):
+ wd_params.append(module.weight)
+ if not module.bias is None:
+ nowd_params.append(module.bias)
+ elif isinstance(module, nn.BatchNorm2d):
+ nowd_params += list(module.parameters())
+ return wd_params, nowd_params
+
+
+### This is not used, since I replace this with the resnet feature with the same size
+class SpatialPath(nn.Module):
+ def __init__(self, *args, **kwargs):
+ super(SpatialPath, self).__init__()
+ self.conv1 = ConvBNReLU(3, 64, ks=7, stride=2, padding=3)
+ self.conv2 = ConvBNReLU(64, 64, ks=3, stride=2, padding=1)
+ self.conv3 = ConvBNReLU(64, 64, ks=3, stride=2, padding=1)
+ self.conv_out = ConvBNReLU(64, 128, ks=1, stride=1, padding=0)
+ self.init_weight()
+
+ def forward(self, x):
+ feat = self.conv1(x)
+ feat = self.conv2(feat)
+ feat = self.conv3(feat)
+ feat = self.conv_out(feat)
+ return feat
+
+ def init_weight(self):
+ for ly in self.children():
+ if isinstance(ly, nn.Conv2d):
+ nn.init.kaiming_normal_(ly.weight, a=1)
+ if not ly.bias is None: nn.init.constant_(ly.bias, 0)
+
+ def get_params(self):
+ wd_params, nowd_params = [], []
+ for name, module in self.named_modules():
+ if isinstance(module, nn.Linear) or isinstance(module, nn.Conv2d):
+ wd_params.append(module.weight)
+ if not module.bias is None:
+ nowd_params.append(module.bias)
+ elif isinstance(module, nn.BatchNorm2d):
+ nowd_params += list(module.parameters())
+ return wd_params, nowd_params
+
+
+class FeatureFusionModule(nn.Module):
+ def __init__(self, in_chan, out_chan, *args, **kwargs):
+ super(FeatureFusionModule, self).__init__()
+ self.convblk = ConvBNReLU(in_chan, out_chan, ks=1, stride=1, padding=0)
+ self.conv1 = nn.Conv2d(out_chan,
+ out_chan//4,
+ kernel_size = 1,
+ stride = 1,
+ padding = 0,
+ bias = False)
+ self.conv2 = nn.Conv2d(out_chan//4,
+ out_chan,
+ kernel_size = 1,
+ stride = 1,
+ padding = 0,
+ bias = False)
+ self.relu = nn.ReLU(inplace=True)
+ self.sigmoid = nn.Sigmoid()
+ self.init_weight()
+
+ def forward(self, fsp, fcp):
+ fcat = torch.cat([fsp, fcp], dim=1)
+ feat = self.convblk(fcat)
+ atten = F.avg_pool2d(feat, feat.size()[2:])
+ atten = self.conv1(atten)
+ atten = self.relu(atten)
+ atten = self.conv2(atten)
+ atten = self.sigmoid(atten)
+ feat_atten = torch.mul(feat, atten)
+ feat_out = feat_atten + feat
+ return feat_out
+
+ def init_weight(self):
+ for ly in self.children():
+ if isinstance(ly, nn.Conv2d):
+ nn.init.kaiming_normal_(ly.weight, a=1)
+ if not ly.bias is None: nn.init.constant_(ly.bias, 0)
+
+ def get_params(self):
+ wd_params, nowd_params = [], []
+ for name, module in self.named_modules():
+ if isinstance(module, nn.Linear) or isinstance(module, nn.Conv2d):
+ wd_params.append(module.weight)
+ if not module.bias is None:
+ nowd_params.append(module.bias)
+ elif isinstance(module, nn.BatchNorm2d):
+ nowd_params += list(module.parameters())
+ return wd_params, nowd_params
+
+
+class BiSeNet(nn.Module):
+ def __init__(self, n_classes, *args, **kwargs):
+ super(BiSeNet, self).__init__()
+ self.cp = ContextPath()
+ ## here self.sp is deleted
+ self.ffm = FeatureFusionModule(256, 256)
+ self.conv_out = BiSeNetOutput(256, 256, n_classes)
+ self.conv_out16 = BiSeNetOutput(128, 64, n_classes)
+ self.conv_out32 = BiSeNetOutput(128, 64, n_classes)
+ self.init_weight()
+
+ def forward(self, x):
+ H, W = x.size()[2:]
+ feat_res8, feat_cp8, feat_cp16 = self.cp(x) # here return res3b1 feature
+ feat_sp = feat_res8 # use res3b1 feature to replace spatial path feature
+ feat_fuse = self.ffm(feat_sp, feat_cp8)
+
+ feat_out = self.conv_out(feat_fuse)
+ feat_out16 = self.conv_out16(feat_cp8)
+ feat_out32 = self.conv_out32(feat_cp16)
+
+ feat_out = F.interpolate(feat_out, (H, W), mode='bilinear', align_corners=True)
+ feat_out16 = F.interpolate(feat_out16, (H, W), mode='bilinear', align_corners=True)
+ feat_out32 = F.interpolate(feat_out32, (H, W), mode='bilinear', align_corners=True)
+ return feat_out, feat_out16, feat_out32
+
+ def init_weight(self):
+ for ly in self.children():
+ if isinstance(ly, nn.Conv2d):
+ nn.init.kaiming_normal_(ly.weight, a=1)
+ if not ly.bias is None: nn.init.constant_(ly.bias, 0)
+
+ def get_params(self):
+ wd_params, nowd_params, lr_mul_wd_params, lr_mul_nowd_params = [], [], [], []
+ for name, child in self.named_children():
+ child_wd_params, child_nowd_params = child.get_params()
+ if isinstance(child, FeatureFusionModule) or isinstance(child, BiSeNetOutput):
+ lr_mul_wd_params += child_wd_params
+ lr_mul_nowd_params += child_nowd_params
+ else:
+ wd_params += child_wd_params
+ nowd_params += child_nowd_params
+ return wd_params, nowd_params, lr_mul_wd_params, lr_mul_nowd_params
+
+
+if __name__ == "__main__":
+ net = BiSeNet(19)
+ net.cuda()
+ net.eval()
+ in_ten = torch.randn(16, 3, 640, 480).cuda()
+ out, out16, out32 = net(in_ten)
+ print(out.shape)
+
+ net.get_params()
diff --git a/models/bisenet/resnet.py b/models/bisenet/resnet.py
new file mode 100644
index 0000000000000000000000000000000000000000..aa2bf95130e9815ba378cb6f73207068b81a04b9
--- /dev/null
+++ b/models/bisenet/resnet.py
@@ -0,0 +1,109 @@
+#!/usr/bin/python
+# -*- encoding: utf-8 -*-
+
+import torch
+import torch.nn as nn
+import torch.nn.functional as F
+import torch.utils.model_zoo as modelzoo
+
+# from modules.bn import InPlaceABNSync as BatchNorm2d
+
+resnet18_url = 'https://download.pytorch.org/models/resnet18-5c106cde.pth'
+
+
+def conv3x3(in_planes, out_planes, stride=1):
+ """3x3 convolution with padding"""
+ return nn.Conv2d(in_planes, out_planes, kernel_size=3, stride=stride,
+ padding=1, bias=False)
+
+
+class BasicBlock(nn.Module):
+ def __init__(self, in_chan, out_chan, stride=1):
+ super(BasicBlock, self).__init__()
+ self.conv1 = conv3x3(in_chan, out_chan, stride)
+ self.bn1 = nn.BatchNorm2d(out_chan)
+ self.conv2 = conv3x3(out_chan, out_chan)
+ self.bn2 = nn.BatchNorm2d(out_chan)
+ self.relu = nn.ReLU(inplace=True)
+ self.downsample = None
+ if in_chan != out_chan or stride != 1:
+ self.downsample = nn.Sequential(
+ nn.Conv2d(in_chan, out_chan,
+ kernel_size=1, stride=stride, bias=False),
+ nn.BatchNorm2d(out_chan),
+ )
+
+ def forward(self, x):
+ residual = self.conv1(x)
+ residual = F.relu(self.bn1(residual))
+ residual = self.conv2(residual)
+ residual = self.bn2(residual)
+
+ shortcut = x
+ if self.downsample is not None:
+ shortcut = self.downsample(x)
+
+ out = shortcut + residual
+ out = self.relu(out)
+ return out
+
+
+def create_layer_basic(in_chan, out_chan, bnum, stride=1):
+ layers = [BasicBlock(in_chan, out_chan, stride=stride)]
+ for i in range(bnum-1):
+ layers.append(BasicBlock(out_chan, out_chan, stride=1))
+ return nn.Sequential(*layers)
+
+
+class Resnet18(nn.Module):
+ def __init__(self):
+ super(Resnet18, self).__init__()
+ self.conv1 = nn.Conv2d(3, 64, kernel_size=7, stride=2, padding=3,
+ bias=False)
+ self.bn1 = nn.BatchNorm2d(64)
+ self.maxpool = nn.MaxPool2d(kernel_size=3, stride=2, padding=1)
+ self.layer1 = create_layer_basic(64, 64, bnum=2, stride=1)
+ self.layer2 = create_layer_basic(64, 128, bnum=2, stride=2)
+ self.layer3 = create_layer_basic(128, 256, bnum=2, stride=2)
+ self.layer4 = create_layer_basic(256, 512, bnum=2, stride=2)
+ self.init_weight()
+
+ def forward(self, x):
+ x = self.conv1(x)
+ x = F.relu(self.bn1(x))
+ x = self.maxpool(x)
+
+ x = self.layer1(x)
+ feat8 = self.layer2(x) # 1/8
+ feat16 = self.layer3(feat8) # 1/16
+ feat32 = self.layer4(feat16) # 1/32
+ return feat8, feat16, feat32
+
+ def init_weight(self):
+ state_dict = modelzoo.load_url(resnet18_url)
+ self_state_dict = self.state_dict()
+ for k, v in state_dict.items():
+ if 'fc' in k: continue
+ self_state_dict.update({k: v})
+ self.load_state_dict(self_state_dict)
+
+ def get_params(self):
+ wd_params, nowd_params = [], []
+ for name, module in self.named_modules():
+ if isinstance(module, (nn.Linear, nn.Conv2d)):
+ wd_params.append(module.weight)
+ if not module.bias is None:
+ nowd_params.append(module.bias)
+ elif isinstance(module, nn.BatchNorm2d):
+ nowd_params += list(module.parameters())
+ return wd_params, nowd_params
+
+
+if __name__ == "__main__":
+ net = Resnet18()
+ x = torch.randn(16, 3, 224, 224)
+ out = net(x)
+ print(out[0].size())
+ print(out[1].size())
+ print(out[2].size())
+ net.get_params()
diff --git a/models/encoders/__init__.py b/models/encoders/__init__.py
new file mode 100644
index 0000000000000000000000000000000000000000..e69de29bb2d1d6434b8b29ae775ad8c2e48c5391
diff --git a/models/encoders/helpers.py b/models/encoders/helpers.py
new file mode 100644
index 0000000000000000000000000000000000000000..b51fdf97141407fcc1c9d249a086ddbfd042469f
--- /dev/null
+++ b/models/encoders/helpers.py
@@ -0,0 +1,119 @@
+from collections import namedtuple
+import torch
+from torch.nn import Conv2d, BatchNorm2d, PReLU, ReLU, Sigmoid, MaxPool2d, AdaptiveAvgPool2d, Sequential, Module
+
+"""
+ArcFace implementation from [TreB1eN](https://github.com/TreB1eN/InsightFace_Pytorch)
+"""
+
+
+class Flatten(Module):
+ def forward(self, input):
+ return input.view(input.size(0), -1)
+
+
+def l2_norm(input, axis=1):
+ norm = torch.norm(input, 2, axis, True)
+ output = torch.div(input, norm)
+ return output
+
+
+class Bottleneck(namedtuple('Block', ['in_channel', 'depth', 'stride'])):
+ """ A named tuple describing a ResNet block. """
+
+
+def get_block(in_channel, depth, num_units, stride=2):
+ return [Bottleneck(in_channel, depth, stride)] + [Bottleneck(depth, depth, 1) for i in range(num_units - 1)]
+
+
+def get_blocks(num_layers):
+ if num_layers == 50:
+ blocks = [
+ get_block(in_channel=64, depth=64, num_units=3),
+ get_block(in_channel=64, depth=128, num_units=4),
+ get_block(in_channel=128, depth=256, num_units=14),
+ get_block(in_channel=256, depth=512, num_units=3)
+ ]
+ elif num_layers == 100:
+ blocks = [
+ get_block(in_channel=64, depth=64, num_units=3),
+ get_block(in_channel=64, depth=128, num_units=13),
+ get_block(in_channel=128, depth=256, num_units=30),
+ get_block(in_channel=256, depth=512, num_units=3)
+ ]
+ elif num_layers == 152:
+ blocks = [
+ get_block(in_channel=64, depth=64, num_units=3),
+ get_block(in_channel=64, depth=128, num_units=8),
+ get_block(in_channel=128, depth=256, num_units=36),
+ get_block(in_channel=256, depth=512, num_units=3)
+ ]
+ else:
+ raise ValueError("Invalid number of layers: {}. Must be one of [50, 100, 152]".format(num_layers))
+ return blocks
+
+
+class SEModule(Module):
+ def __init__(self, channels, reduction):
+ super(SEModule, self).__init__()
+ self.avg_pool = AdaptiveAvgPool2d(1)
+ self.fc1 = Conv2d(channels, channels // reduction, kernel_size=1, padding=0, bias=False)
+ self.relu = ReLU(inplace=True)
+ self.fc2 = Conv2d(channels // reduction, channels, kernel_size=1, padding=0, bias=False)
+ self.sigmoid = Sigmoid()
+
+ def forward(self, x):
+ module_input = x
+ x = self.avg_pool(x)
+ x = self.fc1(x)
+ x = self.relu(x)
+ x = self.fc2(x)
+ x = self.sigmoid(x)
+ return module_input * x
+
+
+class bottleneck_IR(Module):
+ def __init__(self, in_channel, depth, stride):
+ super(bottleneck_IR, self).__init__()
+ if in_channel == depth:
+ self.shortcut_layer = MaxPool2d(1, stride)
+ else:
+ self.shortcut_layer = Sequential(
+ Conv2d(in_channel, depth, (1, 1), stride, bias=False),
+ BatchNorm2d(depth)
+ )
+ self.res_layer = Sequential(
+ BatchNorm2d(in_channel),
+ Conv2d(in_channel, depth, (3, 3), (1, 1), 1, bias=False), PReLU(depth),
+ Conv2d(depth, depth, (3, 3), stride, 1, bias=False), BatchNorm2d(depth)
+ )
+
+ def forward(self, x):
+ shortcut = self.shortcut_layer(x)
+ res = self.res_layer(x)
+ return res + shortcut
+
+
+class bottleneck_IR_SE(Module):
+ def __init__(self, in_channel, depth, stride):
+ super(bottleneck_IR_SE, self).__init__()
+ if in_channel == depth:
+ self.shortcut_layer = MaxPool2d(1, stride)
+ else:
+ self.shortcut_layer = Sequential(
+ Conv2d(in_channel, depth, (1, 1), stride, bias=False),
+ BatchNorm2d(depth)
+ )
+ self.res_layer = Sequential(
+ BatchNorm2d(in_channel),
+ Conv2d(in_channel, depth, (3, 3), (1, 1), 1, bias=False),
+ PReLU(depth),
+ Conv2d(depth, depth, (3, 3), stride, 1, bias=False),
+ BatchNorm2d(depth),
+ SEModule(depth, 16)
+ )
+
+ def forward(self, x):
+ shortcut = self.shortcut_layer(x)
+ res = self.res_layer(x)
+ return res + shortcut
diff --git a/models/encoders/model_irse.py b/models/encoders/model_irse.py
new file mode 100644
index 0000000000000000000000000000000000000000..bc41ace0ba04cf4285c283a28e6c36113a18e6d6
--- /dev/null
+++ b/models/encoders/model_irse.py
@@ -0,0 +1,84 @@
+from torch.nn import Linear, Conv2d, BatchNorm1d, BatchNorm2d, PReLU, Dropout, Sequential, Module
+from models.encoders.helpers import get_blocks, Flatten, bottleneck_IR, bottleneck_IR_SE, l2_norm
+
+"""
+Modified Backbone implementation from [TreB1eN](https://github.com/TreB1eN/InsightFace_Pytorch)
+"""
+
+
+class Backbone(Module):
+ def __init__(self, input_size, num_layers, mode='ir', drop_ratio=0.4, affine=True):
+ super(Backbone, self).__init__()
+ assert input_size in [112, 224], "input_size should be 112 or 224"
+ assert num_layers in [50, 100, 152], "num_layers should be 50, 100 or 152"
+ assert mode in ['ir', 'ir_se'], "mode should be ir or ir_se"
+ blocks = get_blocks(num_layers)
+ if mode == 'ir':
+ unit_module = bottleneck_IR
+ elif mode == 'ir_se':
+ unit_module = bottleneck_IR_SE
+ self.input_layer = Sequential(Conv2d(3, 64, (3, 3), 1, 1, bias=False),
+ BatchNorm2d(64),
+ PReLU(64))
+ if input_size == 112:
+ self.output_layer = Sequential(BatchNorm2d(512),
+ Dropout(drop_ratio),
+ Flatten(),
+ Linear(512 * 7 * 7, 512),
+ BatchNorm1d(512, affine=affine))
+ else:
+ self.output_layer = Sequential(BatchNorm2d(512),
+ Dropout(drop_ratio),
+ Flatten(),
+ Linear(512 * 14 * 14, 512),
+ BatchNorm1d(512, affine=affine))
+
+ modules = []
+ for block in blocks:
+ for bottleneck in block:
+ modules.append(unit_module(bottleneck.in_channel,
+ bottleneck.depth,
+ bottleneck.stride))
+ self.body = Sequential(*modules)
+
+ def forward(self, x):
+ x = self.input_layer(x)
+ x = self.body(x)
+ x = self.output_layer(x)
+ return l2_norm(x)
+
+
+def IR_50(input_size):
+ """Constructs a ir-50 model."""
+ model = Backbone(input_size, num_layers=50, mode='ir', drop_ratio=0.4, affine=False)
+ return model
+
+
+def IR_101(input_size):
+ """Constructs a ir-101 model."""
+ model = Backbone(input_size, num_layers=100, mode='ir', drop_ratio=0.4, affine=False)
+ return model
+
+
+def IR_152(input_size):
+ """Constructs a ir-152 model."""
+ model = Backbone(input_size, num_layers=152, mode='ir', drop_ratio=0.4, affine=False)
+ return model
+
+
+def IR_SE_50(input_size):
+ """Constructs a ir_se-50 model."""
+ model = Backbone(input_size, num_layers=50, mode='ir_se', drop_ratio=0.4, affine=False)
+ return model
+
+
+def IR_SE_101(input_size):
+ """Constructs a ir_se-101 model."""
+ model = Backbone(input_size, num_layers=100, mode='ir_se', drop_ratio=0.4, affine=False)
+ return model
+
+
+def IR_SE_152(input_size):
+ """Constructs a ir_se-152 model."""
+ model = Backbone(input_size, num_layers=152, mode='ir_se', drop_ratio=0.4, affine=False)
+ return model
diff --git a/models/encoders/psp_encoders.py b/models/encoders/psp_encoders.py
new file mode 100644
index 0000000000000000000000000000000000000000..b8ed6a10130312fa44923db44f953be90936f26d
--- /dev/null
+++ b/models/encoders/psp_encoders.py
@@ -0,0 +1,357 @@
+import numpy as np
+import torch
+import torch.nn.functional as F
+from torch import nn
+from torch.nn import Linear, Conv2d, BatchNorm2d, PReLU, Sequential, Module
+
+from models.encoders.helpers import get_blocks, Flatten, bottleneck_IR, bottleneck_IR_SE
+from models.stylegan2.model import EqualLinear
+
+
+class GradualStyleBlock(Module):
+ def __init__(self, in_c, out_c, spatial, max_pooling=False):
+ super(GradualStyleBlock, self).__init__()
+ self.out_c = out_c
+ self.spatial = spatial
+ self.max_pooling = max_pooling
+ num_pools = int(np.log2(spatial))
+ modules = []
+ modules += [Conv2d(in_c, out_c, kernel_size=3, stride=2, padding=1),
+ nn.LeakyReLU()]
+ for i in range(num_pools - 1):
+ modules += [
+ Conv2d(out_c, out_c, kernel_size=3, stride=2, padding=1),
+ nn.LeakyReLU()
+ ]
+ self.convs = nn.Sequential(*modules)
+ self.linear = EqualLinear(out_c, out_c, lr_mul=1)
+
+ def forward(self, x):
+ x = self.convs(x)
+ # To make E accept more general H*W images, we add global average pooling to
+ # resize all features to 1*1*512 before mapping to latent codes
+ if self.max_pooling:
+ x = F.adaptive_max_pool2d(x, 1) ##### modified
+ else:
+ x = F.adaptive_avg_pool2d(x, 1) ##### modified
+ x = x.view(-1, self.out_c)
+ x = self.linear(x)
+ return x
+
+class AdaptiveInstanceNorm(nn.Module):
+ def __init__(self, fin, style_dim=512):
+ super().__init__()
+
+ self.norm = nn.InstanceNorm2d(fin, affine=False)
+ self.style = nn.Linear(style_dim, fin * 2)
+
+ self.style.bias.data[:fin] = 1
+ self.style.bias.data[fin:] = 0
+
+ def forward(self, input, style):
+ style = self.style(style).unsqueeze(2).unsqueeze(3)
+ gamma, beta = style.chunk(2, 1)
+ out = self.norm(input)
+ out = gamma * out + beta
+ return out
+
+
+class FusionLayer(Module): ##### modified
+ def __init__(self, inchannel, outchannel, use_skip_torgb=True, use_att=0):
+ super(FusionLayer, self).__init__()
+
+ self.transform = nn.Sequential(nn.Conv2d(inchannel, outchannel, kernel_size=3, stride=1, padding=1),
+ nn.LeakyReLU())
+ self.fusion_out = nn.Conv2d(outchannel*2, outchannel, kernel_size=3, stride=1, padding=1)
+ self.fusion_out.weight.data *= 0.01
+ self.fusion_out.weight[:,0:outchannel,1,1].data += torch.eye(outchannel)
+
+ self.use_skip_torgb = use_skip_torgb
+ if use_skip_torgb:
+ self.fusion_skip = nn.Conv2d(3+outchannel, 3, kernel_size=3, stride=1, padding=1)
+ self.fusion_skip.weight.data *= 0.01
+ self.fusion_skip.weight[:,0:3,1,1].data += torch.eye(3)
+
+ self.use_att = use_att
+ if use_att:
+ modules = []
+ modules.append(nn.Linear(512, outchannel))
+ for _ in range(use_att):
+ modules.append(nn.LeakyReLU(negative_slope=0.2, inplace=True))
+ modules.append(nn.Linear(outchannel, outchannel))
+ modules.append(nn.LeakyReLU(negative_slope=0.2, inplace=True))
+ self.linear = Sequential(*modules)
+ self.norm = AdaptiveInstanceNorm(outchannel*2, outchannel)
+ self.conv = nn.Conv2d(outchannel*2, 1, 3, 1, 1, bias=True)
+
+ def forward(self, feat, out, skip, editing_w=None):
+ x = self.transform(feat)
+ # similar to VToonify, use editing vector as condition
+ # fuse encoder feature and decoder feature with a predicted attention mask m_E
+ # if self.use_att = False, just fuse them with a simple conv layer
+ if self.use_att and editing_w is not None:
+ label = self.linear(editing_w)
+ m_E = (F.relu(self.conv(self.norm(torch.cat([out, abs(out-x)], dim=1), label)))).tanh()
+ x = x * m_E
+ out = self.fusion_out(torch.cat((out, x), dim=1))
+ if self.use_skip_torgb:
+ skip = self.fusion_skip(torch.cat((skip, x), dim=1))
+ return out, skip
+
+
+class ResnetBlock(nn.Module):
+ def __init__(self, dim):
+ super(ResnetBlock, self).__init__()
+
+ self.conv_block = nn.Sequential(Conv2d(dim, dim, 3, 1, 1),
+ nn.LeakyReLU(),
+ Conv2d(dim, dim, 3, 1, 1))
+ self.relu = nn.LeakyReLU()
+
+ def forward(self, x):
+ out = x + self.conv_block(x)
+ return self.relu(out)
+
+# trainable light-weight translation network T
+# for sketch/mask-to-face translation,
+# we add a trainable T to map y to an intermediate domain where E can more easily extract features.
+class ResnetGenerator(nn.Module):
+ def __init__(self, in_channel=19, res_num=2):
+ super(ResnetGenerator, self).__init__()
+
+ modules = []
+ modules.append(Conv2d(in_channel, 16, 3, 2, 1))
+ modules.append(nn.LeakyReLU())
+ modules.append(Conv2d(16, 16, 3, 2, 1))
+ modules.append(nn.LeakyReLU())
+ for _ in range(res_num):
+ modules.append(ResnetBlock(16))
+ for _ in range(2):
+ modules.append(nn.ConvTranspose2d(16, 16, 3, 2, 1, output_padding=1))
+ modules.append(nn.LeakyReLU())
+ modules.append(Conv2d(16, 64, 3, 1, 1, bias=False))
+ modules.append(BatchNorm2d(64))
+ modules.append(PReLU(64))
+ self.model = Sequential(*modules)
+
+ def forward(self, input):
+ return self.model(input)
+
+class GradualStyleEncoder(Module):
+ def __init__(self, num_layers, mode='ir', opts=None):
+ super(GradualStyleEncoder, self).__init__()
+ assert num_layers in [50, 100, 152], 'num_layers should be 50,100, or 152'
+ assert mode in ['ir', 'ir_se'], 'mode should be ir or ir_se'
+ blocks = get_blocks(num_layers)
+ if mode == 'ir':
+ unit_module = bottleneck_IR
+ elif mode == 'ir_se':
+ unit_module = bottleneck_IR_SE
+
+ # for sketch/mask-to-face translation, add a new network T
+ if opts.input_nc != 3:
+ self.input_label_layer = ResnetGenerator(opts.input_nc, opts.res_num)
+
+ self.input_layer = Sequential(Conv2d(3, 64, (3, 3), 1, 1, bias=False),
+ BatchNorm2d(64),
+ PReLU(64))
+ modules = []
+ for block in blocks:
+ for bottleneck in block:
+ modules.append(unit_module(bottleneck.in_channel,
+ bottleneck.depth,
+ bottleneck.stride))
+ self.body = Sequential(*modules)
+
+ self.styles = nn.ModuleList()
+ self.style_count = opts.n_styles
+ self.coarse_ind = 3
+ self.middle_ind = 7
+ for i in range(self.style_count):
+ if i < self.coarse_ind:
+ style = GradualStyleBlock(512, 512, 16, 'max_pooling' in opts and opts.max_pooling)
+ elif i < self.middle_ind:
+ style = GradualStyleBlock(512, 512, 32, 'max_pooling' in opts and opts.max_pooling)
+ else:
+ style = GradualStyleBlock(512, 512, 64, 'max_pooling' in opts and opts.max_pooling)
+ self.styles.append(style)
+ self.latlayer1 = nn.Conv2d(256, 512, kernel_size=1, stride=1, padding=0)
+ self.latlayer2 = nn.Conv2d(128, 512, kernel_size=1, stride=1, padding=0)
+
+ # we concatenate pSp features in the middle layers and
+ # add a convolution layer to map the concatenated features to the first-layer input feature f of G.
+ self.featlayer = nn.Conv2d(768, 512, kernel_size=1, stride=1, padding=0) ##### modified
+ self.skiplayer = nn.Conv2d(768, 3, kernel_size=1, stride=1, padding=0) ##### modified
+
+ # skip connection
+ if 'use_skip' in opts and opts.use_skip: ##### modified
+ self.fusion = nn.ModuleList()
+ channels = [[256,512], [256,512], [256,512], [256,512], [128,512], [64,256], [64,128]]
+ # opts.skip_max_layer: how many layers are skipped to the decoder
+ for inc, outc in channels[:max(1, min(7, opts.skip_max_layer))]: # from 4 to 256
+ self.fusion.append(FusionLayer(inc, outc, opts.use_skip_torgb, opts.use_att))
+
+ def _upsample_add(self, x, y):
+ '''Upsample and add two feature maps.
+ Args:
+ x: (Variable) top feature map to be upsampled.
+ y: (Variable) lateral feature map.
+ Returns:
+ (Variable) added feature map.
+ Note in PyTorch, when input size is odd, the upsampled feature map
+ with `F.upsample(..., scale_factor=2, mode='nearest')`
+ maybe not equal to the lateral feature map size.
+ e.g.
+ original input size: [N,_,15,15] ->
+ conv2d feature map size: [N,_,8,8] ->
+ upsampled feature map size: [N,_,16,16]
+ So we choose bilinear upsample which supports arbitrary output sizes.
+ '''
+ _, _, H, W = y.size()
+ return F.interpolate(x, size=(H, W), mode='bilinear', align_corners=True) + y
+
+ # return_feat: return f
+ # return_full: return f and the skipped encoder features
+ # return [out, feats]
+ # out is the style latent code w+
+ # feats[0] is f for the 1st conv layer, feats[1] is f for the 1st torgb layer
+ # feats[2-8] is the skipped encoder features
+ def forward(self, x, return_feat=False, return_full=False): ##### modified
+ if x.shape[1] != 3:
+ x = self.input_label_layer(x)
+ else:
+ x = self.input_layer(x)
+ c256 = x ##### modified
+
+ latents = []
+ modulelist = list(self.body._modules.values())
+ for i, l in enumerate(modulelist):
+ x = l(x)
+ if i == 2: ##### modified
+ c128 = x
+ elif i == 6:
+ c1 = x
+ elif i == 10: ##### modified
+ c21 = x ##### modified
+ elif i == 15: ##### modified
+ c22 = x ##### modified
+ elif i == 20:
+ c2 = x
+ elif i == 23:
+ c3 = x
+
+ for j in range(self.coarse_ind):
+ latents.append(self.styles[j](c3))
+
+ p2 = self._upsample_add(c3, self.latlayer1(c2))
+ for j in range(self.coarse_ind, self.middle_ind):
+ latents.append(self.styles[j](p2))
+
+ p1 = self._upsample_add(p2, self.latlayer2(c1))
+ for j in range(self.middle_ind, self.style_count):
+ latents.append(self.styles[j](p1))
+
+ out = torch.stack(latents, dim=1)
+
+ if not return_feat:
+ return out
+
+ feats = [self.featlayer(torch.cat((c21, c22, c2), dim=1)), self.skiplayer(torch.cat((c21, c22, c2), dim=1))]
+
+ if return_full: ##### modified
+ feats += [c2, c2, c22, c21, c1, c128, c256]
+
+ return out, feats
+
+
+ # only compute the first-layer feature f
+ # E_F in the paper
+ def get_feat(self, x): ##### modified
+ # for sketch/mask-to-face translation
+ # use a trainable light-weight translation network T
+ if x.shape[1] != 3:
+ x = self.input_label_layer(x)
+ else:
+ x = self.input_layer(x)
+
+ latents = []
+ modulelist = list(self.body._modules.values())
+ for i, l in enumerate(modulelist):
+ x = l(x)
+ if i == 10: ##### modified
+ c21 = x ##### modified
+ elif i == 15: ##### modified
+ c22 = x ##### modified
+ elif i == 20:
+ c2 = x
+ break
+ return self.featlayer(torch.cat((c21, c22, c2), dim=1))
+
+class BackboneEncoderUsingLastLayerIntoW(Module):
+ def __init__(self, num_layers, mode='ir', opts=None):
+ super(BackboneEncoderUsingLastLayerIntoW, self).__init__()
+ print('Using BackboneEncoderUsingLastLayerIntoW')
+ assert num_layers in [50, 100, 152], 'num_layers should be 50,100, or 152'
+ assert mode in ['ir', 'ir_se'], 'mode should be ir or ir_se'
+ blocks = get_blocks(num_layers)
+ if mode == 'ir':
+ unit_module = bottleneck_IR
+ elif mode == 'ir_se':
+ unit_module = bottleneck_IR_SE
+ self.input_layer = Sequential(Conv2d(opts.input_nc, 64, (3, 3), 1, 1, bias=False),
+ BatchNorm2d(64),
+ PReLU(64))
+ self.output_pool = torch.nn.AdaptiveAvgPool2d((1, 1))
+ self.linear = EqualLinear(512, 512, lr_mul=1)
+ modules = []
+ for block in blocks:
+ for bottleneck in block:
+ modules.append(unit_module(bottleneck.in_channel,
+ bottleneck.depth,
+ bottleneck.stride))
+ self.body = Sequential(*modules)
+
+ def forward(self, x):
+ x = self.input_layer(x)
+ x = self.body(x)
+ x = self.output_pool(x)
+ x = x.view(-1, 512)
+ x = self.linear(x)
+ return x
+
+
+class BackboneEncoderUsingLastLayerIntoWPlus(Module):
+ def __init__(self, num_layers, mode='ir', opts=None):
+ super(BackboneEncoderUsingLastLayerIntoWPlus, self).__init__()
+ print('Using BackboneEncoderUsingLastLayerIntoWPlus')
+ assert num_layers in [50, 100, 152], 'num_layers should be 50,100, or 152'
+ assert mode in ['ir', 'ir_se'], 'mode should be ir or ir_se'
+ blocks = get_blocks(num_layers)
+ if mode == 'ir':
+ unit_module = bottleneck_IR
+ elif mode == 'ir_se':
+ unit_module = bottleneck_IR_SE
+ self.n_styles = opts.n_styles
+ self.input_layer = Sequential(Conv2d(opts.input_nc, 64, (3, 3), 1, 1, bias=False),
+ BatchNorm2d(64),
+ PReLU(64))
+ self.output_layer_2 = Sequential(BatchNorm2d(512),
+ torch.nn.AdaptiveAvgPool2d((7, 7)),
+ Flatten(),
+ Linear(512 * 7 * 7, 512))
+ self.linear = EqualLinear(512, 512 * self.n_styles, lr_mul=1)
+ modules = []
+ for block in blocks:
+ for bottleneck in block:
+ modules.append(unit_module(bottleneck.in_channel,
+ bottleneck.depth,
+ bottleneck.stride))
+ self.body = Sequential(*modules)
+
+ def forward(self, x):
+ x = self.input_layer(x)
+ x = self.body(x)
+ x = self.output_layer_2(x)
+ x = self.linear(x)
+ x = x.view(-1, self.n_styles, 512)
+ return x
diff --git a/models/mtcnn/__init__.py b/models/mtcnn/__init__.py
new file mode 100644
index 0000000000000000000000000000000000000000..e69de29bb2d1d6434b8b29ae775ad8c2e48c5391
diff --git a/models/mtcnn/mtcnn.py b/models/mtcnn/mtcnn.py
new file mode 100644
index 0000000000000000000000000000000000000000..4deacabaaf35e315c363c9eada9ff0c41f2561e5
--- /dev/null
+++ b/models/mtcnn/mtcnn.py
@@ -0,0 +1,156 @@
+import numpy as np
+import torch
+from PIL import Image
+from models.mtcnn.mtcnn_pytorch.src.get_nets import PNet, RNet, ONet
+from models.mtcnn.mtcnn_pytorch.src.box_utils import nms, calibrate_box, get_image_boxes, convert_to_square
+from models.mtcnn.mtcnn_pytorch.src.first_stage import run_first_stage
+from models.mtcnn.mtcnn_pytorch.src.align_trans import get_reference_facial_points, warp_and_crop_face
+
+device = 'cuda:0'
+
+
+class MTCNN():
+ def __init__(self):
+ print(device)
+ self.pnet = PNet().to(device)
+ self.rnet = RNet().to(device)
+ self.onet = ONet().to(device)
+ self.pnet.eval()
+ self.rnet.eval()
+ self.onet.eval()
+ self.refrence = get_reference_facial_points(default_square=True)
+
+ def align(self, img):
+ _, landmarks = self.detect_faces(img)
+ if len(landmarks) == 0:
+ return None, None
+ facial5points = [[landmarks[0][j], landmarks[0][j + 5]] for j in range(5)]
+ warped_face, tfm = warp_and_crop_face(np.array(img), facial5points, self.refrence, crop_size=(112, 112))
+ return Image.fromarray(warped_face), tfm
+
+ def align_multi(self, img, limit=None, min_face_size=30.0):
+ boxes, landmarks = self.detect_faces(img, min_face_size)
+ if limit:
+ boxes = boxes[:limit]
+ landmarks = landmarks[:limit]
+ faces = []
+ tfms = []
+ for landmark in landmarks:
+ facial5points = [[landmark[j], landmark[j + 5]] for j in range(5)]
+ warped_face, tfm = warp_and_crop_face(np.array(img), facial5points, self.refrence, crop_size=(112, 112))
+ faces.append(Image.fromarray(warped_face))
+ tfms.append(tfm)
+ return boxes, faces, tfms
+
+ def detect_faces(self, image, min_face_size=20.0,
+ thresholds=[0.15, 0.25, 0.35],
+ nms_thresholds=[0.7, 0.7, 0.7]):
+ """
+ Arguments:
+ image: an instance of PIL.Image.
