---
language:
- en
license: apache-2.0
tags:
- sentence-transformers
- sentence-similarity
- feature-extraction
- generated_from_trainer
- dataset_size:80543469
- loss:MatryoshkaLoss
- loss:MultipleNegativesRankingLoss
widget:
- source_sentence: What was the Office of Foods in charge of?
sentences:
- This area, stretching northward from the centrally located Great Hall of State,
is believed to have been the site of the Office of Foods. This office stocked
foods other than the rice that was paid as tax, and was in charge of providing
meals for state banquets and rituals held in the palace.
- In 2002, Barclay Records, then as part of Universal Music France, released a digitally
remastered version of the original vinyl in CD and in 10" (25 cm) vinyl record
(LP), under the same name, as part of a compilation containing re-releases of
all of Dalida's studio albums recorded under the Barclay label. The album was
again re-released in 2005.
- Kevin Jon Davies is a British television and video director primarily associated
with documentaries and spin-off videos associated with "Doctor Who", "The Hitchhiker's
Guide to the Galaxy" and "Blake's 7". He also worked on the BAFTA award-winning
animation sequences of the 1981 "Hitchhiker's Guide" television adaptation.
- source_sentence: jak wysłać sf do krs?
sentences:
- '[''Kliknij na pole „Przygotowanie i składanie zgłoszeń”'', ''Następnie kliknij
niebieski przycisk Dodaj zgłoszenie i uzupełnij o numer KRS Twojej jednostki na
stronie „Rejestracja nowego zgłoszenia – Krok 1”'']'
- When you experience a trigger, the insides of your airways swell even more. This
narrows the space for air to move in and out of the lungs. The muscles that wrap
around your airways also can tighten, making breathing even harder. When that
happens, it's called an asthma flare-up, asthma episode or asthma "attack."
- Lie (L) – The Lie scale is intended to identify individuals who are deliberately
trying to avoid answering the MMPI honestly and in a frank manner. The scale measures
attitudes and practices that are culturally laudable, but rarely found in most
people.
- source_sentence: 'Air Pollution in Eastern Asia: An Integrated Perspective: Chapter
14: Observation of Air Pollution over China Using the IASI Thermal Infrared Space
Sensor'
sentences:
- In this chapter we describe what is achievable in terms of pollutant tracking
from space using observations provided by thermal infrared remote sensors. After
a general introduction on infrared remote sensing, we exploit the data provided
by the Infrared Atmospheric Sounding Interferometer (IASI) missions onboard the
Metop series of satellite to illustrate pollution detection at various spatial
and temporal scales. Then, we focus on air pollution over China and discuss three
case studies involving different pollutants. The first example discusses the geophysical
conditions for detection of ammonia (NH3) and sulfur dioxide (SO2), both precursors
of particulate matter (PM). The second case illustrates the seasonal variation
of ozone (O3), in particular during the monsoon period. The third case shows the
local accumulation of enhanced levels of carbon monoxide (CO) when pollution episodes
occur.
- This article explores the political aspects of Islamic parties in Jombang, East
Java. The issue came about during the controversy arising out of the defection
of the leader of the Tarekat Qadiriyah Wa Naqsyabandiyah, an Islamic order, prior
to the 1977 general election. It raised questions as to the political orientation
of Islamic groups in Indonesia during the 1970s and 1980s.
- Abstract Unexpected findings on bone scintigraphy such as asymmetrical uptake
in extremities may cause confusion for the diagnosis. The authors describe three
cases of accidental intraarterial injection of Tc- 99m methylene diphosphonate
( 99m Tc-MDP) on the antecubital region and discuss the findings and differential
diagnosis.
- source_sentence: What type of stimuli do nociceptors response to?
sentences:
- 'After independence, Dutch was dropped as an official language and replaced by
Malay. Yet the Indonesian language inherited many words from Dutch: words for
everyday life as well as scientific and technological terms. One scholar argues
that 20% of Indonesian words can be traced back to Dutch words, many of which
are transliterated to reflect phonetic pronunciation e.g. kantoor (Dutch for "office")
in Indonesian is kantor, while bus ("bus") becomes bis. In addition, many Indonesian
words are calques on Dutch, for example, rumah sakit (Indonesian for "hospital")
is calqued on the Dutch ziekenhuis (literally "house of the sick"), kebun binatang
("zoo") on dierentuin (literally "animal garden"), undang-undang dasar ("constitution")
from grondwet (literally "ground law"). These account for some of the differences
in vocabulary between Indonesian and Malay.'
- Wilhelm Erb's (1874) "intensive" theory, that a pain signal can be generated by
intense enough stimulation of any sensory receptor, has been soundly disproved.
Some sensory fibers do not differentiate between noxious and non-noxious stimuli,
while others, nociceptors, respond only to noxious, high intensity stimuli. At
the peripheral end of the nociceptor, noxious stimuli generate currents that,
above a given threshold, begin to send signals along the nerve fiber to the spinal
cord. The "specificity" (whether it responds to thermal, chemical or mechanical
features of its environment) of a nociceptor is determined by which ion channels
it expresses at its peripheral end. Dozens of different types of nociceptor ion
channels have so far been identified, and their exact functions are still being
determined.
- The first attempt to establish a proper governing body and adopted the current
set of Rugby rules was the Foot Ball Association of Canada, organized on March
24, 1873 followed by the Canadian Rugby Football Union (CRFU) founded June 12,
1880, which included teams from Ontario and Quebec. Later both the Ontario and
Quebec Rugby Football Union (ORFU and QRFU) were formed (January 1883), and then
the Interprovincial (1907) and Western Interprovincial Football Union (1936) (IRFU
and WIFU). The CRFU reorganized into an umbrella organization forming the Canadian
Rugby Union (CRU) in 1891. The original forerunners to the current Canadian Football
League, was established in 1956 when the IRFU and WIFU formed an umbrella organization,
The Canadian Football Council (CFC). And then in 1958 the CFC left The CRFU to
become The CFL.
- source_sentence: 'Gadofosveset-enhanced MR angiography of carotid arteries: does
steady-state imaging improve accuracy of first-pass imaging?'
sentences:
- Prior studies have demonstrated improved clinical outcomes for surgeons with a
high-volume experience with certain open vascular operations. A high-volume experience
with carotid artery stenting (CAS) improves clinical outcomes. Moreover, it is
not known whether experience with other endovascular procedures, including percutaneous
coronary interventions (PCIs), is an adequate substitute for experience with CAS.
The goal of this study was to quantify the effect of increasing clinician volume
of CAS, endovascular aneurysm repair (EVAR), and thoracic endovascular aortic
aneurysm repair (TEVAR), and PCI on the outcomes for CAS.
- While sensitive to internal carotid artery (ICA) occlusion, carotid ultrasound
can produce false-positive results. CT angiography (CTA) has a high specificity
for ICA occlusion and is safer and cheaper than catheter angiography, although
less accurate. We determined the cost-effectiveness of CTA versus catheter angiography
for confirming an ICA occlusion first suggested by carotid ultrasound.
- Brachial artery FMD and GMD and carotid intima media thickness (cIMT) were studied
using ultrasound in 20 patients diagnosed with early RA in whom symptoms had been
present for less than 12 months, and in 20 control subjects matched for age, sex
and established cardiovascular risk factors. FMD and GMD were re-assessed after
12 months in RA patients and the change in each parameter was calculated. Data
were analysed by univariate regression.
- Compared with preoperative clinical and conventional MR data, (1)H MRS improved
the accuracy of MR imaging from 60.9% to 83%. We found (1)H MRS reliably distinguished
between abscess and high-grade tumour, and between high-grade glioma and low-grade
glioma, but was not able to reliably distinguish between recurrent glioma and
radiation necrosis. In 12/23 cases (52%) the (1)H MRS findings positively altered
our clinical management. Two representative cases are presented.
- Different in-plane resolutions have been used for carotid 3T MRI. We compared
the reproducibility, as well as the within- and between reader variability of
high and routinely used spatial resolution in scans of patients with atherosclerotic
carotid artery disease. Since no consensus exists about the optimal segmentation
method, we analysed all imaging data using two different segmentation methods.
- In the population-based Prospective Investigation of the Vasculature in Uppsala
Seniors (PIVUS) study (1016 subjects all aged 70), the prevalence of overt plaques
and echogenectity (grey scale median, GSM) of carotid artery plaques were recorded
by ultrasound in both of the carotid arteries. The thickness (IMT) and echogenicity
(IM-GSM) of the intima-media complex were also measured. Bisphenol A (BPA) and
10 phthalate metabolites were analyzed in serum by a API 4000 liquid chromatograph/tandem
mass spectrometer.
- In a longitudinal study we investigated in vivo alterations of CVO during neuroinflammation,
applying Gadofluorine M- (Gf) enhanced magnetic resonance imaging (MRI) in experimental
autoimmune encephalomyelitis, an animal model of multiple sclerosis. SJL/J mice
were monitored by Gadopentate dimeglumine- (Gd-DTPA) and Gf-enhanced MRI after
adoptive transfer of proteolipid-protein-specific T cells. Mean Gf intensity ratios
were calculated individually for different CVO and correlated to the clinical
disease course. Subsequently, the tissue distribution of fluorescence-labeled
Gf as well as the extent of cellular inflammation was assessed in corresponding
histological slices.
- Development of an improved MR sequence for examining the lung.
- Thirty-seven carotid artery disease patients participated in this study, of whom
24 underwent magnetic resonance imaging before and after CEA. Seventeen control
subjects spanning 5 decades underwent magnetic resonance imaging to assess age-related
changes. Hemodynamic metrics (that is, relative time to peak and amplitude) were
calculated with a γ-variate model. Linear regression was used to relate carotid
artery disease burden to downstream hemodynamics in the circle of Willis.
- Carotid artery stenting (CAS) is associated with a higher risk of both hemodynamic
depression and new ischemic brain lesions on diffusion-weighted imaging than carotid
endarterectomy (CEA). We assessed whether the occurrence of hemodynamic depression
is associated with these lesions in patients with symptomatic carotid stenosis
treated by CAS or CEA in the randomized International Carotid Stenting Study (ICSS)-MRI
substudy.
- Magnetic resonance imaging (MRI) guidance may improve the accuracy of Gleason
score (GS) determination by directing the biopsy to regions of interest (ROI)
that are likely to harbor high-grade prostate cancer (CaP). The aim of this study
was to determine the frequency and predictors of GS upgrading when a subsequent
MRI-guided biopsy is performed on patients with a diagnosis of GS 6 disease on
the basis of conventional, transrectal ultrasound-guided biopsy.
- Measures of carotid-femoral pulse wave velocity (cf-PWV) and carotid augmentation
index (cAI) may be affected by the presence of an abdominal aortic aneurysm (AAA).
We, therefore, investigated series of various measures of arterial stiffness and
wave reflections in patients with AAA, before and 4 weeks after endovascular aneurysm
repair (EVAR).
- High spatial resolution of dynamic contrast-enhanced (DCE) MR imaging allows characterization
of heterogenous tumor microenvironment. Our purpose was to determine which is
the best advanced MR imaging protocol, focused on additional MR perfusion method,
for predicting recurrent metastatic brain tumor following gamma-knife radiosurgery
(GKRS).
- To determine whether acromegalic patients have increased thyroidal vascularity
and blood flow on colour flow Doppler sonography (CFDS).
- To investigate whether an existing method for correction of phase offset errors
in phase-contrast velocity quantification is applicable for assessment of main
pulmonary artery flow with an MR scanner equipped with a high-power gradient system.
- Consecutive patients (n = 292) undergoing carotid endarterectomy for symptomatic
and asymptomatic carotid stenosis were included in the study. Mortality and cardiovascular
ischemic events were recorded during a median follow-up of 5.2 years. Baseline
plasma concentrations of adiponectin were measured. Cox regression models stratified
for gender were used for estimation of risk of events.
- To evaluate the diagnostic accuracy of gadofosveset-enhanced magnetic resonance
(MR) angiography in the assessment of carotid artery stenosis, with digital subtraction
angiography (DSA) as the reference standard, and to determine the value of reading
first-pass, steady-state, and "combined" (first-pass plus steady-state) MR angiograms.