+ min_face_size: a float number.
+ thresholds: a list of length 3.
+ nms_thresholds: a list of length 3.
+
+ Returns:
+ two float numpy arrays of shapes [n_boxes, 4] and [n_boxes, 10],
+ bounding boxes and facial landmarks.
+ """
+
+ # BUILD AN IMAGE PYRAMID
+ width, height = image.size
+ min_length = min(height, width)
+
+ min_detection_size = 12
+ factor = 0.707 # sqrt(0.5)
+
+ # scales for scaling the image
+ scales = []
+
+ # scales the image so that
+ # minimum size that we can detect equals to
+ # minimum face size that we want to detect
+ m = min_detection_size / min_face_size
+ min_length *= m
+
+ factor_count = 0
+ while min_length > min_detection_size:
+ scales.append(m * factor ** factor_count)
+ min_length *= factor
+ factor_count += 1
+
+ # STAGE 1
+
+ # it will be returned
+ bounding_boxes = []
+
+ with torch.no_grad():
+ # run P-Net on different scales
+ for s in scales:
+ boxes = run_first_stage(image, self.pnet, scale=s, threshold=thresholds[0])
+ bounding_boxes.append(boxes)
+
+ # collect boxes (and offsets, and scores) from different scales
+ bounding_boxes = [i for i in bounding_boxes if i is not None]
+ bounding_boxes = np.vstack(bounding_boxes)
+
+ keep = nms(bounding_boxes[:, 0:5], nms_thresholds[0])
+ bounding_boxes = bounding_boxes[keep]
+
+ # use offsets predicted by pnet to transform bounding boxes
+ bounding_boxes = calibrate_box(bounding_boxes[:, 0:5], bounding_boxes[:, 5:])
+ # shape [n_boxes, 5]
+
+ bounding_boxes = convert_to_square(bounding_boxes)
+ bounding_boxes[:, 0:4] = np.round(bounding_boxes[:, 0:4])
+
+ # STAGE 2
+
+ img_boxes = get_image_boxes(bounding_boxes, image, size=24)
+ img_boxes = torch.FloatTensor(img_boxes).to(device)
+
+ output = self.rnet(img_boxes)
+ offsets = output[0].cpu().data.numpy() # shape [n_boxes, 4]
+ probs = output[1].cpu().data.numpy() # shape [n_boxes, 2]
+
+ keep = np.where(probs[:, 1] > thresholds[1])[0]
+ bounding_boxes = bounding_boxes[keep]
+ bounding_boxes[:, 4] = probs[keep, 1].reshape((-1,))
+ offsets = offsets[keep]
+
+ keep = nms(bounding_boxes, nms_thresholds[1])
+ bounding_boxes = bounding_boxes[keep]
+ bounding_boxes = calibrate_box(bounding_boxes, offsets[keep])
+ bounding_boxes = convert_to_square(bounding_boxes)
+ bounding_boxes[:, 0:4] = np.round(bounding_boxes[:, 0:4])
+
+ # STAGE 3
+
+ img_boxes = get_image_boxes(bounding_boxes, image, size=48)
+ if len(img_boxes) == 0:
+ return [], []
+ img_boxes = torch.FloatTensor(img_boxes).to(device)
+ output = self.onet(img_boxes)
+ landmarks = output[0].cpu().data.numpy() # shape [n_boxes, 10]
+ offsets = output[1].cpu().data.numpy() # shape [n_boxes, 4]
+ probs = output[2].cpu().data.numpy() # shape [n_boxes, 2]
+
+ keep = np.where(probs[:, 1] > thresholds[2])[0]
+ bounding_boxes = bounding_boxes[keep]
+ bounding_boxes[:, 4] = probs[keep, 1].reshape((-1,))
+ offsets = offsets[keep]
+ landmarks = landmarks[keep]
+
+ # compute landmark points
+ width = bounding_boxes[:, 2] - bounding_boxes[:, 0] + 1.0
+ height = bounding_boxes[:, 3] - bounding_boxes[:, 1] + 1.0
+ xmin, ymin = bounding_boxes[:, 0], bounding_boxes[:, 1]
+ landmarks[:, 0:5] = np.expand_dims(xmin, 1) + np.expand_dims(width, 1) * landmarks[:, 0:5]
+ landmarks[:, 5:10] = np.expand_dims(ymin, 1) + np.expand_dims(height, 1) * landmarks[:, 5:10]
+
+ bounding_boxes = calibrate_box(bounding_boxes, offsets)
+ keep = nms(bounding_boxes, nms_thresholds[2], mode='min')
+ bounding_boxes = bounding_boxes[keep]
+ landmarks = landmarks[keep]
+
+ return bounding_boxes, landmarks
diff --git a/models/mtcnn/mtcnn_pytorch/__init__.py b/models/mtcnn/mtcnn_pytorch/__init__.py
new file mode 100644
index 0000000000000000000000000000000000000000..e69de29bb2d1d6434b8b29ae775ad8c2e48c5391
diff --git a/models/mtcnn/mtcnn_pytorch/src/__init__.py b/models/mtcnn/mtcnn_pytorch/src/__init__.py
new file mode 100644
index 0000000000000000000000000000000000000000..617ba38c34b1801b2db2e0209b4e886c9d24c490
--- /dev/null
+++ b/models/mtcnn/mtcnn_pytorch/src/__init__.py
@@ -0,0 +1,2 @@
+from .visualization_utils import show_bboxes
+from .detector import detect_faces
diff --git a/models/mtcnn/mtcnn_pytorch/src/align_trans.py b/models/mtcnn/mtcnn_pytorch/src/align_trans.py
new file mode 100644
index 0000000000000000000000000000000000000000..ab5f1df002bc19556ae8a75cabf56310084785a9
--- /dev/null
+++ b/models/mtcnn/mtcnn_pytorch/src/align_trans.py
@@ -0,0 +1,304 @@
+# -*- coding: utf-8 -*-
+"""
+Created on Mon Apr 24 15:43:29 2017
+@author: zhaoy
+"""
+import numpy as np
+import cv2
+
+# from scipy.linalg import lstsq
+# from scipy.ndimage import geometric_transform # , map_coordinates
+
+from models.mtcnn.mtcnn_pytorch.src.matlab_cp2tform import get_similarity_transform_for_cv2
+
+# reference facial points, a list of coordinates (x,y)
+REFERENCE_FACIAL_POINTS = [
+ [30.29459953, 51.69630051],
+ [65.53179932, 51.50139999],
+ [48.02519989, 71.73660278],
+ [33.54930115, 92.3655014],
+ [62.72990036, 92.20410156]
+]
+
+DEFAULT_CROP_SIZE = (96, 112)
+
+
+class FaceWarpException(Exception):
+ def __str__(self):
+ return 'In File {}:{}'.format(
+ __file__, super.__str__(self))
+
+
+def get_reference_facial_points(output_size=None,
+ inner_padding_factor=0.0,
+ outer_padding=(0, 0),
+ default_square=False):
+ """
+ Function:
+ ----------
+ get reference 5 key points according to crop settings:
+ 0. Set default crop_size:
+ if default_square:
+ crop_size = (112, 112)
+ else:
+ crop_size = (96, 112)
+ 1. Pad the crop_size by inner_padding_factor in each side;
+ 2. Resize crop_size into (output_size - outer_padding*2),
+ pad into output_size with outer_padding;
+ 3. Output reference_5point;
+ Parameters:
+ ----------
+ @output_size: (w, h) or None
+ size of aligned face image
+ @inner_padding_factor: (w_factor, h_factor)
+ padding factor for inner (w, h)
+ @outer_padding: (w_pad, h_pad)
+ each row is a pair of coordinates (x, y)
+ @default_square: True or False
+ if True:
+ default crop_size = (112, 112)
+ else:
+ default crop_size = (96, 112);
+ !!! make sure, if output_size is not None:
+ (output_size - outer_padding)
+ = some_scale * (default crop_size * (1.0 + inner_padding_factor))
+ Returns:
+ ----------
+ @reference_5point: 5x2 np.array
+ each row is a pair of transformed coordinates (x, y)
+ """
+ # print('\n===> get_reference_facial_points():')
+
+ # print('---> Params:')
+ # print(' output_size: ', output_size)
+ # print(' inner_padding_factor: ', inner_padding_factor)
+ # print(' outer_padding:', outer_padding)
+ # print(' default_square: ', default_square)
+
+ tmp_5pts = np.array(REFERENCE_FACIAL_POINTS)
+ tmp_crop_size = np.array(DEFAULT_CROP_SIZE)
+
+ # 0) make the inner region a square
+ if default_square:
+ size_diff = max(tmp_crop_size) - tmp_crop_size
+ tmp_5pts += size_diff / 2
+ tmp_crop_size += size_diff
+
+ # print('---> default:')
+ # print(' crop_size = ', tmp_crop_size)
+ # print(' reference_5pts = ', tmp_5pts)
+
+ if (output_size and
+ output_size[0] == tmp_crop_size[0] and
+ output_size[1] == tmp_crop_size[1]):
+ # print('output_size == DEFAULT_CROP_SIZE {}: return default reference points'.format(tmp_crop_size))
+ return tmp_5pts
+
+ if (inner_padding_factor == 0 and
+ outer_padding == (0, 0)):
+ if output_size is None:
+ # print('No paddings to do: return default reference points')
+ return tmp_5pts
+ else:
+ raise FaceWarpException(
+ 'No paddings to do, output_size must be None or {}'.format(tmp_crop_size))
+
+ # check output size
+ if not (0 <= inner_padding_factor <= 1.0):
+ raise FaceWarpException('Not (0 <= inner_padding_factor <= 1.0)')
+
+ if ((inner_padding_factor > 0 or outer_padding[0] > 0 or outer_padding[1] > 0)
+ and output_size is None):
+ output_size = tmp_crop_size * \
+ (1 + inner_padding_factor * 2).astype(np.int32)
+ output_size += np.array(outer_padding)
+ # print(' deduced from paddings, output_size = ', output_size)
+
+ if not (outer_padding[0] < output_size[0]
+ and outer_padding[1] < output_size[1]):
+ raise FaceWarpException('Not (outer_padding[0] < output_size[0]'
+ 'and outer_padding[1] < output_size[1])')
+
+ # 1) pad the inner region according inner_padding_factor
+ # print('---> STEP1: pad the inner region according inner_padding_factor')
+ if inner_padding_factor > 0:
+ size_diff = tmp_crop_size * inner_padding_factor * 2
+ tmp_5pts += size_diff / 2
+ tmp_crop_size += np.round(size_diff).astype(np.int32)
+
+ # print(' crop_size = ', tmp_crop_size)
+ # print(' reference_5pts = ', tmp_5pts)
+
+ # 2) resize the padded inner region
+ # print('---> STEP2: resize the padded inner region')
+ size_bf_outer_pad = np.array(output_size) - np.array(outer_padding) * 2
+ # print(' crop_size = ', tmp_crop_size)
+ # print(' size_bf_outer_pad = ', size_bf_outer_pad)
+
+ if size_bf_outer_pad[0] * tmp_crop_size[1] != size_bf_outer_pad[1] * tmp_crop_size[0]:
+ raise FaceWarpException('Must have (output_size - outer_padding)'
+ '= some_scale * (crop_size * (1.0 + inner_padding_factor)')
+
+ scale_factor = size_bf_outer_pad[0].astype(np.float32) / tmp_crop_size[0]
+ # print(' resize scale_factor = ', scale_factor)
+ tmp_5pts = tmp_5pts * scale_factor
+ # size_diff = tmp_crop_size * (scale_factor - min(scale_factor))
+ # tmp_5pts = tmp_5pts + size_diff / 2
+ tmp_crop_size = size_bf_outer_pad
+ # print(' crop_size = ', tmp_crop_size)
+ # print(' reference_5pts = ', tmp_5pts)
+
+ # 3) add outer_padding to make output_size
+ reference_5point = tmp_5pts + np.array(outer_padding)
+ tmp_crop_size = output_size
+ # print('---> STEP3: add outer_padding to make output_size')
+ # print(' crop_size = ', tmp_crop_size)
+ # print(' reference_5pts = ', tmp_5pts)
+
+ # print('===> end get_reference_facial_points\n')
+
+ return reference_5point
+
+
+def get_affine_transform_matrix(src_pts, dst_pts):
+ """
+ Function:
+ ----------
+ get affine transform matrix 'tfm' from src_pts to dst_pts
+ Parameters:
+ ----------
+ @src_pts: Kx2 np.array
+ source points matrix, each row is a pair of coordinates (x, y)
+ @dst_pts: Kx2 np.array
+ destination points matrix, each row is a pair of coordinates (x, y)
+ Returns:
+ ----------
+ @tfm: 2x3 np.array
+ transform matrix from src_pts to dst_pts
+ """
+
+ tfm = np.float32([[1, 0, 0], [0, 1, 0]])
+ n_pts = src_pts.shape[0]
+ ones = np.ones((n_pts, 1), src_pts.dtype)
+ src_pts_ = np.hstack([src_pts, ones])
+ dst_pts_ = np.hstack([dst_pts, ones])
+
+ # #print(('src_pts_:\n' + str(src_pts_))
+ # #print(('dst_pts_:\n' + str(dst_pts_))
+
+ A, res, rank, s = np.linalg.lstsq(src_pts_, dst_pts_)
+
+ # #print(('np.linalg.lstsq return A: \n' + str(A))
+ # #print(('np.linalg.lstsq return res: \n' + str(res))
+ # #print(('np.linalg.lstsq return rank: \n' + str(rank))
+ # #print(('np.linalg.lstsq return s: \n' + str(s))
+
+ if rank == 3:
+ tfm = np.float32([
+ [A[0, 0], A[1, 0], A[2, 0]],
+ [A[0, 1], A[1, 1], A[2, 1]]
+ ])
+ elif rank == 2:
+ tfm = np.float32([
+ [A[0, 0], A[1, 0], 0],
+ [A[0, 1], A[1, 1], 0]
+ ])
+
+ return tfm
+
+
+def warp_and_crop_face(src_img,
+ facial_pts,
+ reference_pts=None,
+ crop_size=(96, 112),
+ align_type='smilarity'):
+ """
+ Function:
+ ----------
+ apply affine transform 'trans' to uv
+ Parameters:
+ ----------
+ @src_img: 3x3 np.array
+ input image
+ @facial_pts: could be
+ 1)a list of K coordinates (x,y)
+ or
+ 2) Kx2 or 2xK np.array
+ each row or col is a pair of coordinates (x, y)
+ @reference_pts: could be
+ 1) a list of K coordinates (x,y)
+ or
+ 2) Kx2 or 2xK np.array
+ each row or col is a pair of coordinates (x, y)
+ or
+ 3) None
+ if None, use default reference facial points
+ @crop_size: (w, h)
+ output face image size
+ @align_type: transform type, could be one of
+ 1) 'similarity': use similarity transform
+ 2) 'cv2_affine': use the first 3 points to do affine transform,
+ by calling cv2.getAffineTransform()
+ 3) 'affine': use all points to do affine transform
+ Returns:
+ ----------
+ @face_img: output face image with size (w, h) = @crop_size
+ """
+
+ if reference_pts is None:
+ if crop_size[0] == 96 and crop_size[1] == 112:
+ reference_pts = REFERENCE_FACIAL_POINTS
+ else:
+ default_square = False
+ inner_padding_factor = 0
+ outer_padding = (0, 0)
+ output_size = crop_size
+
+ reference_pts = get_reference_facial_points(output_size,
+ inner_padding_factor,
+ outer_padding,
+ default_square)
+
+ ref_pts = np.float32(reference_pts)
+ ref_pts_shp = ref_pts.shape
+ if max(ref_pts_shp) < 3 or min(ref_pts_shp) != 2:
+ raise FaceWarpException(
+ 'reference_pts.shape must be (K,2) or (2,K) and K>2')
+
+ if ref_pts_shp[0] == 2:
+ ref_pts = ref_pts.T
+
+ src_pts = np.float32(facial_pts)
+ src_pts_shp = src_pts.shape
+ if max(src_pts_shp) < 3 or min(src_pts_shp) != 2:
+ raise FaceWarpException(
+ 'facial_pts.shape must be (K,2) or (2,K) and K>2')
+
+ if src_pts_shp[0] == 2:
+ src_pts = src_pts.T
+
+ # #print('--->src_pts:\n', src_pts
+ # #print('--->ref_pts\n', ref_pts
+
+ if src_pts.shape != ref_pts.shape:
+ raise FaceWarpException(
+ 'facial_pts and reference_pts must have the same shape')
+
+ if align_type is 'cv2_affine':
+ tfm = cv2.getAffineTransform(src_pts[0:3], ref_pts[0:3])
+ # #print(('cv2.getAffineTransform() returns tfm=\n' + str(tfm))
+ elif align_type is 'affine':
+ tfm = get_affine_transform_matrix(src_pts, ref_pts)
+ # #print(('get_affine_transform_matrix() returns tfm=\n' + str(tfm))
+ else:
+ tfm = get_similarity_transform_for_cv2(src_pts, ref_pts)
+ # #print(('get_similarity_transform_for_cv2() returns tfm=\n' + str(tfm))
+
+ # #print('--->Transform matrix: '
+ # #print(('type(tfm):' + str(type(tfm)))
+ # #print(('tfm.dtype:' + str(tfm.dtype))
+ # #print( tfm
+
+ face_img = cv2.warpAffine(src_img, tfm, (crop_size[0], crop_size[1]))
+
+ return face_img, tfm
diff --git a/models/mtcnn/mtcnn_pytorch/src/box_utils.py b/models/mtcnn/mtcnn_pytorch/src/box_utils.py
new file mode 100644
index 0000000000000000000000000000000000000000..1e8081b73639a7d70e4391b3d45417569550ddc6
--- /dev/null
+++ b/models/mtcnn/mtcnn_pytorch/src/box_utils.py
@@ -0,0 +1,238 @@
+import numpy as np
+from PIL import Image
+
+
+def nms(boxes, overlap_threshold=0.5, mode='union'):
+ """Non-maximum suppression.
+
+ Arguments:
+ boxes: a float numpy array of shape [n, 5],
+ where each row is (xmin, ymin, xmax, ymax, score).
+ overlap_threshold: a float number.
+ mode: 'union' or 'min'.
+
+ Returns:
+ list with indices of the selected boxes
+ """
+
+ # if there are no boxes, return the empty list
+ if len(boxes) == 0:
+ return []
+
+ # list of picked indices
+ pick = []
+
+ # grab the coordinates of the bounding boxes
+ x1, y1, x2, y2, score = [boxes[:, i] for i in range(5)]
+
+ area = (x2 - x1 + 1.0) * (y2 - y1 + 1.0)
+ ids = np.argsort(score) # in increasing order
+
+ while len(ids) > 0:
+
+ # grab index of the largest value
+ last = len(ids) - 1
+ i = ids[last]
+ pick.append(i)
+
+ # compute intersections
+ # of the box with the largest score
+ # with the rest of boxes
+
+ # left top corner of intersection boxes
+ ix1 = np.maximum(x1[i], x1[ids[:last]])
+ iy1 = np.maximum(y1[i], y1[ids[:last]])
+
+ # right bottom corner of intersection boxes
+ ix2 = np.minimum(x2[i], x2[ids[:last]])
+ iy2 = np.minimum(y2[i], y2[ids[:last]])
+
+ # width and height of intersection boxes
+ w = np.maximum(0.0, ix2 - ix1 + 1.0)
+ h = np.maximum(0.0, iy2 - iy1 + 1.0)
+
+ # intersections' areas
+ inter = w * h
+ if mode == 'min':
+ overlap = inter / np.minimum(area[i], area[ids[:last]])
+ elif mode == 'union':
+ # intersection over union (IoU)
+ overlap = inter / (area[i] + area[ids[:last]] - inter)
+
+ # delete all boxes where overlap is too big
+ ids = np.delete(
+ ids,
+ np.concatenate([[last], np.where(overlap > overlap_threshold)[0]])
+ )
+
+ return pick
+
+
+def convert_to_square(bboxes):
+ """Convert bounding boxes to a square form.
+
+ Arguments:
+ bboxes: a float numpy array of shape [n, 5].
+
+ Returns:
+ a float numpy array of shape [n, 5],
+ squared bounding boxes.
+ """
+
+ square_bboxes = np.zeros_like(bboxes)
+ x1, y1, x2, y2 = [bboxes[:, i] for i in range(4)]
+ h = y2 - y1 + 1.0
+ w = x2 - x1 + 1.0
+ max_side = np.maximum(h, w)
+ square_bboxes[:, 0] = x1 + w * 0.5 - max_side * 0.5
+ square_bboxes[:, 1] = y1 + h * 0.5 - max_side * 0.5
+ square_bboxes[:, 2] = square_bboxes[:, 0] + max_side - 1.0
+ square_bboxes[:, 3] = square_bboxes[:, 1] + max_side - 1.0
+ return square_bboxes
+
+
+def calibrate_box(bboxes, offsets):
+ """Transform bounding boxes to be more like true bounding boxes.
+ 'offsets' is one of the outputs of the nets.
+
+ Arguments:
+ bboxes: a float numpy array of shape [n, 5].
+ offsets: a float numpy array of shape [n, 4].
+
+ Returns:
+ a float numpy array of shape [n, 5].
+ """
+ x1, y1, x2, y2 = [bboxes[:, i] for i in range(4)]
+ w = x2 - x1 + 1.0
+ h = y2 - y1 + 1.0
+ w = np.expand_dims(w, 1)
+ h = np.expand_dims(h, 1)
+
+ # this is what happening here:
+ # tx1, ty1, tx2, ty2 = [offsets[:, i] for i in range(4)]
+ # x1_true = x1 + tx1*w
+ # y1_true = y1 + ty1*h
+ # x2_true = x2 + tx2*w
+ # y2_true = y2 + ty2*h
+ # below is just more compact form of this
+
+ # are offsets always such that
+ # x1 < x2 and y1 < y2 ?
+
+ translation = np.hstack([w, h, w, h]) * offsets
+ bboxes[:, 0:4] = bboxes[:, 0:4] + translation
+ return bboxes
+
+
+def get_image_boxes(bounding_boxes, img, size=24):
+ """Cut out boxes from the image.
+
+ Arguments:
+ bounding_boxes: a float numpy array of shape [n, 5].
+ img: an instance of PIL.Image.
+ size: an integer, size of cutouts.
+
+ Returns:
+ a float numpy array of shape [n, 3, size, size].
+ """
+
+ num_boxes = len(bounding_boxes)
+ width, height = img.size
+
+ [dy, edy, dx, edx, y, ey, x, ex, w, h] = correct_bboxes(bounding_boxes, width, height)
+ img_boxes = np.zeros((num_boxes, 3, size, size), 'float32')
+
+ for i in range(num_boxes):
+ img_box = np.zeros((h[i], w[i], 3), 'uint8')
+
+ img_array = np.asarray(img, 'uint8')
+ img_box[dy[i]:(edy[i] + 1), dx[i]:(edx[i] + 1), :] = \
+ img_array[y[i]:(ey[i] + 1), x[i]:(ex[i] + 1), :]
+
+ # resize
+ img_box = Image.fromarray(img_box)
+ img_box = img_box.resize((size, size), Image.BILINEAR)
+ img_box = np.asarray(img_box, 'float32')
+
+ img_boxes[i, :, :, :] = _preprocess(img_box)
+
+ return img_boxes
+
+
+def correct_bboxes(bboxes, width, height):
+ """Crop boxes that are too big and get coordinates
+ with respect to cutouts.
+
+ Arguments:
+ bboxes: a float numpy array of shape [n, 5],
+ where each row is (xmin, ymin, xmax, ymax, score).
+ width: a float number.
+ height: a float number.
+
+ Returns:
+ dy, dx, edy, edx: a int numpy arrays of shape [n],
+ coordinates of the boxes with respect to the cutouts.
+ y, x, ey, ex: a int numpy arrays of shape [n],
+ corrected ymin, xmin, ymax, xmax.
+ h, w: a int numpy arrays of shape [n],
+ just heights and widths of boxes.
+
+ in the following order:
+ [dy, edy, dx, edx, y, ey, x, ex, w, h].
+ """
+
+ x1, y1, x2, y2 = [bboxes[:, i] for i in range(4)]
+ w, h = x2 - x1 + 1.0, y2 - y1 + 1.0
+ num_boxes = bboxes.shape[0]
+
+ # 'e' stands for end
+ # (x, y) -> (ex, ey)
+ x, y, ex, ey = x1, y1, x2, y2
+
+ # we need to cut out a box from the image.
+ # (x, y, ex, ey) are corrected coordinates of the box
+ # in the image.
+ # (dx, dy, edx, edy) are coordinates of the box in the cutout
+ # from the image.
+ dx, dy = np.zeros((num_boxes,)), np.zeros((num_boxes,))
+ edx, edy = w.copy() - 1.0, h.copy() - 1.0
+
+ # if box's bottom right corner is too far right
+ ind = np.where(ex > width - 1.0)[0]
+ edx[ind] = w[ind] + width - 2.0 - ex[ind]
+ ex[ind] = width - 1.0
+
+ # if box's bottom right corner is too low
+ ind = np.where(ey > height - 1.0)[0]
+ edy[ind] = h[ind] + height - 2.0 - ey[ind]
+ ey[ind] = height - 1.0
+
+ # if box's top left corner is too far left
+ ind = np.where(x < 0.0)[0]
+ dx[ind] = 0.0 - x[ind]
+ x[ind] = 0.0
+
+ # if box's top left corner is too high
+ ind = np.where(y < 0.0)[0]
+ dy[ind] = 0.0 - y[ind]
+ y[ind] = 0.0
+
+ return_list = [dy, edy, dx, edx, y, ey, x, ex, w, h]
+ return_list = [i.astype('int32') for i in return_list]
+
+ return return_list
+
+
+def _preprocess(img):
+ """Preprocessing step before feeding the network.
+
+ Arguments:
+ img: a float numpy array of shape [h, w, c].
+
+ Returns:
+ a float numpy array of shape [1, c, h, w].
+ """
+ img = img.transpose((2, 0, 1))
+ img = np.expand_dims(img, 0)
+ img = (img - 127.5) * 0.0078125
+ return img
diff --git a/models/mtcnn/mtcnn_pytorch/src/detector.py b/models/mtcnn/mtcnn_pytorch/src/detector.py
new file mode 100644
index 0000000000000000000000000000000000000000..b162cff3194cc0114abd1a840e5dc772a55edd25
--- /dev/null
+++ b/models/mtcnn/mtcnn_pytorch/src/detector.py
@@ -0,0 +1,126 @@
+import numpy as np
+import torch
+from torch.autograd import Variable
+from .get_nets import PNet, RNet, ONet
+from .box_utils import nms, calibrate_box, get_image_boxes, convert_to_square
+from .first_stage import run_first_stage
+
+
+def detect_faces(image, min_face_size=20.0,
+ thresholds=[0.6, 0.7, 0.8],
+ nms_thresholds=[0.7, 0.7, 0.7]):
+ """
+ Arguments:
+ image: an instance of PIL.Image.
+ min_face_size: a float number.
+ thresholds: a list of length 3.
+ nms_thresholds: a list of length 3.
+
+ Returns:
+ two float numpy arrays of shapes [n_boxes, 4] and [n_boxes, 10],
+ bounding boxes and facial landmarks.
+ """
+
+ # LOAD MODELS
+ pnet = PNet()
+ rnet = RNet()
+ onet = ONet()
+ onet.eval()
+
+ # BUILD AN IMAGE PYRAMID
+ width, height = image.size
+ min_length = min(height, width)
+
+ min_detection_size = 12
+ factor = 0.707 # sqrt(0.5)
+
+ # scales for scaling the image
+ scales = []
+
+ # scales the image so that
+ # minimum size that we can detect equals to
+ # minimum face size that we want to detect
+ m = min_detection_size / min_face_size
+ min_length *= m
+
+ factor_count = 0
+ while min_length > min_detection_size:
+ scales.append(m * factor ** factor_count)
+ min_length *= factor
+ factor_count += 1
+
+ # STAGE 1
+
+ # it will be returned
+ bounding_boxes = []
+
+ with torch.no_grad():
+ # run P-Net on different scales
+ for s in scales:
+ boxes = run_first_stage(image, pnet, scale=s, threshold=thresholds[0])
+ bounding_boxes.append(boxes)
+
+ # collect boxes (and offsets, and scores) from different scales
+ bounding_boxes = [i for i in bounding_boxes if i is not None]
+ bounding_boxes = np.vstack(bounding_boxes)
+
+ keep = nms(bounding_boxes[:, 0:5], nms_thresholds[0])
+ bounding_boxes = bounding_boxes[keep]
+
+ # use offsets predicted by pnet to transform bounding boxes
+ bounding_boxes = calibrate_box(bounding_boxes[:, 0:5], bounding_boxes[:, 5:])
+ # shape [n_boxes, 5]
+
+ bounding_boxes = convert_to_square(bounding_boxes)
+ bounding_boxes[:, 0:4] = np.round(bounding_boxes[:, 0:4])
+
+ # STAGE 2
+
+ img_boxes = get_image_boxes(bounding_boxes, image, size=24)
+ img_boxes = torch.FloatTensor(img_boxes)
+
+ output = rnet(img_boxes)
+ offsets = output[0].data.numpy() # shape [n_boxes, 4]
+ probs = output[1].data.numpy() # shape [n_boxes, 2]
+
+ keep = np.where(probs[:, 1] > thresholds[1])[0]
+ bounding_boxes = bounding_boxes[keep]
+ bounding_boxes[:, 4] = probs[keep, 1].reshape((-1,))
+ offsets = offsets[keep]
+
+ keep = nms(bounding_boxes, nms_thresholds[1])
+ bounding_boxes = bounding_boxes[keep]
+ bounding_boxes = calibrate_box(bounding_boxes, offsets[keep])
+ bounding_boxes = convert_to_square(bounding_boxes)
+ bounding_boxes[:, 0:4] = np.round(bounding_boxes[:, 0:4])
+
+ # STAGE 3
+
+ img_boxes = get_image_boxes(bounding_boxes, image, size=48)
+ if len(img_boxes) == 0:
+ return [], []
+ img_boxes = torch.FloatTensor(img_boxes)
+ output = onet(img_boxes)
+ landmarks = output[0].data.numpy() # shape [n_boxes, 10]
+ offsets = output[1].data.numpy() # shape [n_boxes, 4]
+ probs = output[2].data.numpy() # shape [n_boxes, 2]
+
+ keep = np.where(probs[:, 1] > thresholds[2])[0]
+ bounding_boxes = bounding_boxes[keep]
+ bounding_boxes[:, 4] = probs[keep, 1].reshape((-1,))
+ offsets = offsets[keep]
+ landmarks = landmarks[keep]
+
+ # compute landmark points
+ width = bounding_boxes[:, 2] - bounding_boxes[:, 0] + 1.0
+ height = bounding_boxes[:, 3] - bounding_boxes[:, 1] + 1.0
+ xmin, ymin = bounding_boxes[:, 0], bounding_boxes[:, 1]
+ landmarks[:, 0:5] = np.expand_dims(xmin, 1) + np.expand_dims(width, 1) * landmarks[:, 0:5]
+ landmarks[:, 5:10] = np.expand_dims(ymin, 1) + np.expand_dims(height, 1) * landmarks[:, 5:10]
+
+ bounding_boxes = calibrate_box(bounding_boxes, offsets)
+ keep = nms(bounding_boxes, nms_thresholds[2], mode='min')
+ bounding_boxes = bounding_boxes[keep]
+ landmarks = landmarks[keep]
+
+ return bounding_boxes, landmarks
diff --git a/models/mtcnn/mtcnn_pytorch/src/first_stage.py b/models/mtcnn/mtcnn_pytorch/src/first_stage.py
new file mode 100644
index 0000000000000000000000000000000000000000..d646f91d5e0348e23bd426701f6afa6000a9b6d1
--- /dev/null
+++ b/models/mtcnn/mtcnn_pytorch/src/first_stage.py
@@ -0,0 +1,101 @@
+import torch
+from torch.autograd import Variable
+import math
+from PIL import Image
+import numpy as np
+from .box_utils import nms, _preprocess
+
+# device = torch.device("cuda:0" if torch.cuda.is_available() else "cpu")
+device = 'cuda:0'
+
+
+def run_first_stage(image, net, scale, threshold):
+ """Run P-Net, generate bounding boxes, and do NMS.
+
+ Arguments:
+ image: an instance of PIL.Image.
+ net: an instance of pytorch's nn.Module, P-Net.
+ scale: a float number,
+ scale width and height of the image by this number.
+ threshold: a float number,
+ threshold on the probability of a face when generating
+ bounding boxes from predictions of the net.
+
+ Returns:
+ a float numpy array of shape [n_boxes, 9],
+ bounding boxes with scores and offsets (4 + 1 + 4).
+ """
+
+ # scale the image and convert it to a float array
+ width, height = image.size
+ sw, sh = math.ceil(width * scale), math.ceil(height * scale)
+ img = image.resize((sw, sh), Image.BILINEAR)
+ img = np.asarray(img, 'float32')
+
+ img = torch.FloatTensor(_preprocess(img)).to(device)
+ with torch.no_grad():
+ output = net(img)
+ probs = output[1].cpu().data.numpy()[0, 1, :, :]
+ offsets = output[0].cpu().data.numpy()
+ # probs: probability of a face at each sliding window
+ # offsets: transformations to true bounding boxes
+
+ boxes = _generate_bboxes(probs, offsets, scale, threshold)
+ if len(boxes) == 0:
+ return None
+
+ keep = nms(boxes[:, 0:5], overlap_threshold=0.5)
+ return boxes[keep]
+
+
+def _generate_bboxes(probs, offsets, scale, threshold):
+ """Generate bounding boxes at places
+ where there is probably a face.
+
+ Arguments:
+ probs: a float numpy array of shape [n, m].
+ offsets: a float numpy array of shape [1, 4, n, m].
+ scale: a float number,
+ width and height of the image were scaled by this number.
+ threshold: a float number.
+
+ Returns:
+ a float numpy array of shape [n_boxes, 9]
+ """
+
+ # applying P-Net is equivalent, in some sense, to
+ # moving 12x12 window with stride 2
+ stride = 2
+ cell_size = 12
+
+ # indices of boxes where there is probably a face
+ inds = np.where(probs > threshold)
+
+ if inds[0].size == 0:
+ return np.array([])
+
+ # transformations of bounding boxes
+ tx1, ty1, tx2, ty2 = [offsets[0, i, inds[0], inds[1]] for i in range(4)]
+ # they are defined as:
+ # w = x2 - x1 + 1
+ # h = y2 - y1 + 1
+ # x1_true = x1 + tx1*w
+ # x2_true = x2 + tx2*w
+ # y1_true = y1 + ty1*h
+ # y2_true = y2 + ty2*h
+
+ offsets = np.array([tx1, ty1, tx2, ty2])
+ score = probs[inds[0], inds[1]]
+
+ # P-Net is applied to scaled images
+ # so we need to rescale bounding boxes back
+ bounding_boxes = np.vstack([
+ np.round((stride * inds[1] + 1.0) / scale),
+ np.round((stride * inds[0] + 1.0) / scale),
+ np.round((stride * inds[1] + 1.0 + cell_size) / scale),
+ np.round((stride * inds[0] + 1.0 + cell_size) / scale),
+ score, offsets
+ ])
+ # why one is added?
+
+ return bounding_boxes.T
diff --git a/models/mtcnn/mtcnn_pytorch/src/get_nets.py b/models/mtcnn/mtcnn_pytorch/src/get_nets.py
new file mode 100644
index 0000000000000000000000000000000000000000..0b5d3cc64734f0d05b19969fda31dc2bff9b18c6
--- /dev/null
+++ b/models/mtcnn/mtcnn_pytorch/src/get_nets.py
@@ -0,0 +1,171 @@
+import torch
+import torch.nn as nn
+import torch.nn.functional as F
+from collections import OrderedDict
+import numpy as np
+
+from configs.paths_config import model_paths
+PNET_PATH = model_paths["mtcnn_pnet"]
+ONET_PATH = model_paths["mtcnn_onet"]
+RNET_PATH = model_paths["mtcnn_rnet"]
+
+
+class Flatten(nn.Module):
+
+ def __init__(self):
+ super(Flatten, self).__init__()
+
+ def forward(self, x):
+ """
+ Arguments:
+ x: a float tensor with shape [batch_size, c, h, w].
+ Returns:
+ a float tensor with shape [batch_size, c*h*w].
+ """
+
+ # without this pretrained model isn't working
+ x = x.transpose(3, 2).contiguous()
+
+ return x.view(x.size(0), -1)
+
+
+class PNet(nn.Module):
+
+ def __init__(self):
+ super().__init__()
+
+ # suppose we have input with size HxW, then
+ # after first layer: H - 2,
+ # after pool: ceil((H - 2)/2),
+ # after second conv: ceil((H - 2)/2) - 2,
+ # after last conv: ceil((H - 2)/2) - 4,
+ # and the same for W
+
+ self.features = nn.Sequential(OrderedDict([
+ ('conv1', nn.Conv2d(3, 10, 3, 1)),
+ ('prelu1', nn.PReLU(10)),
+ ('pool1', nn.MaxPool2d(2, 2, ceil_mode=True)),
+
+ ('conv2', nn.Conv2d(10, 16, 3, 1)),
+ ('prelu2', nn.PReLU(16)),
+
+ ('conv3', nn.Conv2d(16, 32, 3, 1)),
+ ('prelu3', nn.PReLU(32))
+ ]))
+
+ self.conv4_1 = nn.Conv2d(32, 2, 1, 1)
+ self.conv4_2 = nn.Conv2d(32, 4, 1, 1)
+
+ weights = np.load(PNET_PATH, allow_pickle=True)[()]
+ for n, p in self.named_parameters():
+ p.data = torch.FloatTensor(weights[n])
+
+ def forward(self, x):
+ """
+ Arguments:
+ x: a float tensor with shape [batch_size, 3, h, w].
+ Returns:
+ b: a float tensor with shape [batch_size, 4, h', w'].
+ a: a float tensor with shape [batch_size, 2, h', w'].
+ """
+ x = self.features(x)
+ a = self.conv4_1(x)
+ b = self.conv4_2(x)
+ a = F.softmax(a, dim=-1)
+ return b, a
+
+
+class RNet(nn.Module):
+
+ def __init__(self):
+ super().__init__()
+
+ self.features = nn.Sequential(OrderedDict([
+ ('conv1', nn.Conv2d(3, 28, 3, 1)),
+ ('prelu1', nn.PReLU(28)),
+ ('pool1', nn.MaxPool2d(3, 2, ceil_mode=True)),
+
+ ('conv2', nn.Conv2d(28, 48, 3, 1)),
+ ('prelu2', nn.PReLU(48)),
+ ('pool2', nn.MaxPool2d(3, 2, ceil_mode=True)),
+
+ ('conv3', nn.Conv2d(48, 64, 2, 1)),
+ ('prelu3', nn.PReLU(64)),
+
+ ('flatten', Flatten()),
+ ('conv4', nn.Linear(576, 128)),
+ ('prelu4', nn.PReLU(128))
+ ]))
+
+ self.conv5_1 = nn.Linear(128, 2)
+ self.conv5_2 = nn.Linear(128, 4)
+
+ weights = np.load(RNET_PATH, allow_pickle=True)[()]
+ for n, p in self.named_parameters():
+ p.data = torch.FloatTensor(weights[n])
+
+ def forward(self, x):
+ """
+ Arguments:
+ x: a float tensor with shape [batch_size, 3, h, w].
+ Returns:
+ b: a float tensor with shape [batch_size, 4].
+ a: a float tensor with shape [batch_size, 2].
+ """
+ x = self.features(x)
+ a = self.conv5_1(x)
+ b = self.conv5_2(x)
+ a = F.softmax(a, dim=-1)
+ return b, a
+
+
+class ONet(nn.Module):
+
+ def __init__(self):
+ super().__init__()
+
+ self.features = nn.Sequential(OrderedDict([
+ ('conv1', nn.Conv2d(3, 32, 3, 1)),
+ ('prelu1', nn.PReLU(32)),
+ ('pool1', nn.MaxPool2d(3, 2, ceil_mode=True)),
+
+ ('conv2', nn.Conv2d(32, 64, 3, 1)),
+ ('prelu2', nn.PReLU(64)),
+ ('pool2', nn.MaxPool2d(3, 2, ceil_mode=True)),
+
+ ('conv3', nn.Conv2d(64, 64, 3, 1)),
+ ('prelu3', nn.PReLU(64)),
+ ('pool3', nn.MaxPool2d(2, 2, ceil_mode=True)),
+
+ ('conv4', nn.Conv2d(64, 128, 2, 1)),
+ ('prelu4', nn.PReLU(128)),
+
+ ('flatten', Flatten()),
+ ('conv5', nn.Linear(1152, 256)),
+ ('drop5', nn.Dropout(0.25)),
+ ('prelu5', nn.PReLU(256)),
+ ]))
+
+ self.conv6_1 = nn.Linear(256, 2)
+ self.conv6_2 = nn.Linear(256, 4)
+ self.conv6_3 = nn.Linear(256, 10)
+
+ weights = np.load(ONET_PATH, allow_pickle=True)[()]
+ for n, p in self.named_parameters():
+ p.data = torch.FloatTensor(weights[n])
+
+ def forward(self, x):
+ """
+ Arguments:
+ x: a float tensor with shape [batch_size, 3, h, w].
+ Returns:
+ c: a float tensor with shape [batch_size, 10].
+ b: a float tensor with shape [batch_size, 4].
+ a: a float tensor with shape [batch_size, 2].
+ """
+ x = self.features(x)
+ a = self.conv6_1(x)
+ b = self.conv6_2(x)
+ c = self.conv6_3(x)
+ a = F.softmax(a, dim=-1)
+ return c, b, a
diff --git a/models/mtcnn/mtcnn_pytorch/src/matlab_cp2tform.py b/models/mtcnn/mtcnn_pytorch/src/matlab_cp2tform.py
new file mode 100644
index 0000000000000000000000000000000000000000..025b18ec2e64472bd4c0c636f9ae061526bdc8cd
--- /dev/null
+++ b/models/mtcnn/mtcnn_pytorch/src/matlab_cp2tform.py
@@ -0,0 +1,350 @@
+# -*- coding: utf-8 -*-
+"""
+Created on Tue Jul 11 06:54:28 2017
+
+@author: zhaoyafei
+"""
+
+import numpy as np
+from numpy.linalg import inv, norm, lstsq
+from numpy.linalg import matrix_rank as rank
+
+
+class MatlabCp2tormException(Exception):
+ def __str__(self):
+ return 'In File {}:{}'.format(
+ __file__, super.__str__(self))
+
+
+def tformfwd(trans, uv):
+ """
+ Function:
+ ----------
+ apply affine transform 'trans' to uv
+
+ Parameters:
+ ----------
+ @trans: 3x3 np.array
+ transform matrix
+ @uv: Kx2 np.array
+ each row is a pair of coordinates (x, y)
+
+ Returns:
+ ----------
+ @xy: Kx2 np.array
+ each row is a pair of transformed coordinates (x, y)
+ """
+ uv = np.hstack((
+ uv, np.ones((uv.shape[0], 1))
+ ))
+ xy = np.dot(uv, trans)
+ xy = xy[:, 0:-1]
+ return xy
+
+
+def tforminv(trans, uv):
+ """
+ Function:
+ ----------
+ apply the inverse of affine transform 'trans' to uv
+
+ Parameters:
+ ----------
+ @trans: 3x3 np.array
+ transform matrix
+ @uv: Kx2 np.array
+ each row is a pair of coordinates (x, y)
+
+ Returns:
+ ----------
+ @xy: Kx2 np.array
+ each row is a pair of inverse-transformed coordinates (x, y)
+ """
+ Tinv = inv(trans)
+ xy = tformfwd(Tinv, uv)
+ return xy
+
+
+def findNonreflectiveSimilarity(uv, xy, options=None):
+ options = {'K': 2}
+
+ K = options['K']
+ M = xy.shape[0]
+ x = xy[:, 0].reshape((-1, 1)) # use reshape to keep a column vector
+ y = xy[:, 1].reshape((-1, 1)) # use reshape to keep a column vector
+ # print('--->x, y:\n', x, y
+
+ tmp1 = np.hstack((x, y, np.ones((M, 1)), np.zeros((M, 1))))
+ tmp2 = np.hstack((y, -x, np.zeros((M, 1)), np.ones((M, 1))))
+ X = np.vstack((tmp1, tmp2))
+ # print('--->X.shape: ', X.shape
+ # print('X:\n', X
+
+ u = uv[:, 0].reshape((-1, 1)) # use reshape to keep a column vector
+ v = uv[:, 1].reshape((-1, 1)) # use reshape to keep a column vector
+ U = np.vstack((u, v))
+ # print('--->U.shape: ', U.shape
+ # print('U:\n', U
+
+ # We know that X * r = U
+ if rank(X) >= 2 * K:
+ r, _, _, _ = lstsq(X, U, rcond=None) # Make sure this is what I want
+ r = np.squeeze(r)
+ else:
+ raise Exception('cp2tform:twoUniquePointsReq')
+
+ # print('--->r:\n', r
+
+ sc = r[0]
+ ss = r[1]
+ tx = r[2]
+ ty = r[3]
+
+ Tinv = np.array([
+ [sc, -ss, 0],
+ [ss, sc, 0],
+ [tx, ty, 1]
+ ])
+
+ # print('--->Tinv:\n', Tinv
+
+ T = inv(Tinv)
+ # print('--->T:\n', T
+
+ T[:, 2] = np.array([0, 0, 1])
+
+ return T, Tinv
+
+
+def findSimilarity(uv, xy, options=None):
+ options = {'K': 2}
+
+ # uv = np.array(uv)
+ # xy = np.array(xy)
+
+ # Solve for trans1
+ trans1, trans1_inv = findNonreflectiveSimilarity(uv, xy, options)
+
+ # Solve for trans2
+
+ # manually reflect the xy data across the Y-axis
+ xyR = xy
+ xyR[:, 0] = -1 * xyR[:, 0]
+
+ trans2r, trans2r_inv = findNonreflectiveSimilarity(uv, xyR, options)
+
+ # manually reflect the tform to undo the reflection done on xyR
+ TreflectY = np.array([
+ [-1, 0, 0],
+ [0, 1, 0],
+ [0, 0, 1]
+ ])
+
+ trans2 = np.dot(trans2r, TreflectY)
+
+ # Figure out if trans1 or trans2 is better
+ xy1 = tformfwd(trans1, uv)
+ norm1 = norm(xy1 - xy)
+
+ xy2 = tformfwd(trans2, uv)
+ norm2 = norm(xy2 - xy)
+
+ if norm1 <= norm2:
+ return trans1, trans1_inv
+ else:
+ trans2_inv = inv(trans2)
+ return trans2, trans2_inv
+
+
+def get_similarity_transform(src_pts, dst_pts, reflective=True):
+ """
+ Function:
+ ----------
+ Find Similarity Transform Matrix 'trans':
+ u = src_pts[:, 0]
+ v = src_pts[:, 1]
+ x = dst_pts[:, 0]
+ y = dst_pts[:, 1]
+ [x, y, 1] = [u, v, 1] * trans
+
+ Parameters:
+ ----------
+ @src_pts: Kx2 np.array
+ source points, each row is a pair of coordinates (x, y)
+ @dst_pts: Kx2 np.array
+ destination points, each row is a pair of transformed
+ coordinates (x, y)
+ @reflective: True or False
+ if True:
+ use reflective similarity transform
+ else:
+ use non-reflective similarity transform
+
+ Returns:
+ ----------
+ @trans: 3x3 np.array
+ transform matrix from uv to xy
+ trans_inv: 3x3 np.array
+ inverse of trans, transform matrix from xy to uv
+ """
+
+ if reflective:
+ trans, trans_inv = findSimilarity(src_pts, dst_pts)
+ else:
+ trans, trans_inv = findNonreflectiveSimilarity(src_pts, dst_pts)
+
+ return trans, trans_inv
+
+
+def cvt_tform_mat_for_cv2(trans):
+ """
+ Function:
+ ----------
+ Convert Transform Matrix 'trans' into 'cv2_trans' which could be
+ directly used by cv2.warpAffine():
+ u = src_pts[:, 0]
+ v = src_pts[:, 1]
+ x = dst_pts[:, 0]
+ y = dst_pts[:, 1]
+ [x, y].T = cv_trans * [u, v, 1].T
+
+ Parameters:
+ ----------
+ @trans: 3x3 np.array
+ transform matrix from uv to xy
+
+ Returns:
+ ----------
+ @cv2_trans: 2x3 np.array
+ transform matrix from src_pts to dst_pts, could be directly used
+ for cv2.warpAffine()
+ """
+ cv2_trans = trans[:, 0:2].T
+
+ return cv2_trans
+
+
+def get_similarity_transform_for_cv2(src_pts, dst_pts, reflective=True):
+ """
+ Function:
+ ----------
+ Find Similarity Transform Matrix 'cv2_trans' which could be
+ directly used by cv2.warpAffine():
+ u = src_pts[:, 0]
+ v = src_pts[:, 1]
+ x = dst_pts[:, 0]
+ y = dst_pts[:, 1]
+ [x, y].T = cv_trans * [u, v, 1].T
+
+ Parameters:
+ ----------
+ @src_pts: Kx2 np.array
+ source points, each row is a pair of coordinates (x, y)
+ @dst_pts: Kx2 np.array
+ destination points, each row is a pair of transformed
+ coordinates (x, y)
+ reflective: True or False
+ if True:
+ use reflective similarity transform
+ else:
+ use non-reflective similarity transform
+
+ Returns:
+ ----------
+ @cv2_trans: 2x3 np.array
+ transform matrix from src_pts to dst_pts, could be directly used
+ for cv2.warpAffine()
+ """
+ trans, trans_inv = get_similarity_transform(src_pts, dst_pts, reflective)
+ cv2_trans = cvt_tform_mat_for_cv2(trans)
+
+ return cv2_trans
+
+
+if __name__ == '__main__':
+ """
+ u = [0, 6, -2]
+ v = [0, 3, 5]
+ x = [-1, 0, 4]
+ y = [-1, -10, 4]
+
+ # In Matlab, run:
+ #
+ # uv = [u'; v'];
+ # xy = [x'; y'];
+ # tform_sim=cp2tform(uv,xy,'similarity');
+ #
+ # trans = tform_sim.tdata.T
+ # ans =
+ # -0.0764 -1.6190 0
+ # 1.6190 -0.0764 0
+ # -3.2156 0.0290 1.0000
+ # trans_inv = tform_sim.tdata.Tinv
+ # ans =
+ #
+ # -0.0291 0.6163 0
+ # -0.6163 -0.0291 0
+ # -0.0756 1.9826 1.0000
+ # xy_m=tformfwd(tform_sim, u,v)
+ #
+ # xy_m =
+ #
+ # -3.2156 0.0290
+ # 1.1833 -9.9143
+ # 5.0323 2.8853
+ # uv_m=tforminv(tform_sim, x,y)
+ #
+ # uv_m =
+ #
+ # 0.5698 1.3953
+ # 6.0872 2.2733
+ # -2.6570 4.3314
+ """
+ u = [0, 6, -2]
+ v = [0, 3, 5]
+ x = [-1, 0, 4]
+ y = [-1, -10, 4]
+
+ uv = np.array((u, v)).T
+ xy = np.array((x, y)).T
+
+ print('\n--->uv:')
+ print(uv)
+ print('\n--->xy:')
+ print(xy)
+
+ trans, trans_inv = get_similarity_transform(uv, xy)
+
+ print('\n--->trans matrix:')
+ print(trans)
+
+ print('\n--->trans_inv matrix:')
+ print(trans_inv)
+
+ print('\n---> apply transform to uv')
+ print('\nxy_m = uv_augmented * trans')
+ uv_aug = np.hstack((
+ uv, np.ones((uv.shape[0], 1))
+ ))
+ xy_m = np.dot(uv_aug, trans)
+ print(xy_m)
+
+ print('\nxy_m = tformfwd(trans, uv)')
+ xy_m = tformfwd(trans, uv)
+ print(xy_m)
+
+ print('\n---> apply inverse transform to xy')
+ print('\nuv_m = xy_augmented * trans_inv')
+ xy_aug = np.hstack((
+ xy, np.ones((xy.shape[0], 1))
+ ))
+ uv_m = np.dot(xy_aug, trans_inv)
+ print(uv_m)
+
+ print('\nuv_m = tformfwd(trans_inv, xy)')
+ uv_m = tformfwd(trans_inv, xy)
+ print(uv_m)
+
+ uv_m = tforminv(trans, xy)
+ print('\nuv_m = tforminv(trans, xy)')
+ print(uv_m)
diff --git a/models/mtcnn/mtcnn_pytorch/src/visualization_utils.py b/models/mtcnn/mtcnn_pytorch/src/visualization_utils.py
new file mode 100644
index 0000000000000000000000000000000000000000..bab02be31a6ca44486f98d57de4ab4bfa89394b7
--- /dev/null
+++ b/models/mtcnn/mtcnn_pytorch/src/visualization_utils.py
@@ -0,0 +1,31 @@
+from PIL import ImageDraw
+
+
+def show_bboxes(img, bounding_boxes, facial_landmarks=[]):
+ """Draw bounding boxes and facial landmarks.
+
+ Arguments:
+ img: an instance of PIL.Image.
+ bounding_boxes: a float numpy array of shape [n, 5].
+ facial_landmarks: a float numpy array of shape [n, 10].
+
+ Returns:
+ an instance of PIL.Image.
+ """
+
+ img_copy = img.copy()
+ draw = ImageDraw.Draw(img_copy)
+
+ for b in bounding_boxes:
+ draw.rectangle([
+ (b[0], b[1]), (b[2], b[3])
+ ], outline='white')
+
+ for p in facial_landmarks:
+ for i in range(5):
+ draw.ellipse([
+ (p[i] - 1.0, p[i + 5] - 1.0),
+ (p[i] + 1.0, p[i + 5] + 1.0)
+ ], outline='blue')
+
+ return img_copy
diff --git a/models/mtcnn/mtcnn_pytorch/src/weights/onet.npy b/models/mtcnn/mtcnn_pytorch/src/weights/onet.npy
new file mode 100644
index 0000000000000000000000000000000000000000..cdca73b8bbd154e574b4be82945e3e10982acd56
--- /dev/null
+++ b/models/mtcnn/mtcnn_pytorch/src/weights/onet.npy
@@ -0,0 +1,3 @@
+version https://git-lfs.github.com/spec/v1
+oid sha256:313141c3646bebb73cb8350a2d5fee4c7f044fb96304b46ccc21aeea8b818f83
+size 2345483
diff --git a/models/mtcnn/mtcnn_pytorch/src/weights/pnet.npy b/models/mtcnn/mtcnn_pytorch/src/weights/pnet.npy
new file mode 100644
index 0000000000000000000000000000000000000000..344e6beba228f3ce0d191d45125ac2c6954c3fca
--- /dev/null
+++ b/models/mtcnn/mtcnn_pytorch/src/weights/pnet.npy
@@ -0,0 +1,3 @@
+version https://git-lfs.github.com/spec/v1
+oid sha256:03e19e5c473932ab38f5a6308fe6210624006994a687e858d1dcda53c66f18cb
+size 41271
diff --git a/models/mtcnn/mtcnn_pytorch/src/weights/rnet.npy b/models/mtcnn/mtcnn_pytorch/src/weights/rnet.npy
new file mode 100644
index 0000000000000000000000000000000000000000..08699a2123aa6742b146e8c0a5dada489359a1b8
--- /dev/null
+++ b/models/mtcnn/mtcnn_pytorch/src/weights/rnet.npy
@@ -0,0 +1,3 @@
+version https://git-lfs.github.com/spec/v1
+oid sha256:5660aad67688edc9e8a3dd4e47ed120932835e06a8a711a423252a6f2c747083
+size 604651
diff --git a/models/psp.py b/models/psp.py
new file mode 100644
index 0000000000000000000000000000000000000000..607a05aa8aa0d29ca58a4959e78c9b2065953a9e
--- /dev/null
+++ b/models/psp.py
@@ -0,0 +1,148 @@
+"""
+This file defines the core research contribution
+"""
+import matplotlib
+matplotlib.use('Agg')
+import math
+
+import torch
+from torch import nn
+from models.encoders import psp_encoders
+from models.stylegan2.model import Generator
+from configs.paths_config import model_paths
+import torch.nn.functional as F
+
+def get_keys(d, name):
+ if 'state_dict' in d:
+ d = d['state_dict']
+ d_filt = {k[len(name) + 1:]: v for k, v in d.items() if k[:len(name)] == name}
+ return d_filt
+
+
+class pSp(nn.Module):
+
+ def __init__(self, opts, ckpt=None):
+ super(pSp, self).__init__()
+ self.set_opts(opts)
+ # compute number of style inputs based on the output resolution
+ self.opts.n_styles = int(math.log(self.opts.output_size, 2)) * 2 - 2
+ # Define architecture
+ self.encoder = self.set_encoder()
+ self.decoder = Generator(self.opts.output_size, 512, 8)
+ self.face_pool = torch.nn.AdaptiveAvgPool2d((256, 256))
+ # Load weights if needed
+ self.load_weights(ckpt)
+
+ def set_encoder(self):
+ if self.opts.encoder_type == 'GradualStyleEncoder':
+ encoder = psp_encoders.GradualStyleEncoder(50, 'ir_se', self.opts)
+ elif self.opts.encoder_type == 'BackboneEncoderUsingLastLayerIntoW':
+ encoder = psp_encoders.BackboneEncoderUsingLastLayerIntoW(50, 'ir_se', self.opts)
+ elif self.opts.encoder_type == 'BackboneEncoderUsingLastLayerIntoWPlus':
+ encoder = psp_encoders.BackboneEncoderUsingLastLayerIntoWPlus(50, 'ir_se', self.opts)
+ else:
+ raise Exception('{} is not a valid encoders'.format(self.opts.encoder_type))
+ return encoder
+
+ def load_weights(self, ckpt=None):
+ if self.opts.checkpoint_path is not None:
+ print('Loading pSp from checkpoint: {}'.format(self.opts.checkpoint_path))
+ if ckpt is None:
+ ckpt = torch.load(self.opts.checkpoint_path, map_location='cpu')
+ self.encoder.load_state_dict(get_keys(ckpt, 'encoder'), strict=False)
+ self.decoder.load_state_dict(get_keys(ckpt, 'decoder'), strict=False)
+ self.__load_latent_avg(ckpt)
+ else:
+ print('Loading encoders weights from irse50!')
+ encoder_ckpt = torch.load(model_paths['ir_se50'])
+ # if input to encoder is not an RGB image, do not load the input layer weights
+ if self.opts.label_nc != 0:
+ encoder_ckpt = {k: v for k, v in encoder_ckpt.items() if "input_layer" not in k}
+ self.encoder.load_state_dict(encoder_ckpt, strict=False)
+ print('Loading decoder weights from pretrained!')
+ ckpt = torch.load(self.opts.stylegan_weights)
+ self.decoder.load_state_dict(ckpt['g_ema'], strict=False)
+ if self.opts.learn_in_w:
+ self.__load_latent_avg(ckpt, repeat=1)
+ else:
+ self.__load_latent_avg(ckpt, repeat=self.opts.n_styles)
+ # for video toonification, we load G0' model
+ if self.opts.toonify_weights is not None: ##### modified
+ ckpt = torch.load(self.opts.toonify_weights)
+ self.decoder.load_state_dict(ckpt['g_ema'], strict=False)
+ self.opts.toonify_weights = None
+
+ # x1: image for first-layer feature f.
+ # x2: image for style latent code w+. If not specified, x2=x1.
+ # inject_latent: for sketch/mask-to-face translation, another latent code to fuse with w+
+ # latent_mask: fuse w+ and inject_latent with the mask (1~7 use w+ and 8~18 use inject_latent)
+ # use_feature: use f. Otherwise, use the orginal StyleGAN first-layer constant 4*4 feature
+ # first_layer_feature_ind: always=0, means the 1st layer of G accept f
+ # use_skip: use skip connection.
+ # zero_noise: use zero noises.
+ # editing_w: the editing vector v for video face editing
+ def forward(self, x1, x2=None, resize=True, latent_mask=None, randomize_noise=True,
+ inject_latent=None, return_latents=False, alpha=None, use_feature=True,
+ first_layer_feature_ind=0, use_skip=False, zero_noise=False, editing_w=None): ##### modified
+
+ feats = None # f and the skipped encoder features
+ codes, feats = self.encoder(x1, return_feat=True, return_full=use_skip) ##### modified
+ if x2 is not None: ##### modified
+ codes = self.encoder(x2) ##### modified
+ # normalize with respect to the center of an average face
+ if self.opts.start_from_latent_avg:
+ if self.opts.learn_in_w:
+ codes = codes + self.latent_avg.repeat(codes.shape[0], 1)
+ else:
+ codes = codes + self.latent_avg.repeat(codes.shape[0], 1, 1)
+
+ # E_W^{1:7}(T(x1)) concatenate E_W^{8:18}(w~)
+ if latent_mask is not None:
+ for i in latent_mask:
+ if inject_latent is not None:
+ if alpha is not None:
+ codes[:, i] = alpha * inject_latent[:, i] + (1 - alpha) * codes[:, i]
+ else:
+ codes[:, i] = inject_latent[:, i]
+ else:
+ codes[:, i] = 0
+
+ first_layer_feats, skip_layer_feats, fusion = None, None, None ##### modified
+ if use_feature: ##### modified
+ first_layer_feats = feats[0:2] # use f
+ if use_skip: ##### modified
+ skip_layer_feats = feats[2:] # use skipped encoder feature
+ fusion = self.encoder.fusion # use fusion layer to fuse encoder feature and decoder feature.
+
+ images, result_latent = self.decoder([codes],
+ input_is_latent=True,
+ randomize_noise=randomize_noise,
+ return_latents=return_latents,
+ first_layer_feature=first_layer_feats,
+ first_layer_feature_ind=first_layer_feature_ind,
+ skip_layer_feature=skip_layer_feats,
+ fusion_block=fusion,
+ zero_noise=zero_noise,
+ editing_w=editing_w) ##### modified
+
+ if resize:
+ if self.opts.output_size == 1024: ##### modified
+ images = F.adaptive_avg_pool2d(images, (images.shape[2]//4, images.shape[3]//4)) ##### modified
+ else:
+ images = self.face_pool(images)
+
+ if return_latents:
+ return images, result_latent
+ else:
+ return images
+
+ def set_opts(self, opts):
+ self.opts = opts
+
+ def __load_latent_avg(self, ckpt, repeat=None):
+ if 'latent_avg' in ckpt:
+ self.latent_avg = ckpt['latent_avg'].to(self.opts.device)
+ if repeat is not None:
+ self.latent_avg = self.latent_avg.repeat(repeat, 1)
+ else:
+ self.latent_avg = None
diff --git a/models/stylegan2/__init__.py b/models/stylegan2/__init__.py
new file mode 100644
index 0000000000000000000000000000000000000000..e69de29bb2d1d6434b8b29ae775ad8c2e48c5391
diff --git a/models/stylegan2/lpips/__init__.py b/models/stylegan2/lpips/__init__.py
new file mode 100644
index 0000000000000000000000000000000000000000..22252ce8594c5bd2c9dc17e75f977ed21c94447f
--- /dev/null
+++ b/models/stylegan2/lpips/__init__.py
@@ -0,0 +1,161 @@
+
+from __future__ import absolute_import
+from __future__ import division
+from __future__ import print_function
+
+import numpy as np
+#from skimage.measure import compare_ssim
+from skimage.metrics import structural_similarity as compare_ssim
+import torch
+from torch.autograd import Variable
+
+from models.stylegan2.lpips import dist_model
+
+class PerceptualLoss(torch.nn.Module):
+ def __init__(self, model='net-lin', net='alex', colorspace='rgb', spatial=False, use_gpu=True, gpu_ids=[0]): # VGG using our perceptually-learned weights (LPIPS metric)
+ # def __init__(self, model='net', net='vgg', use_gpu=True): # "default" way of using VGG as a perceptual loss
+ super(PerceptualLoss, self).__init__()
+ print('Setting up Perceptual loss...')
+ self.use_gpu = use_gpu
+ self.spatial = spatial
+ self.gpu_ids = gpu_ids
+ self.model = dist_model.DistModel()
+ self.model.initialize(model=model, net=net, use_gpu=use_gpu, colorspace=colorspace, spatial=self.spatial, gpu_ids=gpu_ids)
+ print('...[%s] initialized'%self.model.name())
+ print('...Done')
+
+ def forward(self, pred, target, normalize=False):
+ """
+ Pred and target are Variables.
+ If normalize is True, assumes the images are between [0,1] and then scales them between [-1,+1]
+ If normalize is False, assumes the images are already between [-1,+1]
+
+ Inputs pred and target are Nx3xHxW
+ Output pytorch Variable N long
+ """
+
+ if normalize:
+ target = 2 * target - 1
+ pred = 2 * pred - 1
+
+ return self.model.forward(target, pred)
+
+def normalize_tensor(in_feat,eps=1e-10):
+ norm_factor = torch.sqrt(torch.sum(in_feat**2,dim=1,keepdim=True))
+ return in_feat/(norm_factor+eps)
+
+def l2(p0, p1, range=255.):
+ return .5*np.mean((p0 / range - p1 / range)**2)
+
+def psnr(p0, p1, peak=255.):
+ return 10*np.log10(peak**2/np.mean((1.*p0-1.*p1)**2))
+
+def dssim(p0, p1, range=255.):
+ return (1 - compare_ssim(p0, p1, data_range=range, multichannel=True)) / 2.
+
+def rgb2lab(in_img,mean_cent=False):
+ from skimage import color
+ img_lab = color.rgb2lab(in_img)
+ if(mean_cent):
+ img_lab[:,:,0] = img_lab[:,:,0]-50
+ return img_lab
+
+def tensor2np(tensor_obj):
+ # change dimension of a tensor object into a numpy array
+ return tensor_obj[0].cpu().float().numpy().transpose((1,2,0))
+
+def np2tensor(np_obj):
+ # change dimenion of np array into tensor array
+ return torch.Tensor(np_obj[:, :, :, np.newaxis].transpose((3, 2, 0, 1)))
+
+def tensor2tensorlab(image_tensor,to_norm=True,mc_only=False):
+ # image tensor to lab tensor
+ from skimage import color
+
+ img = tensor2im(image_tensor)
+ img_lab = color.rgb2lab(img)
+ if(mc_only):
+ img_lab[:,:,0] = img_lab[:,:,0]-50
+ if(to_norm and not mc_only):
+ img_lab[:,:,0] = img_lab[:,:,0]-50
+ img_lab = img_lab/100.
+
+ return np2tensor(img_lab)
+
+def tensorlab2tensor(lab_tensor,return_inbnd=False):
+ from skimage import color
+ import warnings
+ warnings.filterwarnings("ignore")
+
+ lab = tensor2np(lab_tensor)*100.
+ lab[:,:,0] = lab[:,:,0]+50
+
+ rgb_back = 255.*np.clip(color.lab2rgb(lab.astype('float')),0,1)
+ if(return_inbnd):
+ # convert back to lab, see if we match
+ lab_back = color.rgb2lab(rgb_back.astype('uint8'))
+ mask = 1.*np.isclose(lab_back,lab,atol=2.)
+ mask = np2tensor(np.prod(mask,axis=2)[:,:,np.newaxis])
+ return (im2tensor(rgb_back),mask)
+ else:
+ return im2tensor(rgb_back)
+
+def rgb2lab(input):
+ from skimage import color
+ return color.rgb2lab(input / 255.)
+
+def tensor2im(image_tensor, imtype=np.uint8, cent=1., factor=255./2.):
+ image_numpy = image_tensor[0].cpu().float().numpy()
+ image_numpy = (np.transpose(image_numpy, (1, 2, 0)) + cent) * factor
+ return image_numpy.astype(imtype)
+
+def im2tensor(image, imtype=np.uint8, cent=1., factor=255./2.):
+ return torch.Tensor((image / factor - cent)
+ [:, :, :, np.newaxis].transpose((3, 2, 0, 1)))
+
+def tensor2vec(vector_tensor):
+ return vector_tensor.data.cpu().numpy()[:, :, 0, 0]
+
+def voc_ap(rec, prec, use_07_metric=False):
+ """ ap = voc_ap(rec, prec, [use_07_metric])
+ Compute VOC AP given precision and recall.
+ If use_07_metric is true, uses the
+ VOC 07 11 point method (default:False).
+ """
+ if use_07_metric:
+ # 11 point metric
+ ap = 0.
+ for t in np.arange(0., 1.1, 0.1):
+ if np.sum(rec >= t) == 0:
+ p = 0
+ else:
+ p = np.max(prec[rec >= t])
+ ap = ap + p / 11.
+ else:
+ # correct AP calculation
+ # first append sentinel values at the end
+ mrec = np.concatenate(([0.], rec, [1.]))
+ mpre = np.concatenate(([0.], prec, [0.]))