- Phase-contrast Cardiovascular Magnetic Resonance Imaging (CMR) generally requires
the analysis of stationary tissue adjacent to a blood vessel to serve as a baseline
reference for zero velocity. However, for the heart and great vessels, there is
often no stationary tissue immediately adjacent to the vessel. Consequently, uncorrected
velocity offsets may introduce substantial errors in flow quantification. The
purpose of this study was to assess the magnitude of these flow errors and to
validate a clinically applicable method for their correction.
- This study was a post hoc analysis of a prospective cohort comprising 485 consecutive
patients undergoing carotid endarterectomy for high-grade ICAS. Patients were
classified by their clinical presentation, ie, asymptomatic (n = 213) or symptomatic
(within 6 months of surgery; n = 272, comprising both transient ischemic attack
[TIA; n = 163] and stroke [n = 109]). We investigated the association of cl-ICAS
with the primary outcome in adjusted regression models.
- Several studies reported on the moderate diagnostic yield of elective invasive
coronary angiography (ICA) regarding the presence of coronary artery disease (CAD),
but limited data are available on how prior testing for ischaemia may contribute
to improve the diagnostic yield in an every-day clinical setting. This study aimed
to assess the value and use of cardiac myocardial perfusion single photon emission
computed tomography (MPS) in patient selection prior to elective ICA.
- The feasibility of carotid stenting (CS) is no longer questionable, although its
indications remain debatable. Until the results of randomized trials are available,
personal series and registries should help in the comparison of long-term results
of CS with those of endarterectomy. We report here the long-term results of a
large series of CS in our department with a long follow-up. This retrospective
study reviews a single surgeon's 11-year experience with CS. Our results are compared
with those of conventional surgery emanating from our own series and the North
American Symptomatic Carotid Endarterectomy Trial (NASCET), European Carotid Surgery
Trial (ECST), and Asymptomatic Carotid Atherosclerosis Study (ACAS).
datasets:
- sentence-transformers/gooaq
- sentence-transformers/msmarco-co-condenser-margin-mse-sym-mnrl-mean-v1
- sentence-transformers/squad
- sentence-transformers/s2orc
- sentence-transformers/all-nli
- sentence-transformers/paq
- sentence-transformers/trivia-qa
- bclavie/msmarco-10m-triplets
- nthakur/swim-ir-monolingual
- sentence-transformers/pubmedqa
- sentence-transformers/miracl
- sentence-transformers/mldr
- sentence-transformers/mr-tydi
pipeline_tag: sentence-similarity
library_name: sentence-transformers
metrics:
- cosine_accuracy@1
- cosine_accuracy@3
- cosine_accuracy@5
- cosine_accuracy@10
- cosine_precision@1
- cosine_precision@3
- cosine_precision@5
- cosine_precision@10
- cosine_recall@1
- cosine_recall@3
- cosine_recall@5
- cosine_recall@10
- cosine_ndcg@10
- cosine_mrr@10
- cosine_map@100
co2_eq_emissions:
emissions: 1014.8766030829654
energy_consumed: 2.610937435575236
source: codecarbon
training_type: fine-tuning
on_cloud: false
cpu_model: 13th Gen Intel(R) Core(TM) i7-13700K
ram_total_size: 31.777088165283203
hours_used: 17.883
hardware_used: 1 x NVIDIA GeForce RTX 3090
model-index:
- name: Static Embeddings with BERT uncased tokenizer finetuned on various datasets
results:
- task:
type: information-retrieval
name: Information Retrieval
dataset:
name: NanoClimateFEVER
type: NanoClimateFEVER
metrics:
- type: cosine_accuracy@1
value: 0.32
name: Cosine Accuracy@1
- type: cosine_accuracy@3
value: 0.52
name: Cosine Accuracy@3
- type: cosine_accuracy@5
value: 0.6
name: Cosine Accuracy@5
- type: cosine_accuracy@10
value: 0.78
name: Cosine Accuracy@10
- type: cosine_precision@1
value: 0.32
name: Cosine Precision@1
- type: cosine_precision@3
value: 0.19333333333333333
name: Cosine Precision@3
- type: cosine_precision@5
value: 0.14
name: Cosine Precision@5
- type: cosine_precision@10
value: 0.10399999999999998
name: Cosine Precision@10
- type: cosine_recall@1
value: 0.14666666666666664
name: Cosine Recall@1
- type: cosine_recall@3
value: 0.239
name: Cosine Recall@3
- type: cosine_recall@5
value: 0.27899999999999997
name: Cosine Recall@5
- type: cosine_recall@10
value: 0.4196666666666667
name: Cosine Recall@10
- type: cosine_ndcg@10
value: 0.33085031011968163
name: Cosine Ndcg@10
- type: cosine_mrr@10
value: 0.44530158730158725
name: Cosine Mrr@10
- type: cosine_map@100
value: 0.259819611075427
name: Cosine Map@100
- task:
type: information-retrieval
name: Information Retrieval
dataset:
name: NanoDBPedia
type: NanoDBPedia
metrics:
- type: cosine_accuracy@1
value: 0.7
name: Cosine Accuracy@1
- type: cosine_accuracy@3
value: 0.84
name: Cosine Accuracy@3
- type: cosine_accuracy@5
value: 0.9
name: Cosine Accuracy@5
- type: cosine_accuracy@10
value: 0.94
name: Cosine Accuracy@10
- type: cosine_precision@1
value: 0.7
name: Cosine Precision@1
- type: cosine_precision@3
value: 0.5866666666666666
name: Cosine Precision@3
- type: cosine_precision@5
value: 0.544
name: Cosine Precision@5
- type: cosine_precision@10
value: 0.45199999999999996
name: Cosine Precision@10
- type: cosine_recall@1
value: 0.0804732343549837
name: Cosine Recall@1
- type: cosine_recall@3
value: 0.16047472236902457
name: Cosine Recall@3
- type: cosine_recall@5
value: 0.21798474210348842
name: Cosine Recall@5
- type: cosine_recall@10
value: 0.31433571884014205
name: Cosine Recall@10
- type: cosine_ndcg@10
value: 0.5681388031303078
name: Cosine Ndcg@10
- type: cosine_mrr@10
value: 0.7853888888888889
name: Cosine Mrr@10
- type: cosine_map@100
value: 0.4334843491187922
name: Cosine Map@100
- task:
type: information-retrieval
name: Information Retrieval
dataset:
name: NanoFEVER
type: NanoFEVER
metrics:
- type: cosine_accuracy@1
value: 0.46
name: Cosine Accuracy@1
- type: cosine_accuracy@3
value: 0.8
name: Cosine Accuracy@3
- type: cosine_accuracy@5
value: 0.84
name: Cosine Accuracy@5
- type: cosine_accuracy@10
value: 0.94
name: Cosine Accuracy@10
- type: cosine_precision@1
value: 0.46
name: Cosine Precision@1
- type: cosine_precision@3
value: 0.26666666666666666
name: Cosine Precision@3
- type: cosine_precision@5
value: 0.18
name: Cosine Precision@5
- type: cosine_precision@10
value: 0.09999999999999998
name: Cosine Precision@10
- type: cosine_recall@1
value: 0.4366666666666667
name: Cosine Recall@1
- type: cosine_recall@3
value: 0.7466666666666667
name: Cosine Recall@3
- type: cosine_recall@5
value: 0.8033333333333332
name: Cosine Recall@5
- type: cosine_recall@10
value: 0.9033333333333333
name: Cosine Recall@10
- type: cosine_ndcg@10
value: 0.6921500788245725
name: Cosine Ndcg@10
- type: cosine_mrr@10
value: 0.639690476190476
name: Cosine Mrr@10
- type: cosine_map@100
value: 0.62054338159709
name: Cosine Map@100
- task:
type: information-retrieval
name: Information Retrieval
dataset:
name: NanoFiQA2018
type: NanoFiQA2018
metrics:
- type: cosine_accuracy@1
value: 0.28
name: Cosine Accuracy@1
- type: cosine_accuracy@3
value: 0.44
name: Cosine Accuracy@3
- type: cosine_accuracy@5
value: 0.54
name: Cosine Accuracy@5
- type: cosine_accuracy@10
value: 0.64
name: Cosine Accuracy@10
- type: cosine_precision@1
value: 0.28
name: Cosine Precision@1
- type: cosine_precision@3
value: 0.19333333333333333
name: Cosine Precision@3
- type: cosine_precision@5
value: 0.16
name: Cosine Precision@5
- type: cosine_precision@10
value: 0.10399999999999998
name: Cosine Precision@10
- type: cosine_recall@1
value: 0.15188888888888888
name: Cosine Recall@1
- type: cosine_recall@3
value: 0.29826984126984124
name: Cosine Recall@3
- type: cosine_recall@5
value: 0.3792936507936508
name: Cosine Recall@5
- type: cosine_recall@10
value: 0.4837936507936508
name: Cosine Recall@10
- type: cosine_ndcg@10
value: 0.3651145030243953
name: Cosine Ndcg@10
- type: cosine_mrr@10
value: 0.3914603174603174
name: Cosine Mrr@10
- type: cosine_map@100
value: 0.3023673541934707
name: Cosine Map@100
- task:
type: information-retrieval
name: Information Retrieval
dataset:
name: NanoHotpotQA
type: NanoHotpotQA
metrics:
- type: cosine_accuracy@1
value: 0.64
name: Cosine Accuracy@1
- type: cosine_accuracy@3
value: 0.82
name: Cosine Accuracy@3
- type: cosine_accuracy@5
value: 0.86
name: Cosine Accuracy@5
- type: cosine_accuracy@10
value: 0.96
name: Cosine Accuracy@10
- type: cosine_precision@1
value: 0.64
name: Cosine Precision@1
- type: cosine_precision@3
value: 0.3733333333333333
name: Cosine Precision@3
- type: cosine_precision@5
value: 0.26
name: Cosine Precision@5
- type: cosine_precision@10
value: 0.14799999999999996
name: Cosine Precision@10
- type: cosine_recall@1
value: 0.32
name: Cosine Recall@1
- type: cosine_recall@3
value: 0.56
name: Cosine Recall@3
- type: cosine_recall@5
value: 0.65
name: Cosine Recall@5
- type: cosine_recall@10
value: 0.74
name: Cosine Recall@10
- type: cosine_ndcg@10
value: 0.6547177705459605
name: Cosine Ndcg@10
- type: cosine_mrr@10
value: 0.7485238095238096
name: Cosine Mrr@10
- type: cosine_map@100
value: 0.5797919554359183
name: Cosine Map@100
- task:
type: information-retrieval
name: Information Retrieval
dataset:
name: NanoMSMARCO
type: NanoMSMARCO
metrics:
- type: cosine_accuracy@1
value: 0.18
name: Cosine Accuracy@1
- type: cosine_accuracy@3
value: 0.42
name: Cosine Accuracy@3
- type: cosine_accuracy@5
value: 0.5
name: Cosine Accuracy@5
- type: cosine_accuracy@10
value: 0.66
name: Cosine Accuracy@10
- type: cosine_precision@1
value: 0.18
name: Cosine Precision@1
- type: cosine_precision@3
value: 0.13999999999999999
name: Cosine Precision@3
- type: cosine_precision@5
value: 0.10000000000000002
name: Cosine Precision@5
- type: cosine_precision@10
value: 0.066
name: Cosine Precision@10
- type: cosine_recall@1
value: 0.18
name: Cosine Recall@1
- type: cosine_recall@3
value: 0.42
name: Cosine Recall@3
- type: cosine_recall@5
value: 0.5