+
+ # compute the precision envelope
+ for i in range(mpre.size - 1, 0, -1):
+ mpre[i - 1] = np.maximum(mpre[i - 1], mpre[i])
+
+ # to calculate area under PR curve, look for points
+ # where X axis (recall) changes value
+ i = np.where(mrec[1:] != mrec[:-1])[0]
+
+ # and sum (\Delta recall) * prec
+ ap = np.sum((mrec[i + 1] - mrec[i]) * mpre[i + 1])
+ return ap
+
+def tensor2im(image_tensor, imtype=np.uint8, cent=1., factor=255./2.):
+# def tensor2im(image_tensor, imtype=np.uint8, cent=1., factor=1.):
+ image_numpy = image_tensor[0].cpu().float().numpy()
+ image_numpy = (np.transpose(image_numpy, (1, 2, 0)) + cent) * factor
+ return image_numpy.astype(imtype)
+
+def im2tensor(image, imtype=np.uint8, cent=1., factor=255./2.):
+# def im2tensor(image, imtype=np.uint8, cent=1., factor=1.):
+ return torch.Tensor((image / factor - cent)
+ [:, :, :, np.newaxis].transpose((3, 2, 0, 1)))
diff --git a/models/stylegan2/lpips/base_model.py b/models/stylegan2/lpips/base_model.py
new file mode 100644
index 0000000000000000000000000000000000000000..8de1d16f0c7fa52d8067139abc6e769e96d0a6a1
--- /dev/null
+++ b/models/stylegan2/lpips/base_model.py
@@ -0,0 +1,58 @@
+import os
+import numpy as np
+import torch
+from torch.autograd import Variable
+from pdb import set_trace as st
+from IPython import embed
+
+class BaseModel():
+ def __init__(self):
+ pass;
+
+ def name(self):
+ return 'BaseModel'
+
+ def initialize(self, use_gpu=True, gpu_ids=[0]):
+ self.use_gpu = use_gpu
+ self.gpu_ids = gpu_ids
+
+ def forward(self):
+ pass
+
+ def get_image_paths(self):
+ pass
+
+ def optimize_parameters(self):
+ pass
+
+ def get_current_visuals(self):
+ return self.input
+
+ def get_current_errors(self):
+ return {}
+
+ def save(self, label):
+ pass
+
+ # helper saving function that can be used by subclasses
+ def save_network(self, network, path, network_label, epoch_label):
+ save_filename = '%s_net_%s.pth' % (epoch_label, network_label)
+ save_path = os.path.join(path, save_filename)
+ torch.save(network.state_dict(), save_path)
+
+ # helper loading function that can be used by subclasses
+ def load_network(self, network, network_label, epoch_label):
+ save_filename = '%s_net_%s.pth' % (epoch_label, network_label)
+ save_path = os.path.join(self.save_dir, save_filename)
+ print('Loading network from %s'%save_path)
+ network.load_state_dict(torch.load(save_path))
+
+ def update_learning_rate():
+ pass
+
+ def get_image_paths(self):
+ return self.image_paths
+
+ def save_done(self, flag=False):
+ np.save(os.path.join(self.save_dir, 'done_flag'),flag)
+ np.savetxt(os.path.join(self.save_dir, 'done_flag'),[flag,],fmt='%i')
diff --git a/models/stylegan2/lpips/dist_model.py b/models/stylegan2/lpips/dist_model.py
new file mode 100644
index 0000000000000000000000000000000000000000..117fd18899608ce9c7398bafa62d75c8b6efc603
--- /dev/null
+++ b/models/stylegan2/lpips/dist_model.py
@@ -0,0 +1,284 @@
+
+from __future__ import absolute_import
+
+import sys
+import numpy as np
+import torch
+from torch import nn
+import os
+from collections import OrderedDict
+from torch.autograd import Variable
+import itertools
+from models.stylegan2.lpips.base_model import BaseModel
+from scipy.ndimage import zoom
+import fractions
+import functools
+import skimage.transform
+from tqdm import tqdm
+
+from IPython import embed
+
+from models.stylegan2.lpips import networks_basic as networks
+import models.stylegan2.lpips as util
+
+class DistModel(BaseModel):
+ def name(self):
+ return self.model_name
+
+ def initialize(self, model='net-lin', net='alex', colorspace='Lab', pnet_rand=False, pnet_tune=False, model_path=None,
+ use_gpu=True, printNet=False, spatial=False,
+ is_train=False, lr=.0001, beta1=0.5, version='0.1', gpu_ids=[0]):
+ '''
+ INPUTS
+ model - ['net-lin'] for linearly calibrated network
+ ['net'] for off-the-shelf network
+ ['L2'] for L2 distance in Lab colorspace
+ ['SSIM'] for ssim in RGB colorspace
+ net - ['squeeze','alex','vgg']
+ model_path - if None, will look in weights/[NET_NAME].pth
+ colorspace - ['Lab','RGB'] colorspace to use for L2 and SSIM
+ use_gpu - bool - whether or not to use a GPU
+ printNet - bool - whether or not to print network architecture out
+ spatial - bool - whether to output an array containing varying distances across spatial dimensions
+ spatial_shape - if given, output spatial shape. if None then spatial shape is determined automatically via spatial_factor (see below).
+ spatial_factor - if given, specifies upsampling factor relative to the largest spatial extent of a convolutional layer. if None then resized to size of input images.
+ spatial_order - spline order of filter for upsampling in spatial mode, by default 1 (bilinear).
+ is_train - bool - [True] for training mode
+ lr - float - initial learning rate
+ beta1 - float - initial momentum term for adam
+ version - 0.1 for latest, 0.0 was original (with a bug)
+ gpu_ids - int array - [0] by default, gpus to use
+ '''
+ BaseModel.initialize(self, use_gpu=use_gpu, gpu_ids=gpu_ids)
+
+ self.model = model
+ self.net = net
+ self.is_train = is_train
+ self.spatial = spatial
+ self.gpu_ids = gpu_ids
+ self.model_name = '%s [%s]'%(model,net)
+
+ if(self.model == 'net-lin'): # pretrained net + linear layer
+ self.net = networks.PNetLin(pnet_rand=pnet_rand, pnet_tune=pnet_tune, pnet_type=net,
+ use_dropout=True, spatial=spatial, version=version, lpips=True)
+ kw = {}
+ if not use_gpu:
+ kw['map_location'] = 'cpu'
+ if(model_path is None):
+ import inspect
+ model_path = os.path.abspath(os.path.join(inspect.getfile(self.initialize), '..', 'weights/v%s/%s.pth'%(version,net)))
+
+ if(not is_train):
+ print('Loading model from: %s'%model_path)
+ self.net.load_state_dict(torch.load(model_path, **kw), strict=False)
+
+ elif(self.model=='net'): # pretrained network
+ self.net = networks.PNetLin(pnet_rand=pnet_rand, pnet_type=net, lpips=False)
+ elif(self.model in ['L2','l2']):
+ self.net = networks.L2(use_gpu=use_gpu,colorspace=colorspace) # not really a network, only for testing
+ self.model_name = 'L2'
+ elif(self.model in ['DSSIM','dssim','SSIM','ssim']):
+ self.net = networks.DSSIM(use_gpu=use_gpu,colorspace=colorspace)
+ self.model_name = 'SSIM'
+ else:
+ raise ValueError("Model [%s] not recognized." % self.model)
+
+ self.parameters = list(self.net.parameters())
+
+ if self.is_train: # training mode
+ # extra network on top to go from distances (d0,d1) => predicted human judgment (h*)
+ self.rankLoss = networks.BCERankingLoss()
+ self.parameters += list(self.rankLoss.net.parameters())
+ self.lr = lr
+ self.old_lr = lr
+ self.optimizer_net = torch.optim.Adam(self.parameters, lr=lr, betas=(beta1, 0.999))
+ else: # test mode
+ self.net.eval()
+
+ if(use_gpu):
+ self.net.to(gpu_ids[0])
+ self.net = torch.nn.DataParallel(self.net, device_ids=gpu_ids)
+ if(self.is_train):
+ self.rankLoss = self.rankLoss.to(device=gpu_ids[0]) # just put this on GPU0
+
+ if(printNet):
+ print('---------- Networks initialized -------------')
+ networks.print_network(self.net)
+ print('-----------------------------------------------')
+
+ def forward(self, in0, in1, retPerLayer=False):
+ ''' Function computes the distance between image patches in0 and in1
+ INPUTS
+ in0, in1 - torch.Tensor object of shape Nx3xXxY - image patch scaled to [-1,1]
+ OUTPUT
+ computed distances between in0 and in1
+ '''
+
+ return self.net.forward(in0, in1, retPerLayer=retPerLayer)
+
+ # ***** TRAINING FUNCTIONS *****
+ def optimize_parameters(self):
+ self.forward_train()
+ self.optimizer_net.zero_grad()
+ self.backward_train()
+ self.optimizer_net.step()
+ self.clamp_weights()
+
+ def clamp_weights(self):
+ for module in self.net.modules():
+ if(hasattr(module, 'weight') and module.kernel_size==(1,1)):
+ module.weight.data = torch.clamp(module.weight.data,min=0)
+
+ def set_input(self, data):
+ self.input_ref = data['ref']
+ self.input_p0 = data['p0']
+ self.input_p1 = data['p1']
+ self.input_judge = data['judge']
+
+ if(self.use_gpu):
+ self.input_ref = self.input_ref.to(device=self.gpu_ids[0])
+ self.input_p0 = self.input_p0.to(device=self.gpu_ids[0])
+ self.input_p1 = self.input_p1.to(device=self.gpu_ids[0])
+ self.input_judge = self.input_judge.to(device=self.gpu_ids[0])
+
+ self.var_ref = Variable(self.input_ref,requires_grad=True)
+ self.var_p0 = Variable(self.input_p0,requires_grad=True)
+ self.var_p1 = Variable(self.input_p1,requires_grad=True)
+
+ def forward_train(self): # run forward pass
+ # print(self.net.module.scaling_layer.shift)
+ # print(torch.norm(self.net.module.net.slice1[0].weight).item(), torch.norm(self.net.module.lin0.model[1].weight).item())
+
+ self.d0 = self.forward(self.var_ref, self.var_p0)
+ self.d1 = self.forward(self.var_ref, self.var_p1)
+ self.acc_r = self.compute_accuracy(self.d0,self.d1,self.input_judge)
+
+ self.var_judge = Variable(1.*self.input_judge).view(self.d0.size())
+
+ self.loss_total = self.rankLoss.forward(self.d0, self.d1, self.var_judge*2.-1.)
+
+ return self.loss_total
+
+ def backward_train(self):
+ torch.mean(self.loss_total).backward()
+
+ def compute_accuracy(self,d0,d1,judge):
+ ''' d0, d1 are Variables, judge is a Tensor '''
+ d1_lt_d0 = (d1 %f' % (type,self.old_lr, lr))
+ self.old_lr = lr
+
+def score_2afc_dataset(data_loader, func, name=''):
+ ''' Function computes Two Alternative Forced Choice (2AFC) score using
+ distance function 'func' in dataset 'data_loader'
+ INPUTS
+ data_loader - CustomDatasetDataLoader object - contains a TwoAFCDataset inside
+ func - callable distance function - calling d=func(in0,in1) should take 2
+ pytorch tensors with shape Nx3xXxY, and return numpy array of length N
+ OUTPUTS
+ [0] - 2AFC score in [0,1], fraction of time func agrees with human evaluators
+ [1] - dictionary with following elements
+ d0s,d1s - N arrays containing distances between reference patch to perturbed patches
+ gts - N array in [0,1], preferred patch selected by human evaluators
+ (closer to "0" for left patch p0, "1" for right patch p1,
+ "0.6" means 60pct people preferred right patch, 40pct preferred left)
+ scores - N array in [0,1], corresponding to what percentage function agreed with humans
+ CONSTS
+ N - number of test triplets in data_loader
+ '''
+
+ d0s = []
+ d1s = []
+ gts = []
+
+ for data in tqdm(data_loader.load_data(), desc=name):
+ d0s+=func(data['ref'],data['p0']).data.cpu().numpy().flatten().tolist()
+ d1s+=func(data['ref'],data['p1']).data.cpu().numpy().flatten().tolist()
+ gts+=data['judge'].cpu().numpy().flatten().tolist()
+
+ d0s = np.array(d0s)
+ d1s = np.array(d1s)
+ gts = np.array(gts)
+ scores = (d0s 1:
+ kernel = kernel * (upsample_factor ** 2)
+
+ self.register_buffer('kernel', kernel)
+
+ self.pad = pad
+
+ def forward(self, input):
+ out = upfirdn2d(input, self.kernel, pad=self.pad)
+
+ return out
+
+
+class EqualConv2d(nn.Module):
+ def __init__(
+ self, in_channel, out_channel, kernel_size, stride=1, padding=0, bias=True, dilation=1 ## modified
+ ):
+ super().__init__()
+
+ self.weight = nn.Parameter(
+ torch.randn(out_channel, in_channel, kernel_size, kernel_size)
+ )
+ self.scale = 1 / math.sqrt(in_channel * kernel_size ** 2)
+
+ self.stride = stride
+ self.padding = padding
+ self.dilation = dilation ## modified
+
+ if bias:
+ self.bias = nn.Parameter(torch.zeros(out_channel))
+
+ else:
+ self.bias = None
+
+ def forward(self, input):
+ out = F.conv2d(
+ input,
+ self.weight * self.scale,
+ bias=self.bias,
+ stride=self.stride,
+ padding=self.padding,
+ dilation=self.dilation, ## modified
+ )
+
+ return out
+
+ def __repr__(self):
+ return (
+ f"{self.__class__.__name__}({self.weight.shape[1]}, {self.weight.shape[0]},"
+ f" {self.weight.shape[2]}, stride={self.stride}, padding={self.padding}, dilation={self.dilation})" ## modified
+ )
+
+
+class EqualLinear(nn.Module):
+ def __init__(
+ self, in_dim, out_dim, bias=True, bias_init=0, lr_mul=1, activation=None
+ ):
+ super().__init__()
+
+ self.weight = nn.Parameter(torch.randn(out_dim, in_dim).div_(lr_mul))
+
+ if bias:
+ self.bias = nn.Parameter(torch.zeros(out_dim).fill_(bias_init))
+
+ else:
+ self.bias = None
+
+ self.activation = activation
+
+ self.scale = (1 / math.sqrt(in_dim)) * lr_mul
+ self.lr_mul = lr_mul
+
+ def forward(self, input):
+ if self.activation:
+ out = F.linear(input, self.weight * self.scale)
+ out = fused_leaky_relu(out, self.bias * self.lr_mul)
+
+ else:
+ out = F.linear(
+ input, self.weight * self.scale, bias=self.bias * self.lr_mul
+ )
+
+ return out
+
+ def __repr__(self):
+ return (
+ f'{self.__class__.__name__}({self.weight.shape[1]}, {self.weight.shape[0]})'
+ )
+
+
+class ScaledLeakyReLU(nn.Module):
+ def __init__(self, negative_slope=0.2):
+ super().__init__()
+
+ self.negative_slope = negative_slope
+
+ def forward(self, input):
+ out = F.leaky_relu(input, negative_slope=self.negative_slope)
+
+ return out * math.sqrt(2)
+
+
+class ModulatedConv2d(nn.Module):
+ def __init__(
+ self,
+ in_channel,
+ out_channel,
+ kernel_size,
+ style_dim,
+ demodulate=True,
+ upsample=False,
+ downsample=False,
+ blur_kernel=[1, 3, 3, 1],
+ dilation=1, ##### modified
+ ):
+ super().__init__()
+
+ self.eps = 1e-8
+ self.kernel_size = kernel_size
+ self.in_channel = in_channel
+ self.out_channel = out_channel
+ self.upsample = upsample
+ self.downsample = downsample
+ self.dilation = dilation ##### modified
+
+ if upsample:
+ factor = 2
+ p = (len(blur_kernel) - factor) - (kernel_size - 1)
+ pad0 = (p + 1) // 2 + factor - 1
+ pad1 = p // 2 + 1
+
+ self.blur = Blur(blur_kernel, pad=(pad0, pad1), upsample_factor=factor)
+
+ # to simulate transconv + blur
+ # we use dilated transposed conv with blur kernel as weight + dilated transconv
+ if dilation > 1: ##### modified
+ blur_weight = torch.randn(1, 1, 3, 3) * 0 + 1
+ blur_weight[:,:,0,1] = 2
+ blur_weight[:,:,1,0] = 2
+ blur_weight[:,:,1,2] = 2
+ blur_weight[:,:,2,1] = 2
+ blur_weight[:,:,1,1] = 4
+ blur_weight = blur_weight / 16.0
+ self.register_buffer("blur_weight", blur_weight)
+
+ if downsample:
+ factor = 2
+ p = (len(blur_kernel) - factor) + (kernel_size - 1)
+ pad0 = (p + 1) // 2
+ pad1 = p // 2
+
+ self.blur = Blur(blur_kernel, pad=(pad0, pad1))
+
+ fan_in = in_channel * kernel_size ** 2
+ self.scale = 1 / math.sqrt(fan_in)
+ self.padding = kernel_size // 2 + dilation - 1 ##### modified
+
+ self.weight = nn.Parameter(
+ torch.randn(1, out_channel, in_channel, kernel_size, kernel_size)
+ )
+
+ self.modulation = EqualLinear(style_dim, in_channel, bias_init=1)
+
+ self.demodulate = demodulate
+
+ def __repr__(self):
+ return (
+ f'{self.__class__.__name__}({self.in_channel}, {self.out_channel}, {self.kernel_size}, '
+ f'upsample={self.upsample}, downsample={self.downsample})'
+ )
+
+ def forward(self, input, style):
+ batch, in_channel, height, width = input.shape
+
+ style = self.modulation(style).view(batch, 1, in_channel, 1, 1)
+ weight = self.scale * self.weight * style
+
+ if self.demodulate:
+ demod = torch.rsqrt(weight.pow(2).sum([2, 3, 4]) + 1e-8)
+ weight = weight * demod.view(batch, self.out_channel, 1, 1, 1)
+
+ weight = weight.view(
+ batch * self.out_channel, in_channel, self.kernel_size, self.kernel_size
+ )
+
+ if self.upsample:
+ input = input.view(1, batch * in_channel, height, width)
+ weight = weight.view(
+ batch, self.out_channel, in_channel, self.kernel_size, self.kernel_size
+ )
+ weight = weight.transpose(1, 2).reshape(
+ batch * in_channel, self.out_channel, self.kernel_size, self.kernel_size
+ )
+
+ if self.dilation > 1: ##### modified
+ # to simulate out = self.blur(out)
+ out = F.conv_transpose2d(
+ input, self.blur_weight.repeat(batch*in_channel,1,1,1), padding=0, groups=batch*in_channel, dilation=self.dilation//2)
+ # to simulate the next line
+ out = F.conv_transpose2d(
+ out, weight, padding=self.dilation, groups=batch, dilation=self.dilation//2)
+ _, _, height, width = out.shape
+ out = out.view(batch, self.out_channel, height, width)
+ return out
+
+ out = F.conv_transpose2d(input, weight, padding=0, stride=2, groups=batch)
+ _, _, height, width = out.shape
+ out = out.view(batch, self.out_channel, height, width)
+ out = self.blur(out)
+
+ elif self.downsample:
+ input = self.blur(input)
+ _, _, height, width = input.shape
+ input = input.view(1, batch * in_channel, height, width)
+ out = F.conv2d(input, weight, padding=0, stride=2, groups=batch)
+ _, _, height, width = out.shape
+ out = out.view(batch, self.out_channel, height, width)
+
+ else:
+ input = input.view(1, batch * in_channel, height, width)
+ out = F.conv2d(input, weight, padding=self.padding, groups=batch, dilation=self.dilation) ##### modified
+ _, _, height, width = out.shape
+ out = out.view(batch, self.out_channel, height, width)
+
+ return out
+
+
+class NoiseInjection(nn.Module):
+ def __init__(self):
+ super().__init__()
+
+ self.weight = nn.Parameter(torch.zeros(1))
+
+ def forward(self, image, noise=None):
+ if noise is None:
+ batch, _, height, width = image.shape
+ noise = image.new_empty(batch, 1, height, width).normal_()
+ else: ##### modified, to make the resolution matches
+ batch, _, height, width = image.shape
+ _, _, height1, width1 = noise.shape
+ if height != height1 or width != width1:
+ noise = F.adaptive_avg_pool2d(noise, (height, width))
+
+ return image + self.weight * noise
+
+
+class ConstantInput(nn.Module):
+ def __init__(self, channel, size=4):
+ super().__init__()
+
+ self.input = nn.Parameter(torch.randn(1, channel, size, size))
+
+ def forward(self, input):
+ batch = input.shape[0]
+ out = self.input.repeat(batch, 1, 1, 1)
+
+ return out
+
+
+class StyledConv(nn.Module):
+ def __init__(
+ self,
+ in_channel,
+ out_channel,
+ kernel_size,
+ style_dim,
+ upsample=False,
+ blur_kernel=[1, 3, 3, 1],
+ demodulate=True,
+ dilation=1, ##### modified
+ ):
+ super().__init__()
+
+ self.conv = ModulatedConv2d(
+ in_channel,
+ out_channel,
+ kernel_size,
+ style_dim,
+ upsample=upsample,
+ blur_kernel=blur_kernel,
+ demodulate=demodulate,
+ dilation=dilation, ##### modified
+ )
+
+ self.noise = NoiseInjection()
+ self.activate = FusedLeakyReLU(out_channel)
+
+ def forward(self, input, style, noise=None):
+ out = self.conv(input, style)
+ out = self.noise(out, noise=noise)
+ out = self.activate(out)
+
+ return out
+
+
+class ToRGB(nn.Module):
+ def __init__(self, in_channel, style_dim, upsample=True, blur_kernel=[1, 3, 3, 1], dilation=1): ##### modified
+ super().__init__()
+
+ if upsample:
+ self.upsample = Upsample(blur_kernel)
+
+ self.conv = ModulatedConv2d(in_channel, 3, 1, style_dim, demodulate=False)
+ self.bias = nn.Parameter(torch.zeros(1, 3, 1, 1))
+
+ self.dilation = dilation ##### modified
+ if dilation > 1: ##### modified
+ blur_weight = torch.randn(1, 1, 3, 3) * 0 + 1
+ blur_weight[:,:,0,1] = 2
+ blur_weight[:,:,1,0] = 2
+ blur_weight[:,:,1,2] = 2
+ blur_weight[:,:,2,1] = 2
+ blur_weight[:,:,1,1] = 4
+ blur_weight = blur_weight / 16.0
+ self.register_buffer("blur_weight", blur_weight)
+
+ def forward(self, input, style, skip=None):
+ out = self.conv(input, style)
+ out = out + self.bias
+
+ if skip is not None:
+ if self.dilation == 1:
+ skip = self.upsample(skip)
+ else: ##### modified, to simulate skip = self.upsample(skip)
+ batch, in_channel, _, _ = skip.shape
+ skip = F.conv2d(skip, self.blur_weight.repeat(in_channel,1,1,1),
+ padding=self.dilation//2, groups=in_channel, dilation=self.dilation//2)
+
+ out = out + skip
+
+ return out
+
+
+class Generator(nn.Module):
+ def __init__(
+ self,
+ size,
+ style_dim,
+ n_mlp,
+ channel_multiplier=2,
+ blur_kernel=[1, 3, 3, 1],
+ lr_mlp=0.01,
+ ):
+ super().__init__()
+
+ self.size = size
+
+ self.style_dim = style_dim
+
+ layers = [PixelNorm()]
+
+ for i in range(n_mlp):
+ layers.append(
+ EqualLinear(
+ style_dim, style_dim, lr_mul=lr_mlp, activation='fused_lrelu'
+ )
+ )
+
+ self.style = nn.Sequential(*layers)
+
+ self.channels = {
+ 4: 512,
+ 8: 512,
+ 16: 512,
+ 32: 512,
+ 64: 256 * channel_multiplier,
+ 128: 128 * channel_multiplier,
+ 256: 64 * channel_multiplier,
+ 512: 32 * channel_multiplier,
+ 1024: 16 * channel_multiplier,
+ }
+
+ self.input = ConstantInput(self.channels[4])
+ self.conv1 = StyledConv(
+ self.channels[4], self.channels[4], 3, style_dim, blur_kernel=blur_kernel, dilation=8 ##### modified
+ )
+ self.to_rgb1 = ToRGB(self.channels[4], style_dim, upsample=False)
+
+ self.log_size = int(math.log(size, 2))
+ self.num_layers = (self.log_size - 2) * 2 + 1
+
+ self.convs = nn.ModuleList()
+ self.upsamples = nn.ModuleList()
+ self.to_rgbs = nn.ModuleList()
+ self.noises = nn.Module()
+
+ in_channel = self.channels[4]
+
+ for layer_idx in range(self.num_layers):
+ res = (layer_idx + 5) // 2
+ shape = [1, 1, 2 ** res, 2 ** res]
+ self.noises.register_buffer(f'noise_{layer_idx}', torch.randn(*shape))
+
+ for i in range(3, self.log_size + 1):
+ out_channel = self.channels[2 ** i]
+
+ self.convs.append(
+ StyledConv(
+ in_channel,
+ out_channel,
+ 3,
+ style_dim,
+ upsample=True,
+ blur_kernel=blur_kernel,
+ dilation=max(1, 32 // (2**(i-1))) ##### modified
+ )
+ )
+
+ self.convs.append(
+ StyledConv(
+ out_channel, out_channel, 3, style_dim, blur_kernel=blur_kernel, dilation=max(1, 32 // (2**i)) ##### modified
+ )
+ )
+
+ self.to_rgbs.append(ToRGB(out_channel, style_dim, dilation=max(1, 32 // (2**(i-1))))) ##### modified
+
+ in_channel = out_channel
+
+ self.n_latent = self.log_size * 2 - 2
+
+ def make_noise(self):
+ device = self.input.input.device
+
+ noises = [torch.randn(1, 1, 2 ** 2, 2 ** 2, device=device)]
+
+ for i in range(3, self.log_size + 1):
+ for _ in range(2):
+ noises.append(torch.randn(1, 1, 2 ** i, 2 ** i, device=device))
+
+ return noises
+
+ def mean_latent(self, n_latent):
+ latent_in = torch.randn(
+ n_latent, self.style_dim, device=self.input.input.device
+ )
+ latent = self.style(latent_in).mean(0, keepdim=True)
+
+ return latent
+
+ def get_latent(self, input):
+ return self.style(input)
+
+ # styles is the latent code w+
+ # first_layer_feature is the first-layer input feature f
+ # first_layer_feature_ind indicate which layer of G accepts f (should always=0, the first layer)
+ # skip_layer_feature is the encoder features sent by skip connection
+ # fusion_block is the network to fuse the encoder feature and decoder feature
+ # zero_noise is to force the noise to be zero (to avoid flickers for videos)
+ # editing_w is the editing vector v used in video face editing
+ def forward(
+ self,
+ styles,
+ return_latents=False,
+ return_features=False,
+ inject_index=None,
+ truncation=1,
+ truncation_latent=None,
+ input_is_latent=False,
+ noise=None,
+ randomize_noise=True,
+ first_layer_feature = None, ##### modified
+ first_layer_feature_ind = 0, ##### modified
+ skip_layer_feature = None, ##### modified
+ fusion_block = None, ##### modified
+ zero_noise = False, ##### modified
+ editing_w = None, ##### modified
+ ):
+ if not input_is_latent:
+ styles = [self.style(s) for s in styles]
+
+ if zero_noise:
+ noise = [
+ getattr(self.noises, f'noise_{i}') * 0.0 for i in range(self.num_layers)
+ ]
+ elif noise is None:
+ if randomize_noise:
+ noise = [None] * self.num_layers
+ else:
+ noise = [
+ getattr(self.noises, f'noise_{i}') for i in range(self.num_layers)
+ ]
+
+ if truncation < 1:
+ style_t = []
+
+ for style in styles:
+ style_t.append(
+ truncation_latent + truncation * (style - truncation_latent)
+ )
+
+ styles = style_t
+
+ if len(styles) < 2:
+ inject_index = self.n_latent
+
+ if styles[0].ndim < 3:
+ latent = styles[0].unsqueeze(1).repeat(1, inject_index, 1)
+ else:
+ latent = styles[0]
+
+ else:
+ if inject_index is None:
+ inject_index = random.randint(1, self.n_latent - 1)
+
+ latent = styles[0].unsqueeze(1).repeat(1, inject_index, 1)
+ latent2 = styles[1].unsqueeze(1).repeat(1, self.n_latent - inject_index, 1)
+
+ latent = torch.cat([latent, latent2], 1)
+
+ # w+ + v for video face editing
+ if editing_w is not None: ##### modified
+ latent = latent + editing_w
+
+ # the original StyleGAN
+ if first_layer_feature is None: ##### modified
+ out = self.input(latent)
+ out = F.adaptive_avg_pool2d(out, 32) ##### modified
+ out = self.conv1(out, latent[:, 0], noise=noise[0])
+ skip = self.to_rgb1(out, latent[:, 1])
+ # the default StyleGANEX, replacing the first layer of G
+ elif first_layer_feature_ind == 0: ##### modified
+ out = first_layer_feature[0] ##### modified
+ out = self.conv1(out, latent[:, 0], noise=noise[0])
+ skip = self.to_rgb1(out, latent[:, 1])
+ # maybe we can also use the second layer of G to accept f?
+ else: ##### modified
+ out = first_layer_feature[0] ##### modified
+ skip = first_layer_feature[1] ##### modified
+
+ i = 1
+ for conv1, conv2, noise1, noise2, to_rgb in zip(
+ self.convs[::2], self.convs[1::2], noise[1::2], noise[2::2], self.to_rgbs
+ ):
+ # these layers accepts skipped encoder layer, use fusion block to fuse the encoder feature and decoder feature
+ if skip_layer_feature and fusion_block and i//2 < len(skip_layer_feature) and i//2 < len(fusion_block):
+ if editing_w is None:
+ out, skip = fusion_block[i//2](skip_layer_feature[i//2], out, skip)
+ else:
+ out, skip = fusion_block[i//2](skip_layer_feature[i//2], out, skip, editing_w[:,i])
+ out = conv1(out, latent[:, i], noise=noise1)
+ out = conv2(out, latent[:, i + 1], noise=noise2)
+ skip = to_rgb(out, latent[:, i + 2], skip)
+
+ i += 2
+
+ image = skip
+
+ if return_latents:
+ return image, latent
+ elif return_features:
+ return image, out
+ else:
+ return image, None
+
+
+class ConvLayer(nn.Sequential):
+ def __init__(
+ self,
+ in_channel,
+ out_channel,
+ kernel_size,
+ downsample=False,
+ blur_kernel=[1, 3, 3, 1],
+ bias=True,
+ activate=True,
+ dilation=1, ## modified
+ ):
+ layers = []
+
+ if downsample:
+ factor = 2
+ p = (len(blur_kernel) - factor) + (kernel_size - 1)
+ pad0 = (p + 1) // 2
+ pad1 = p // 2
+
+ layers.append(Blur(blur_kernel, pad=(pad0, pad1)))
+
+ stride = 2
+ self.padding = 0
+
+ else:
+ stride = 1
+ self.padding = kernel_size // 2 + dilation-1 ## modified
+
+ layers.append(
+ EqualConv2d(
+ in_channel,
+ out_channel,
+ kernel_size,
+ padding=self.padding,
+ stride=stride,
+ bias=bias and not activate,
+ dilation=dilation, ## modified
+ )
+ )
+
+ if activate:
+ if bias:
+ layers.append(FusedLeakyReLU(out_channel))
+
+ else:
+ layers.append(ScaledLeakyReLU(0.2))
+
+ super().__init__(*layers)
+
+
+class ResBlock(nn.Module):
+ def __init__(self, in_channel, out_channel, blur_kernel=[1, 3, 3, 1]):
+ super().__init__()
+
+ self.conv1 = ConvLayer(in_channel, in_channel, 3)
+ self.conv2 = ConvLayer(in_channel, out_channel, 3, downsample=True)
+
+ self.skip = ConvLayer(
+ in_channel, out_channel, 1, downsample=True, activate=False, bias=False
+ )
+
+ def forward(self, input):
+ out = self.conv1(input)
+ out = self.conv2(out)
+
+ skip = self.skip(input)
+ out = (out + skip) / math.sqrt(2)
+
+ return out
+
+
+class Discriminator(nn.Module):
+ def __init__(self, size, channel_multiplier=2, blur_kernel=[1, 3, 3, 1], img_channel=3):
+ super().__init__()
+
+ channels = {
+ 4: 512,
+ 8: 512,
+ 16: 512,
+ 32: 512,
+ 64: 256 * channel_multiplier,
+ 128: 128 * channel_multiplier,
+ 256: 64 * channel_multiplier,
+ 512: 32 * channel_multiplier,
+ 1024: 16 * channel_multiplier,
+ }
+
+ convs = [ConvLayer(img_channel, channels[size], 1)]
+
+ log_size = int(math.log(size, 2))
+
+ in_channel = channels[size]
+
+ for i in range(log_size, 2, -1):
+ out_channel = channels[2 ** (i - 1)]
+
+ convs.append(ResBlock(in_channel, out_channel, blur_kernel))
+
+ in_channel = out_channel
+
+ self.convs = nn.Sequential(*convs)
+
+ self.stddev_group = 4
+ self.stddev_feat = 1
+
+ self.final_conv = ConvLayer(in_channel + 1, channels[4], 3)
+ self.final_linear = nn.Sequential(
+ EqualLinear(channels[4] * 4 * 4, channels[4], activation='fused_lrelu'),
+ EqualLinear(channels[4], 1),
+ )
+
+ self.size = size ##### modified
+
+ def forward(self, input):
+ # for input that not satisfies the target size, we crop it to extract a small image of the target size.
+ _, _, h, w = input.shape ##### modified
+ i, j = torch.randint(0, h+1-self.size, size=(1,)).item(), torch.randint(0, w+1-self.size, size=(1,)).item() ##### modified
+ out = self.convs(input[:,:,i:i+self.size,j:j+self.size]) ##### modified
+
+ batch, channel, height, width = out.shape
+ group = min(batch, self.stddev_group)
+ stddev = out.view(
+ group, -1, self.stddev_feat, channel // self.stddev_feat, height, width
+ )
+ stddev = torch.sqrt(stddev.var(0, unbiased=False) + 1e-8)
+ stddev = stddev.mean([2, 3, 4], keepdims=True).squeeze(2)
+ stddev = stddev.repeat(group, 1, height, width)
+ out = torch.cat([out, stddev], 1)
+
+ out = self.final_conv(out)
+
+ out = out.view(batch, -1)
+ out = self.final_linear(out)
+
+ return out
\ No newline at end of file
diff --git a/models/stylegan2/op/__init__.py b/models/stylegan2/op/__init__.py
new file mode 100644
index 0000000000000000000000000000000000000000..d0918d92285955855be89f00096b888ee5597ce3
--- /dev/null
+++ b/models/stylegan2/op/__init__.py
@@ -0,0 +1,2 @@
+from .fused_act import FusedLeakyReLU, fused_leaky_relu
+from .upfirdn2d import upfirdn2d
diff --git a/models/stylegan2/op/conv2d_gradfix.py b/models/stylegan2/op/conv2d_gradfix.py
new file mode 100644
index 0000000000000000000000000000000000000000..c4485b11991c5426939e87e6c363307eb9017438
--- /dev/null
+++ b/models/stylegan2/op/conv2d_gradfix.py
@@ -0,0 +1,227 @@
+import contextlib
+import warnings
+
+import torch
+from torch import autograd
+from torch.nn import functional as F
+
+enabled = True
+weight_gradients_disabled = False
+
+
+@contextlib.contextmanager
+def no_weight_gradients():
+ global weight_gradients_disabled
+
+ old = weight_gradients_disabled
+ weight_gradients_disabled = True
+ yield
+ weight_gradients_disabled = old
+
+
+def conv2d(input, weight, bias=None, stride=1, padding=0, dilation=1, groups=1):
+ if could_use_op(input):
+ return conv2d_gradfix(
+ transpose=False,
+ weight_shape=weight.shape,
+ stride=stride,
+ padding=padding,
+ output_padding=0,
+ dilation=dilation,
+ groups=groups,
+ ).apply(input, weight, bias)
+
+ return F.conv2d(
+ input=input,
+ weight=weight,
+ bias=bias,
+ stride=stride,
+ padding=padding,
+ dilation=dilation,
+ groups=groups,
+ )
+
+
+def conv_transpose2d(
+ input,
+ weight,
+ bias=None,
+ stride=1,
+ padding=0,
+ output_padding=0,
+ groups=1,
+ dilation=1,
+):
+ if could_use_op(input):
+ return conv2d_gradfix(
+ transpose=True,
+ weight_shape=weight.shape,
+ stride=stride,
+ padding=padding,
+ output_padding=output_padding,
+ groups=groups,
+ dilation=dilation,
+ ).apply(input, weight, bias)
+
+ return F.conv_transpose2d(
+ input=input,
+ weight=weight,
+ bias=bias,
+ stride=stride,
+ padding=padding,
+ output_padding=output_padding,
+ dilation=dilation,
+ groups=groups,
+ )
+
+
+def could_use_op(input):
+ if (not enabled) or (not torch.backends.cudnn.enabled):
+ return False
+
+ if input.device.type != "cuda":
+ return False
+
+ if any(torch.__version__.startswith(x) for x in ["1.7.", "1.8."]):
+ return True
+
+ warnings.warn(
+ f"conv2d_gradfix not supported on PyTorch {torch.__version__}. Falling back to torch.nn.functional.conv2d()."
+ )
+
+ return False
+
+
+def ensure_tuple(xs, ndim):
+ xs = tuple(xs) if isinstance(xs, (tuple, list)) else (xs,) * ndim
+
+ return xs
+
+
+conv2d_gradfix_cache = dict()
+
+
+def conv2d_gradfix(
+ transpose, weight_shape, stride, padding, output_padding, dilation, groups
+):
+ ndim = 2
+ weight_shape = tuple(weight_shape)
+ stride = ensure_tuple(stride, ndim)
+ padding = ensure_tuple(padding, ndim)
+ output_padding = ensure_tuple(output_padding, ndim)
+ dilation = ensure_tuple(dilation, ndim)
+
+ key = (transpose, weight_shape, stride, padding, output_padding, dilation, groups)
+ if key in conv2d_gradfix_cache:
+ return conv2d_gradfix_cache[key]
+
+ common_kwargs = dict(
+ stride=stride, padding=padding, dilation=dilation, groups=groups
+ )
+
+ def calc_output_padding(input_shape, output_shape):
+ if transpose:
+ return [0, 0]
+
+ return [
+ input_shape[i + 2]
+ - (output_shape[i + 2] - 1) * stride[i]
+ - (1 - 2 * padding[i])
+ - dilation[i] * (weight_shape[i + 2] - 1)
+ for i in range(ndim)
+ ]
+
+ class Conv2d(autograd.Function):
+ @staticmethod
+ def forward(ctx, input, weight, bias):
+ if not transpose:
+ out = F.conv2d(input=input, weight=weight, bias=bias, **common_kwargs)
+
+ else:
+ out = F.conv_transpose2d(
+ input=input,
+ weight=weight,
+ bias=bias,
+ output_padding=output_padding,
+ **common_kwargs,
+ )
+
+ ctx.save_for_backward(input, weight)
+
+ return out
+
+ @staticmethod
+ def backward(ctx, grad_output):
+ input, weight = ctx.saved_tensors
+ grad_input, grad_weight, grad_bias = None, None, None
+
+ if ctx.needs_input_grad[0]:
+ p = calc_output_padding(
+ input_shape=input.shape, output_shape=grad_output.shape
+ )
+ grad_input = conv2d_gradfix(
+ transpose=(not transpose),
+ weight_shape=weight_shape,
+ output_padding=p,
+ **common_kwargs,
+ ).apply(grad_output, weight, None)
+
+ if ctx.needs_input_grad[1] and not weight_gradients_disabled:
+ grad_weight = Conv2dGradWeight.apply(grad_output, input)
+
+ if ctx.needs_input_grad[2]:
+ grad_bias = grad_output.sum((0, 2, 3))
+
+ return grad_input, grad_weight, grad_bias
+
+ class Conv2dGradWeight(autograd.Function):
+ @staticmethod
+ def forward(ctx, grad_output, input):
+ op = torch._C._jit_get_operation(
+ "aten::cudnn_convolution_backward_weight"
+ if not transpose
+ else "aten::cudnn_convolution_transpose_backward_weight"
+ )
+ flags = [
+ torch.backends.cudnn.benchmark,
+ torch.backends.cudnn.deterministic,
+ torch.backends.cudnn.allow_tf32,
+ ]
+ grad_weight = op(
+ weight_shape,
+ grad_output,
+ input,
+ padding,
+ stride,
+ dilation,
+ groups,
+ *flags,
+ )
+ ctx.save_for_backward(grad_output, input)
+
+ return grad_weight
+
+ @staticmethod
+ def backward(ctx, grad_grad_weight):
+ grad_output, input = ctx.saved_tensors
+ grad_grad_output, grad_grad_input = None, None
+
+ if ctx.needs_input_grad[0]:
+ grad_grad_output = Conv2d.apply(input, grad_grad_weight, None)
+
+ if ctx.needs_input_grad[1]:
+ p = calc_output_padding(
+ input_shape=input.shape, output_shape=grad_output.shape
+ )
+ grad_grad_input = conv2d_gradfix(
+ transpose=(not transpose),
+ weight_shape=weight_shape,
+ output_padding=p,
+ **common_kwargs,
+ ).apply(grad_output, grad_grad_weight, None)
+
+ return grad_grad_output, grad_grad_input
+
+ conv2d_gradfix_cache[key] = Conv2d
+
+ return Conv2d
diff --git a/models/stylegan2/op/fused_act.py b/models/stylegan2/op/fused_act.py
new file mode 100644
index 0000000000000000000000000000000000000000..74815adafbf7a37d5d4def41ac60dbdeefdbff30
--- /dev/null
+++ b/models/stylegan2/op/fused_act.py
@@ -0,0 +1,34 @@
+import torch
+from torch import nn
+from torch.nn import functional as F
+
+
+class FusedLeakyReLU(nn.Module):
+ def __init__(self, channel, bias=True, negative_slope=0.2, scale=2 ** 0.5):
+ super().__init__()
+
+ if bias:
+ self.bias = nn.Parameter(torch.zeros(channel))
+
+ else:
+ self.bias = None
+
+ self.negative_slope = negative_slope
+ self.scale = scale
+
+ def forward(self, inputs):
+ return fused_leaky_relu(inputs, self.bias, self.negative_slope, self.scale)
+
+
+def fused_leaky_relu(inputs, bias=None, negative_slope=0.2, scale=2 ** 0.5):
+ if bias is not None:
+ rest_dim = [1] * (inputs.ndim - bias.ndim - 1)
+ return (
+ F.leaky_relu(
+ inputs + bias.view(1, bias.shape[0], *rest_dim), negative_slope=negative_slope
+ )
+ * scale
+ )
+
+ else:
+ return F.leaky_relu(inputs, negative_slope=negative_slope) * scale
\ No newline at end of file
diff --git a/models/stylegan2/op/readme.md b/models/stylegan2/op/readme.md
new file mode 100644
index 0000000000000000000000000000000000000000..7cffcfc72069ff9a098d292f9e37035031e19081
--- /dev/null
+++ b/models/stylegan2/op/readme.md
@@ -0,0 +1,12 @@
+Code from [rosinality-stylegan2-pytorch-cp](https://github.com/senior-sigan/rosinality-stylegan2-pytorch-cpu)
+
+Scripts to convert rosinality/stylegan2-pytorch to the CPU compatible format
+
+If you would like to use CPU for testing or have a problem regarding the cpp extention (fused and upfirdn2d), please make the following changes:
+
+Change `model.stylegan.op` to `model.stylegan.op_cpu`
+https://github.com/williamyang1991/VToonify/blob/01b383efc00007f9b069585db41a7d31a77a8806/util.py#L14
+
+https://github.com/williamyang1991/VToonify/blob/01b383efc00007f9b069585db41a7d31a77a8806/model/simple_augment.py#L12
+
+https://github.com/williamyang1991/VToonify/blob/01b383efc00007f9b069585db41a7d31a77a8806/model/stylegan/model.py#L11
diff --git a/models/stylegan2/op/upfirdn2d.py b/models/stylegan2/op/upfirdn2d.py
new file mode 100644
index 0000000000000000000000000000000000000000..d509eb5e11e8cd01468dded5e5b53f5326057706
--- /dev/null
+++ b/models/stylegan2/op/upfirdn2d.py
@@ -0,0 +1,61 @@
+from collections import abc
+
+import torch
+from torch.nn import functional as F
+
+
+def upfirdn2d(inputs, kernel, up=1, down=1, pad=(0, 0)):
+ if not isinstance(up, abc.Iterable):
+ up = (up, up)
+
+ if not isinstance(down, abc.Iterable):
+ down = (down, down)
+
+ if len(pad) == 2:
+ pad = (pad[0], pad[1], pad[0], pad[1])
+
+ return upfirdn2d_native(inputs, kernel, *up, *down, *pad)
+
+
+def upfirdn2d_native(
+ inputs, kernel, up_x, up_y, down_x, down_y, pad_x0, pad_x1, pad_y0, pad_y1
+):
+ _, channel, in_h, in_w = inputs.shape
+ inputs = inputs.reshape(-1, in_h, in_w, 1)
+
+ _, in_h, in_w, minor = inputs.shape
+ kernel_h, kernel_w = kernel.shape
+
+ out = inputs.view(-1, in_h, 1, in_w, 1, minor)
+ out = F.pad(out, [0, 0, 0, up_x - 1, 0, 0, 0, up_y - 1])
+ out = out.view(-1, in_h * up_y, in_w * up_x, minor)
+
+ out = F.pad(
+ out, [0, 0, max(pad_x0, 0), max(pad_x1, 0), max(pad_y0, 0), max(pad_y1, 0)]
+ )
+ out = out[
+ :,
+ max(-pad_y0, 0): out.shape[1] - max(-pad_y1, 0),
+ max(-pad_x0, 0): out.shape[2] - max(-pad_x1, 0),
+ :,
+ ]
+
+ out = out.permute(0, 3, 1, 2)
+ out = out.reshape(
+ [-1, 1, in_h * up_y + pad_y0 + pad_y1, in_w * up_x + pad_x0 + pad_x1]
+ )
+ w = torch.flip(kernel, [0, 1]).view(1, 1, kernel_h, kernel_w)
+ out = F.conv2d(out, w)
+ out = out.reshape(
+ -1,
+ minor,
+ in_h * up_y + pad_y0 + pad_y1 - kernel_h + 1,
+ in_w * up_x + pad_x0 + pad_x1 - kernel_w + 1,
+ )
+ out = out.permute(0, 2, 3, 1)
+ out = out[:, ::down_y, ::down_x, :]
+
+ out_h = (in_h * up_y + pad_y0 + pad_y1 - kernel_h + down_y) // down_y
+ out_w = (in_w * up_x + pad_x0 + pad_x1 - kernel_w + down_x) // down_x
+
+ return out.view(-1, channel, out_h, out_w)
\ No newline at end of file
diff --git a/models/stylegan2/op_ori/__init__.py b/models/stylegan2/op_ori/__init__.py
new file mode 100644
index 0000000000000000000000000000000000000000..d0918d92285955855be89f00096b888ee5597ce3
--- /dev/null
+++ b/models/stylegan2/op_ori/__init__.py
@@ -0,0 +1,2 @@
+from .fused_act import FusedLeakyReLU, fused_leaky_relu
+from .upfirdn2d import upfirdn2d
diff --git a/models/stylegan2/op_ori/fused_act.py b/models/stylegan2/op_ori/fused_act.py
new file mode 100644
index 0000000000000000000000000000000000000000..973a84fffde53668d31397da5fb993bbc95f7be0
--- /dev/null
+++ b/models/stylegan2/op_ori/fused_act.py
@@ -0,0 +1,85 @@
+import os
+
+import torch
+from torch import nn
+from torch.autograd import Function
+from torch.utils.cpp_extension import load
+
+module_path = os.path.dirname(__file__)
+fused = load(
+ 'fused',
+ sources=[
+ os.path.join(module_path, 'fused_bias_act.cpp'),
+ os.path.join(module_path, 'fused_bias_act_kernel.cu'),
+ ],
+)
+
+
+class FusedLeakyReLUFunctionBackward(Function):
+ @staticmethod
+ def forward(ctx, grad_output, out, negative_slope, scale):
+ ctx.save_for_backward(out)
+ ctx.negative_slope = negative_slope
+ ctx.scale = scale
+
+ empty = grad_output.new_empty(0)
+
+ grad_input = fused.fused_bias_act(
+ grad_output, empty, out, 3, 1, negative_slope, scale
+ )
+
+ dim = [0]
+
+ if grad_input.ndim > 2:
+ dim += list(range(2, grad_input.ndim))
+
+ grad_bias = grad_input.sum(dim).detach()
+
+ return grad_input, grad_bias
+
+ @staticmethod
+ def backward(ctx, gradgrad_input, gradgrad_bias):
+ out, = ctx.saved_tensors
+ gradgrad_out = fused.fused_bias_act(
+ gradgrad_input, gradgrad_bias, out, 3, 1, ctx.negative_slope, ctx.scale
+ )
+
+ return gradgrad_out, None, None, None
+
+
+class FusedLeakyReLUFunction(Function):
+ @staticmethod
+ def forward(ctx, input, bias, negative_slope, scale):
+ empty = input.new_empty(0)
+ out = fused.fused_bias_act(input, bias, empty, 3, 0, negative_slope, scale)
+ ctx.save_for_backward(out)
+ ctx.negative_slope = negative_slope
+ ctx.scale = scale
+
+ return out
+
+ @staticmethod
+ def backward(ctx, grad_output):
+ out, = ctx.saved_tensors
+
+ grad_input, grad_bias = FusedLeakyReLUFunctionBackward.apply(
+ grad_output, out, ctx.negative_slope, ctx.scale
+ )
+
+ return grad_input, grad_bias, None, None
+
+
+class FusedLeakyReLU(nn.Module):
+ def __init__(self, channel, negative_slope=0.2, scale=2 ** 0.5):
+ super().__init__()
+
+ self.bias = nn.Parameter(torch.zeros(channel))
+ self.negative_slope = negative_slope
+ self.scale = scale
+
+ def forward(self, input):
+ return fused_leaky_relu(input, self.bias, self.negative_slope, self.scale)
+
+
+def fused_leaky_relu(input, bias, negative_slope=0.2, scale=2 ** 0.5):
+ return FusedLeakyReLUFunction.apply(input, bias, negative_slope, scale)
diff --git a/models/stylegan2/op_ori/fused_bias_act.cpp b/models/stylegan2/op_ori/fused_bias_act.cpp
new file mode 100644
index 0000000000000000000000000000000000000000..02be898f970bcc8ea297867fcaa4e71b24b3d949
--- /dev/null
+++ b/models/stylegan2/op_ori/fused_bias_act.cpp
@@ -0,0 +1,21 @@
+#include
+
+
+torch::Tensor fused_bias_act_op(const torch::Tensor& input, const torch::Tensor& bias, const torch::Tensor& refer,
+ int act, int grad, float alpha, float scale);
+
+#define CHECK_CUDA(x) TORCH_CHECK(x.type().is_cuda(), #x " must be a CUDA tensor")
+#define CHECK_CONTIGUOUS(x) TORCH_CHECK(x.is_contiguous(), #x " must be contiguous")
+#define CHECK_INPUT(x) CHECK_CUDA(x); CHECK_CONTIGUOUS(x)
+
+torch::Tensor fused_bias_act(const torch::Tensor& input, const torch::Tensor& bias, const torch::Tensor& refer,
+ int act, int grad, float alpha, float scale) {
+ CHECK_CUDA(input);
+ CHECK_CUDA(bias);
+
+ return fused_bias_act_op(input, bias, refer, act, grad, alpha, scale);
+}
+
+PYBIND11_MODULE(TORCH_EXTENSION_NAME, m) {
+ m.def("fused_bias_act", &fused_bias_act, "fused bias act (CUDA)");
+}
\ No newline at end of file
diff --git a/models/stylegan2/op_ori/fused_bias_act_kernel.cu b/models/stylegan2/op_ori/fused_bias_act_kernel.cu
new file mode 100644
index 0000000000000000000000000000000000000000..c9fa56fea7ede7072dc8925cfb0148f136eb85b8
--- /dev/null
+++ b/models/stylegan2/op_ori/fused_bias_act_kernel.cu
@@ -0,0 +1,99 @@
+// Copyright (c) 2019, NVIDIA Corporation. All rights reserved.
+//
+// This work is made available under the Nvidia Source Code License-NC.
+// To view a copy of this license, visit
+// https://nvlabs.github.io/stylegan2/license.html
+
+#include
+
+#include
+#include
+#include
+#include
+
+#include
+#include
+
+
+template
+static __global__ void fused_bias_act_kernel(scalar_t* out, const scalar_t* p_x, const scalar_t* p_b, const scalar_t* p_ref,
+ int act, int grad, scalar_t alpha, scalar_t scale, int loop_x, int size_x, int step_b, int size_b, int use_bias, int use_ref) {
+ int xi = blockIdx.x * loop_x * blockDim.x + threadIdx.x;
+
+ scalar_t zero = 0.0;
+
+ for (int loop_idx = 0; loop_idx < loop_x && xi < size_x; loop_idx++, xi += blockDim.x) {
+ scalar_t x = p_x[xi];
+
+ if (use_bias) {
+ x += p_b[(xi / step_b) % size_b];
+ }
+
+ scalar_t ref = use_ref ? p_ref[xi] : zero;
+
+ scalar_t y;
+
+ switch (act * 10 + grad) {
+ default:
+ case 10: y = x; break;
+ case 11: y = x; break;
+ case 12: y = 0.0; break;
+
+ case 30: y = (x > 0.0) ? x : x * alpha; break;
+ case 31: y = (ref > 0.0) ? x : x * alpha; break;
+ case 32: y = 0.0; break;
+ }
+
+ out[xi] = y * scale;
+ }
+}
+
+
+torch::Tensor fused_bias_act_op(const torch::Tensor& input, const torch::Tensor& bias, const torch::Tensor& refer,
+ int act, int grad, float alpha, float scale) {
+ int curDevice = -1;
+ cudaGetDevice(&curDevice);
+ cudaStream_t stream = at::cuda::getCurrentCUDAStream(curDevice);
+
+ auto x = input.contiguous();
+ auto b = bias.contiguous();
+ auto ref = refer.contiguous();
+
+ int use_bias = b.numel() ? 1 : 0;
+ int use_ref = ref.numel() ? 1 : 0;
+
+ int size_x = x.numel();
+ int size_b = b.numel();
+ int step_b = 1;
+
+ for (int i = 1 + 1; i < x.dim(); i++) {
+ step_b *= x.size(i);
+ }
+
+ int loop_x = 4;
+ int block_size = 4 * 32;
+ int grid_size = (size_x - 1) / (loop_x * block_size) + 1;
+
+ auto y = torch::empty_like(x);
+
+ AT_DISPATCH_FLOATING_TYPES_AND_HALF(x.scalar_type(), "fused_bias_act_kernel", [&] {
+ fused_bias_act_kernel<<>>(
+ y.data_ptr(),
+ x.data_ptr(),
+ b.data_ptr(),
+ ref.data_ptr(),
+ act,
+ grad,
+ alpha,
+ scale,
+ loop_x,
+ size_x,
+ step_b,
+ size_b,
+ use_bias,
+ use_ref
+ );
+ });
+
+ return y;
+}
\ No newline at end of file
diff --git a/models/stylegan2/op_ori/upfirdn2d.cpp b/models/stylegan2/op_ori/upfirdn2d.cpp
new file mode 100644
index 0000000000000000000000000000000000000000..d2e633dc896433c205e18bc3e455539192ff968e
--- /dev/null
+++ b/models/stylegan2/op_ori/upfirdn2d.cpp
@@ -0,0 +1,23 @@
+#include
+
+
+torch::Tensor upfirdn2d_op(const torch::Tensor& input, const torch::Tensor& kernel,
+ int up_x, int up_y, int down_x, int down_y,
+ int pad_x0, int pad_x1, int pad_y0, int pad_y1);
+
+#define CHECK_CUDA(x) TORCH_CHECK(x.type().is_cuda(), #x " must be a CUDA tensor")
+#define CHECK_CONTIGUOUS(x) TORCH_CHECK(x.is_contiguous(), #x " must be contiguous")
+#define CHECK_INPUT(x) CHECK_CUDA(x); CHECK_CONTIGUOUS(x)
+
+torch::Tensor upfirdn2d(const torch::Tensor& input, const torch::Tensor& kernel,
+ int up_x, int up_y, int down_x, int down_y,
+ int pad_x0, int pad_x1, int pad_y0, int pad_y1) {
+ CHECK_CUDA(input);
+ CHECK_CUDA(kernel);
+
+ return upfirdn2d_op(input, kernel, up_x, up_y, down_x, down_y, pad_x0, pad_x1, pad_y0, pad_y1);
+}
+
+PYBIND11_MODULE(TORCH_EXTENSION_NAME, m) {
+ m.def("upfirdn2d", &upfirdn2d, "upfirdn2d (CUDA)");
+}
\ No newline at end of file
diff --git a/models/stylegan2/op_ori/upfirdn2d.py b/models/stylegan2/op_ori/upfirdn2d.py
new file mode 100644
index 0000000000000000000000000000000000000000..e9cb52219689592e2745600abb19fad02740a139
--- /dev/null
+++ b/models/stylegan2/op_ori/upfirdn2d.py
@@ -0,0 +1,184 @@
+import os
+
+import torch
+from torch.autograd import Function
+from torch.utils.cpp_extension import load
+
+module_path = os.path.dirname(__file__)
+upfirdn2d_op = load(
+ 'upfirdn2d',
+ sources=[
+ os.path.join(module_path, 'upfirdn2d.cpp'),
+ os.path.join(module_path, 'upfirdn2d_kernel.cu'),
+ ],
+)
+
+
+class UpFirDn2dBackward(Function):
+ @staticmethod
+ def forward(
+ ctx, grad_output, kernel, grad_kernel, up, down, pad, g_pad, in_size, out_size
+ ):
+ up_x, up_y = up
+ down_x, down_y = down
+ g_pad_x0, g_pad_x1, g_pad_y0, g_pad_y1 = g_pad
+
+ grad_output = grad_output.reshape(-1, out_size[0], out_size[1], 1)
+
+ grad_input = upfirdn2d_op.upfirdn2d(
+ grad_output,
+ grad_kernel,
+ down_x,
+ down_y,
+ up_x,
+ up_y,
+ g_pad_x0,
+ g_pad_x1,
+ g_pad_y0,
+ g_pad_y1,
+ )
+ grad_input = grad_input.view(in_size[0], in_size[1], in_size[2], in_size[3])
+
+ ctx.save_for_backward(kernel)
+
+ pad_x0, pad_x1, pad_y0, pad_y1 = pad
+
+ ctx.up_x = up_x
+ ctx.up_y = up_y
+ ctx.down_x = down_x
+ ctx.down_y = down_y
+ ctx.pad_x0 = pad_x0
+ ctx.pad_x1 = pad_x1
+ ctx.pad_y0 = pad_y0
+ ctx.pad_y1 = pad_y1
+ ctx.in_size = in_size
+ ctx.out_size = out_size
+
+ return grad_input
+
+ @staticmethod
+ def backward(ctx, gradgrad_input):
+ kernel, = ctx.saved_tensors
+
+ gradgrad_input = gradgrad_input.reshape(-1, ctx.in_size[2], ctx.in_size[3], 1)
+
+ gradgrad_out = upfirdn2d_op.upfirdn2d(
+ gradgrad_input,
+ kernel,
+ ctx.up_x,
+ ctx.up_y,
+ ctx.down_x,
+ ctx.down_y,
+ ctx.pad_x0,
+ ctx.pad_x1,
+ ctx.pad_y0,
+ ctx.pad_y1,
+ )
+ # gradgrad_out = gradgrad_out.view(ctx.in_size[0], ctx.out_size[0], ctx.out_size[1], ctx.in_size[3])
+ gradgrad_out = gradgrad_out.view(
+ ctx.in_size[0], ctx.in_size[1], ctx.out_size[0], ctx.out_size[1]
+ )
+
+ return gradgrad_out, None, None, None, None, None, None, None, None
+
+
+class UpFirDn2d(Function):
+ @staticmethod
+ def forward(ctx, input, kernel, up, down, pad):
+ up_x, up_y = up
+ down_x, down_y = down
+ pad_x0, pad_x1, pad_y0, pad_y1 = pad
+
+ kernel_h, kernel_w = kernel.shape
+ batch, channel, in_h, in_w = input.shape
+ ctx.in_size = input.shape
+
+ input = input.reshape(-1, in_h, in_w, 1)
+
+ ctx.save_for_backward(kernel, torch.flip(kernel, [0, 1]))
+
+ out_h = (in_h * up_y + pad_y0 + pad_y1 - kernel_h) // down_y + 1
+ out_w = (in_w * up_x + pad_x0 + pad_x1 - kernel_w) // down_x + 1
+ ctx.out_size = (out_h, out_w)
+
+ ctx.up = (up_x, up_y)
+ ctx.down = (down_x, down_y)
+ ctx.pad = (pad_x0, pad_x1, pad_y0, pad_y1)
+
+ g_pad_x0 = kernel_w - pad_x0 - 1
+ g_pad_y0 = kernel_h - pad_y0 - 1
+ g_pad_x1 = in_w * up_x - out_w * down_x + pad_x0 - up_x + 1
+ g_pad_y1 = in_h * up_y - out_h * down_y + pad_y0 - up_y + 1
+
+ ctx.g_pad = (g_pad_x0, g_pad_x1, g_pad_y0, g_pad_y1)
+
+ out = upfirdn2d_op.upfirdn2d(
+ input, kernel, up_x, up_y, down_x, down_y, pad_x0, pad_x1, pad_y0, pad_y1
+ )
+ # out = out.view(major, out_h, out_w, minor)
+ out = out.view(-1, channel, out_h, out_w)
+
+ return out
+
+ @staticmethod
+ def backward(ctx, grad_output):
+ kernel, grad_kernel = ctx.saved_tensors
+
+ grad_input = UpFirDn2dBackward.apply(
+ grad_output,
+ kernel,
+ grad_kernel,
+ ctx.up,
+ ctx.down,
+ ctx.pad,
+ ctx.g_pad,
+ ctx.in_size,
+ ctx.out_size,
+ )
+
+ return grad_input, None, None, None, None
+
+
+def upfirdn2d(input, kernel, up=1, down=1, pad=(0, 0)):
+ out = UpFirDn2d.apply(
+ input, kernel, (up, up), (down, down), (pad[0], pad[1], pad[0], pad[1])
+ )
+
+ return out
+
+
+def upfirdn2d_native(
+ input, kernel, up_x, up_y, down_x, down_y, pad_x0, pad_x1, pad_y0, pad_y1
+):
+ _, in_h, in_w, minor = input.shape
+ kernel_h, kernel_w = kernel.shape
+
+ out = input.view(-1, in_h, 1, in_w, 1, minor)
+ out = F.pad(out, [0, 0, 0, up_x - 1, 0, 0, 0, up_y - 1])
+ out = out.view(-1, in_h * up_y, in_w * up_x, minor)
+
+ out = F.pad(
+ out, [0, 0, max(pad_x0, 0), max(pad_x1, 0), max(pad_y0, 0), max(pad_y1, 0)]
+ )
+ out = out[
+ :,
+ max(-pad_y0, 0): out.shape[1] - max(-pad_y1, 0),
+ max(-pad_x0, 0): out.shape[2] - max(-pad_x1, 0),
+ :,
+ ]
+
+ out = out.permute(0, 3, 1, 2)
+ out = out.reshape(
+ [-1, 1, in_h * up_y + pad_y0 + pad_y1, in_w * up_x + pad_x0 + pad_x1]
+ )
+ w = torch.flip(kernel, [0, 1]).view(1, 1, kernel_h, kernel_w)
+ out = F.conv2d(out, w)
+ out = out.reshape(
+ -1,
+ minor,
+ in_h * up_y + pad_y0 + pad_y1 - kernel_h + 1,
+ in_w * up_x + pad_x0 + pad_x1 - kernel_w + 1,
+ )
+ out = out.permute(0, 2, 3, 1)
+
+ return out[:, ::down_y, ::down_x, :]
\ No newline at end of file
diff --git a/models/stylegan2/op_ori/upfirdn2d_kernel.cu b/models/stylegan2/op_ori/upfirdn2d_kernel.cu
new file mode 100644
index 0000000000000000000000000000000000000000..2a710aa6adc3d43ac93136a1814e3c39970e1c7e
--- /dev/null
+++ b/models/stylegan2/op_ori/upfirdn2d_kernel.cu
@@ -0,0 +1,272 @@
+// Copyright (c) 2019, NVIDIA Corporation. All rights reserved.
+//
+// This work is made available under the Nvidia Source Code License-NC.