name: Cosine Recall@5
- type: cosine_recall@10
value: 0.66
name: Cosine Recall@10
- type: cosine_ndcg@10
value: 0.4040678769319761
name: Cosine Ndcg@10
- type: cosine_mrr@10
value: 0.3244682539682539
name: Cosine Mrr@10
- type: cosine_map@100
value: 0.33886403445504565
name: Cosine Map@100
- task:
type: information-retrieval
name: Information Retrieval
dataset:
name: NanoNFCorpus
type: NanoNFCorpus
metrics:
- type: cosine_accuracy@1
value: 0.42
name: Cosine Accuracy@1
- type: cosine_accuracy@3
value: 0.56
name: Cosine Accuracy@3
- type: cosine_accuracy@5
value: 0.62
name: Cosine Accuracy@5
- type: cosine_accuracy@10
value: 0.72
name: Cosine Accuracy@10
- type: cosine_precision@1
value: 0.42
name: Cosine Precision@1
- type: cosine_precision@3
value: 0.3733333333333333
name: Cosine Precision@3
- type: cosine_precision@5
value: 0.32
name: Cosine Precision@5
- type: cosine_precision@10
value: 0.244
name: Cosine Precision@10
- type: cosine_recall@1
value: 0.04278202363094378
name: Cosine Recall@1
- type: cosine_recall@3
value: 0.09842444348194118
name: Cosine Recall@3
- type: cosine_recall@5
value: 0.11962677523904507
name: Cosine Recall@5
- type: cosine_recall@10
value: 0.1389182072247147
name: Cosine Recall@10
- type: cosine_ndcg@10
value: 0.3241949561078219
name: Cosine Ndcg@10
- type: cosine_mrr@10
value: 0.5040793650793652
name: Cosine Mrr@10
- type: cosine_map@100
value: 0.1448579573714899
name: Cosine Map@100
- task:
type: information-retrieval
name: Information Retrieval
dataset:
name: NanoNQ
type: NanoNQ
metrics:
- type: cosine_accuracy@1
value: 0.24
name: Cosine Accuracy@1
- type: cosine_accuracy@3
value: 0.44
name: Cosine Accuracy@3
- type: cosine_accuracy@5
value: 0.58
name: Cosine Accuracy@5
- type: cosine_accuracy@10
value: 0.7
name: Cosine Accuracy@10
- type: cosine_precision@1
value: 0.24
name: Cosine Precision@1
- type: cosine_precision@3
value: 0.14666666666666667
name: Cosine Precision@3
- type: cosine_precision@5
value: 0.124
name: Cosine Precision@5
- type: cosine_precision@10
value: 0.07600000000000001
name: Cosine Precision@10
- type: cosine_recall@1
value: 0.24
name: Cosine Recall@1
- type: cosine_recall@3
value: 0.43
name: Cosine Recall@3
- type: cosine_recall@5
value: 0.58
name: Cosine Recall@5
- type: cosine_recall@10
value: 0.69
name: Cosine Recall@10
- type: cosine_ndcg@10
value: 0.4533881733265689
name: Cosine Ndcg@10
- type: cosine_mrr@10
value: 0.3764047619047619
name: Cosine Mrr@10
- type: cosine_map@100
value: 0.3890107375543526
name: Cosine Map@100
- task:
type: information-retrieval
name: Information Retrieval
dataset:
name: NanoQuoraRetrieval
type: NanoQuoraRetrieval
metrics:
- type: cosine_accuracy@1
value: 0.8
name: Cosine Accuracy@1
- type: cosine_accuracy@3
value: 0.96
name: Cosine Accuracy@3
- type: cosine_accuracy@5
value: 1.0
name: Cosine Accuracy@5
- type: cosine_accuracy@10
value: 1.0
name: Cosine Accuracy@10
- type: cosine_precision@1
value: 0.8
name: Cosine Precision@1
- type: cosine_precision@3
value: 0.38666666666666655
name: Cosine Precision@3
- type: cosine_precision@5
value: 0.24799999999999997
name: Cosine Precision@5
- type: cosine_precision@10
value: 0.12999999999999998
name: Cosine Precision@10
- type: cosine_recall@1
value: 0.7106666666666667
name: Cosine Recall@1
- type: cosine_recall@3
value: 0.9253333333333333
name: Cosine Recall@3
- type: cosine_recall@5
value: 0.9626666666666668
name: Cosine Recall@5
- type: cosine_recall@10
value: 0.9793333333333334
name: Cosine Recall@10
- type: cosine_ndcg@10
value: 0.895097527564125
name: Cosine Ndcg@10
- type: cosine_mrr@10
value: 0.88
name: Cosine Mrr@10
- type: cosine_map@100
value: 0.8594406482406483
name: Cosine Map@100
- task:
type: information-retrieval
name: Information Retrieval
dataset:
name: NanoSCIDOCS
type: NanoSCIDOCS
metrics:
- type: cosine_accuracy@1
value: 0.28
name: Cosine Accuracy@1
- type: cosine_accuracy@3
value: 0.48
name: Cosine Accuracy@3
- type: cosine_accuracy@5
value: 0.54
name: Cosine Accuracy@5
- type: cosine_accuracy@10
value: 0.7
name: Cosine Accuracy@10
- type: cosine_precision@1
value: 0.28
name: Cosine Precision@1
- type: cosine_precision@3
value: 0.22666666666666666
name: Cosine Precision@3
- type: cosine_precision@5
value: 0.188
name: Cosine Precision@5
- type: cosine_precision@10
value: 0.14
name: Cosine Precision@10
- type: cosine_recall@1
value: 0.059666666666666666
name: Cosine Recall@1
- type: cosine_recall@3
value: 0.14166666666666666
name: Cosine Recall@3
- type: cosine_recall@5
value: 0.19466666666666668
name: Cosine Recall@5
- type: cosine_recall@10
value: 0.2886666666666667
name: Cosine Recall@10
- type: cosine_ndcg@10
value: 0.26425784158945775
name: Cosine Ndcg@10
- type: cosine_mrr@10
value: 0.39979365079365076
name: Cosine Mrr@10
- type: cosine_map@100
value: 0.20502449880105952
name: Cosine Map@100
- task:
type: information-retrieval
name: Information Retrieval
dataset:
name: NanoArguAna
type: NanoArguAna
metrics:
- type: cosine_accuracy@1
value: 0.1
name: Cosine Accuracy@1
- type: cosine_accuracy@3
value: 0.46
name: Cosine Accuracy@3
- type: cosine_accuracy@5
value: 0.56
name: Cosine Accuracy@5
- type: cosine_accuracy@10
value: 0.74
name: Cosine Accuracy@10
- type: cosine_precision@1
value: 0.1
name: Cosine Precision@1
- type: cosine_precision@3
value: 0.15333333333333332
name: Cosine Precision@3
- type: cosine_precision@5
value: 0.11200000000000003
name: Cosine Precision@5
- type: cosine_precision@10
value: 0.07400000000000001
name: Cosine Precision@10
- type: cosine_recall@1
value: 0.1
name: Cosine Recall@1
- type: cosine_recall@3
value: 0.46
name: Cosine Recall@3
- type: cosine_recall@5
value: 0.56
name: Cosine Recall@5
- type: cosine_recall@10
value: 0.74
name: Cosine Recall@10
- type: cosine_ndcg@10
value: 0.4077879341218404
name: Cosine Ndcg@10
- type: cosine_mrr@10
value: 0.3033888888888889
name: Cosine Mrr@10
- type: cosine_map@100
value: 0.31510434322531095
name: Cosine Map@100
- task:
type: information-retrieval
name: Information Retrieval
dataset:
name: NanoSciFact
type: NanoSciFact
metrics:
- type: cosine_accuracy@1
value: 0.52
name: Cosine Accuracy@1
- type: cosine_accuracy@3
value: 0.6
name: Cosine Accuracy@3
- type: cosine_accuracy@5
value: 0.62
name: Cosine Accuracy@5
- type: cosine_accuracy@10
value: 0.76
name: Cosine Accuracy@10
- type: cosine_precision@1
value: 0.52
name: Cosine Precision@1
- type: cosine_precision@3
value: 0.20666666666666667
name: Cosine Precision@3
- type: cosine_precision@5
value: 0.132
name: Cosine Precision@5
- type: cosine_precision@10
value: 0.08399999999999999
name: Cosine Precision@10
- type: cosine_recall@1
value: 0.485
name: Cosine Recall@1
- type: cosine_recall@3
value: 0.57
name: Cosine Recall@3
- type: cosine_recall@5
value: 0.595
name: Cosine Recall@5
- type: cosine_recall@10
value: 0.75
name: Cosine Recall@10
- type: cosine_ndcg@10
value: 0.6111476167014296
name: Cosine Ndcg@10
- type: cosine_mrr@10
value: 0.5836904761904762
name: Cosine Mrr@10
- type: cosine_map@100
value: 0.5683309026222819
name: Cosine Map@100
- task:
type: information-retrieval
name: Information Retrieval
dataset:
name: NanoTouche2020
type: NanoTouche2020
metrics:
- type: cosine_accuracy@1
value: 0.5714285714285714
name: Cosine Accuracy@1
- type: cosine_accuracy@3
value: 0.8979591836734694
name: Cosine Accuracy@3
- type: cosine_accuracy@5
value: 0.9795918367346939
name: Cosine Accuracy@5
- type: cosine_accuracy@10
value: 1.0
name: Cosine Accuracy@10
- type: cosine_precision@1
value: 0.5714285714285714
name: Cosine Precision@1
- type: cosine_precision@3
value: 0.6054421768707482
name: Cosine Precision@3
- type: cosine_precision@5
value: 0.6204081632653061
name: Cosine Precision@5
- type: cosine_precision@10
value: 0.5306122448979592
name: Cosine Precision@10
- type: cosine_recall@1
value: 0.03980518443040866
name: Cosine Recall@1
- type: cosine_recall@3
value: 0.12364050983083796
name: Cosine Recall@3
- type: cosine_recall@5
value: 0.20953289383493803
name: Cosine Recall@5
- type: cosine_recall@10
value: 0.33697859476017505
name: Cosine Recall@10
- type: cosine_ndcg@10
value: 0.5702638593808323
name: Cosine Ndcg@10
- type: cosine_mrr@10
value: 0.744047619047619
name: Cosine Mrr@10
- type: cosine_map@100
value: 0.4469881140237455
name: Cosine Map@100
- task:
type: nano-beir
name: Nano BEIR
dataset:
name: NanoBEIR mean
type: NanoBEIR_mean
metrics:
- type: cosine_accuracy@1
value: 0.4239560439560439
name: Cosine Accuracy@1
- type: cosine_accuracy@3
value: 0.6336891679748822
name: Cosine Accuracy@3
- type: cosine_accuracy@5
value: 0.7030455259026686
name: Cosine Accuracy@5
- type: cosine_accuracy@10
value: 0.8107692307692307
name: Cosine Accuracy@10
- type: cosine_precision@1
value: 0.4239560439560439
name: Cosine Precision@1
- type: cosine_precision@3
value: 0.2963160648874934
name: Cosine Precision@3
- type: cosine_precision@5
value: 0.24064678178963897
name: Cosine Precision@5
- type: cosine_precision@10
value: 0.17327786499215073
name: Cosine Precision@10
- type: cosine_recall@1
value: 0.2302781536901455
name: Cosine Recall@1
- type: cosine_recall@3
value: 0.3979597064321778
name: Cosine Recall@3
- type: cosine_recall@5
value: 0.4654695945105992
name: Cosine Recall@5
- type: cosine_recall@10
value: 0.5726943208937448
name: Cosine Recall@10
- type: cosine_ndcg@10
value: 0.503167480874536
name: Cosine Ndcg@10
- type: cosine_mrr@10
value: 0.5481721611721612
name: Cosine Mrr@10
- type: cosine_map@100
value: 0.420279068285741
name: Cosine Map@100
- dataset:
config: default
name: MTEB AILACasedocs (default)
revision: 4106e6bcc72e0698d714ea8b101355e3e238431a
split: test
type: mteb/AILA_casedocs
metrics:
- type: main_score
value: 25.342
- type: map_at_1
value: 7.2059999999999995
- type: map_at_10
value: 17.343
- type: map_at_100
value: 21.356
- type: map_at_1000
value: 21.719
- type: map_at_20
value: 18.765
- type: map_at_3
value: 12.395
- type: map_at_5
value: 14.796000000000001
- type: mrr_at_1
value: 20.0
- type: mrr_at_10