+// To view a copy of this license, visit
+// https://nvlabs.github.io/stylegan2/license.html
+
+#include
+
+#include
+#include
+#include
+#include
+
+#include
+#include
+
+
+static __host__ __device__ __forceinline__ int floor_div(int a, int b) {
+ int c = a / b;
+
+ if (c * b > a) {
+ c--;
+ }
+
+ return c;
+}
+
+
+struct UpFirDn2DKernelParams {
+ int up_x;
+ int up_y;
+ int down_x;
+ int down_y;
+ int pad_x0;
+ int pad_x1;
+ int pad_y0;
+ int pad_y1;
+
+ int major_dim;
+ int in_h;
+ int in_w;
+ int minor_dim;
+ int kernel_h;
+ int kernel_w;
+ int out_h;
+ int out_w;
+ int loop_major;
+ int loop_x;
+};
+
+
+template
+__global__ void upfirdn2d_kernel(scalar_t* out, const scalar_t* input, const scalar_t* kernel, const UpFirDn2DKernelParams p) {
+ const int tile_in_h = ((tile_out_h - 1) * down_y + kernel_h - 1) / up_y + 1;
+ const int tile_in_w = ((tile_out_w - 1) * down_x + kernel_w - 1) / up_x + 1;
+
+ __shared__ volatile float sk[kernel_h][kernel_w];
+ __shared__ volatile float sx[tile_in_h][tile_in_w];
+
+ int minor_idx = blockIdx.x;
+ int tile_out_y = minor_idx / p.minor_dim;
+ minor_idx -= tile_out_y * p.minor_dim;
+ tile_out_y *= tile_out_h;
+ int tile_out_x_base = blockIdx.y * p.loop_x * tile_out_w;
+ int major_idx_base = blockIdx.z * p.loop_major;
+
+ if (tile_out_x_base >= p.out_w | tile_out_y >= p.out_h | major_idx_base >= p.major_dim) {
+ return;
+ }
+
+ for (int tap_idx = threadIdx.x; tap_idx < kernel_h * kernel_w; tap_idx += blockDim.x) {
+ int ky = tap_idx / kernel_w;
+ int kx = tap_idx - ky * kernel_w;
+ scalar_t v = 0.0;
+
+ if (kx < p.kernel_w & ky < p.kernel_h) {
+ v = kernel[(p.kernel_h - 1 - ky) * p.kernel_w + (p.kernel_w - 1 - kx)];
+ }
+
+ sk[ky][kx] = v;
+ }
+
+ for (int loop_major = 0, major_idx = major_idx_base; loop_major < p.loop_major & major_idx < p.major_dim; loop_major++, major_idx++) {
+ for (int loop_x = 0, tile_out_x = tile_out_x_base; loop_x < p.loop_x & tile_out_x < p.out_w; loop_x++, tile_out_x += tile_out_w) {
+ int tile_mid_x = tile_out_x * down_x + up_x - 1 - p.pad_x0;
+ int tile_mid_y = tile_out_y * down_y + up_y - 1 - p.pad_y0;
+ int tile_in_x = floor_div(tile_mid_x, up_x);
+ int tile_in_y = floor_div(tile_mid_y, up_y);
+
+ __syncthreads();
+
+ for (int in_idx = threadIdx.x; in_idx < tile_in_h * tile_in_w; in_idx += blockDim.x) {
+ int rel_in_y = in_idx / tile_in_w;
+ int rel_in_x = in_idx - rel_in_y * tile_in_w;
+ int in_x = rel_in_x + tile_in_x;
+ int in_y = rel_in_y + tile_in_y;
+
+ scalar_t v = 0.0;
+
+ if (in_x >= 0 & in_y >= 0 & in_x < p.in_w & in_y < p.in_h) {
+ v = input[((major_idx * p.in_h + in_y) * p.in_w + in_x) * p.minor_dim + minor_idx];
+ }
+
+ sx[rel_in_y][rel_in_x] = v;
+ }
+
+ __syncthreads();
+ for (int out_idx = threadIdx.x; out_idx < tile_out_h * tile_out_w; out_idx += blockDim.x) {
+ int rel_out_y = out_idx / tile_out_w;
+ int rel_out_x = out_idx - rel_out_y * tile_out_w;
+ int out_x = rel_out_x + tile_out_x;
+ int out_y = rel_out_y + tile_out_y;
+
+ int mid_x = tile_mid_x + rel_out_x * down_x;
+ int mid_y = tile_mid_y + rel_out_y * down_y;
+ int in_x = floor_div(mid_x, up_x);
+ int in_y = floor_div(mid_y, up_y);
+ int rel_in_x = in_x - tile_in_x;
+ int rel_in_y = in_y - tile_in_y;
+ int kernel_x = (in_x + 1) * up_x - mid_x - 1;
+ int kernel_y = (in_y + 1) * up_y - mid_y - 1;
+
+ scalar_t v = 0.0;
+
+ #pragma unroll
+ for (int y = 0; y < kernel_h / up_y; y++)
+ #pragma unroll
+ for (int x = 0; x < kernel_w / up_x; x++)
+ v += sx[rel_in_y + y][rel_in_x + x] * sk[kernel_y + y * up_y][kernel_x + x * up_x];
+
+ if (out_x < p.out_w & out_y < p.out_h) {
+ out[((major_idx * p.out_h + out_y) * p.out_w + out_x) * p.minor_dim + minor_idx] = v;
+ }
+ }
+ }
+ }
+}
+
+
+torch::Tensor upfirdn2d_op(const torch::Tensor& input, const torch::Tensor& kernel,
+ int up_x, int up_y, int down_x, int down_y,
+ int pad_x0, int pad_x1, int pad_y0, int pad_y1) {
+ int curDevice = -1;
+ cudaGetDevice(&curDevice);
+ cudaStream_t stream = at::cuda::getCurrentCUDAStream(curDevice);
+
+ UpFirDn2DKernelParams p;
+
+ auto x = input.contiguous();
+ auto k = kernel.contiguous();
+
+ p.major_dim = x.size(0);
+ p.in_h = x.size(1);
+ p.in_w = x.size(2);
+ p.minor_dim = x.size(3);
+ p.kernel_h = k.size(0);
+ p.kernel_w = k.size(1);
+ p.up_x = up_x;
+ p.up_y = up_y;
+ p.down_x = down_x;
+ p.down_y = down_y;
+ p.pad_x0 = pad_x0;
+ p.pad_x1 = pad_x1;
+ p.pad_y0 = pad_y0;
+ p.pad_y1 = pad_y1;
+
+ p.out_h = (p.in_h * p.up_y + p.pad_y0 + p.pad_y1 - p.kernel_h + p.down_y) / p.down_y;
+ p.out_w = (p.in_w * p.up_x + p.pad_x0 + p.pad_x1 - p.kernel_w + p.down_x) / p.down_x;
+
+ auto out = at::empty({p.major_dim, p.out_h, p.out_w, p.minor_dim}, x.options());
+
+ int mode = -1;
+
+ int tile_out_h;
+ int tile_out_w;
+
+ if (p.up_x == 1 && p.up_y == 1 && p.down_x == 1 && p.down_y == 1 && p.kernel_h <= 4 && p.kernel_w <= 4) {
+ mode = 1;
+ tile_out_h = 16;
+ tile_out_w = 64;
+ }
+
+ if (p.up_x == 1 && p.up_y == 1 && p.down_x == 1 && p.down_y == 1 && p.kernel_h <= 3 && p.kernel_w <= 3) {
+ mode = 2;
+ tile_out_h = 16;
+ tile_out_w = 64;
+ }
+
+ if (p.up_x == 2 && p.up_y == 2 && p.down_x == 1 && p.down_y == 1 && p.kernel_h <= 4 && p.kernel_w <= 4) {
+ mode = 3;
+ tile_out_h = 16;
+ tile_out_w = 64;
+ }
+
+ if (p.up_x == 2 && p.up_y == 2 && p.down_x == 1 && p.down_y == 1 && p.kernel_h <= 2 && p.kernel_w <= 2) {
+ mode = 4;
+ tile_out_h = 16;
+ tile_out_w = 64;
+ }
+
+ if (p.up_x == 1 && p.up_y == 1 && p.down_x == 2 && p.down_y == 2 && p.kernel_h <= 4 && p.kernel_w <= 4) {
+ mode = 5;
+ tile_out_h = 8;
+ tile_out_w = 32;
+ }
+
+ if (p.up_x == 1 && p.up_y == 1 && p.down_x == 2 && p.down_y == 2 && p.kernel_h <= 2 && p.kernel_w <= 2) {
+ mode = 6;
+ tile_out_h = 8;
+ tile_out_w = 32;
+ }
+
+ dim3 block_size;
+ dim3 grid_size;
+
+ if (tile_out_h > 0 && tile_out_w) {
+ p.loop_major = (p.major_dim - 1) / 16384 + 1;
+ p.loop_x = 1;
+ block_size = dim3(32 * 8, 1, 1);
+ grid_size = dim3(((p.out_h - 1) / tile_out_h + 1) * p.minor_dim,
+ (p.out_w - 1) / (p.loop_x * tile_out_w) + 1,
+ (p.major_dim - 1) / p.loop_major + 1);
+ }
+
+ AT_DISPATCH_FLOATING_TYPES_AND_HALF(x.scalar_type(), "upfirdn2d_cuda", [&] {
+ switch (mode) {
+ case 1:
+ upfirdn2d_kernel<<>>(
+ out.data_ptr(), x.data_ptr(), k.data_ptr(), p
+ );
+
+ break;
+
+ case 2:
+ upfirdn2d_kernel<<>>(
+ out.data_ptr(), x.data_ptr(), k.data_ptr(), p
+ );
+
+ break;
+
+ case 3:
+ upfirdn2d_kernel<<>>(
+ out.data_ptr(), x.data_ptr(), k.data_ptr(), p
+ );
+
+ break;
+
+ case 4:
+ upfirdn2d_kernel<<>>(
+ out.data_ptr(), x.data_ptr(), k.data_ptr(), p
+ );
+
+ break;
+
+ case 5:
+ upfirdn2d_kernel<<>>(
+ out.data_ptr(), x.data_ptr(), k.data_ptr(), p
+ );
+
+ break;
+
+ case 6:
+ upfirdn2d_kernel<<>>(
+ out.data_ptr(), x.data_ptr(), k.data_ptr(), p
+ );
+
+ break;
+ }
+ });
+
+ return out;
+}
\ No newline at end of file
diff --git a/models/stylegan2/simple_augment.py b/models/stylegan2/simple_augment.py
new file mode 100644
index 0000000000000000000000000000000000000000..77776cd134046dc012e021d0ab80c1e0b90d2275
--- /dev/null
+++ b/models/stylegan2/simple_augment.py
@@ -0,0 +1,478 @@
+import math
+
+import torch
+from torch import autograd
+from torch.nn import functional as F
+import numpy as np
+
+from torch import distributed as dist
+#from distributed import reduce_sum
+from models.stylegan2.op2 import upfirdn2d
+
+def reduce_sum(tensor):
+ if not dist.is_available():
+ return tensor
+
+ if not dist.is_initialized():
+ return tensor
+
+ tensor = tensor.clone()
+ dist.all_reduce(tensor, op=dist.ReduceOp.SUM)
+
+ return tensor
+
+
+class AdaptiveAugment:
+ def __init__(self, ada_aug_target, ada_aug_len, update_every, device):
+ self.ada_aug_target = ada_aug_target
+ self.ada_aug_len = ada_aug_len
+ self.update_every = update_every
+
+ self.ada_update = 0
+ self.ada_aug_buf = torch.tensor([0.0, 0.0], device=device)
+ self.r_t_stat = 0
+ self.ada_aug_p = 0
+
+ @torch.no_grad()
+ def tune(self, real_pred):
+ self.ada_aug_buf += torch.tensor(
+ (torch.sign(real_pred).sum().item(), real_pred.shape[0]),
+ device=real_pred.device,
+ )
+ self.ada_update += 1
+
+ if self.ada_update % self.update_every == 0:
+ self.ada_aug_buf = reduce_sum(self.ada_aug_buf)
+ pred_signs, n_pred = self.ada_aug_buf.tolist()
+
+ self.r_t_stat = pred_signs / n_pred
+
+ if self.r_t_stat > self.ada_aug_target:
+ sign = 1
+
+ else:
+ sign = -1
+
+ self.ada_aug_p += sign * n_pred / self.ada_aug_len
+ self.ada_aug_p = min(1, max(0, self.ada_aug_p))
+ self.ada_aug_buf.mul_(0)
+ self.ada_update = 0
+
+ return self.ada_aug_p
+
+
+SYM6 = (
+ 0.015404109327027373,
+ 0.0034907120842174702,
+ -0.11799011114819057,
+ -0.048311742585633,
+ 0.4910559419267466,
+ 0.787641141030194,
+ 0.3379294217276218,
+ -0.07263752278646252,
+ -0.021060292512300564,
+ 0.04472490177066578,
+ 0.0017677118642428036,
+ -0.007800708325034148,
+)
+
+
+def translate_mat(t_x, t_y, device="cpu"):
+ batch = t_x.shape[0]
+
+ mat = torch.eye(3, device=device).unsqueeze(0).repeat(batch, 1, 1)
+ translate = torch.stack((t_x, t_y), 1)
+ mat[:, :2, 2] = translate
+
+ return mat
+
+
+def rotate_mat(theta, device="cpu"):
+ batch = theta.shape[0]
+
+ mat = torch.eye(3, device=device).unsqueeze(0).repeat(batch, 1, 1)
+ sin_t = torch.sin(theta)
+ cos_t = torch.cos(theta)
+ rot = torch.stack((cos_t, -sin_t, sin_t, cos_t), 1).view(batch, 2, 2)
+ mat[:, :2, :2] = rot
+
+ return mat
+
+
+def scale_mat(s_x, s_y, device="cpu"):
+ batch = s_x.shape[0]
+
+ mat = torch.eye(3, device=device).unsqueeze(0).repeat(batch, 1, 1)
+ mat[:, 0, 0] = s_x
+ mat[:, 1, 1] = s_y
+
+ return mat
+
+
+def translate3d_mat(t_x, t_y, t_z):
+ batch = t_x.shape[0]
+
+ mat = torch.eye(4).unsqueeze(0).repeat(batch, 1, 1)
+ translate = torch.stack((t_x, t_y, t_z), 1)
+ mat[:, :3, 3] = translate
+
+ return mat
+
+
+def rotate3d_mat(axis, theta):
+ batch = theta.shape[0]
+
+ u_x, u_y, u_z = axis
+
+ eye = torch.eye(3).unsqueeze(0)
+ cross = torch.tensor([(0, -u_z, u_y), (u_z, 0, -u_x), (-u_y, u_x, 0)]).unsqueeze(0)
+ outer = torch.tensor(axis)
+ outer = (outer.unsqueeze(1) * outer).unsqueeze(0)
+
+ sin_t = torch.sin(theta).view(-1, 1, 1)
+ cos_t = torch.cos(theta).view(-1, 1, 1)
+
+ rot = cos_t * eye + sin_t * cross + (1 - cos_t) * outer
+
+ eye_4 = torch.eye(4).unsqueeze(0).repeat(batch, 1, 1)
+ eye_4[:, :3, :3] = rot
+
+ return eye_4
+
+
+def scale3d_mat(s_x, s_y, s_z):
+ batch = s_x.shape[0]
+
+ mat = torch.eye(4).unsqueeze(0).repeat(batch, 1, 1)
+ mat[:, 0, 0] = s_x
+ mat[:, 1, 1] = s_y
+ mat[:, 2, 2] = s_z
+
+ return mat
+
+
+def luma_flip_mat(axis, i):
+ batch = i.shape[0]
+
+ eye = torch.eye(4).unsqueeze(0).repeat(batch, 1, 1)
+ axis = torch.tensor(axis + (0,))
+ flip = 2 * torch.ger(axis, axis) * i.view(-1, 1, 1)
+
+ return eye - flip
+
+
+def saturation_mat(axis, i):
+ batch = i.shape[0]
+
+ eye = torch.eye(4).unsqueeze(0).repeat(batch, 1, 1)
+ axis = torch.tensor(axis + (0,))
+ axis = torch.ger(axis, axis)
+ saturate = axis + (eye - axis) * i.view(-1, 1, 1)
+
+ return saturate
+
+
+def lognormal_sample(size, mean=0, std=1, device="cpu"):
+ return torch.empty(size, device=device).log_normal_(mean=mean, std=std)
+
+
+def category_sample(size, categories, device="cpu"):
+ category = torch.tensor(categories, device=device)
+ sample = torch.randint(high=len(categories), size=(size,), device=device)
+
+ return category[sample]
+
+
+def uniform_sample(size, low, high, device="cpu"):
+ return torch.empty(size, device=device).uniform_(low, high)
+
+
+def normal_sample(size, mean=0, std=1, device="cpu"):
+ return torch.empty(size, device=device).normal_(mean, std)
+
+
+def bernoulli_sample(size, p, device="cpu"):
+ return torch.empty(size, device=device).bernoulli_(p)
+
+
+def random_mat_apply(p, transform, prev, eye, device="cpu"):
+ size = transform.shape[0]
+ select = bernoulli_sample(size, p, device=device).view(size, 1, 1)
+ select_transform = select * transform + (1 - select) * eye
+
+ return select_transform @ prev
+
+
+def sample_affine(p, size, height, width, device="cpu"):
+ G = torch.eye(3, device=device).unsqueeze(0).repeat(size, 1, 1)
+ eye = G
+
+ # flip
+ #param = category_sample(size, (0, 1))
+ #Gc = scale_mat(1 - 2.0 * param, torch.ones(size), device=device)
+ #G = random_mat_apply(p, Gc, G, eye, device=device)
+ # print('flip', G, scale_mat(1 - 2.0 * param, torch.ones(size)), sep='\n')
+
+ # 90 rotate
+ #param = category_sample(size, (0, 3))
+ #Gc = rotate_mat(-math.pi / 2 * param, device=device)
+ #G = random_mat_apply(p, Gc, G, eye, device=device)
+ # print('90 rotate', G, rotate_mat(-math.pi / 2 * param), sep='\n')
+
+ # integer translate
+ param = uniform_sample(size, -0.125, 0.125)
+ param_height = torch.round(param * height) / height
+ param_width = torch.round(param * width) / width
+ Gc = translate_mat(param_width, param_height, device=device)
+ G = random_mat_apply(p, Gc, G, eye, device=device)
+ # print('integer translate', G, translate_mat(param_width, param_height), sep='\n')
+
+ # isotropic scale
+ param = lognormal_sample(size, std=0.1 * math.log(2))
+ Gc = scale_mat(param, param, device=device)
+ G = random_mat_apply(p, Gc, G, eye, device=device)
+ # print('isotropic scale', G, scale_mat(param, param), sep='\n')
+
+ p_rot = 1 - math.sqrt(1 - p)
+
+ # pre-rotate
+ param = uniform_sample(size, -math.pi * 0.25, math.pi * 0.25)
+ Gc = rotate_mat(-param, device=device)
+ G = random_mat_apply(p_rot, Gc, G, eye, device=device)
+ # print('pre-rotate', G, rotate_mat(-param), sep='\n')
+
+ # anisotropic scale
+ param = lognormal_sample(size, std=0.1 * math.log(2))
+ Gc = scale_mat(param, 1 / param, device=device)
+ G = random_mat_apply(p, Gc, G, eye, device=device)
+ # print('anisotropic scale', G, scale_mat(param, 1 / param), sep='\n')
+
+ # post-rotate
+ param = uniform_sample(size, -math.pi * 0.25, math.pi * 0.25)
+ Gc = rotate_mat(-param, device=device)
+ G = random_mat_apply(p_rot, Gc, G, eye, device=device)
+ # print('post-rotate', G, rotate_mat(-param), sep='\n')
+
+ # fractional translate
+ param = normal_sample(size, std=0.125)
+ Gc = translate_mat(param, param, device=device)
+ G = random_mat_apply(p, Gc, G, eye, device=device)
+ # print('fractional translate', G, translate_mat(param, param), sep='\n')
+
+ return G
+
+
+def sample_color(p, size):
+ C = torch.eye(4).unsqueeze(0).repeat(size, 1, 1)
+ eye = C
+ axis_val = 1 / math.sqrt(3)
+ axis = (axis_val, axis_val, axis_val)
+
+ # brightness
+ param = normal_sample(size, std=0.2)
+ Cc = translate3d_mat(param, param, param)
+ C = random_mat_apply(p, Cc, C, eye)
+
+ # contrast
+ param = lognormal_sample(size, std=0.5 * math.log(2))
+ Cc = scale3d_mat(param, param, param)
+ C = random_mat_apply(p, Cc, C, eye)
+
+ # luma flip
+ param = category_sample(size, (0, 1))
+ Cc = luma_flip_mat(axis, param)
+ C = random_mat_apply(p, Cc, C, eye)
+
+ # hue rotation
+ param = uniform_sample(size, -math.pi, math.pi)
+ Cc = rotate3d_mat(axis, param)
+ C = random_mat_apply(p, Cc, C, eye)
+
+ # saturation
+ param = lognormal_sample(size, std=1 * math.log(2))
+ Cc = saturation_mat(axis, param)
+ C = random_mat_apply(p, Cc, C, eye)
+
+ return C
+
+
+def make_grid(shape, x0, x1, y0, y1, device):
+ n, c, h, w = shape
+ grid = torch.empty(n, h, w, 3, device=device)
+ grid[:, :, :, 0] = torch.linspace(x0, x1, w, device=device)
+ grid[:, :, :, 1] = torch.linspace(y0, y1, h, device=device).unsqueeze(-1)
+ grid[:, :, :, 2] = 1
+
+ return grid
+
+
+def affine_grid(grid, mat):
+ n, h, w, _ = grid.shape
+ return (grid.view(n, h * w, 3) @ mat.transpose(1, 2)).view(n, h, w, 2)
+
+
+def get_padding(G, height, width, kernel_size):
+ device = G.device
+
+ cx = (width - 1) / 2
+ cy = (height - 1) / 2
+ cp = torch.tensor(
+ [(-cx, -cy, 1), (cx, -cy, 1), (cx, cy, 1), (-cx, cy, 1)], device=device
+ )
+ cp = G @ cp.T
+
+ pad_k = kernel_size // 4
+
+ pad = cp[:, :2, :].permute(1, 0, 2).flatten(1)
+ pad = torch.cat((-pad, pad)).max(1).values
+ pad = pad + torch.tensor([pad_k * 2 - cx, pad_k * 2 - cy] * 2, device=device)
+ pad = pad.max(torch.tensor([0, 0] * 2, device=device))
+ pad = pad.min(torch.tensor([width - 1, height - 1] * 2, device=device))
+
+ pad_x1, pad_y1, pad_x2, pad_y2 = pad.ceil().to(torch.int32)
+
+ return pad_x1, pad_x2, pad_y1, pad_y2
+
+
+def try_sample_affine_and_pad(img, p, kernel_size, G=None):
+ batch, _, height, width = img.shape
+
+ G_try = G
+
+ if G is None:
+ G_try = torch.inverse(sample_affine(p, batch, height, width))
+
+ pad_x1, pad_x2, pad_y1, pad_y2 = get_padding(G_try, height, width, kernel_size)
+
+ img_pad = F.pad(img, (pad_x1, pad_x2, pad_y1, pad_y2), mode="reflect")
+
+ return img_pad, G_try, (pad_x1, pad_x2, pad_y1, pad_y2)
+
+
+class GridSampleForward(autograd.Function):
+ @staticmethod
+ def forward(ctx, input, grid):
+ out = F.grid_sample(
+ input, grid, mode="bilinear", padding_mode="zeros", align_corners=False
+ )
+ ctx.save_for_backward(input, grid)
+
+ return out
+
+ @staticmethod
+ def backward(ctx, grad_output):
+ input, grid = ctx.saved_tensors
+ grad_input, grad_grid = GridSampleBackward.apply(grad_output, input, grid)
+
+ return grad_input, grad_grid
+
+
+class GridSampleBackward(autograd.Function):
+ @staticmethod
+ def forward(ctx, grad_output, input, grid):
+ op = torch._C._jit_get_operation("aten::grid_sampler_2d_backward")
+ grad_input, grad_grid = op(grad_output, input, grid, 0, 0, False)
+ ctx.save_for_backward(grid)
+
+ return grad_input, grad_grid
+
+ @staticmethod
+ def backward(ctx, grad_grad_input, grad_grad_grid):
+ grid, = ctx.saved_tensors
+ grad_grad_output = None
+
+ if ctx.needs_input_grad[0]:
+ grad_grad_output = GridSampleForward.apply(grad_grad_input, grid)
+
+ return grad_grad_output, None, None
+
+
+grid_sample = GridSampleForward.apply
+
+
+def scale_mat_single(s_x, s_y):
+ return torch.tensor(((s_x, 0, 0), (0, s_y, 0), (0, 0, 1)), dtype=torch.float32)
+
+
+def translate_mat_single(t_x, t_y):
+ return torch.tensor(((1, 0, t_x), (0, 1, t_y), (0, 0, 1)), dtype=torch.float32)
+
+
+def random_apply_affine(img, p, G=None, antialiasing_kernel=SYM6):
+ kernel = antialiasing_kernel
+ len_k = len(kernel)
+
+ kernel = torch.as_tensor(kernel).to(img)
+ # kernel = torch.ger(kernel, kernel).to(img)
+ kernel_flip = torch.flip(kernel, (0,))
+
+ img_pad, G, (pad_x1, pad_x2, pad_y1, pad_y2) = try_sample_affine_and_pad(
+ img, p, len_k, G
+ )
+
+ G_inv = (
+ translate_mat_single((pad_x1 - pad_x2).item() / 2, (pad_y1 - pad_y2).item() / 2)
+ @ G
+ )
+ up_pad = (
+ (len_k + 2 - 1) // 2,
+ (len_k - 2) // 2,
+ (len_k + 2 - 1) // 2,
+ (len_k - 2) // 2,
+ )
+ img_2x = upfirdn2d(img_pad, kernel.unsqueeze(0), up=(2, 1), pad=(*up_pad[:2], 0, 0))
+ img_2x = upfirdn2d(img_2x, kernel.unsqueeze(1), up=(1, 2), pad=(0, 0, *up_pad[2:]))
+ G_inv = scale_mat_single(2, 2) @ G_inv @ scale_mat_single(1 / 2, 1 / 2)
+ G_inv = translate_mat_single(-0.5, -0.5) @ G_inv @ translate_mat_single(0.5, 0.5)
+ batch_size, channel, height, width = img.shape
+ pad_k = len_k // 4
+ shape = (batch_size, channel, (height + pad_k * 2) * 2, (width + pad_k * 2) * 2)
+ G_inv = (
+ scale_mat_single(2 / img_2x.shape[3], 2 / img_2x.shape[2])
+ @ G_inv
+ @ scale_mat_single(1 / (2 / shape[3]), 1 / (2 / shape[2]))
+ )
+ grid = F.affine_grid(G_inv[:, :2, :].to(img_2x), shape, align_corners=False)
+ img_affine = grid_sample(img_2x, grid)
+ d_p = -pad_k * 2
+ down_pad = (
+ d_p + (len_k - 2 + 1) // 2,
+ d_p + (len_k - 2) // 2,
+ d_p + (len_k - 2 + 1) // 2,
+ d_p + (len_k - 2) // 2,
+ )
+ img_down = upfirdn2d(
+ img_affine, kernel_flip.unsqueeze(0), down=(2, 1), pad=(*down_pad[:2], 0, 0)
+ )
+ img_down = upfirdn2d(
+ img_down, kernel_flip.unsqueeze(1), down=(1, 2), pad=(0, 0, *down_pad[2:])
+ )
+
+ return img_down, G
+
+
+def apply_color(img, mat):
+ batch = img.shape[0]
+ img = img.permute(0, 2, 3, 1)
+ mat_mul = mat[:, :3, :3].transpose(1, 2).view(batch, 1, 3, 3)
+ mat_add = mat[:, :3, 3].view(batch, 1, 1, 3)
+ img = img @ mat_mul + mat_add
+ img = img.permute(0, 3, 1, 2)
+
+ return img
+
+
+def random_apply_color(img, p, C=None):
+ if C is None:
+ C = sample_color(p, img.shape[0])
+
+ img = apply_color(img, C.to(img))
+
+ return img, C
+
+
+def augment(img, p, transform_matrix=(None, None)):
+ img, G = random_apply_affine(img, p, transform_matrix[0])
+ img, C = random_apply_color(img, p, transform_matrix[1])
+
+ return img, (G, C)
diff --git a/requirements.txt b/requirements.txt
new file mode 100644
index 0000000000000000000000000000000000000000..2b6d120ed740de70f917c842aeb0c49c660970ec
--- /dev/null
+++ b/requirements.txt
@@ -0,0 +1,9 @@
+torch==1.7.1
+torchvision
+dlib
+numpy
+opencv-contrib-python
+Pillow
+scipy
+scikit-image
+ipython
\ No newline at end of file
diff --git a/scripts/align_all_parallel.py b/scripts/align_all_parallel.py
new file mode 100644
index 0000000000000000000000000000000000000000..85d23ca8142b29e97421d92b8e9ddadec04d15de
--- /dev/null
+++ b/scripts/align_all_parallel.py
@@ -0,0 +1,215 @@
+"""
+brief: face alignment with FFHQ method (https://github.com/NVlabs/ffhq-dataset)
+author: lzhbrian (https://lzhbrian.me)
+date: 2020.1.5
+note: code is heavily borrowed from
+ https://github.com/NVlabs/ffhq-dataset
+ http://dlib.net/face_landmark_detection.py.html
+
+requirements:
+ apt install cmake
+ conda install Pillow numpy scipy
+ pip install dlib
+ # download face landmark model from:
+ # http://dlib.net/files/shape_predictor_68_face_landmarks.dat.bz2
+"""
+from argparse import ArgumentParser
+import time
+import numpy as np
+import PIL
+import PIL.Image
+import os
+import scipy
+import scipy.ndimage
+import dlib
+import multiprocessing as mp
+import math
+
+from configs.paths_config import model_paths
+SHAPE_PREDICTOR_PATH = model_paths["shape_predictor"]
+
+
+def get_landmark(filepath, predictor):
+ """get landmark with dlib
+ :return: np.array shape=(68, 2)
+ """
+ detector = dlib.get_frontal_face_detector()
+ if type(filepath) == str:
+ img = dlib.load_rgb_image(filepath)
+ else:
+ img = filepath
+ dets = detector(img, 1)
+
+ if len(dets) == 0:
+ print('Error: no face detected! If you are sure there are faces in your input, you may rerun the code or change the image several times until the face is detected. Sometimes the detector is unstable.')
+ return None
+
+ shape = None
+ for k, d in enumerate(dets):
+ shape = predictor(img, d)
+
+ t = list(shape.parts())
+ a = []
+ for tt in t:
+ a.append([tt.x, tt.y])
+ lm = np.array(a)
+ return lm
+
+
+def align_face(filepath, predictor):
+ """
+ :param filepath: str
+ :return: PIL Image
+ """
+
+ lm = get_landmark(filepath, predictor)
+ if lm is None:
+ return None
+
+ lm_chin = lm[0: 17] # left-right
+ lm_eyebrow_left = lm[17: 22] # left-right
+ lm_eyebrow_right = lm[22: 27] # left-right
+ lm_nose = lm[27: 31] # top-down
+ lm_nostrils = lm[31: 36] # top-down
+ lm_eye_left = lm[36: 42] # left-clockwise
+ lm_eye_right = lm[42: 48] # left-clockwise
+ lm_mouth_outer = lm[48: 60] # left-clockwise
+ lm_mouth_inner = lm[60: 68] # left-clockwise
+
+ # Calculate auxiliary vectors.
+ eye_left = np.mean(lm_eye_left, axis=0)
+ eye_right = np.mean(lm_eye_right, axis=0)
+ eye_avg = (eye_left + eye_right) * 0.5
+ eye_to_eye = eye_right - eye_left
+ mouth_left = lm_mouth_outer[0]
+ mouth_right = lm_mouth_outer[6]
+ mouth_avg = (mouth_left + mouth_right) * 0.5
+ eye_to_mouth = mouth_avg - eye_avg
+
+ # Choose oriented crop rectangle.
+ x = eye_to_eye - np.flipud(eye_to_mouth) * [-1, 1]
+ x /= np.hypot(*x)
+ x *= max(np.hypot(*eye_to_eye) * 2.0, np.hypot(*eye_to_mouth) * 1.8)
+ y = np.flipud(x) * [-1, 1]
+ c = eye_avg + eye_to_mouth * 0.1
+ quad = np.stack([c - x - y, c - x + y, c + x + y, c + x - y])
+ qsize = np.hypot(*x) * 2
+
+ # read image
+ if type(filepath) == str:
+ img = PIL.Image.open(filepath)
+ else:
+ img = PIL.Image.fromarray(filepath)
+
+ output_size = 256
+ transform_size = 256
+ enable_padding = True
+
+ # Shrink.
+ shrink = int(np.floor(qsize / output_size * 0.5))
+ if shrink > 1:
+ rsize = (int(np.rint(float(img.size[0]) / shrink)), int(np.rint(float(img.size[1]) / shrink)))
+ img = img.resize(rsize, PIL.Image.ANTIALIAS)
+ quad /= shrink
+ qsize /= shrink
+
+ # Crop.
+ border = max(int(np.rint(qsize * 0.1)), 3)
+ crop = (int(np.floor(min(quad[:, 0]))), int(np.floor(min(quad[:, 1]))), int(np.ceil(max(quad[:, 0]))),
+ int(np.ceil(max(quad[:, 1]))))
+ crop = (max(crop[0] - border, 0), max(crop[1] - border, 0), min(crop[2] + border, img.size[0]),
+ min(crop[3] + border, img.size[1]))
+ if crop[2] - crop[0] < img.size[0] or crop[3] - crop[1] < img.size[1]:
+ img = img.crop(crop)
+ quad -= crop[0:2]
+
+ # Pad.
+ pad = (int(np.floor(min(quad[:, 0]))), int(np.floor(min(quad[:, 1]))), int(np.ceil(max(quad[:, 0]))),
+ int(np.ceil(max(quad[:, 1]))))
+ pad = (max(-pad[0] + border, 0), max(-pad[1] + border, 0), max(pad[2] - img.size[0] + border, 0),
+ max(pad[3] - img.size[1] + border, 0))
+ if enable_padding and max(pad) > border - 4:
+ pad = np.maximum(pad, int(np.rint(qsize * 0.3)))
+ img = np.pad(np.float32(img), ((pad[1], pad[3]), (pad[0], pad[2]), (0, 0)), 'reflect')
+ h, w, _ = img.shape
+ y, x, _ = np.ogrid[:h, :w, :1]
+ mask = np.maximum(1.0 - np.minimum(np.float32(x) / pad[0], np.float32(w - 1 - x) / pad[2]),
+ 1.0 - np.minimum(np.float32(y) / pad[1], np.float32(h - 1 - y) / pad[3]))
+ blur = qsize * 0.02
+ img += (scipy.ndimage.gaussian_filter(img, [blur, blur, 0]) - img) * np.clip(mask * 3.0 + 1.0, 0.0, 1.0)
+ img += (np.median(img, axis=(0, 1)) - img) * np.clip(mask, 0.0, 1.0)
+ img = PIL.Image.fromarray(np.uint8(np.clip(np.rint(img), 0, 255)), 'RGB')
+ quad += pad[:2]
+
+ # Transform.
+ img = img.transform((transform_size, transform_size), PIL.Image.QUAD, (quad + 0.5).flatten(), PIL.Image.BILINEAR)
+ if output_size < transform_size:
+ img = img.resize((output_size, output_size), PIL.Image.ANTIALIAS)
+
+ # Save aligned image.
+ return img
+
+
+def chunks(lst, n):
+ """Yield successive n-sized chunks from lst."""
+ for i in range(0, len(lst), n):
+ yield lst[i:i + n]
+
+
+def extract_on_paths(file_paths):
+ predictor = dlib.shape_predictor(SHAPE_PREDICTOR_PATH)
+ pid = mp.current_process().name
+ print('\t{} is starting to extract on #{} images'.format(pid, len(file_paths)))
+ tot_count = len(file_paths)
+ count = 0
+ for file_path, res_path in file_paths:
+ count += 1
+ if count % 100 == 0:
+ print('{} done with {}/{}'.format(pid, count, tot_count))
+ try:
+ res = align_face(file_path, predictor)
+ res = res.convert('RGB')
+ os.makedirs(os.path.dirname(res_path), exist_ok=True)
+ res.save(res_path)
+ except Exception:
+ continue
+ print('\tDone!')
+
+
+def parse_args():
+ parser = ArgumentParser(add_help=False)
+ parser.add_argument('--num_threads', type=int, default=1)
+ parser.add_argument('--root_path', type=str, default='')
+ args = parser.parse_args()
+ return args
+
+
+def run(args):
+ root_path = args.root_path
+ out_crops_path = root_path + '_crops'
+ if not os.path.exists(out_crops_path):
+ os.makedirs(out_crops_path, exist_ok=True)
+
+ file_paths = []
+ for root, dirs, files in os.walk(root_path):
+ for file in files:
+ file_path = os.path.join(root, file)
+ fname = os.path.join(out_crops_path, os.path.relpath(file_path, root_path))
+ res_path = '{}.jpg'.format(os.path.splitext(fname)[0])
+ if os.path.splitext(file_path)[1] == '.txt' or os.path.exists(res_path):
+ continue
+ file_paths.append((file_path, res_path))
+
+ file_chunks = list(chunks(file_paths, int(math.ceil(len(file_paths) / args.num_threads))))
+ print(len(file_chunks))
+ pool = mp.Pool(args.num_threads)
+ print('Running on {} paths\nHere we goooo'.format(len(file_paths)))
+ tic = time.time()
+ pool.map(extract_on_paths, file_chunks)
+ toc = time.time()
+ print('Mischief managed in {}s'.format(toc - tic))
+
+
+if __name__ == '__main__':
+ args = parse_args()
+ run(args)
diff --git a/scripts/calc_id_loss_parallel.py b/scripts/calc_id_loss_parallel.py
new file mode 100644
index 0000000000000000000000000000000000000000..efc82bf851b252e92c45be3c87be877616f44ead
--- /dev/null
+++ b/scripts/calc_id_loss_parallel.py
@@ -0,0 +1,119 @@
+from argparse import ArgumentParser
+import time
+import numpy as np
+import os
+import json
+import sys
+from PIL import Image
+import multiprocessing as mp
+import math
+import torch
+import torchvision.transforms as trans
+
+sys.path.append(".")
+sys.path.append("..")
+
+from models.mtcnn.mtcnn import MTCNN
+from models.encoders.model_irse import IR_101
+from configs.paths_config import model_paths
+CIRCULAR_FACE_PATH = model_paths['circular_face']
+
+
+def chunks(lst, n):
+ """Yield successive n-sized chunks from lst."""
+ for i in range(0, len(lst), n):
+ yield lst[i:i + n]
+
+
+def extract_on_paths(file_paths):
+ facenet = IR_101(input_size=112)
+ facenet.load_state_dict(torch.load(CIRCULAR_FACE_PATH))
+ facenet.cuda()
+ facenet.eval()
+ mtcnn = MTCNN()
+ id_transform = trans.Compose([
+ trans.ToTensor(),
+ trans.Normalize([0.5, 0.5, 0.5], [0.5, 0.5, 0.5])
+ ])
+
+ pid = mp.current_process().name
+ print('\t{} is starting to extract on {} images'.format(pid, len(file_paths)))
+ tot_count = len(file_paths)
+ count = 0
+
+ scores_dict = {}
+ for res_path, gt_path in file_paths:
+ count += 1
+ if count % 100 == 0:
+ print('{} done with {}/{}'.format(pid, count, tot_count))
+ if True:
+ input_im = Image.open(res_path)
+ input_im, _ = mtcnn.align(input_im)
+ if input_im is None:
+ print('{} skipping {}'.format(pid, res_path))
+ continue
+
+ input_id = facenet(id_transform(input_im).unsqueeze(0).cuda())[0]
+
+ result_im = Image.open(gt_path)
+ result_im, _ = mtcnn.align(result_im)
+ if result_im is None:
+ print('{} skipping {}'.format(pid, gt_path))
+ continue
+
+ result_id = facenet(id_transform(result_im).unsqueeze(0).cuda())[0]
+ score = float(input_id.dot(result_id))
+ scores_dict[os.path.basename(gt_path)] = score
+
+ return scores_dict
+
+
+def parse_args():
+ parser = ArgumentParser(add_help=False)
+ parser.add_argument('--num_threads', type=int, default=4)
+ parser.add_argument('--data_path', type=str, default='results')
+ parser.add_argument('--gt_path', type=str, default='gt_images')
+ args = parser.parse_args()
+ return args
+
+
+def run(args):
+ file_paths = []
+ for f in os.listdir(args.data_path):
+ image_path = os.path.join(args.data_path, f)
+ gt_path = os.path.join(args.gt_path, f)
+ if f.endswith(".jpg") or f.endswith('.png'):
+ file_paths.append([image_path, gt_path.replace('.png','.jpg')])
+
+ file_chunks = list(chunks(file_paths, int(math.ceil(len(file_paths) / args.num_threads))))
+ pool = mp.Pool(args.num_threads)
+ print('Running on {} paths\nHere we goooo'.format(len(file_paths)))
+
+ tic = time.time()
+ results = pool.map(extract_on_paths, file_chunks)
+ scores_dict = {}
+ for d in results:
+ scores_dict.update(d)
+
+ all_scores = list(scores_dict.values())
+ mean = np.mean(all_scores)
+ std = np.std(all_scores)
+ result_str = 'New Average score is {:.2f}+-{:.2f}'.format(mean, std)
+ print(result_str)
+
+ out_path = os.path.join(os.path.dirname(args.data_path), 'inference_metrics')
+ if not os.path.exists(out_path):
+ os.makedirs(out_path)
+
+ with open(os.path.join(out_path, 'stat_id.txt'), 'w') as f:
+ f.write(result_str)
+ with open(os.path.join(out_path, 'scores_id.json'), 'w') as f:
+ json.dump(scores_dict, f)
+
+ toc = time.time()
+ print('Mischief managed in {}s'.format(toc - tic))
+
+
+if __name__ == '__main__':
+ args = parse_args()
+ run(args)
diff --git a/scripts/calc_losses_on_images.py b/scripts/calc_losses_on_images.py
new file mode 100644
index 0000000000000000000000000000000000000000..436348db28a625d94f63bbb86ff779b92d28b419
--- /dev/null
+++ b/scripts/calc_losses_on_images.py
@@ -0,0 +1,84 @@
+from argparse import ArgumentParser
+import os
+import json
+import sys
+from tqdm import tqdm
+import numpy as np
+import torch
+from torch.utils.data import DataLoader
+import torchvision.transforms as transforms
+
+sys.path.append(".")