value: 29.019047619047615
- type: mrr_at_100
value: 30.079478514482233
- type: mrr_at_1000
value: 30.11575302428615
- type: mrr_at_20
value: 29.311976911976913
- type: mrr_at_3
value: 25.0
- type: mrr_at_5
value: 27.399999999999995
- type: ndcg_at_1
value: 20.0
- type: ndcg_at_10
value: 25.342
- type: ndcg_at_100
value: 39.728
- type: ndcg_at_1000
value: 42.605
- type: ndcg_at_20
value: 28.157
- type: ndcg_at_3
value: 21.041999999999998
- type: ndcg_at_5
value: 22.147
- type: precision_at_1
value: 20.0
- type: precision_at_10
value: 12.2
- type: precision_at_100
value: 3.38
- type: precision_at_1000
value: 0.38999999999999996
- type: precision_at_20
value: 8.3
- type: precision_at_3
value: 18.0
- type: precision_at_5
value: 16.0
- type: recall_at_1
value: 7.2059999999999995
- type: recall_at_10
value: 32.214
- type: recall_at_100
value: 85.658
- type: recall_at_1000
value: 100.0
- type: recall_at_20
value: 39.265
- type: recall_at_3
value: 16.335
- type: recall_at_5
value: 21.897
task:
type: Retrieval
- dataset:
config: default
name: MTEB AILAStatutes (default)
revision: ebfcd844eadd3d667efa3c57fc5c8c87f5c2867e
split: test
type: mteb/AILA_statutes
metrics:
- type: main_score
value: 22.184
- type: map_at_1
value: 5.167
- type: map_at_10
value: 12.325
- type: map_at_100
value: 19.326999999999998
- type: map_at_1000
value: 19.326999999999998
- type: map_at_20
value: 14.405999999999999
- type: map_at_3
value: 9.4
- type: map_at_5
value: 10.05
- type: mrr_at_1
value: 22.0
- type: mrr_at_10
value: 36.64682539682539
- type: mrr_at_100
value: 37.85209121304697
- type: mrr_at_1000
value: 37.85209121304697
- type: mrr_at_20
value: 37.4718241682638
- type: mrr_at_3
value: 32.666666666666664
- type: mrr_at_5
value: 33.46666666666667
- type: ndcg_at_1
value: 22.0
- type: ndcg_at_10
value: 22.184
- type: ndcg_at_100
value: 45.896
- type: ndcg_at_1000
value: 45.896
- type: ndcg_at_20
value: 27.881
- type: ndcg_at_3
value: 18.976000000000003
- type: ndcg_at_5
value: 16.728
- type: precision_at_1
value: 22.0
- type: precision_at_10
value: 10.8
- type: precision_at_100
value: 4.34
- type: precision_at_1000
value: 0.434
- type: precision_at_20
value: 8.6
- type: precision_at_3
value: 17.333000000000002
- type: precision_at_5
value: 12.0
- type: recall_at_1
value: 5.167
- type: recall_at_10
value: 26.133
- type: recall_at_100
value: 100.0
- type: recall_at_1000
value: 100.0
- type: recall_at_20
value: 40.5
- type: recall_at_3
value: 13.633000000000001
- type: recall_at_5
value: 15.533
task:
type: Retrieval
- dataset:
config: default
name: MTEB ARCChallenge (default)
revision: c481e0da3dcbbad8bce7721dea9085b74320a0a3
split: test
type: RAR-b/ARC-Challenge
metrics:
- type: main_score
value: 6.042
- type: map_at_1
value: 1.451
- type: map_at_10
value: 4.253
- type: map_at_100
value: 4.8340000000000005
- type: map_at_1000
value: 4.9430000000000005
- type: map_at_20
value: 4.475
- type: map_at_3
value: 3.2140000000000004
- type: map_at_5
value: 3.7600000000000002
- type: mrr_at_1
value: 1.4505119453924915
- type: mrr_at_10
value: 4.250196381169083
- type: mrr_at_100
value: 4.831355631222712
- type: mrr_at_1000
value: 4.940667606986945
- type: mrr_at_20
value: 4.472323277417513
- type: mrr_at_3
value: 3.213879408418658
- type: mrr_at_5
value: 3.742889647326509
- type: ndcg_at_1
value: 1.451
- type: ndcg_at_10
value: 6.042
- type: ndcg_at_100
value: 9.765
- type: ndcg_at_1000
value: 13.655000000000001
- type: ndcg_at_20
value: 6.914
- type: ndcg_at_3
value: 3.852
- type: ndcg_at_5
value: 4.836
- type: precision_at_1
value: 1.451
- type: precision_at_10
value: 1.1860000000000002
- type: precision_at_100
value: 0.313
- type: precision_at_1000
value: 0.064
- type: precision_at_20
value: 0.772
- type: precision_at_3
value: 1.9060000000000001
- type: precision_at_5
value: 1.6209999999999998
- type: recall_at_1
value: 1.451
- type: recall_at_10
value: 11.86
- type: recall_at_100
value: 31.313999999999997
- type: recall_at_1000
value: 64.078
- type: recall_at_20
value: 15.443999999999999
- type: recall_at_3
value: 5.717
- type: recall_at_5
value: 8.106
task:
type: Retrieval
- dataset:
config: default
name: MTEB AlphaNLI (default)
revision: 303f40ef3d50918d3dc43577d33f2f7344ad72c1
split: test
type: RAR-b/alphanli
metrics:
- type: main_score
value: 20.586
- type: map_at_1
value: 13.184999999999999
- type: map_at_10
value: 17.898
- type: map_at_100
value: 18.593
- type: map_at_1000
value: 18.679000000000002
- type: map_at_20
value: 18.238
- type: map_at_3
value: 16.362
- type: map_at_5
value: 17.217
- type: mrr_at_1
value: 13.185378590078328
- type: mrr_at_10
value: 17.89819822620082
- type: mrr_at_100
value: 18.5933695842888
- type: mrr_at_1000
value: 18.679218327500653
- type: mrr_at_20
value: 18.237717139340596
- type: mrr_at_3
value: 16.362053959965195
- type: mrr_at_5
value: 17.21714534377719
- type: ndcg_at_1
value: 13.184999999999999
- type: ndcg_at_10
value: 20.586
- type: ndcg_at_100
value: 24.571
- type: ndcg_at_1000
value: 27.161
- type: ndcg_at_20
value: 21.834
- type: ndcg_at_3
value: 17.375
- type: ndcg_at_5
value: 18.926000000000002
- type: precision_at_1
value: 13.184999999999999
- type: precision_at_10
value: 2.924
- type: precision_at_100
value: 0.49300000000000005
- type: precision_at_1000
value: 0.06999999999999999
- type: precision_at_20
value: 1.71
- type: precision_at_3
value: 6.7669999999999995
- type: precision_at_5
value: 4.817
- type: recall_at_1
value: 13.184999999999999
- type: recall_at_10
value: 29.243000000000002
- type: recall_at_100
value: 49.282
- type: recall_at_1000
value: 70.366
- type: recall_at_20
value: 34.204
- type: recall_at_3
value: 20.3
- type: recall_at_5
value: 24.086
task:
type: Retrieval
- dataset:
config: default
name: MTEB AppsRetrieval (default)
revision: f22508f96b7a36c2415181ed8bb76f76e04ae2d5
split: test
type: CoIR-Retrieval/apps
metrics:
- type: main_score
value: 4.557
- type: map_at_1
value: 2.895
- type: map_at_10
value: 3.91
- type: map_at_100
value: 4.294
- type: map_at_1000
value: 4.391
- type: map_at_20
value: 4.089
- type: map_at_3
value: 3.4750000000000005
- type: map_at_5
value: 3.7130000000000005
- type: mrr_at_1
value: 2.895086321381142
- type: mrr_at_10
value: 3.909515377642868
- type: mrr_at_100
value: 4.293672586421636
- type: mrr_at_1000
value: 4.390523922890202
- type: mrr_at_20
value: 4.08917821169434
- type: mrr_at_3
value: 3.474988933156263
- type: mrr_at_5
value: 3.712704736609119
- type: ndcg_at_1
value: 2.895
- type: ndcg_at_10
value: 4.557
- type: ndcg_at_100
value: 6.868
- type: ndcg_at_1000
value: 10.407
- type: ndcg_at_20
value: 5.219
- type: ndcg_at_3
value: 3.6609999999999996
- type: ndcg_at_5
value: 4.088
- type: precision_at_1
value: 2.895
- type: precision_at_10
value: 0.6669999999999999
- type: precision_at_100
value: 0.185
- type: precision_at_1000
value: 0.049
- type: precision_at_20
value: 0.46499999999999997
- type: precision_at_3
value: 1.399
- type: precision_at_5
value: 1.046
- type: recall_at_1
value: 2.895
- type: recall_at_10
value: 6.666999999999999
- type: recall_at_100
value: 18.539
- type: recall_at_1000
value: 48.579
- type: recall_at_20
value: 9.296
- type: recall_at_3
value: 4.197
- type: recall_at_5
value: 5.232
task:
type: Retrieval
- dataset:
config: default
name: MTEB ArguAna (default)
revision: c22ab2a51041ffd869aaddef7af8d8215647e41a
split: test
type: mteb/arguana
metrics:
- type: main_score
value: 44.41
- type: map_at_1
value: 21.479
- type: map_at_10
value: 35.995
- type: map_at_100
value: 37.258
- type: map_at_1000
value: 37.273
- type: map_at_20
value: 36.908
- type: map_at_3
value: 31.247000000000003
- type: map_at_5
value: 33.751
- type: mrr_at_1
value: 21.763869132290186
- type: mrr_at_10
value: 36.12420691368057
- type: mrr_at_100
value: 37.378777397870884
- type: mrr_at_1000
value: 37.39392969162474
- type: mrr_at_20
value: 37.03325723357897
- type: mrr_at_3
value: 31.33001422475101
- type: mrr_at_5
value: 33.91180654338541
- type: ndcg_at_1
value: 21.479
- type: ndcg_at_10
value: 44.41
- type: ndcg_at_100
value: 50.032
- type: ndcg_at_1000
value: 50.388
- type: ndcg_at_20
value: 47.642
- type: ndcg_at_3
value: 34.505
- type: ndcg_at_5
value: 39.031
- type: precision_at_1
value: 21.479
- type: precision_at_10
value: 7.148000000000001
- type: precision_at_100
value: 0.967
- type: precision_at_1000
value: 0.099
- type: precision_at_20
value: 4.202999999999999
- type: precision_at_3
value: 14.651
- type: precision_at_5
value: 10.996
- type: recall_at_1
value: 21.479
- type: recall_at_10
value: 71.479
- type: recall_at_100
value: 96.65700000000001
- type: recall_at_1000
value: 99.36
- type: recall_at_20
value: 84.068
- type: recall_at_3
value: 43.954
- type: recall_at_5
value: 54.979
task:
type: Retrieval
- dataset:
config: default
name: MTEB CQADupstackAndroidRetrieval (default)
revision: f46a197baaae43b4f621051089b82a364682dfeb
split: test
type: mteb/cqadupstack-android
metrics:
- type: main_score
value: 31.177
- type: map_at_1
value: 19.758
- type: map_at_10
value: 26.619999999999997
- type: map_at_100
value: 27.784
- type: map_at_1000
value: 27.937
- type: map_at_20
value: 27.206999999999997
- type: map_at_3
value: 24.245
- type: map_at_5
value: 25.713
- type: mrr_at_1
value: 24.892703862660944
- type: mrr_at_10
value: 31.704702863501144
- type: mrr_at_100
value: 32.608301500063966
- type: mrr_at_1000
value: 32.68725795268983
- type: mrr_at_20
value: 32.177359559981575
- type: mrr_at_3
value: 29.685264663805444
- type: mrr_at_5
value: 30.958512160228906
- type: ndcg_at_1
value: 24.893
- type: ndcg_at_10
value: 31.177
- type: ndcg_at_100
value: 36.546
- type: ndcg_at_1000
value: 39.706
- type: ndcg_at_20
value: 32.926
- type: ndcg_at_3
value: 27.58
- type: ndcg_at_5
value: 29.465000000000003
- type: precision_at_1
value: 24.893
- type: precision_at_10
value: 5.966
- type: precision_at_100
value: 1.079
- type: precision_at_1000
value: 0.166
- type: precision_at_20
value: 3.5909999999999997