+sys.path.append("..")
+
+from criteria.lpips.lpips import LPIPS
+from datasets.gt_res_dataset import GTResDataset
+
+
+def parse_args():
+ parser = ArgumentParser(add_help=False)
+ parser.add_argument('--mode', type=str, default='lpips', choices=['lpips', 'l2'])
+ parser.add_argument('--data_path', type=str, default='results')
+ parser.add_argument('--gt_path', type=str, default='gt_images')
+ parser.add_argument('--workers', type=int, default=4)
+ parser.add_argument('--batch_size', type=int, default=4)
+ args = parser.parse_args()
+ return args
+
+
+def run(args):
+
+ transform = transforms.Compose([transforms.Resize((256, 256)),
+ transforms.ToTensor(),
+ transforms.Normalize([0.5, 0.5, 0.5], [0.5, 0.5, 0.5])])
+
+ print('Loading dataset')
+ dataset = GTResDataset(root_path=args.data_path,
+ gt_dir=args.gt_path,
+ transform=transform)
+
+ dataloader = DataLoader(dataset,
+ batch_size=args.batch_size,
+ shuffle=False,
+ num_workers=int(args.workers),
+ drop_last=True)
+
+ if args.mode == 'lpips':
+ loss_func = LPIPS(net_type='alex')
+ elif args.mode == 'l2':
+ loss_func = torch.nn.MSELoss()
+ else:
+ raise Exception('Not a valid mode!')
+ loss_func.cuda()
+
+ global_i = 0
+ scores_dict = {}
+ all_scores = []
+ for result_batch, gt_batch in tqdm(dataloader):
+ for i in range(args.batch_size):
+ loss = float(loss_func(result_batch[i:i+1].cuda(), gt_batch[i:i+1].cuda()))
+ all_scores.append(loss)
+ im_path = dataset.pairs[global_i][0]
+ scores_dict[os.path.basename(im_path)] = loss
+ global_i += 1
+
+ all_scores = list(scores_dict.values())
+ mean = np.mean(all_scores)
+ std = np.std(all_scores)
+ result_str = 'Average loss is {:.2f}+-{:.2f}'.format(mean, std)
+ print('Finished with ', args.data_path)
+ print(result_str)
+
+ out_path = os.path.join(os.path.dirname(args.data_path), 'inference_metrics')
+ if not os.path.exists(out_path):
+ os.makedirs(out_path)
+
+ with open(os.path.join(out_path, 'stat_{}.txt'.format(args.mode)), 'w') as f:
+ f.write(result_str)
+ with open(os.path.join(out_path, 'scores_{}.json'.format(args.mode)), 'w') as f:
+ json.dump(scores_dict, f)
+
+
+if __name__ == '__main__':
+ args = parse_args()
+ run(args)
diff --git a/scripts/generate_sketch_data.py b/scripts/generate_sketch_data.py
new file mode 100644
index 0000000000000000000000000000000000000000..a13acf949bf2efb3449f13922b7489e5c06880a3
--- /dev/null
+++ b/scripts/generate_sketch_data.py
@@ -0,0 +1,62 @@
+from torchvision import transforms
+from torchvision.utils import save_image
+from torch.utils.serialization import load_lua
+import os
+import cv2
+import numpy as np
+
+"""
+NOTE!: Must have torch==0.4.1 and torchvision==0.2.1
+The sketch simplification model (sketch_gan.t7) from Simo Serra et al. can be downloaded from their official implementation:
+ https://github.com/bobbens/sketch_simplification
+"""
+
+
+def sobel(img):
+ opImgx = cv2.Sobel(img, cv2.CV_8U, 0, 1, ksize=3)
+ opImgy = cv2.Sobel(img, cv2.CV_8U, 1, 0, ksize=3)
+ return cv2.bitwise_or(opImgx, opImgy)
+
+
+def sketch(frame):
+ frame = cv2.GaussianBlur(frame, (3, 3), 0)
+ invImg = 255 - frame
+ edgImg0 = sobel(frame)
+ edgImg1 = sobel(invImg)
+ edgImg = cv2.addWeighted(edgImg0, 0.75, edgImg1, 0.75, 0)
+ opImg = 255 - edgImg
+ return opImg
+
+
+def get_sketch_image(image_path):
+ original = cv2.imread(image_path)
+ original = cv2.cvtColor(original, cv2.COLOR_BGR2GRAY)
+ sketch_image = sketch(original)
+ return sketch_image[:, :, np.newaxis]
+
+
+use_cuda = True
+
+cache = load_lua("/path/to/sketch_gan.t7")
+model = cache.model
+immean = cache.mean
+imstd = cache.std
+model.evaluate()
+
+data_path = "/path/to/data/imgs"
+images = [os.path.join(data_path, f) for f in os.listdir(data_path)]
+
+output_dir = "/path/to/data/edges"
+if not os.path.exists(output_dir):
+ os.makedirs(output_dir)
+
+for idx, image_path in enumerate(images):
+ if idx % 50 == 0:
+ print("{} out of {}".format(idx, len(images)))
+ data = get_sketch_image(image_path)
+ data = ((transforms.ToTensor()(data) - immean) / imstd).unsqueeze(0)
+ if use_cuda:
+ pred = model.cuda().forward(data.cuda()).float()
+ else:
+ pred = model.forward(data)
+ save_image(pred[0], os.path.join(output_dir, "{}_edges.jpg".format(image_path.split("/")[-1].split('.')[0])))
diff --git a/scripts/inference.py b/scripts/inference.py
new file mode 100644
index 0000000000000000000000000000000000000000..9250d4b5b05d8a31527603d42823fd8b10234ce9
--- /dev/null
+++ b/scripts/inference.py
@@ -0,0 +1,136 @@
+import os
+from argparse import Namespace
+
+from tqdm import tqdm
+import time
+import numpy as np
+import torch
+from PIL import Image
+from torch.utils.data import DataLoader
+import sys
+
+sys.path.append(".")
+sys.path.append("..")
+
+from configs import data_configs
+from datasets.inference_dataset import InferenceDataset
+from utils.common import tensor2im, log_input_image
+from options.test_options import TestOptions
+from models.psp import pSp
+
+
+def run():
+ test_opts = TestOptions().parse()
+
+ if test_opts.resize_factors is not None:
+ assert len(
+ test_opts.resize_factors.split(',')) == 1, "When running inference, provide a single downsampling factor!"
+ out_path_results = os.path.join(test_opts.exp_dir, 'inference_results',
+ 'downsampling_{}'.format(test_opts.resize_factors))
+ out_path_coupled = os.path.join(test_opts.exp_dir, 'inference_coupled',
+ 'downsampling_{}'.format(test_opts.resize_factors))
+ else:
+ out_path_results = os.path.join(test_opts.exp_dir, 'inference_results')
+ out_path_coupled = os.path.join(test_opts.exp_dir, 'inference_coupled')
+
+ os.makedirs(out_path_results, exist_ok=True)
+ os.makedirs(out_path_coupled, exist_ok=True)
+
+ # update test options with options used during training
+ ckpt = torch.load(test_opts.checkpoint_path, map_location='cpu')
+ opts = ckpt['opts']
+ opts.update(vars(test_opts))
+ if 'learn_in_w' not in opts:
+ opts['learn_in_w'] = False
+ if 'output_size' not in opts:
+ opts['output_size'] = 1024
+ opts = Namespace(**opts)
+
+ net = pSp(opts)
+ net.eval()
+ net.cuda()
+
+ print('Loading dataset for {}'.format(opts.dataset_type))
+ dataset_args = data_configs.DATASETS[opts.dataset_type]
+ transforms_dict = dataset_args['transforms'](opts).get_transforms()
+ dataset = InferenceDataset(root=opts.data_path,
+ transform=transforms_dict['transform_inference'],
+ opts=opts)
+ dataloader = DataLoader(dataset,
+ batch_size=opts.test_batch_size,
+ shuffle=False,
+ num_workers=int(opts.test_workers),
+ drop_last=True)
+
+ if opts.n_images is None:
+ opts.n_images = len(dataset)
+
+ global_i = 0
+ global_time = []
+ for input_batch in tqdm(dataloader):
+ if global_i >= opts.n_images:
+ break
+ with torch.no_grad():
+ input_cuda = input_batch.cuda().float()
+ tic = time.time()
+ result_batch = run_on_batch(input_cuda, net, opts)
+ toc = time.time()
+ global_time.append(toc - tic)
+
+ for i in range(opts.test_batch_size):
+ result = tensor2im(result_batch[i])
+ im_path = dataset.paths[global_i]
+
+ if opts.couple_outputs or global_i % 100 == 0:
+ input_im = log_input_image(input_batch[i], opts)
+ resize_amount = (256, 256) if opts.resize_outputs else (opts.output_size, opts.output_size)
+ if opts.resize_factors is not None:
+ # for super resolution, save the original, down-sampled, and output
+ source = Image.open(im_path)
+ res = np.concatenate([np.array(source.resize(resize_amount)),
+ np.array(input_im.resize(resize_amount, resample=Image.NEAREST)),
+ np.array(result.resize(resize_amount))], axis=1)
+ else:
+ # otherwise, save the original and output
+ res = np.concatenate([np.array(input_im.resize(resize_amount)),
+ np.array(result.resize(resize_amount))], axis=1)
+ Image.fromarray(res).save(os.path.join(out_path_coupled, os.path.basename(im_path)))
+
+ im_save_path = os.path.join(out_path_results, os.path.basename(im_path))
+ Image.fromarray(np.array(result)).save(im_save_path)
+
+ global_i += 1
+
+ stats_path = os.path.join(opts.exp_dir, 'stats.txt')
+ result_str = 'Runtime {:.4f}+-{:.4f}'.format(np.mean(global_time), np.std(global_time))
+ print(result_str)
+
+ with open(stats_path, 'w') as f:
+ f.write(result_str)
+
+
+def run_on_batch(inputs, net, opts):
+ if opts.latent_mask is None:
+ result_batch = net(inputs, randomize_noise=False, resize=opts.resize_outputs)
+ else:
+ latent_mask = [int(l) for l in opts.latent_mask.split(",")]
+ result_batch = []
+ for image_idx, input_image in enumerate(inputs):
+ # get latent vector to inject into our input image
+ vec_to_inject = np.random.randn(1, 512).astype('float32')
+ _, latent_to_inject = net(torch.from_numpy(vec_to_inject).to("cuda"),
+ input_code=True,
+ return_latents=True)
+ # get output image with injected style vector
+ res = net(input_image.unsqueeze(0).to("cuda").float(),
+ latent_mask=latent_mask,
+ inject_latent=latent_to_inject,
+ alpha=opts.mix_alpha,
+ resize=opts.resize_outputs)
+ result_batch.append(res)
+ result_batch = torch.cat(result_batch, dim=0)
+ return result_batch
+
+
+if __name__ == '__main__':
+ run()
diff --git a/scripts/style_mixing.py b/scripts/style_mixing.py
new file mode 100644
index 0000000000000000000000000000000000000000..e252b418adb26ac5dc9e30998d44279c2ff60cb7
--- /dev/null
+++ b/scripts/style_mixing.py
@@ -0,0 +1,101 @@
+import os
+from argparse import Namespace
+
+from tqdm import tqdm
+import numpy as np
+from PIL import Image
+import torch
+from torch.utils.data import DataLoader
+import sys
+
+sys.path.append(".")
+sys.path.append("..")
+
+from configs import data_configs
+from datasets.inference_dataset import InferenceDataset
+from utils.common import tensor2im, log_input_image
+from options.test_options import TestOptions
+from models.psp import pSp
+
+
+def run():
+ test_opts = TestOptions().parse()
+
+ if test_opts.resize_factors is not None:
+ factors = test_opts.resize_factors.split(',')
+ assert len(factors) == 1, "When running inference, please provide a single downsampling factor!"
+ mixed_path_results = os.path.join(test_opts.exp_dir, 'style_mixing',
+ 'downsampling_{}'.format(test_opts.resize_factors))
+ else:
+ mixed_path_results = os.path.join(test_opts.exp_dir, 'style_mixing')
+ os.makedirs(mixed_path_results, exist_ok=True)
+
+ # update test options with options used during training
+ ckpt = torch.load(test_opts.checkpoint_path, map_location='cpu')
+ opts = ckpt['opts']
+ opts.update(vars(test_opts))
+ if 'learn_in_w' not in opts:
+ opts['learn_in_w'] = False
+ if 'output_size' not in opts:
+ opts['output_size'] = 1024
+ opts = Namespace(**opts)
+
+ net = pSp(opts)
+ net.eval()
+ net.cuda()
+
+ print('Loading dataset for {}'.format(opts.dataset_type))
+ dataset_args = data_configs.DATASETS[opts.dataset_type]
+ transforms_dict = dataset_args['transforms'](opts).get_transforms()
+ dataset = InferenceDataset(root=opts.data_path,
+ transform=transforms_dict['transform_inference'],
+ opts=opts)
+ dataloader = DataLoader(dataset,
+ batch_size=opts.test_batch_size,
+ shuffle=False,
+ num_workers=int(opts.test_workers),
+ drop_last=True)
+
+ latent_mask = [int(l) for l in opts.latent_mask.split(",")]
+ if opts.n_images is None:
+ opts.n_images = len(dataset)
+
+ global_i = 0
+ for input_batch in tqdm(dataloader):
+ if global_i >= opts.n_images:
+ break
+ with torch.no_grad():
+ input_batch = input_batch.cuda()
+ for image_idx, input_image in enumerate(input_batch):
+ # generate random vectors to inject into input image
+ vecs_to_inject = np.random.randn(opts.n_outputs_to_generate, 512).astype('float32')
+ multi_modal_outputs = []
+ for vec_to_inject in vecs_to_inject:
+ cur_vec = torch.from_numpy(vec_to_inject).unsqueeze(0).to("cuda")
+ # get latent vector to inject into our input image
+ _, latent_to_inject = net(cur_vec,
+ input_code=True,
+ return_latents=True)
+ # get output image with injected style vector
+ res = net(input_image.unsqueeze(0).to("cuda").float(),
+ latent_mask=latent_mask,
+ inject_latent=latent_to_inject,
+ alpha=opts.mix_alpha,
+ resize=opts.resize_outputs)
+ multi_modal_outputs.append(res[0])
+
+ # visualize multi modal outputs
+ input_im_path = dataset.paths[global_i]
+ image = input_batch[image_idx]
+ input_image = log_input_image(image, opts)
+ resize_amount = (256, 256) if opts.resize_outputs else (opts.output_size, opts.output_size)
+ res = np.array(input_image.resize(resize_amount))
+ for output in multi_modal_outputs:
+ output = tensor2im(output)
+ res = np.concatenate([res, np.array(output.resize(resize_amount))], axis=1)
+ Image.fromarray(res).save(os.path.join(mixed_path_results, os.path.basename(input_im_path)))
+ global_i += 1
+
+
+if __name__ == '__main__':
+ run()
diff --git a/scripts/train.py b/scripts/train.py
new file mode 100644
index 0000000000000000000000000000000000000000..21026ebf1619cf19dda8fb5a05909b22f0f0fcbc
--- /dev/null
+++ b/scripts/train.py
@@ -0,0 +1,32 @@
+"""
+This file runs the main training/val loop
+"""
+import os
+import json
+import sys
+import pprint
+
+sys.path.append(".")
+sys.path.append("..")
+
+from options.train_options import TrainOptions
+from training.coach import Coach
+
+
+def main():
+ opts = TrainOptions().parse()
+ if os.path.exists(opts.exp_dir):
+ raise Exception('Oops... {} already exists'.format(opts.exp_dir))
+ os.makedirs(opts.exp_dir)
+
+ opts_dict = vars(opts)
+ pprint.pprint(opts_dict)
+ with open(os.path.join(opts.exp_dir, 'opt.json'), 'w') as f:
+ json.dump(opts_dict, f, indent=4, sort_keys=True)
+
+ coach = Coach(opts)
+ coach.train()
+
+
+if __name__ == '__main__':
+ main()
diff --git a/utils/__init__.py b/utils/__init__.py
new file mode 100644
index 0000000000000000000000000000000000000000..e69de29bb2d1d6434b8b29ae775ad8c2e48c5391
diff --git a/utils/common.py b/utils/common.py
new file mode 100644
index 0000000000000000000000000000000000000000..4813fe311ee40720697e4862c5fbfad811d39237
--- /dev/null
+++ b/utils/common.py
@@ -0,0 +1,87 @@
+import cv2
+import numpy as np
+from PIL import Image
+import matplotlib.pyplot as plt
+
+
+# Log images
+def log_input_image(x, opts):
+ if opts.label_nc == 0:
+ return tensor2im(x)
+ elif opts.label_nc == 1:
+ return tensor2sketch(x)
+ else:
+ return tensor2map(x)
+
+
+def tensor2im(var):
+ var = var.cpu().detach().transpose(0, 2).transpose(0, 1).numpy()
+ var = ((var + 1) / 2)
+ var[var < 0] = 0
+ var[var > 1] = 1
+ var = var * 255
+ return Image.fromarray(var.astype('uint8'))
+
+
+def tensor2map(var):
+ mask = np.argmax(var.data.cpu().numpy(), axis=0)
+ colors = get_colors()
+ mask_image = np.ones(shape=(mask.shape[0], mask.shape[1], 3))
+ for class_idx in np.unique(mask):
+ mask_image[mask == class_idx] = colors[class_idx]
+ mask_image = mask_image.astype('uint8')
+ return Image.fromarray(mask_image)
+
+
+def tensor2sketch(var):
+ im = var[0].cpu().detach().numpy()
+ im = cv2.cvtColor(im, cv2.COLOR_GRAY2BGR)
+ im = (im * 255).astype(np.uint8)
+ return Image.fromarray(im)
+
+
+# Visualization utils
+def get_colors():
+ # currently support up to 19 classes (for the celebs-hq-mask dataset)
+ colors = [[0, 0, 0], [204, 0, 0], [76, 153, 0], [204, 204, 0], [51, 51, 255], [204, 0, 204], [0, 255, 255],
+ [255, 204, 204], [102, 51, 0], [255, 0, 0], [102, 204, 0], [255, 255, 0], [0, 0, 153], [0, 0, 204],
+ [255, 51, 153], [0, 204, 204], [0, 51, 0], [255, 153, 51], [0, 204, 0]]
+ return colors
+
+
+def vis_faces(log_hooks):
+ display_count = len(log_hooks)
+ fig = plt.figure(figsize=(8, 4 * display_count))
+ gs = fig.add_gridspec(display_count, 3)
+ for i in range(display_count):
+ hooks_dict = log_hooks[i]
+ fig.add_subplot(gs[i, 0])
+ if 'diff_input' in hooks_dict:
+ vis_faces_with_id(hooks_dict, fig, gs, i)
+ else:
+ vis_faces_no_id(hooks_dict, fig, gs, i)
+ plt.tight_layout()
+ return fig
+
+
+def vis_faces_with_id(hooks_dict, fig, gs, i):
+ plt.imshow(hooks_dict['input_face'])
+ plt.title('Input\nOut Sim={:.2f}'.format(float(hooks_dict['diff_input'])))
+ fig.add_subplot(gs[i, 1])
+ plt.imshow(hooks_dict['target_face'])
+ plt.title('Target\nIn={:.2f}, Out={:.2f}'.format(float(hooks_dict['diff_views']),
+ float(hooks_dict['diff_target'])))
+ fig.add_subplot(gs[i, 2])
+ plt.imshow(hooks_dict['output_face'])
+ plt.title('Output\n Target Sim={:.2f}'.format(float(hooks_dict['diff_target'])))
+
+
+def vis_faces_no_id(hooks_dict, fig, gs, i):
+ plt.imshow(hooks_dict['input_face'], cmap="gray")
+ plt.title('Input')
+ fig.add_subplot(gs[i, 1])
+ plt.imshow(hooks_dict['target_face'])
+ plt.title('Target')
+ fig.add_subplot(gs[i, 2])
+ plt.imshow(hooks_dict['output_face'])
+ plt.title('Output')
diff --git a/utils/data_utils.py b/utils/data_utils.py
new file mode 100644
index 0000000000000000000000000000000000000000..f1ba79f4a2d5cc2b97dce76d87bf6e7cdebbc257
--- /dev/null
+++ b/utils/data_utils.py
@@ -0,0 +1,25 @@
+"""
+Code adopted from pix2pixHD:
+https://github.com/NVIDIA/pix2pixHD/blob/master/data/image_folder.py
+"""
+import os
+
+IMG_EXTENSIONS = [
+ '.jpg', '.JPG', '.jpeg', '.JPEG',
+ '.png', '.PNG', '.ppm', '.PPM', '.bmp', '.BMP', '.tiff'
+]
+
+
+def is_image_file(filename):
+ return any(filename.endswith(extension) for extension in IMG_EXTENSIONS)
+
+
+def make_dataset(dir):
+ images = []
+ assert os.path.isdir(dir), '%s is not a valid directory' % dir
+ for root, _, fnames in sorted(os.walk(dir)):
+ for fname in fnames:
+ if is_image_file(fname):
+ path = os.path.join(root, fname)
+ images.append(path)
+ return images
diff --git a/utils/inference_utils.py b/utils/inference_utils.py
new file mode 100644
index 0000000000000000000000000000000000000000..4e993cac404d3e0d6749cad54005179a7b375a10
--- /dev/null
+++ b/utils/inference_utils.py
@@ -0,0 +1,182 @@
+import numpy as np
+import matplotlib.pyplot as plt
+from PIL import Image
+import cv2
+import random
+import math
+import argparse
+import torch
+from torch.utils import data
+from torch.nn import functional as F
+from torch import autograd
+from torch.nn import init
+import torchvision.transforms as transforms
+from scripts.align_all_parallel import get_landmark
+
+def visualize(img_arr, dpi):
+ plt.figure(figsize=(10,10),dpi=dpi)
+ plt.imshow(((img_arr.detach().cpu().numpy().transpose(1, 2, 0) + 1.0) * 127.5).astype(np.uint8))
+ plt.axis('off')
+ plt.show()
+
+def save_image(img, filename):
+ tmp = ((img.detach().cpu().numpy().transpose(1, 2, 0) + 1.0) * 127.5).astype(np.uint8)
+ cv2.imwrite(filename, cv2.cvtColor(tmp, cv2.COLOR_RGB2BGR))
+
+def load_image(filename):
+ transform = transforms.Compose([
+ transforms.ToTensor(),
+ transforms.Normalize(mean=[0.5, 0.5, 0.5],std=[0.5,0.5,0.5]),
+ ])
+
+ img = Image.open(filename)
+ img = transform(img)
+ return img.unsqueeze(dim=0)
+
+def get_video_crop_parameter(filepath, predictor, padding=[256,256,256,256]):
+ if type(filepath) == str:
+ img = dlib.load_rgb_image(filepath)
+ else:
+ img = filepath
+ lm = get_landmark(img, predictor)
+ if lm is None:
+ return None
+ lm_chin = lm[0 : 17] # left-right
+ lm_eyebrow_left = lm[17 : 22] # left-right
+ lm_eyebrow_right = lm[22 : 27] # left-right
+ lm_nose = lm[27 : 31] # top-down
+ lm_nostrils = lm[31 : 36] # top-down
+ lm_eye_left = lm[36 : 42] # left-clockwise
+ lm_eye_right = lm[42 : 48] # left-clockwise
+ lm_mouth_outer = lm[48 : 60] # left-clockwise
+ lm_mouth_inner = lm[60 : 68] # left-clockwise
+
+ scale = 64. / (np.mean(lm_eye_right[:,0])-np.mean(lm_eye_left[:,0]))
+ center = ((np.mean(lm_eye_right, axis=0)+np.mean(lm_eye_left, axis=0)) / 2) * scale
+ h, w = round(img.shape[0] * scale), round(img.shape[1] * scale)
+ left = max(round(center[0] - padding[0]), 0) // 8 * 8
+ right = min(round(center[0] + padding[1]), w) // 8 * 8
+ top = max(round(center[1] - padding[2]), 0) // 8 * 8
+ bottom = min(round(center[1] + padding[3]), h) // 8 * 8
+ return h,w,top,bottom,left,right,scale
+
+def tensor2cv2(img):
+ tmp = ((img.cpu().numpy().transpose(1, 2, 0) + 1.0) * 127.5).astype(np.uint8)
+ return cv2.cvtColor(tmp, cv2.COLOR_RGB2BGR)
+
+def noise_regularize(noises):
+ loss = 0
+
+ for noise in noises:
+ size = noise.shape[2]
+
+ while True:
+ loss = (
+ loss
+ + (noise * torch.roll(noise, shifts=1, dims=3)).mean().pow(2)
+ + (noise * torch.roll(noise, shifts=1, dims=2)).mean().pow(2)
+ )
+
+ if size <= 8:
+ break
+
+ #noise = noise.reshape([-1, 1, size // 2, 2, size // 2, 2])
+ #noise = noise.mean([3, 5])
+ noise = F.interpolate(noise, scale_factor=0.5, mode='bilinear')
+ size //= 2
+
+ return loss
+
+
+def noise_normalize_(noises):
+ for noise in noises:
+ mean = noise.mean()
+ std = noise.std()
+
+ noise.data.add_(-mean).div_(std)
+
+
+def get_lr(t, initial_lr, rampdown=0.25, rampup=0.05):
+ lr_ramp = min(1, (1 - t) / rampdown)
+ lr_ramp = 0.5 - 0.5 * math.cos(lr_ramp * math.pi)
+ lr_ramp = lr_ramp * min(1, t / rampup)
+
+ return initial_lr * lr_ramp
+
+
+def latent_noise(latent, strength):
+ noise = torch.randn_like(latent) * strength
+
+ return latent + noise
+
+
+def make_image(tensor):
+ return (
+ tensor.detach()
+ .clamp_(min=-1, max=1)
+ .add(1)
+ .div_(2)
+ .mul(255)
+ .type(torch.uint8)
+ .permute(0, 2, 3, 1)
+ .to("cpu")
+ .numpy()
+ )
+
+
+# from pix2pixeHD
+# Converts a one-hot tensor into a colorful label map
+def tensor2label(label_tensor, n_label, imtype=np.uint8):
+ if n_label == 0:
+ return tensor2im(label_tensor, imtype)
+ label_tensor = label_tensor.cpu().float()
+ if label_tensor.size()[0] > 1:
+ label_tensor = label_tensor.max(0, keepdim=True)[1]
+ label_tensor = Colorize(n_label)(label_tensor)
+ label_numpy = np.transpose(label_tensor.numpy(), (1, 2, 0))
+ return label_numpy.astype(imtype)
+
+def uint82bin(n, count=8):
+ """returns the binary of integer n, count refers to amount of bits"""
+ return ''.join([str((n >> y) & 1) for y in range(count-1, -1, -1)])
+
+def labelcolormap(N):
+ if N == 35: # cityscape
+ cmap = np.array([( 0, 0, 0), ( 0, 0, 0), ( 0, 0, 0), ( 0, 0, 0), ( 0, 0, 0), (111, 74, 0), ( 81, 0, 81),
+ (128, 64,128), (244, 35,232), (250,170,160), (230,150,140), ( 70, 70, 70), (102,102,156), (190,153,153),
+ (180,165,180), (150,100,100), (150,120, 90), (153,153,153), (153,153,153), (250,170, 30), (220,220, 0),
+ (107,142, 35), (152,251,152), ( 70,130,180), (220, 20, 60), (255, 0, 0), ( 0, 0,142), ( 0, 0, 70),
+ ( 0, 60,100), ( 0, 0, 90), ( 0, 0,110), ( 0, 80,100), ( 0, 0,230), (119, 11, 32), ( 0, 0,142)],
+ dtype=np.uint8)
+ else:
+ cmap = np.zeros((N, 3), dtype=np.uint8)
+ for i in range(N):
+ r, g, b = 0, 0, 0
+ id = i
+ for j in range(7):
+ str_id = uint82bin(id)
+ r = r ^ (np.uint8(str_id[-1]) << (7-j))
+ g = g ^ (np.uint8(str_id[-2]) << (7-j))
+ b = b ^ (np.uint8(str_id[-3]) << (7-j))
+ id = id >> 3
+ cmap[i, 0] = r
+ cmap[i, 1] = g
+ cmap[i, 2] = b
+ return cmap
+
+class Colorize(object):
+ def __init__(self, n=35):
+ self.cmap = labelcolormap(n)
+ self.cmap = torch.from_numpy(self.cmap[:n])
+
+ def __call__(self, gray_image):
+ size = gray_image.size()
+ color_image = torch.ByteTensor(3, size[1], size[2]).fill_(0)
+
+ for label in range(0, len(self.cmap)):
+ mask = (label == gray_image[0]).cpu()
+ color_image[0][mask] = self.cmap[label][0]
+ color_image[1][mask] = self.cmap[label][1]
+ color_image[2][mask] = self.cmap[label][2]
+
+ return color_image
\ No newline at end of file
diff --git a/utils/train_utils.py b/utils/train_utils.py
new file mode 100644
index 0000000000000000000000000000000000000000..0c55177f7442010bc1fcc64de3d142585c22adc0
--- /dev/null
+++ b/utils/train_utils.py
@@ -0,0 +1,13 @@
+
+def aggregate_loss_dict(agg_loss_dict):
+ mean_vals = {}
+ for output in agg_loss_dict:
+ for key in output:
+ mean_vals[key] = mean_vals.setdefault(key, []) + [output[key]]
+ for key in mean_vals:
+ if len(mean_vals[key]) > 0:
+ mean_vals[key] = sum(mean_vals[key]) / len(mean_vals[key])
+ else:
+ print('{} has no value'.format(key))
+ mean_vals[key] = 0
+ return mean_vals
diff --git a/utils/wandb_utils.py b/utils/wandb_utils.py
new file mode 100644
index 0000000000000000000000000000000000000000..0061eb569dee40bbe68f244b286976412fe6dece
--- /dev/null
+++ b/utils/wandb_utils.py
@@ -0,0 +1,47 @@
+import datetime
+import os
+import numpy as np
+import wandb
+
+from utils import common
+
+
+class WBLogger:
+
+ def __init__(self, opts):
+ wandb_run_name = os.path.basename(opts.exp_dir)
+ wandb.init(project="pixel2style2pixel", config=vars(opts), name=wandb_run_name)
+
+ @staticmethod
+ def log_best_model():
+ wandb.run.summary["best-model-save-time"] = datetime.datetime.now()
+
+ @staticmethod
+ def log(prefix, metrics_dict, global_step):
+ log_dict = {f'{prefix}_{key}': value for key, value in metrics_dict.items()}
+ log_dict["global_step"] = global_step
+ wandb.log(log_dict)
+
+ @staticmethod
+ def log_dataset_wandb(dataset, dataset_name, n_images=16):
+ idxs = np.random.choice(a=range(len(dataset)), size=n_images, replace=False)
+ data = [wandb.Image(dataset.source_paths[idx]) for idx in idxs]
+ wandb.log({f"{dataset_name} Data Samples": data})
+
+ @staticmethod
+ def log_images_to_wandb(x, y, y_hat, id_logs, prefix, step, opts):
+ im_data = []
+ column_names = ["Source", "Target", "Output"]
+ if id_logs is not None:
+ column_names.append("ID Diff Output to Target")
+ for i in range(len(x)):
+ cur_im_data = [
+ wandb.Image(common.log_input_image(x[i], opts)),
+ wandb.Image(common.tensor2im(y[i])),
+ wandb.Image(common.tensor2im(y_hat[i])),
+ ]
+ if id_logs is not None:
+ cur_im_data.append(id_logs[i]["diff_target"])
+ im_data.append(cur_im_data)
+ outputs_table = wandb.Table(data=im_data, columns=column_names)
+ wandb.log({f"{prefix.title()} Step {step} Output Samples": outputs_table})
diff --git a/webUI/app_task.py b/webUI/app_task.py
new file mode 100644
index 0000000000000000000000000000000000000000..daf6d529771de355a91b73aa58b787e41ff4c225
--- /dev/null
+++ b/webUI/app_task.py
@@ -0,0 +1,305 @@
+from __future__ import annotations
+from huggingface_hub import hf_hub_download
+import numpy as np
+import gradio as gr
+
+
+def create_demo_sr(process):
+ with gr.Blocks() as demo:
+ with gr.Row():
+ gr.Markdown('## Face Super Resolution')
+ with gr.Row():
+ with gr.Column():
+ input_image = gr.Image(source='upload', type='filepath')
+ model_type = gr.Radio(label='Model Type', choices=['SR for 32x','SR for 4x-48x'], value='SR for 32x')
+ resize_scale = gr.Slider(label='Resize Scale',
+ minimum=4,
+ maximum=48,
+ value=32,
+ step=4)
+ run_button = gr.Button(label='Run')
+ gr.Examples(
+ examples =[['pexels-daniel-xavier-1239291.jpg', 'SR for 32x', 32],
+ ['ILip77SbmOE.png', 'SR for 32x', 32],
+ ['ILip77SbmOE.png', 'SR for 4x-48x', 48],
+ ],
+ inputs = [input_image, model_type, resize_scale],
+ )
+ with gr.Column():
+ #lrinput = gr.Image(label='Low-resolution input',type='numpy', interactive=False)
+ #result = gr.Image(label='Output',type='numpy', interactive=False)
+ result = gr.Gallery(label='LR input and Output',
+ elem_id='gallery').style(grid=2,
+ height='auto')
+
+ inputs = [
+ input_image,
+ resize_scale,
+ model_type,
+ ]
+ run_button.click(fn=process,
+ inputs=inputs,
+ outputs=[result],
+ api_name='sr')
+ return demo
+
+def create_demo_s2f(process):
+ with gr.Blocks() as demo:
+ with gr.Row():
+ gr.Markdown('## Sketch-to-Face Translation')
+ with gr.Row():
+ with gr.Column():
+ input_image = gr.Image(source='upload', type='filepath')
+ gr.Markdown("""Note: Input will be cropped if larger than 512x512.""")
+ seed = gr.Slider(label='Seed for appearance',
+ minimum=0,
+ maximum=2147483647,
+ step=1,
+ randomize=True)
+ #input_info = gr.Textbox(label='Process Information', interactive=False, value='n.a.')