- type: precision_at_3
value: 13.209000000000001
- type: precision_at_5
value: 9.728
- type: recall_at_1
value: 19.758
- type: recall_at_10
value: 39.397
- type: recall_at_100
value: 63.446999999999996
- type: recall_at_1000
value: 85.083
- type: recall_at_20
value: 45.846
- type: recall_at_3
value: 28.855999999999998
- type: recall_at_5
value: 34.165
task:
type: Retrieval
- dataset:
config: default
name: MTEB CQADupstackEnglishRetrieval (default)
revision: ad9991cb51e31e31e430383c75ffb2885547b5f0
split: test
type: mteb/cqadupstack-english
metrics:
- type: main_score
value: 25.901999999999997
- type: map_at_1
value: 16.730999999999998
- type: map_at_10
value: 22.24
- type: map_at_100
value: 23.168
- type: map_at_1000
value: 23.289
- type: map_at_20
value: 22.720000000000002
- type: map_at_3
value: 20.335
- type: map_at_5
value: 21.371000000000002
- type: mrr_at_1
value: 20.31847133757962
- type: mrr_at_10
value: 25.743908603781197
- type: mrr_at_100
value: 26.511937121538402
- type: mrr_at_1000
value: 26.58619222690668
- type: mrr_at_20
value: 26.15910380102564
- type: mrr_at_3
value: 23.77919320594478
- type: mrr_at_5
value: 24.846072186836484
- type: ndcg_at_1
value: 20.318
- type: ndcg_at_10
value: 25.901999999999997
- type: ndcg_at_100
value: 30.259999999999998
- type: ndcg_at_1000
value: 32.984
- type: ndcg_at_20
value: 27.47
- type: ndcg_at_3
value: 22.432
- type: ndcg_at_5
value: 23.999000000000002
- type: precision_at_1
value: 20.318
- type: precision_at_10
value: 4.707
- type: precision_at_100
value: 0.8580000000000001
- type: precision_at_1000
value: 0.134
- type: precision_at_20
value: 2.869
- type: precision_at_3
value: 10.552
- type: precision_at_5
value: 7.567
- type: recall_at_1
value: 16.730999999999998
- type: recall_at_10
value: 33.48
- type: recall_at_100
value: 52.245
- type: recall_at_1000
value: 70.634
- type: recall_at_20
value: 39.189
- type: recall_at_3
value: 23.805
- type: recall_at_5
value: 27.898
task:
type: Retrieval
- dataset:
config: default
name: MTEB CQADupstackGamingRetrieval (default)
revision: 4885aa143210c98657558c04aaf3dc47cfb54340
split: test
type: mteb/cqadupstack-gaming
metrics:
- type: main_score
value: 36.312
- type: map_at_1
value: 23.072
- type: map_at_10
value: 31.64
- type: map_at_100
value: 32.761
- type: map_at_1000
value: 32.862
- type: map_at_20
value: 32.24
- type: map_at_3
value: 28.921999999999997
- type: map_at_5
value: 30.603
- type: mrr_at_1
value: 26.70846394984326
- type: mrr_at_10
value: 34.47342886998059
- type: mrr_at_100
value: 35.406961114894706
- type: mrr_at_1000
value: 35.47747613970401
- type: mrr_at_20
value: 34.984787094473404
- type: mrr_at_3
value: 32.15256008359454
- type: mrr_at_5
value: 33.544409613375095
- type: ndcg_at_1
value: 26.708
- type: ndcg_at_10
value: 36.312
- type: ndcg_at_100
value: 41.748000000000005
- type: ndcg_at_1000
value: 44.206
- type: ndcg_at_20
value: 38.257000000000005
- type: ndcg_at_3
value: 31.439
- type: ndcg_at_5
value: 34.036
- type: precision_at_1
value: 26.708
- type: precision_at_10
value: 6.0440000000000005
- type: precision_at_100
value: 0.966
- type: precision_at_1000
value: 0.125
- type: precision_at_20
value: 3.549
- type: precision_at_3
value: 14.086000000000002
- type: precision_at_5
value: 10.169
- type: recall_at_1
value: 23.072
- type: recall_at_10
value: 47.687000000000005
- type: recall_at_100
value: 72.469
- type: recall_at_1000
value: 90.568
- type: recall_at_20
value: 54.861000000000004
- type: recall_at_3
value: 34.758
- type: recall_at_5
value: 41.052
task:
type: Retrieval
- dataset:
config: default
name: MTEB CQADupstackGisRetrieval (default)
revision: 5003b3064772da1887988e05400cf3806fe491f2
split: test
type: mteb/cqadupstack-gis
metrics:
- type: main_score
value: 19.756999999999998
- type: map_at_1
value: 12.546
- type: map_at_10
value: 17.009
- type: map_at_100
value: 17.758
- type: map_at_1000
value: 17.866
- type: map_at_20
value: 17.399
- type: map_at_3
value: 15.532000000000002
- type: map_at_5
value: 16.305
- type: mrr_at_1
value: 13.559322033898304
- type: mrr_at_10
value: 18.144067796610162
- type: mrr_at_100
value: 18.89867843656649
- type: mrr_at_1000
value: 18.995819754371045
- type: mrr_at_20
value: 18.54987150762981
- type: mrr_at_3
value: 16.666666666666664
- type: mrr_at_5
value: 17.43502824858757
- type: ndcg_at_1
value: 13.559
- type: ndcg_at_10
value: 19.756999999999998
- type: ndcg_at_100
value: 23.931
- type: ndcg_at_1000
value: 27.203
- type: ndcg_at_20
value: 21.173000000000002
- type: ndcg_at_3
value: 16.778000000000002
- type: ndcg_at_5
value: 18.104
- type: precision_at_1
value: 13.559
- type: precision_at_10
value: 3.141
- type: precision_at_100
value: 0.5539999999999999
- type: precision_at_1000
value: 0.08800000000000001
- type: precision_at_20
value: 1.8929999999999998
- type: precision_at_3
value: 7.156
- type: precision_at_5
value: 5.04
- type: recall_at_1
value: 12.546
- type: recall_at_10
value: 27.093
- type: recall_at_100
value: 47.325
- type: recall_at_1000
value: 72.965
- type: recall_at_20
value: 32.491
- type: recall_at_3
value: 19.122
- type: recall_at_5
value: 22.264999999999997
task:
type: Retrieval
- dataset:
config: default
name: MTEB CQADupstackMathematicaRetrieval (default)
revision: 90fceea13679c63fe563ded68f3b6f06e50061de
split: test
type: mteb/cqadupstack-mathematica
metrics:
- type: main_score
value: 12.806000000000001
- type: map_at_1
value: 5.881
- type: map_at_10
value: 9.803
- type: map_at_100
value: 10.717
- type: map_at_1000
value: 10.816
- type: map_at_20
value: 10.244
- type: map_at_3
value: 8.163
- type: map_at_5
value: 8.956
- type: mrr_at_1
value: 7.462686567164178
- type: mrr_at_10
value: 12.077805417357661
- type: mrr_at_100
value: 12.966483256051529
- type: mrr_at_1000
value: 13.047246067329347
- type: mrr_at_20
value: 12.525499289049966
- type: mrr_at_3
value: 10.261194029850747
- type: mrr_at_5
value: 11.113184079601991
- type: ndcg_at_1
value: 7.463
- type: ndcg_at_10
value: 12.806000000000001
- type: ndcg_at_100
value: 17.807000000000002
- type: ndcg_at_1000
value: 20.979999999999997
- type: ndcg_at_20
value: 14.350999999999999
- type: ndcg_at_3
value: 9.468
- type: ndcg_at_5
value: 10.776
- type: precision_at_1
value: 7.463
- type: precision_at_10
value: 2.637
- type: precision_at_100
value: 0.613
- type: precision_at_1000
value: 0.101
- type: precision_at_20
value: 1.7229999999999999
- type: precision_at_3
value: 4.643
- type: precision_at_5
value: 3.6319999999999997
- type: recall_at_1
value: 5.881
- type: recall_at_10
value: 20.013
- type: recall_at_100
value: 42.92
- type: recall_at_1000
value: 66.943
- type: recall_at_20
value: 25.621
- type: recall_at_3
value: 10.768
- type: recall_at_5
value: 14.007
task:
type: Retrieval
- dataset:
config: default
name: MTEB CQADupstackPhysicsRetrieval (default)
revision: 79531abbd1fb92d06c6d6315a0cbbbf5bb247ea4
split: test
type: mteb/cqadupstack-physics
metrics:
- type: main_score
value: 28.765
- type: map_at_1
value: 17.519000000000002
- type: map_at_10
value: 24.136
- type: map_at_100
value: 25.352999999999998
- type: map_at_1000
value: 25.499
- type: map_at_20
value: 24.776999999999997
- type: map_at_3
value: 21.59
- type: map_at_5
value: 23.017000000000003
- type: mrr_at_1
value: 21.36669874879692
- type: mrr_at_10
value: 28.47930702598651
- type: mrr_at_100
value: 29.504734721457147
- type: mrr_at_1000
value: 29.58610606296599
- type: mrr_at_20
value: 29.06239382160277
- type: mrr_at_3
value: 25.85819698427977
- type: mrr_at_5
value: 27.523259544433753
- type: ndcg_at_1
value: 21.367
- type: ndcg_at_10
value: 28.765
- type: ndcg_at_100
value: 34.772999999999996
- type: ndcg_at_1000
value: 37.924
- type: ndcg_at_20
value: 30.891999999999996
- type: ndcg_at_3
value: 24.248
- type: ndcg_at_5
value: 26.479999999999997
- type: precision_at_1
value: 21.367
- type: precision_at_10
value: 5.351
- type: precision_at_100
value: 0.989
- type: precision_at_1000
value: 0.147
- type: precision_at_20
value: 3.325
- type: precision_at_3
value: 11.325000000000001
- type: precision_at_5
value: 8.469999999999999
- type: recall_at_1
value: 17.519000000000002
- type: recall_at_10
value: 38.602
- type: recall_at_100
value: 65.377
- type: recall_at_1000
value: 86.812
- type: recall_at_20
value: 46.161
- type: recall_at_3
value: 25.898
- type: recall_at_5
value: 31.654
task:
type: Retrieval
- dataset:
config: default
name: MTEB CQADupstackProgrammersRetrieval (default)
revision: 6184bc1440d2dbc7612be22b50686b8826d22b32
split: test
type: mteb/cqadupstack-programmers
metrics:
- type: main_score
value: 22.706
- type: map_at_1
value: 12.979
- type: map_at_10
value: 18.898
- type: map_at_100
value: 20.087
- type: map_at_1000
value: 20.223
- type: map_at_20
value: 19.516
- type: map_at_3
value: 16.955000000000002
- type: map_at_5
value: 18.043
- type: mrr_at_1
value: 15.639269406392694
- type: mrr_at_10
value: 22.00872472276581
- type: mrr_at_100
value: 23.052476310365996
- type: mrr_at_1000
value: 23.1408565851826
- type: mrr_at_20
value: 22.589418169993035
- type: mrr_at_3
value: 19.99619482496195
- type: mrr_at_5
value: 21.2062404870624
- type: ndcg_at_1
value: 15.639
- type: ndcg_at_10
value: 22.706
- type: ndcg_at_100
value: 28.477999999999998
- type: ndcg_at_1000
value: 31.756
- type: ndcg_at_20
value: 24.836
- type: ndcg_at_3
value: 19.049
- type: ndcg_at_5
value: 20.807000000000002
- type: precision_at_1
value: 15.639
- type: precision_at_10
value: 4.258
- type: precision_at_100
value: 0.865
- type: precision_at_1000
value: 0.133
- type: precision_at_20
value: 2.7969999999999997
- type: precision_at_3
value: 9.056000000000001
- type: precision_at_5
value: 6.758
- type: recall_at_1
value: 12.979
- type: recall_at_10
value: 31.16
- type: recall_at_100
value: 56.245
- type: recall_at_1000
value: 79.526
- type: recall_at_20
value: 38.696000000000005
- type: recall_at_3
value: 21.302
- type: recall_at_5
value: 25.615
task:
type: Retrieval
- dataset:
config: default
name: MTEB CQADupstackRetrieval (default)
revision: CQADupstackRetrieval_is_a_combined_dataset
split: test
type: CQADupstackRetrieval_is_a_combined_dataset
metrics:
- type: main_score
value: 21.956083333333336
- type: ndcg_at_10
value: 21.956083333333336
task:
type: Retrieval
- dataset:
config: default
name: MTEB CQADupstackStatsRetrieval (default)
revision: 65ac3a16b8e91f9cee4c9828cc7c335575432a2a
split: test
type: mteb/cqadupstack-stats
metrics:
- type: main_score
value: 17.395
- type: map_at_1
value: 10.088
- type: map_at_10
value: 14.539
- type: map_at_100
value: 15.362
- type: map_at_1000
value: 15.464
- type: map_at_20
value: 14.924000000000001
- type: map_at_3
value: 13.136999999999999
- type: map_at_5
value: 13.937
- type: mrr_at_1
value: 11.96319018404908
- type: mrr_at_10
value: 16.467767065926576
- type: mrr_at_100
value: 17.283614344094357
- type: mrr_at_1000
value: 17.375873381573232
- type: mrr_at_20
value: 16.854301079891787
- type: mrr_at_3
value: 15.08179959100204
- type: mrr_at_5
value: 15.848670756646216
- type: ndcg_at_1
value: 11.963
- type: ndcg_at_10
value: 17.395
- type: ndcg_at_100
value: 21.911
- type: ndcg_at_1000
value: 24.796000000000003
- type: ndcg_at_20
value: 18.773999999999997
- type: ndcg_at_3
value: 14.719
- type: ndcg_at_5
value: 16.032
- type: precision_at_1
value: 11.963
- type: precision_at_10
value: 2.96
- type: precision_at_100
value: 0.569
- type: precision_at_1000
value: 0.08800000000000001
- type: precision_at_20
value: 1.817
- type: precision_at_3
value: 6.748
- type: precision_at_5
value: 4.877
- type: recall_at_1
value: 10.088
- type: recall_at_10
value: 24.356
- type: recall_at_100
value: 45.73
- type: recall_at_1000
value: 67.577
- type: recall_at_20
value: 29.534
- type: recall_at_3
value: 16.944
- type: recall_at_5
value: 20.392
task:
type: Retrieval
- dataset:
config: default
name: MTEB CQADupstackTexRetrieval (default)
revision: 46989137a86843e03a6195de44b09deda022eec7
split: test
type: mteb/cqadupstack-tex
metrics:
- type: main_score
value: 12.592999999999998
- type: map_at_1
value: 7.1290000000000004
- type: map_at_10
value: 10.302
- type: map_at_100
value: 10.994
- type: map_at_1000
value: 11.118
- type: map_at_20
value: 10.653
- type: map_at_3
value: 9.361
- type: map_at_5
value: 9.803
- type: mrr_at_1
value: 8.843771507226428
- type: mrr_at_10
value: 12.421700040419921
- type: mrr_at_100
value: 13.14928923530448
- type: mrr_at_1000
value: 13.252448388769883
- type: mrr_at_20
value: 12.803691756524197
- type: mrr_at_3
value: 11.344345033264513
- type: mrr_at_5
value: 11.824386327139244
- type: ndcg_at_1
value: 8.844000000000001
- type: ndcg_at_10
value: 12.592999999999998
- type: ndcg_at_100
value: 16.409000000000002
- type: ndcg_at_1000
value: 19.906
- type: ndcg_at_20
value: 13.831
- type: ndcg_at_3
value: 10.7
- type: ndcg_at_5
value: 11.359
- type: precision_at_1
value: 8.844000000000001
- type: precision_at_10
value: 2.33
- type: precision_at_100
value: 0.506
- type: precision_at_1000
value: 0.096
- type: precision_at_20
value: 1.512
- type: precision_at_3
value: 5.116
- type: precision_at_5
value: 3.599
- type: recall_at_1
value: 7.1290000000000004
- type: recall_at_10
value: 17.549999999999997
- type: recall_at_100
value: 35.393
- type: recall_at_1000
value: 61.23800000000001
- type: recall_at_20
value: 22.124
- type: recall_at_3
value: 12.109
- type: recall_at_5
value: 13.832
task:
type: Retrieval
- dataset:
config: default
name: MTEB CQADupstackUnixRetrieval (default)
revision: 6c6430d3a6d36f8d2a829195bc5dc94d7e063e53
split: test
type: mteb/cqadupstack-unix
metrics:
- type: main_score
value: 19.111
- type: map_at_1
value: 12.577
- type: map_at_10
value: 16.275000000000002
- type: map_at_100
value: 17.083000000000002
- type: map_at_1000
value: 17.206
- type: map_at_20
value: 16.68
- type: map_at_3
value: 14.783
- type: map_at_5
value: 15.654000000000002
- type: mrr_at_1
value: 14.645522388059701
- type: mrr_at_10
value: 18.617404051172702
- type: mrr_at_100
value: 19.434952661619388
- type: mrr_at_1000
value: 19.536374825069274
- type: mrr_at_20
value: 19.039596975787966
- type: mrr_at_3
value: 16.977611940298516
- type: mrr_at_5
value: 17.938432835820894
- type: ndcg_at_1
value: 14.646
- type: ndcg_at_10
value: 19.111
- type: ndcg_at_100
value: 23.541999999999998
- type: ndcg_at_1000
value: 26.901999999999997
- type: ndcg_at_20
value: 20.593
- type: ndcg_at_3
value: 16.104
- type: ndcg_at_5
value: 17.577
- type: precision_at_1
value: 14.646
- type: precision_at_10
value: 3.237
- type: precision_at_100
value: 0.607
- type: precision_at_1000
value: 0.1
- type: precision_at_20
value: 1.9869999999999999
- type: precision_at_3
value: 7.027
- type: precision_at_5
value: 5.187
- type: recall_at_1
value: 12.577
- type: recall_at_10
value: 25.642
- type: recall_at_100
value: 46.296
- type: recall_at_1000
value: 70.901
- type: recall_at_20
value: 31.202
- type: recall_at_3
value: 17.396
- type: recall_at_5
value: 21.046
task:
type: Retrieval
- dataset:
config: default
name: MTEB CQADupstackWebmastersRetrieval (default)
revision: 160c094312a0e1facb97e55eeddb698c0abe3571
split: test
type: mteb/cqadupstack-webmasters
metrics:
- type: main_score
value: 22.359
- type: map_at_1
value: 14.202
- type: map_at_10
value: 18.528
- type: map_at_100
value: 19.649
- type: map_at_1000
value: 19.838
- type: map_at_20
value: 19.067
- type: map_at_3
value: 16.656000000000002
- type: map_at_5
value: 17.564
- type: mrr_at_1
value: 17.786561264822133
- type: mrr_at_10
value: 22.50015684798293
- type: mrr_at_100
value: 23.36103535586478
- type: mrr_at_1000
value: 23.447240155046412
- type: mrr_at_20
value: 22.897233666294404
- type: mrr_at_3
value: 20.685111989459813
- type: mrr_at_5
value: 21.58432147562582
- type: ndcg_at_1
value: 17.787
- type: ndcg_at_10
value: 22.359
- type: ndcg_at_100
value: 27.339999999999996
- type: ndcg_at_1000
value: 30.94
- type: ndcg_at_20
value: 23.915
- type: ndcg_at_3
value: 19.187
- type: ndcg_at_5
value: 20.415
- type: precision_at_1
value: 17.787
- type: precision_at_10
value: 4.348
- type: precision_at_100
value: 1.016
- type: precision_at_1000
value: 0.187
- type: precision_at_20
value: 2.826
- type: precision_at_3
value: 8.959
- type: precision_at_5
value: 6.601
- type: recall_at_1
value: 14.202
- type: recall_at_10
value: 29.507
- type: recall_at_100
value: 52.574
- type: recall_at_1000
value: 77.41799999999999
- type: recall_at_20
value: 35.733
- type: recall_at_3
value: 19.345000000000002
- type: recall_at_5
value: 22.99
task:
type: Retrieval
- dataset:
config: default
name: MTEB CQADupstackWordpressRetrieval (default)
revision: 4ffe81d471b1924886b33c7567bfb200e9eec5c4
split: test
type: mteb/cqadupstack-wordpress
metrics:
- type: main_score
value: 14.59
- type: map_at_1
value: 9.341000000000001
- type: map_at_10
value: 12.495000000000001
- type: map_at_100
value: 13.328000000000001
- type: map_at_1000
value: 13.443
- type: map_at_20
value: 12.919
- type: map_at_3
value: 11.448
- type: map_at_5
value: 12.016
- type: mrr_at_1
value: 10.536044362292053
- type: mrr_at_10
value: 13.933045799958926
- type: mrr_at_100
value: 14.753327128738034
- type: mrr_at_1000
value: 14.84798752653836
- type: mrr_at_20
value: 14.348175993628182
- type: mrr_at_3
value: 12.6309303758472
- type: mrr_at_5
value: 13.28712261244609
- type: ndcg_at_1
value: 10.536
- type: ndcg_at_10
value: 14.59
- type: ndcg_at_100
value: 19.322
- type: ndcg_at_1000
value: 22.735
- type: ndcg_at_20
value: 16.072
- type: ndcg_at_3
value: 12.36
- type: ndcg_at_5
value: 13.364999999999998
- type: precision_at_1
value: 10.536
- type: precision_at_10
value: 2.311
- type: precision_at_100
value: 0.508
- type: precision_at_1000
value: 0.086
- type: precision_at_20
value: 1.488
- type: precision_at_3
value: 5.176
- type: precision_at_5
value: 3.66
- type: recall_at_1
value: 9.341000000000001
- type: recall_at_10
value: 19.707
- type: recall_at_100
value: 42.89
- type: recall_at_1000
value: 69.447
- type: recall_at_20
value: 25.330000000000002
- type: recall_at_3
value: 13.814000000000002
- type: recall_at_5
value: 16.217000000000002
task:
type: Retrieval
- dataset:
config: default
name: MTEB ClimateFEVER (default)
revision: 47f2ac6acb640fc46020b02a5b59fdda04d39380
split: test
type: mteb/climate-fever
metrics:
- type: main_score
value: 20.433
- type: map_at_1
value: 7.469
- type: map_at_10
value: 13.536999999999999
- type: map_at_100
value: 15.222
- type: map_at_1000
value: 15.424
- type: map_at_20
value: 14.41
- type: map_at_3
value: 10.911999999999999
- type: map_at_5
value: 12.232
- type: mrr_at_1
value: 17.133550488599347
- type: mrr_at_10
value: 27.41355152267201
- type: mrr_at_100
value: 28.50611626391541
- type: mrr_at_1000
value: 28.568789326404005
- type: mrr_at_20
value: 28.08885051017031
- type: mrr_at_3
value: 23.724212812160687
- type: mrr_at_5
value: 25.8707926167209
- type: ndcg_at_1
value: 17.134
- type: ndcg_at_10
value: 20.433
- type: ndcg_at_100
value: 27.783
- type: ndcg_at_1000
value: 31.787
- type: ndcg_at_20
value: 23.108999999999998
- type: ndcg_at_3
value: 15.565999999999999
- type: ndcg_at_5
value: 17.354
- type: precision_at_1
value: 17.134
- type: precision_at_10
value: 6.866
- type: precision_at_100
value: 1.47
- type: precision_at_1000
value: 0.22100000000000003
- type: precision_at_20
value: 4.531000000000001
- type: precision_at_3
value: 11.965
- type: precision_at_5
value: 9.707
- type: recall_at_1
value: 7.469
- type: recall_at_10
value: 26.285999999999998
- type: recall_at_100
value: 52.376999999999995
- type: recall_at_1000
value: 75.261
- type: recall_at_20
value: 34.035
- type: recall_at_3
value: 14.526
- type: recall_at_5
value: 19.306
task:
type: Retrieval
- dataset:
config: default
name: MTEB ClimateFEVERHardNegatives (default)
revision: 3a309e201f3c2c4b13bd4a367a8f37eee2ec1d21
split: test
type: mteb/ClimateFEVER_test_top_250_only_w_correct-v2
metrics:
- type: main_score
value: 22.605
- type: map_at_1