+ run_button = gr.Button(label='Run')
+ gr.Examples(
+ examples =[['234_sketch.jpg', 1024]],
+ inputs = [input_image, seed],
+ )
+ with gr.Column():
+ result = gr.Image(label='Output',type='numpy', interactive=False)
+
+ inputs = [
+ input_image, seed
+ ]
+ run_button.click(fn=process,
+ inputs=inputs,
+ outputs=[result],
+ api_name='s2f')
+ return demo
+
+
+def create_demo_m2f(process):
+ with gr.Blocks() as demo:
+ with gr.Row():
+ gr.Markdown('## Mask-to-Face Translation')
+ with gr.Row():
+ with gr.Column():
+ input_image = gr.Image(source='upload', type='filepath')
+ input_type = gr.Radio(label='Input Type', choices=['color image','parsing mask'], value='color image')
+ seed = gr.Slider(label='Seed for appearance',
+ minimum=0,
+ maximum=2147483647,
+ step=1,
+ randomize=True)
+ #input_info = gr.Textbox(label='Process Information', interactive=False, value='n.a.')
+ run_button = gr.Button(label='Run')
+ gr.Examples(
+ examples =[['ILip77SbmOE.png', 'color image', 4], ['ILip77SbmOE_mask.png', 'parsing mask', 4]],
+ inputs = [input_image, input_type, seed],
+ )
+ with gr.Column():
+ #vizmask = gr.Image(label='Visualized mask',type='numpy', interactive=False)
+ #result = gr.Image(label='Output',type='numpy', interactive=False)
+ result = gr.Gallery(label='Visualized mask and Output',
+ elem_id='gallery').style(grid=2,
+ height='auto')
+
+ inputs = [
+ input_image, input_type, seed
+ ]
+ run_button.click(fn=process,
+ inputs=inputs,
+ outputs=[result],
+ api_name='m2f')
+ return demo
+
+def create_demo_editing(process):
+ with gr.Blocks() as demo:
+ with gr.Row():
+ gr.Markdown('## Video Face Editing (for image input)')
+ with gr.Row():
+ with gr.Column():
+ input_image = gr.Image(source='upload', type='filepath')
+ model_type = gr.Radio(label='Editing Type', choices=['reduce age','light hair color'], value='color image')
+ scale_factor = gr.Slider(label='editing degree (-2~2)',
+ minimum=-2,
+ maximum=2,
+ value=1,
+ step=0.1)
+ #input_info = gr.Textbox(label='Process Information', interactive=False, value='n.a.')
+ run_button = gr.Button(label='Run')
+ gr.Examples(
+ examples =[['ILip77SbmOE.png', 'reduce age', -2],
+ ['ILip77SbmOE.png', 'light hair color', 1]],
+ inputs = [input_image, model_type, scale_factor],
+ )
+ with gr.Column():
+ result = gr.Image(label='Output',type='numpy', interactive=False)
+
+ inputs = [
+ input_image, scale_factor, model_type
+ ]
+ run_button.click(fn=process,
+ inputs=inputs,
+ outputs=[result],
+ api_name='editing')
+ return demo
+
+def create_demo_toonify(process):
+ with gr.Blocks() as demo:
+ with gr.Row():
+ gr.Markdown('## Video Face Toonification (for image input)')
+ with gr.Row():
+ with gr.Column():
+ input_image = gr.Image(source='upload', type='filepath')
+ style_type = gr.Radio(label='Style Type', choices=['Pixar','Cartoon','Arcane'], value='Pixar')
+ #input_info = gr.Textbox(label='Process Information', interactive=False, value='n.a.')
+ run_button = gr.Button(label='Run')
+ gr.Examples(
+ examples =[['ILip77SbmOE.png', 'Pixar'], ['ILip77SbmOE.png', 'Cartoon'], ['ILip77SbmOE.png', 'Arcane']],
+ inputs = [input_image, style_type],
+ )
+ with gr.Column():
+ result = gr.Image(label='Output',type='numpy', interactive=False)
+
+ inputs = [
+ input_image, style_type
+ ]
+ run_button.click(fn=process,
+ inputs=inputs,
+ outputs=[result],
+ api_name='toonify')
+ return demo
+
+
+def create_demo_vediting(process, max_frame_num = 4):
+ with gr.Blocks() as demo:
+ with gr.Row():
+ gr.Markdown('## Video Face Editing (for video input)')
+ with gr.Row():
+ with gr.Column():
+ input_video = gr.Video(source='upload', mirror_webcam=False, type='filepath')
+ model_type = gr.Radio(label='Editing Type', choices=['reduce age','light hair color'], value='color image')
+ scale_factor = gr.Slider(label='editing degree (-2~2)',
+ minimum=-2,
+ maximum=2,
+ value=1,
+ step=0.1)
+ frame_num = gr.Slider(label='Number of frames to edit (full video editing is not allowed so as not to slow down the demo, \
+ but you can duplicate the Space to modify the number limit to a large value)',
+ minimum=1,
+ maximum=max_frame_num,
+ value=4,
+ step=1)
+ #input_info = gr.Textbox(label='Process Information', interactive=False, value='n.a.')
+ run_button = gr.Button(label='Run')
+ gr.Examples(
+ examples =[['684.mp4', 'reduce age', 1.5, 2],
+ ['684.mp4', 'light hair color', 0.7, 2]],
+ inputs = [input_video, model_type, scale_factor],
+ )
+ with gr.Column():
+ viz_result = gr.Gallery(label='Several edited frames', elem_id='gallery').style(grid=2, height='auto')
+ result = gr.Video(label='Output', type='mp4', interactive=False)
+
+ inputs = [
+ input_video, scale_factor, model_type, frame_num
+ ]
+ run_button.click(fn=process,
+ inputs=inputs,
+ outputs=[viz_result, result],
+ api_name='vediting')
+ return demo
+
+def create_demo_vtoonify(process, max_frame_num = 4):
+ with gr.Blocks() as demo:
+ with gr.Row():
+ gr.Markdown('## Video Face Toonification (for video input)')
+ with gr.Row():
+ with gr.Column():
+ input_video = gr.Video(source='upload', mirror_webcam=False, type='filepath')
+ style_type = gr.Radio(label='Style Type', choices=['Pixar','Cartoon','Arcane'], value='Pixar')
+ frame_num = gr.Slider(label='Number of frames to toonify (full video toonification is not allowed so as not to slow down the demo, \
+ but you can duplicate the Space to modify the number limit to a large value)',
+ minimum=1,
+ maximum=max_frame_num,
+ value=4,
+ step=1)
+ #input_info = gr.Textbox(label='Process Information', interactive=False, value='n.a.')
+ run_button = gr.Button(label='Run')
+ gr.Examples(
+ examples =[['529_2.mp4', 'Arcane'],
+ ['pexels-anthony-shkraba-production-8136210.mp4', 'Pixar'],
+ ['684.mp4', 'Cartoon']],
+ inputs = [input_video, style_type],
+ )
+ with gr.Column():
+ viz_result = gr.Gallery(label='Several toonified frames', elem_id='gallery').style(grid=2, height='auto')
+ result = gr.Video(label='Output', type='mp4', interactive=False)
+
+ inputs = [
+ input_video, style_type, frame_num
+ ]
+ run_button.click(fn=process,
+ inputs=inputs,
+ outputs=[viz_result, result],
+ api_name='vtoonify')
+ return demo
+
+def create_demo_inversion(process, allow_optimization=False):
+ with gr.Blocks() as demo:
+ with gr.Row():
+ gr.Markdown('## StyleGANEX Inversion for Editing')
+ with gr.Row():
+ with gr.Column():
+ input_image = gr.Image(source='upload', type='filepath')
+ optimize = gr.Radio(label='Whether optimize latent (latent optimization is not allowed so as not to slow down the demo, \
+ but you can duplicate the Space to modify the option or directly upload an optimized latent file. \
+ The file can be computed by inversion.py from the github page or colab)', choices=['No optimization','Latent optimization'],
+ value='No optimization', interactive=allow_optimization)
+ input_latent = gr.File(label='Optimized latent code (optional)', file_types=[".pt"])
+ editing_options = gr.Dropdown(['None', 'Style Mixing',
+ 'Attribute Editing: smile',
+ 'Attribute Editing: open_eye',
+ 'Attribute Editing: open_mouth',
+ 'Attribute Editing: pose',
+ 'Attribute Editing: reduce_age',
+ 'Attribute Editing: glasses',
+ 'Attribute Editing: light_hair_color',
+ 'Attribute Editing: slender',
+ 'Domain Transfer: disney_princess',
+ 'Domain Transfer: vintage_comics',
+ 'Domain Transfer: pixar',
+ 'Domain Transfer: edvard_munch',
+ 'Domain Transfer: modigliani',
+ ],
+ label="editing options",
+ value='None')
+ scale_factor = gr.Slider(label='editing degree (-2~2) for Attribute Editing',
+ minimum=-2,
+ maximum=2,
+ value=2,
+ step=0.1)
+ seed = gr.Slider(label='Appearance Seed for Style Mixing',
+ minimum=0,
+ maximum=2147483647,
+ step=1,
+ randomize=True)
+ #input_info = gr.Textbox(label='Process Information', interactive=False, value='n.a.')
+ run_button = gr.Button(label='Run')
+ gr.Examples(
+ examples =[['ILip77SbmOE.png', 'ILip77SbmOE_inversion.pt', 'Domain Transfer: vintage_comics'],
+ ['ILip77SbmOE.png', 'ILip77SbmOE_inversion.pt', 'Attribute Editing: smile'],
+ ['ILip77SbmOE.png', 'ILip77SbmOE_inversion.pt', 'Style Mixing'],
+ ],
+ inputs = [input_image, input_latent, editing_options],
+ )
+ with gr.Column():
+ result = gr.Image(label='Inversion output',type='numpy', interactive=False)
+ editing_result = gr.Image(label='Editing output',type='numpy', interactive=False)
+
+ inputs = [
+ input_image, optimize, input_latent, editing_options, scale_factor, seed
+ ]
+ run_button.click(fn=process,
+ inputs=inputs,
+ outputs=[result, editing_result],
+ api_name='inversion')
+ return demo
\ No newline at end of file
diff --git a/webUI/styleganex_model.py b/webUI/styleganex_model.py
new file mode 100644
index 0000000000000000000000000000000000000000..18c679bffc56b0783da2c909a92f4568ec91adaf
--- /dev/null
+++ b/webUI/styleganex_model.py
@@ -0,0 +1,492 @@
+from __future__ import annotations
+import numpy as np
+import gradio as gr
+
+import os
+import pathlib
+import gc
+import torch
+import dlib
+import cv2
+import PIL
+from tqdm import tqdm
+import numpy as np
+import torch.nn.functional as F
+import torchvision
+from torchvision import transforms, utils
+from argparse import Namespace
+from datasets import augmentations
+from huggingface_hub import hf_hub_download
+from scripts.align_all_parallel import align_face
+from latent_optimization import latent_optimization
+from utils.inference_utils import save_image, load_image, visualize, get_video_crop_parameter, tensor2cv2, tensor2label, labelcolormap
+from models.psp import pSp
+from models.bisenet.model import BiSeNet
+from models.stylegan2.model import Generator
+
+class Model():
+ def __init__(self, device):
+ super().__init__()
+
+ self.device = device
+ self.task_name = None
+ self.editing_w = None
+ self.pspex = None
+ self.landmarkpredictor = dlib.shape_predictor(hf_hub_download('PKUWilliamYang/VToonify', 'models/shape_predictor_68_face_landmarks.dat'))
+ self.transform = transforms.Compose([
+ transforms.ToTensor(),
+ transforms.Normalize(mean=[0.5, 0.5, 0.5],std=[0.5,0.5,0.5]),
+ ])
+ self.to_tensor = transforms.Compose([
+ transforms.ToTensor(),
+ transforms.Normalize((0.485, 0.456, 0.406), (0.229, 0.224, 0.225)),
+ ])
+ self.maskpredictor = BiSeNet(n_classes=19)
+ self.maskpredictor.load_state_dict(torch.load(hf_hub_download('PKUWilliamYang/VToonify', 'models/faceparsing.pth'), map_location='cpu'))
+ self.maskpredictor.to(self.device).eval()
+ self.parameters = {}
+ self.parameters['inversion'] = {'path':'pretrained_models/styleganex_inversion.pt', 'image_path':'./data/ILip77SbmOE.png'}
+ self.parameters['sr-32'] = {'path':'pretrained_models/styleganex_sr32.pt', 'image_path':'./data/pexels-daniel-xavier-1239291.jpg'}
+ self.parameters['sr'] = {'path':'pretrained_models/styleganex_sr.pt', 'image_path':'./data/pexels-daniel-xavier-1239291.jpg'}
+ self.parameters['sketch2face'] = {'path':'pretrained_models/styleganex_sketch2face.pt', 'image_path':'./data/234_sketch.jpg'}
+ self.parameters['mask2face'] = {'path':'pretrained_models/styleganex_mask2face.pt', 'image_path':'./data/540.jpg'}
+ self.parameters['edit_age'] = {'path':'pretrained_models/styleganex_edit_age.pt', 'image_path':'./data/390.mp4'}
+ self.parameters['edit_hair'] = {'path':'pretrained_models/styleganex_edit_hair.pt', 'image_path':'./data/390.mp4'}
+ self.parameters['toonify_pixar'] = {'path':'pretrained_models/styleganex_toonify_pixar.pt', 'image_path':'./data/pexels-anthony-shkraba-production-8136210.mp4'}
+ self.parameters['toonify_cartoon'] = {'path':'pretrained_models/styleganex_toonify_cartoon.pt', 'image_path':'./data/pexels-anthony-shkraba-production-8136210.mp4'}
+ self.parameters['toonify_arcane'] = {'path':'pretrained_models/styleganex_toonify_arcane.pt', 'image_path':'./data/pexels-anthony-shkraba-production-8136210.mp4'}
+ self.print_log = True
+ self.editing_dicts = torch.load(hf_hub_download('PKUWilliamYang/StyleGANEX', 'direction_dics.pt'))
+ self.generator = Generator(1024, 512, 8)
+ self.model_type = None
+ self.error_info = 'Error: no face detected! \
+ StyleGANEX uses dlib.get_frontal_face_detector but sometimes it fails to detect a face. \
+ You can try several times or use other images until a face is detected, \
+ then switch back to the original image.'
+
+ def load_model(self, task_name: str) -> None:
+ if task_name == self.task_name:
+ return
+ if self.pspex is not None:
+ del self.pspex
+ torch.cuda.empty_cache()
+ gc.collect()
+ path = self.parameters[task_name]['path']
+ local_path = hf_hub_download('PKUWilliamYang/StyleGANEX', path)
+ ckpt = torch.load(local_path, map_location='cpu')
+ opts = ckpt['opts']
+ opts['checkpoint_path'] = local_path
+ opts['device'] = self.device
+ opts = Namespace(**opts)
+ self.pspex = pSp(opts, ckpt).to(self.device).eval()
+ self.pspex.latent_avg = self.pspex.latent_avg.to(self.device)
+ if 'editing_w' in ckpt.keys():
+ self.editing_w = ckpt['editing_w'].clone().to(self.device)
+ self.task_name = task_name
+ torch.cuda.empty_cache()
+ gc.collect()
+
+ def load_G_model(self, model_type: str) -> None:
+ if model_type == self.model_type:
+ return
+ torch.cuda.empty_cache()
+ gc.collect()
+ local_path = hf_hub_download('rinong/stylegan-nada-models', model_type+'.pt')
+ self.generator.load_state_dict(torch.load(local_path, map_location='cpu')['g_ema'], strict=False)
+ self.generator.to(self.device).eval()
+ self.model_type = model_type
+ torch.cuda.empty_cache()
+ gc.collect()
+
+ def tensor2np(self, img):
+ tmp = ((img.cpu().numpy().transpose(1, 2, 0) + 1.0) * 127.5).astype(np.uint8)
+ return tmp
+
+ def process_sr(self, input_image: str, resize_scale: int, model: str) -> list[np.ndarray]:
+ #false_image = np.zeros((256,256,3), np.uint8)
+ #info = 'Error: no face detected! Please retry or change the photo.'
+
+ if input_image is None:
+ #return [false_image, false_image], 'Error: fail to load empty file.'
+ raise gr.Error("Error: fail to load empty file.")
+ frame = cv2.imread(input_image)
+ if frame is None:
+ #return [false_image, false_image], 'Error: fail to load the image.'
+ raise gr.Error("Error: fail to load the image.")
+ frame = cv2.cvtColor(frame, cv2.COLOR_BGR2RGB)
+
+ if model is None or model == 'SR for 32x':
+ task_name = 'sr-32'
+ resize_scale = 32
+ else:
+ task_name = 'sr'
+
+ with torch.no_grad():
+ paras = get_video_crop_parameter(frame, self.landmarkpredictor)
+ if paras is None:
+ #return [false_image, false_image], info
+ raise gr.Error(self.error_info)
+ h,w,top,bottom,left,right,scale = paras
+ H, W = int(bottom-top), int(right-left)
+ frame = cv2.resize(frame, (w, h))[top:bottom, left:right]
+ x1 = PIL.Image.fromarray(np.uint8(frame))
+ x1 = augmentations.BilinearResize(factors=[resize_scale//4])(x1)
+ x1_up = x1.resize((W, H))
+ x2_up = align_face(np.array(x1_up), self.landmarkpredictor)
+ if x2_up is None:
+ #return [false_image, false_image], 'Error: no face detected! Please retry or change the photo.'
+ raise gr.Error(self.error_info)
+ x1_up = transforms.ToTensor()(x1_up).unsqueeze(dim=0).to(self.device) * 2 - 1
+ x2_up = self.transform(x2_up).unsqueeze(dim=0).to(self.device)
+ if self.print_log: print('image loaded')
+ self.load_model(task_name)
+ if self.print_log: print('model %s loaded'%(task_name))
+ y_hat = torch.clamp(self.pspex(x1=x1_up, x2=x2_up, use_skip=self.pspex.opts.use_skip, resize=False), -1, 1)
+
+ return [self.tensor2np(x1_up[0]), self.tensor2np(y_hat[0])]
+
+
+ def process_s2f(self, input_image: str, seed: int) -> np.ndarray:
+ task_name = 'sketch2face'
+ with torch.no_grad():
+ x1 = transforms.ToTensor()(PIL.Image.open(input_image)).unsqueeze(0).to(self.device)
+ if x1.shape[2] > 513:
+ x1 = x1[:,:,(x1.shape[2]//2-256)//8*8:(x1.shape[2]//2+256)//8*8]
+ if x1.shape[3] > 513:
+ x1 = x1[:,:,:,(x1.shape[3]//2-256)//8*8:(x1.shape[3]//2+256)//8*8]
+ x1 = x1[:,0:1] # uploaded files will be transformed to 3-channel RGB image!
+ if self.print_log: print('image loaded')
+ self.load_model(task_name)
+ if self.print_log: print('model %s loaded'%(task_name))
+ self.pspex.train()
+ torch.manual_seed(seed)
+ y_hat = self.pspex(x1=x1, resize=False, latent_mask=[8,9,10,11,12,13,14,15,16,17], use_skip=self.pspex.opts.use_skip,
+ inject_latent= self.pspex.decoder.style(torch.randn(1, 512).to(self.device)).unsqueeze(1).repeat(1,18,1) * 0.7)
+ y_hat = torch.clamp(y_hat, -1, 1)
+ self.pspex.eval()
+ return self.tensor2np(y_hat[0])
+
+ def process_m2f(self, input_image: str, input_type: str, seed: int) -> list[np.ndarray]:
+ #false_image = np.zeros((256,256,3), np.uint8)
+ if input_image is None:
+ raise gr.Error('Error: fail to load empty file.' )
+ #return [false_image, false_image], 'Error: fail to load empty file.'
+ task_name = 'mask2face'
+ with torch.no_grad():
+ if input_type == 'parsing mask':
+ x1 = PIL.Image.open(input_image).getchannel(0) # uploaded files will be transformed to 3-channel RGB image!
+ x1 = augmentations.ToOneHot(19)(x1)
+ x1 = transforms.ToTensor()(x1).unsqueeze(dim=0).float().to(self.device)
+ #print(x1.shape)
+ else:
+ frame = cv2.imread(input_image)
+ if frame is None:
+ #return [false_image, false_image], 'Error: fail to load the image.'
+ raise gr.Error('Error: fail to load the image.' )
+ frame = cv2.cvtColor(frame, cv2.COLOR_BGR2RGB)
+ paras = get_video_crop_parameter(frame, self.landmarkpredictor)
+ if paras is None:
+ #return [false_image, false_image], 'Error: no face detected! Please retry or change the photo.'
+ raise gr.Error(self.error_info)
+ h,w,top,bottom,left,right,scale = paras
+ H, W = int(bottom-top), int(right-left)
+ frame = cv2.resize(frame, (w, h))[top:bottom, left:right]
+ # convert face image to segmentation mask
+ x1 = self.to_tensor(frame).unsqueeze(0).to(self.device)
+ # upsample image for precise segmentation
+ x1 = F.interpolate(x1, scale_factor=2, mode='bilinear')
+ x1 = self.maskpredictor(x1)[0]
+ x1 = F.interpolate(x1, scale_factor=0.5).argmax(dim=1)
+ x1 = F.one_hot(x1, num_classes=19).permute(0, 3, 1, 2).float().to(self.device)
+
+ if x1.shape[2] > 513:
+ x1 = x1[:,:,(x1.shape[2]//2-256)//8*8:(x1.shape[2]//2+256)//8*8]
+ if x1.shape[3] > 513:
+ x1 = x1[:,:,:,(x1.shape[3]//2-256)//8*8:(x1.shape[3]//2+256)//8*8]
+
+ x1_viz = (tensor2label(x1[0], 19) / 192 * 256).astype(np.uint8)
+
+ if self.print_log: print('image loaded')
+ self.load_model(task_name)
+ if self.print_log: print('model %s loaded'%(task_name))
+ self.pspex.train()
+ torch.manual_seed(seed)
+ y_hat = self.pspex(x1=x1, resize=False, latent_mask=[8,9,10,11,12,13,14,15,16,17], use_skip=self.pspex.opts.use_skip,
+ inject_latent= self.pspex.decoder.style(torch.randn(1, 512).to(self.device)).unsqueeze(1).repeat(1,18,1) * 0.7)
+ y_hat = torch.clamp(y_hat, -1, 1)
+ self.pspex.eval()
+ return [x1_viz, self.tensor2np(y_hat[0])]
+
+
+ def process_editing(self, input_image: str, scale_factor: float, model_type: str) -> np.ndarray:
+ #false_image = np.zeros((256,256,3), np.uint8)
+ #info = 'Error: no face detected! Please retry or change the photo.'
+
+ if input_image is None:
+ #return false_image, false_image, 'Error: fail to load empty file.'
+ raise gr.Error('Error: fail to load empty file.')
+ frame = cv2.imread(input_image)
+ if frame is None:
+ #return false_image, false_image, 'Error: fail to load the image.'
+ raise gr.Error('Error: fail to load the image.')
+ frame = cv2.cvtColor(frame, cv2.COLOR_BGR2RGB)
+
+ if model_type is None or model_type == 'reduce age':
+ task_name = 'edit_age'
+ else:
+ task_name = 'edit_hair'
+
+ with torch.no_grad():
+ paras = get_video_crop_parameter(frame, self.landmarkpredictor)
+ if paras is None:
+ #return false_image, false_image, info
+ raise gr.Error(self.error_info)
+ h,w,top,bottom,left,right,scale = paras
+ H, W = int(bottom-top), int(right-left)
+ frame = cv2.resize(frame, (w, h))[top:bottom, left:right]
+ x1 = self.transform(frame).unsqueeze(0).to(self.device)
+ x2 = align_face(frame, self.landmarkpredictor)
+ if x2 is None:
+ #return false_image, 'Error: no face detected! Please retry or change the photo.'
+ raise gr.Error(self.error_info)
+ x2 = self.transform(x2).unsqueeze(dim=0).to(self.device)
+ if self.print_log: print('image loaded')
+ self.load_model(task_name)
+ if self.print_log: print('model %s loaded'%(task_name))
+ y_hat = self.pspex(x1=x1, x2=x2, use_skip=self.pspex.opts.use_skip, zero_noise=True,
+ resize=False, editing_w= - scale_factor* self.editing_w[0:1])
+ y_hat = torch.clamp(y_hat, -1, 1)
+
+ return self.tensor2np(y_hat[0])
+
+ def process_vediting(self, input_video: str, scale_factor: float, model_type: str, frame_num: int) -> tuple[list[np.ndarray], str]:
+ #false_image = np.zeros((256,256,3), np.uint8)
+ #info = 'Error: no face detected! Please retry or change the video.'
+
+ if input_video is None:
+ #return [false_image], 'default.mp4', 'Error: fail to load empty file.'
+ raise gr.Error('Error: fail to load empty file.')
+ video_cap = cv2.VideoCapture(input_video)
+ success, frame = video_cap.read()
+ if success is False:
+ #return [false_image], 'default.mp4', 'Error: fail to load the video.'
+ raise gr.Error('Error: fail to load the video.')
+ frame = cv2.cvtColor(frame, cv2.COLOR_BGR2RGB)
+
+ if model_type is None or model_type == 'reduce age':
+ task_name = 'edit_age'
+ else:
+ task_name = 'edit_hair'
+
+ with torch.no_grad():
+ paras = get_video_crop_parameter(frame, self.landmarkpredictor)
+ if paras is None:
+ #return [false_image], 'default.mp4', info
+ raise gr.Error(self.error_info)
+ h,w,top,bottom,left,right,scale = paras
+ H, W = int(bottom-top), int(right-left)
+ frame = cv2.resize(frame, (w, h))[top:bottom, left:right]
+ x1 = self.transform(frame).unsqueeze(0).to(self.device)
+ x2 = align_face(frame, self.landmarkpredictor)
+ if x2 is None:
+ #return [false_image], 'default.mp4', info
+ raise gr.Error(self.error_info)
+ x2 = self.transform(x2).unsqueeze(dim=0).to(self.device)
+ if self.print_log: print('first frame loaded')
+ self.load_model(task_name)
+ if self.print_log: print('model %s loaded'%(task_name))
+
+ fourcc = cv2.VideoWriter_fourcc(*'mp4v')
+ videoWriter = cv2.VideoWriter('output.mp4', fourcc, video_cap.get(5), (4*W, 4*H))
+
+ viz_frames = []
+ for i in range(frame_num):
+ if i > 0:
+ success, frame = video_cap.read()
+ frame = cv2.cvtColor(frame, cv2.COLOR_BGR2RGB)
+ frame = cv2.resize(frame, (w, h))[top:bottom, left:right]
+ x1 = self.transform(frame).unsqueeze(0).to(self.device)
+ y_hat = self.pspex(x1=x1, x2=x2, use_skip=self.pspex.opts.use_skip, zero_noise=True,
+ resize=False, editing_w= - scale_factor * self.editing_w[0:1])
+ y_hat = torch.clamp(y_hat, -1, 1)
+ videoWriter.write(tensor2cv2(y_hat[0].cpu()))
+ if i < min(frame_num, 4):
+ viz_frames += [self.tensor2np(y_hat[0])]
+
+ videoWriter.release()
+
+ return viz_frames, 'output.mp4'
+
+
+ def process_toonify(self, input_image: str, style_type: str) -> np.ndarray:
+ #false_image = np.zeros((256,256,3), np.uint8)
+ #info = 'Error: no face detected! Please retry or change the photo.'
+
+ if input_image is None:
+ raise gr.Error('Error: fail to load empty file.')
+ #return false_image, false_image, 'Error: fail to load empty file.'
+ frame = cv2.imread(input_image)
+ if frame is None:
+ raise gr.Error('Error: fail to load the image.')
+ #return false_image, false_image, 'Error: fail to load the image.'
+ frame = cv2.cvtColor(frame, cv2.COLOR_BGR2RGB)
+
+ if style_type is None or style_type == 'Pixar':
+ task_name = 'toonify_pixar'
+ elif style_type == 'Cartoon':
+ task_name = 'toonify_cartoon'
+ else:
+ task_name = 'toonify_arcane'
+
+ with torch.no_grad():
+ paras = get_video_crop_parameter(frame, self.landmarkpredictor)
+ if paras is None:
+ raise gr.Error(self.error_info)
+ #return false_image, false_image, info
+ h,w,top,bottom,left,right,scale = paras
+ H, W = int(bottom-top), int(right-left)
+ frame = cv2.resize(frame, (w, h))[top:bottom, left:right]
+ x1 = self.transform(frame).unsqueeze(0).to(self.device)
+ x2 = align_face(frame, self.landmarkpredictor)
+ if x2 is None:
+ raise gr.Error(self.error_info)
+ #return false_image, 'Error: no face detected! Please retry or change the photo.'
+ x2 = self.transform(x2).unsqueeze(dim=0).to(self.device)
+ if self.print_log: print('image loaded')
+ self.load_model(task_name)
+ if self.print_log: print('model %s loaded'%(task_name))
+ y_hat = self.pspex(x1=x1, x2=x2, use_skip=self.pspex.opts.use_skip, zero_noise=True, resize=False)
+ y_hat = torch.clamp(y_hat, -1, 1)
+
+ return self.tensor2np(y_hat[0])
+
+
+ def process_vtoonify(self, input_video: str, style_type: str, frame_num: int) -> tuple[list[np.ndarray], str]:
+ #false_image = np.zeros((256,256,3), np.uint8)
+ #info = 'Error: no face detected! Please retry or change the video.'
+
+ if input_video is None:
+ raise gr.Error('Error: fail to load empty file.')
+ #return [false_image], 'default.mp4', 'Error: fail to load empty file.'
+ video_cap = cv2.VideoCapture(input_video)
+ success, frame = video_cap.read()
+ if success is False:
+ raise gr.Error('Error: fail to load the video.')
+ #return [false_image], 'default.mp4', 'Error: fail to load the video.'
+ frame = cv2.cvtColor(frame, cv2.COLOR_BGR2RGB)
+
+ if style_type is None or style_type == 'Pixar':
+ task_name = 'toonify_pixar'
+ elif style_type == 'Cartoon':
+ task_name = 'toonify_cartoon'
+ else:
+ task_name = 'toonify_arcane'
+
+ with torch.no_grad():
+ paras = get_video_crop_parameter(frame, self.landmarkpredictor)
+ if paras is None:
+ raise gr.Error(self.error_info)
+ #return [false_image], 'default.mp4', info
+ h,w,top,bottom,left,right,scale = paras
+ H, W = int(bottom-top), int(right-left)
+ frame = cv2.resize(frame, (w, h))[top:bottom, left:right]
+ x1 = self.transform(frame).unsqueeze(0).to(self.device)
+ x2 = align_face(frame, self.landmarkpredictor)
+ if x2 is None:
+ raise gr.Error(self.error_info)
+ #return [false_image], 'default.mp4', info
+ x2 = self.transform(x2).unsqueeze(dim=0).to(self.device)
+ if self.print_log: print('first frame loaded')
+ self.load_model(task_name)
+ if self.print_log: print('model %s loaded'%(task_name))
+
+ fourcc = cv2.VideoWriter_fourcc(*'mp4v')
+ videoWriter = cv2.VideoWriter('output.mp4', fourcc, video_cap.get(5), (4*W, 4*H))
+
+ viz_frames = []
+ for i in range(frame_num):
+ if i > 0:
+ success, frame = video_cap.read()
+ frame = cv2.cvtColor(frame, cv2.COLOR_BGR2RGB)
+ frame = cv2.resize(frame, (w, h))[top:bottom, left:right]
+ x1 = self.transform(frame).unsqueeze(0).to(self.device)
+ y_hat = self.pspex(x1=x1, x2=x2, use_skip=self.pspex.opts.use_skip, zero_noise=True, resize=False)
+ y_hat = torch.clamp(y_hat, -1, 1)
+ videoWriter.write(tensor2cv2(y_hat[0].cpu()))
+ if i < min(frame_num, 4):
+ viz_frames += [self.tensor2np(y_hat[0])]
+
+ videoWriter.release()
+
+ return viz_frames, 'output.mp4'
+
+
+ def process_inversion(self, input_image: str, optimize: str, input_latent: file-object, editing_options: str,
+ scale_factor: float, seed: int) -> tuple[np.ndarray, np.ndarray]:
+ #false_image = np.zeros((256,256,3), np.uint8)
+ #info = 'Error: no face detected! Please retry or change the photo.'
+
+ if input_image is None:
+ raise gr.Error('Error: fail to load empty file.')
+ #return false_image, false_image, 'Error: fail to load empty file.'
+ frame = cv2.imread(input_image)
+ if frame is None:
+ raise gr.Error('Error: fail to load the image.')
+ #return false_image, false_image, 'Error: fail to load the image.'
+ frame = cv2.cvtColor(frame, cv2.COLOR_BGR2RGB)
+
+ task_name = 'inversion'
+ self.load_model(task_name)
+ if self.print_log: print('model %s loaded'%(task_name))
+ if input_latent is not None:
+ if '.pt' not in input_latent.name:
+ raise gr.Error('Error: the latent format is wrong')
+ #return false_image, false_image, 'Error: the latent format is wrong'
+ latents = torch.load(input_latent.name)
+ if 'wplus' not in latents.keys() or 'f' not in latents.keys():
+ raise gr.Error('Error: the latent format is wrong')
+ #return false_image, false_image, 'Error: the latent format is wrong'
+ wplus = latents['wplus'].to(self.device) # w+
+ f = [latents['f'][0].to(self.device)] # f
+ elif optimize == 'Latent optimization':
+ wplus, f, _, _, _ = latent_optimization(frame, self.pspex, self.landmarkpredictor,
+ step=500, device=self.device)
+ else:
+ with torch.no_grad():
+ paras = get_video_crop_parameter(frame, self.landmarkpredictor)
+ if paras is None:
+ raise gr.Error(self.error_info)
+ #return false_image, false_image, info
+ h,w,top,bottom,left,right,scale = paras
+ H, W = int(bottom-top), int(right-left)
+ frame = cv2.resize(frame, (w, h))[top:bottom, left:right]
+ x1 = self.transform(frame).unsqueeze(0).to(self.device)
+ x2 = align_face(frame, self.landmarkpredictor)
+ if x2 is None:
+ raise gr.Error(self.error_info)
+ #return false_image, false_image, 'Error: no face detected! Please retry or change the photo.'
+ x2 = self.transform(x2).unsqueeze(dim=0).to(self.device)
+ if self.print_log: print('image loaded')
+ wplus = self.pspex.encoder(x2) + self.pspex.latent_avg.unsqueeze(0)
+ _, f = self.pspex.encoder(x1, return_feat=True)
+
+ with torch.no_grad():
+ y_hat, _ = self.pspex.decoder([wplus], input_is_latent=True, first_layer_feature=f)
+ y_hat = torch.clamp(y_hat, -1, 1)
+
+ if 'Style Mixing' in editing_options:
+ torch.manual_seed(seed)
+ wplus[:, 8:] = self.pspex.decoder.style(torch.randn(1, 512).to(self.device)).unsqueeze(1).repeat(1,10,1) * 0.7
+ y_hat_edit, _ = self.pspex.decoder([wplus], input_is_latent=True, first_layer_feature=f)
+ elif 'Attribute Editing' in editing_options:
+ editing_w = self.editing_dicts[editing_options[19:]].to(self.device)
+ y_hat_edit, _ = self.pspex.decoder([wplus+scale_factor*editing_w], input_is_latent=True, first_layer_feature=f)
+ elif 'Domain Transfer' in editing_options:
+ self.load_G_model(editing_options[17:])
+ if self.print_log: print('model %s loaded'%(editing_options[17:]))
+ y_hat_edit, _ = self.generator([wplus], input_is_latent=True, first_layer_feature=f)
+ else:
+ y_hat_edit = y_hat
+ y_hat_edit = torch.clamp(y_hat_edit, -1, 1)
+
+ return self.tensor2np(y_hat[0]), self.tensor2np(y_hat_edit[0])
\ No newline at end of file