value: 8.6
- type: map_at_10
value: 15.281
- type: map_at_100
value: 17.282
- type: map_at_1000
value: 17.522
- type: map_at_20
value: 16.339000000000002
- type: map_at_3
value: 12.429
- type: map_at_5
value: 13.922
- type: mrr_at_1
value: 18.5
- type: mrr_at_10
value: 29.625952380952388
- type: mrr_at_100
value: 30.77892068089231
- type: mrr_at_1000
value: 30.83853566495065
- type: mrr_at_20
value: 30.3662752048433
- type: mrr_at_3
value: 25.833333333333357
- type: mrr_at_5
value: 27.89333333333332
- type: ndcg_at_1
value: 18.5
- type: ndcg_at_10
value: 22.605
- type: ndcg_at_100
value: 31.097
- type: ndcg_at_1000
value: 35.576
- type: ndcg_at_20
value: 25.775
- type: ndcg_at_3
value: 17.43
- type: ndcg_at_5
value: 19.368
- type: precision_at_1
value: 18.5
- type: precision_at_10
value: 7.46
- type: precision_at_100
value: 1.6580000000000001
- type: precision_at_1000
value: 0.25
- type: precision_at_20
value: 5.06
- type: precision_at_3
value: 13.433
- type: precision_at_5
value: 10.74
- type: recall_at_1
value: 8.6
- type: recall_at_10
value: 28.882
- type: recall_at_100
value: 58.998
- type: recall_at_1000
value: 84.243
- type: recall_at_20
value: 37.957
- type: recall_at_3
value: 16.55
- type: recall_at_5
value: 21.648
task:
type: Retrieval
- dataset:
config: default
name: MTEB CodeFeedbackMT (default)
revision: b0f12fa0c0dd67f59c95a5c33d02aeeb4c398c5f
split: test
type: CoIR-Retrieval/codefeedback-mt
metrics:
- type: main_score
value: 46.045
- type: map_at_1
value: 38.412
- type: map_at_10
value: 43.41
- type: map_at_100
value: 43.976
- type: map_at_1000
value: 44.037
- type: map_at_20
value: 43.736000000000004
- type: map_at_3
value: 42.055
- type: map_at_5
value: 42.829
- type: mrr_at_1
value: 38.41229193341869
- type: mrr_at_10
value: 43.40960498582686
- type: mrr_at_100
value: 43.97561445897139
- type: mrr_at_1000
value: 44.03658359938551
- type: mrr_at_20
value: 43.73609592536922
- type: mrr_at_3
value: 42.05518314880356
- type: mrr_at_5
value: 42.828701262835196
- type: ndcg_at_1
value: 38.412
- type: ndcg_at_10
value: 46.045
- type: ndcg_at_100
value: 49.061
- type: ndcg_at_1000
value: 50.941
- type: ndcg_at_20
value: 47.245
- type: ndcg_at_3
value: 43.245
- type: ndcg_at_5
value: 44.639
- type: precision_at_1
value: 38.412
- type: precision_at_10
value: 5.442
- type: precision_at_100
value: 0.6910000000000001
- type: precision_at_1000
value: 0.08499999999999999
- type: precision_at_20
value: 2.959
- type: precision_at_3
value: 15.562999999999999
- type: precision_at_5
value: 10.014000000000001
- type: recall_at_1
value: 38.412
- type: recall_at_10
value: 54.417
- type: recall_at_100
value: 69.15
- type: recall_at_1000
value: 84.515
- type: recall_at_20
value: 59.185
- type: recall_at_3
value: 46.69
- type: recall_at_5
value: 50.072
task:
type: Retrieval
- dataset:
config: default
name: MTEB CodeFeedbackST (default)
revision: d213819e87aab9010628da8b73ab4eb337c89340
split: test
type: CoIR-Retrieval/codefeedback-st
metrics:
- type: main_score
value: 45.592
- type: map_at_1
value: 34.54
- type: map_at_10
value: 41.855
- type: map_at_100
value: 42.528
- type: map_at_1000
value: 42.587
- type: map_at_20
value: 42.239
- type: map_at_3
value: 39.985
- type: map_at_5
value: 41.075
- type: mrr_at_1
value: 34.42151664217722
- type: mrr_at_10
value: 41.78311069737728
- type: mrr_at_100
value: 42.45614518998771
- type: mrr_at_1000
value: 42.51517890438335
- type: mrr_at_20
value: 42.16798542795404
- type: mrr_at_3
value: 39.90715304840442
- type: mrr_at_5
value: 41.00055367448295
- type: ndcg_at_1
value: 34.54
- type: ndcg_at_10
value: 45.592
- type: ndcg_at_100
value: 49.13
- type: ndcg_at_1000
value: 50.885999999999996
- type: ndcg_at_20
value: 46.989999999999995
- type: ndcg_at_3
value: 41.754000000000005
- type: ndcg_at_5
value: 43.714999999999996
- type: precision_at_1
value: 34.54
- type: precision_at_10
value: 5.74
- type: precision_at_100
value: 0.746
- type: precision_at_1000
value: 0.089
- type: precision_at_20
value: 3.1460000000000004
- type: precision_at_3
value: 15.623999999999999
- type: precision_at_5
value: 10.325
- type: recall_at_1
value: 34.54
- type: recall_at_10
value: 57.403999999999996
- type: recall_at_100
value: 74.577
- type: recall_at_1000
value: 88.801
- type: recall_at_20
value: 62.927
- type: recall_at_3
value: 46.873
- type: recall_at_5
value: 51.623
task:
type: Retrieval
- dataset:
config: default
name: MTEB CosQA (default)
revision: bc5efb7e9d437246ce393ed19d772e08e4a79535
split: test
type: CoIR-Retrieval/cosqa
metrics:
- type: main_score
value: 7.939
- type: map_at_1
value: 3.2
- type: map_at_10
value: 6.1240000000000006
- type: map_at_100
value: 6.961
- type: map_at_1000
value: 7.124
- type: map_at_20
value: 6.494
- type: map_at_3
value: 5.033
- type: map_at_5
value: 5.623
- type: mrr_at_1
value: 3.2
- type: mrr_at_10
value: 5.470238095238093
- type: mrr_at_100
value: 6.320663781727482
- type: mrr_at_1000
value: 6.484552484927204
- type: mrr_at_20
value: 5.840692146690597
- type: mrr_at_3
value: 4.3999999999999995
- type: mrr_at_5
value: 4.919999999999999
- type: ndcg_at_1
value: 3.2
- type: ndcg_at_10
value: 7.939
- type: ndcg_at_100
value: 12.909
- type: ndcg_at_1000
value: 17.705000000000002
- type: ndcg_at_20
value: 9.266
- type: ndcg_at_3
value: 5.688
- type: ndcg_at_5
value: 6.755
- type: precision_at_1
value: 3.2
- type: precision_at_10
value: 1.38
- type: precision_at_100
value: 0.392
- type: precision_at_1000
value: 0.078
- type: precision_at_20
value: 0.95
- type: precision_at_3
value: 2.533
- type: precision_at_5
value: 2.04
- type: recall_at_1
value: 3.2
- type: recall_at_10
value: 13.8
- type: recall_at_100
value: 39.2
- type: recall_at_1000
value: 78.0
- type: recall_at_20
value: 19.0
- type: recall_at_3
value: 7.6
- type: recall_at_5
value: 10.2
task:
type: Retrieval
- dataset:
config: default
name: MTEB DBPedia (default)
revision: c0f706b76e590d620bd6618b3ca8efdd34e2d659
split: dev
type: mteb/dbpedia
metrics:
- type: main_score
value: 29.817
- type: map_at_1
value: 6.151
- type: map_at_10
value: 12.292
- type: map_at_100
value: 18.139
- type: map_at_1000
value: 19.84
- type: map_at_20
value: 14.495
- type: map_at_3
value: 8.426
- type: map_at_5
value: 10.192
- type: mrr_at_1
value: 46.26865671641791
- type: mrr_at_10
value: 57.92466240227434
- type: mrr_at_100
value: 58.67349319471301
- type: mrr_at_1000
value: 58.68212283999546
- type: mrr_at_20
value: 58.47241542478595
- type: mrr_at_3
value: 54.726368159203986
- type: mrr_at_5
value: 57.33830845771145
- type: ndcg_at_1
value: 38.06
- type: ndcg_at_10
value: 29.817
- type: ndcg_at_100
value: 36.472
- type: ndcg_at_1000
value: 45.576
- type: ndcg_at_20
value: 30.009000000000004
- type: ndcg_at_3
value: 32.839
- type: ndcg_at_5
value: 32.301
- type: precision_at_1
value: 46.269
- type: precision_at_10
value: 25.820999999999998
- type: precision_at_100
value: 8.552
- type: precision_at_1000
value: 1.576
- type: precision_at_20
value: 20.075000000000003
- type: precision_at_3
value: 35.821
- type: precision_at_5
value: 34.327999999999996
- type: recall_at_1
value: 6.151
- type: recall_at_10
value: 16.838
- type: recall_at_100
value: 48.427
- type: recall_at_1000
value: 77.018
- type: recall_at_20
value: 26.147
- type: recall_at_3
value: 9.221
- type: recall_at_5
value: 12.453
task:
type: Retrieval
- dataset:
config: default
name: MTEB DBPedia (default)
revision: c0f706b76e590d620bd6618b3ca8efdd34e2d659
split: test
type: mteb/dbpedia
metrics:
- type: main_score
value: 27.377000000000002
- type: map_at_1
value: 5.527
- type: map_at_10
value: 12.384
- type: map_at_100
value: 17.660999999999998
- type: map_at_1000
value: 18.98
- type: map_at_20
value: 14.424999999999999
- type: map_at_3
value: 8.484
- type: map_at_5
value: 10.174
- type: mrr_at_1
value: 44.25
- type: mrr_at_10
value: 55.620238095238086
- type: mrr_at_100
value: 56.311713506324445
- type: mrr_at_1000
value: 56.33739917164095
- type: mrr_at_20
value: 56.11873017655717
- type: mrr_at_3
value: 52.95833333333334
- type: mrr_at_5
value: 54.595833333333324
- type: ndcg_at_1
value: 31.75
- type: ndcg_at_10
value: 27.377000000000002
- type: ndcg_at_100
value: 32.164
- type: ndcg_at_1000
value: 40.050000000000004
- type: ndcg_at_20
value: 27.424
- type: ndcg_at_3
value: 28.683999999999997
- type: ndcg_at_5
value: 28.283
- type: precision_at_1
value: 44.25
- type: precision_at_10
value: 24.45
- type: precision_at_100
value: 7.704999999999999
- type: precision_at_1000
value: 1.5970000000000002
- type: precision_at_20
value: 18.462
- type: precision_at_3
value: 35.167
- type: precision_at_5
value: 30.95
- type: recall_at_1
value: 5.527
- type: recall_at_10
value: 18.016
- type: recall_at_100
value: 41.656
- type: recall_at_1000
value: 67.38300000000001
- type: recall_at_20
value: 24.21
- type: recall_at_3
value: 9.936
- type: recall_at_5
value: 13.187999999999999
task:
type: Retrieval
- dataset:
config: default
name: MTEB DBPediaHardNegatives (default)
revision: 943ec7fdfef3728b2ad1966c5b6479ff9ffd26c9
split: test
type: mteb/DBPedia_test_top_250_only_w_correct-v2
metrics:
- type: main_score
value: 30.444
- type: map_at_1
value: 5.722
- type: map_at_10
value: 13.688
- type: map_at_100
value: 22.032
- type: map_at_1000
value: 25.386999999999997
- type: map_at_20
value: 16.307
- type: map_at_3
value: 9.008
- type: map_at_5
value: 11.056000000000001
- type: mrr_at_1
value: 47.0
- type: mrr_at_10
value: 59.097420634920624
- type: mrr_at_100
value: 59.821938296328106
- type: mrr_at_1000
value: 59.83760663887243
- type: mrr_at_20
value: 59.59489258161859
- type: mrr_at_3
value: 56.458333333333336
- type: mrr_at_5
value: 58.18333333333333
- type: ndcg_at_1
value: 33.5
- type: ndcg_at_10
value: 30.444
- type: ndcg_at_100
value: 40.474
- type: ndcg_at_1000
value: 51.964
- type: ndcg_at_20
value: 31.356
- type: ndcg_at_3
value: 30.772
- type: ndcg_at_5
value: 30.576999999999998
- type: precision_at_1
value: 47.0
- type: precision_at_10
value: 27.975
- type: precision_at_100
value: 12.055
- type: precision_at_1000
value: 2.9579999999999997
- type: precision_at_20
value: 22.275
- type: precision_at_3
value: 38.083
- type: precision_at_5
value: 33.75
- type: recall_at_1
value: 5.722
- type: recall_at_10
value: 20.571
- type: recall_at_100
value: 55.967999999999996
- type: recall_at_1000
value: 91.362
- type: recall_at_20
value: 28.526
- type: recall_at_3
value: 10.761999999999999
- type: recall_at_5
value: 14.854999999999999
task:
type: Retrieval
- dataset:
config: default
name: MTEB FEVER (default)
revision: bea83ef9e8fb933d90a2f1d5515737465d613e12
split: test
type: mteb/fever
metrics:
- type: main_score
value: 42.997
- type: map_at_1
value: 22.017
- type: map_at_10
value: 35.199000000000005
- type: map_at_100
value: 36.254999999999995
- type: map_at_1000
value: 36.298
- type: map_at_20
value: 35.855
- type: map_at_3
value: 31.072
- type: map_at_5
value: 33.461
- type: mrr_at_1
value: 23.582358235823584
- type: mrr_at_10
value: 37.35784888012616
- type: mrr_at_100
value: 38.36344206815839
- type: mrr_at_1000
value: 38.39238175644681
- type: mrr_at_20
value: 38.01212529885376
- type: mrr_at_3
value: 33.098309830982956
- type: mrr_at_5
value: 35.579557955795615
- type: ndcg_at_1
value: 23.582
- type: ndcg_at_10
value: 42.997
- type: ndcg_at_100
value: 47.979
- type: ndcg_at_1000
value: 48.994
- type: ndcg_at_20
value: 45.35
- type: ndcg_at_3
value: 34.579
- type: ndcg_at_5
value: 38.851
- type: precision_at_1
value: 23.582
- type: precision_at_10
value: 7.1290000000000004
- type: precision_at_100
value: 0.9820000000000001
- type: precision_at_1000
value: 0.109
- type: precision_at_20
value: 4.0840000000000005
- type: precision_at_3
value: 15.342
- type: precision_at_5
value: 11.479000000000001
- type: recall_at_1
value: 22.017
- type: recall_at_10
value: 65.354
- type: recall_at_100
value: 87.75800000000001
- type: recall_at_1000
value: 95.212
- type: recall_at_20
value: 74.38
- type: recall_at_3
value: 42.581
- type: recall_at_5
value: 52.844
task:
type: Retrieval
- dataset:
config: default
name: MTEB FEVERHardNegatives (default)
revision: 080c9ed6267b65029207906e815d44a9240bafca
split: test
type: mteb/FEVER_test_top_250_only_w_correct-v2
metrics:
- type: main_score
value: 46.277
- type: map_at_1
value: 23.945
- type: map_at_10
value: 37.564
- type: map_at_100
value: 38.562000000000005
- type: map_at_1000
value: 38.602
- type: map_at_20
value: 38.173
- type: map_at_3
value: 32.208999999999996
- type: map_at_5
value: 35.538
- type: mrr_at_1
value: 25.5
- type: mrr_at_10
value: 39.60134920634916
- type: mrr_at_100
value: 40.523753358000675
- type: mrr_at_1000
value: 40.53762691263701
- type: mrr_at_20
value: 40.19828779622504
- type: mrr_at_3
value: 34.14999999999997
- type: mrr_at_5
value: 37.579999999999934
- type: ndcg_at_1
value: 25.5
- type: ndcg_at_10
value: 46.277
- type: ndcg_at_100
value: 51.07000000000001
- type: ndcg_at_1000
value: 51.783
- type: ndcg_at_20
value: 48.473
- type: ndcg_at_3
value: 35.497
- type: ndcg_at_5
value: 41.467
- type: precision_at_1
value: 25.5
- type: precision_at_10
value: 7.76
- type: precision_at_100
value: 1.036
- type: precision_at_1000
value: 0.11299999999999999
- type: precision_at_20
value: 4.3549999999999995
- type: precision_at_3
value: 15.367
- type: precision_at_5
value: 12.280000000000001
- type: recall_at_1
value: 23.945
- type: recall_at_10
value: 71.967
- type: recall_at_100
value: 93.765
- type: recall_at_1000
value: 98.47
- type: recall_at_20
value: 80.497
- type: recall_at_3
value: 43.033
- type: recall_at_5
value: 57.447
task:
type: Retrieval
- dataset:
config: default
name: MTEB FaithDial (default)
revision: 7a414e80725eac766f2602676dc8b39f80b061e4
split: test
type: McGill-NLP/FaithDial
metrics:
- type: main_score
value: 20.793
- type: map_at_1
value: 5.779
- type: map_at_10
value: 14.64
- type: map_at_100
value: 16.412
- type: map_at_1000
value: 16.478
- type: map_at_20
value: 15.638
- type: map_at_3
value: 10.545
- type: map_at_5
value: 12.82
- type: mrr_at_1
value: 5.093046033300686
- type: mrr_at_10
value: 14.511255693919727
- type: mrr_at_100
value: 16.266082070705043
- type: mrr_at_1000
value: 16.333152055297443
- type: mrr_at_20
value: 15.49481390088696
- type: mrr_at_3
value: 10.381978452497567
- type: mrr_at_5
value: 12.74975514201763
- type: ndcg_at_1
value: 5.779
- type: ndcg_at_10
value: 20.793
- type: ndcg_at_100
value: 30.137000000000004
- type: ndcg_at_1000
value: 31.706
- type: ndcg_at_20
value: 24.431
- type: ndcg_at_3
value: 12.264
- type: ndcg_at_5
value: 16.35
- type: precision_at_1
value: 5.779
- type: precision_at_10
value: 4.099
- type: precision_at_100
value: 0.8630000000000001
- type: precision_at_1000
value: 0.098
- type: precision_at_20
value: 2.769
- type: precision_at_3
value: 5.762
- type: precision_at_5
value: 5.436
- type: recall_at_1
value: 5.779
- type: recall_at_10
value: 40.989
- type: recall_at_100
value: 86.337
- type: recall_at_1000
value: 98.335
- type: recall_at_20
value: 55.387
- type: recall_at_3
value: 17.287
- type: recall_at_5
value: 27.179
task:
type: Retrieval
- dataset:
config: default
name: MTEB FeedbackQARetrieval (default)
revision: 1ee1cd0
split: test
type: lt2c/fqa
metrics:
- type: main_score
value: 27.159
- type: map_at_1
value: 27.159
- type: map_at_10
value: 36.533
- type: map_at_100
value: 37.653999999999996
- type: map_at_1000
value: 37.719
- type: map_at_20
value: 37.19
- type: map_at_3
value: 33.650999999999996
- type: map_at_5
value: 35.338
- type: mrr_at_1
value: 27.158634538152608
- type: mrr_at_10
value: 36.53293730477466
- type: mrr_at_100
value: 37.65359357224721
- type: mrr_at_1000
value: 37.71854110065475
- type: mrr_at_20
value: 37.18989930977979
- type: mrr_at_3
value: 33.65127175368139
- type: mrr_at_5
value: 35.33801874163323
- type: ndcg_at_1
value: 27.159
- type: ndcg_at_10
value: 41.756
- type: ndcg_at_100
value: 47.424
- type: ndcg_at_1000
value: 49.128
- type: ndcg_at_20
value: 44.111
- type: ndcg_at_3
value: 35.798
- type: ndcg_at_5
value: 38.827
- type: precision_at_1
value: 27.159
- type: precision_at_10
value: 5.848
- type: precision_at_100
value: 0.855
- type: precision_at_1000
value: 0.099
- type: precision_at_20
value: 3.386
- type: precision_at_3
value: 14.005999999999998
- type: precision_at_5
value: 9.869
- type: recall_at_1
value: 27.159
- type: recall_at_10
value: 58.484
- type: recall_at_100
value: 85.492
- type: recall_at_1000
value: 98.845
- type: recall_at_20
value: 67.721
- type: recall_at_3
value: 42.018
- type: recall_at_5
value: 49.347
task:
type: Retrieval
- dataset:
config: default
name: MTEB FiQA2018 (default)
revision: 27a168819829fe9bcd655c2df245fb19452e8e06
split: dev
type: mteb/fiqa
metrics:
- type: main_score
value: 19.159000000000002
- type: map_at_1
value: 9.577
- type: map_at_10
value: 14.629
- type: map_at_100
value: 15.926000000000002
- type: map_at_1000
value: 16.099
- type: map_at_20
value: 15.204
- type: map_at_3
value: 12.788
- type: map_at_5
value: 13.821
- type: mrr_at_1
value: 16.2
- type: mrr_at_10
value: 22.73920634920635
- type: mrr_at_100
value: 23.811442879807622
- type: mrr_at_1000
value: 23.904303078394555
- type: mrr_at_20
value: 23.37503016505339
- type: mrr_at_3
value: 20.733333333333327
- type: mrr_at_5
value: 21.873333333333328
- type: ndcg_at_1
value: 16.2
- type: ndcg_at_10
value: 19.159000000000002
- type: ndcg_at_100
value: 25.229000000000003
- type: ndcg_at_1000
value: 29.294999999999998
- type: ndcg_at_20
value: 21.109
- type: ndcg_at_3
value: 16.481
- type: ndcg_at_5
value: 17.488999999999997
- type: precision_at_1
value: 16.2
- type: precision_at_10
value: 5.04
- type: precision_at_100
value: 1.124
- type: precision_at_1000
value: 0.179
- type: precision_at_20
value: 3.34
- type: precision_at_3
value: 10.133000000000001
- type: precision_at_5
value: 7.76
- type: recall_at_1
value: 9.577
- type: recall_at_10
value: 24.362000000000002
- type: recall_at_100
value: 48.222
- type: recall_at_1000
value: 74.358
- type: recall_at_20
value: 30.465999999999998
- type: recall_at_3
value: 16.057
- type: recall_at_5
value: 19.516
task:
type: Retrieval
- dataset:
config: default
name: MTEB FiQA2018 (default)
revision: 27a168819829fe9bcd655c2df245fb19452e8e06
split: test
type: mteb/fiqa
metrics:
- type: main_score
value: 19.986
- type: map_at_1
value: 9.157
- type: map_at_10
value: 14.877
- type: map_at_100
value: 16.185
- type: map_at_1000
value: 16.366
- type: map_at_20
value: 15.551
- type: map_at_3
value: 12.574
- type: map_at_5
value: 13.694999999999999
- type: mrr_at_1
value: 16.51234567901235
- type: mrr_at_10
value: 23.66169410150891
- type: mrr_at_100
value: 24.6092001023614
- type: mrr_at_1000
value: 24.695544929151346
- type: mrr_at_20
value: 24.17998019538123
- type: mrr_at_3
value: 20.98765432098765
- type: mrr_at_5
value: 22.32253086419753
- type: ndcg_at_1
value: 16.512
- type: ndcg_at_10
value: 19.986
- type: ndcg_at_100
value: 25.840999999999998
- type: ndcg_at_1000
value: 29.999
- type: ndcg_at_20
value: 22.047
- type: ndcg_at_3
value: 16.401
- type: ndcg_at_5
value: 17.552
- type: precision_at_1
value: 16.512
- type: precision_at_10
value: 5.602
- type: precision_at_100
value: 1.171
- type: precision_at_1000
value: 0.19
- type: precision_at_20
value: 3.588
- type: precision_at_3
value: 10.545
- type: precision_at_5
value: 8.025
- type: recall_at_1
value: 9.157
- type: recall_at_10
value: 26.253999999999998
- type: recall_at_100
value: 48.175000000000004
- type: recall_at_1000
value: 74.236
- type: recall_at_20
value: 32.786
- type: recall_at_3
value: 15.631999999999998
- type: recall_at_5
value: 19.608
task:
type: Retrieval
- dataset:
config: default
name: MTEB FiQA2018 (default)
revision: 27a168819829fe9bcd655c2df245fb19452e8e06
split: train
type: mteb/fiqa
metrics:
- type: main_score
value: 17.858
- type: map_at_1
value: 8.012
- type: map_at_10
value: 13.209000000000001
- type: map_at_100
value: 14.477
- type: map_at_1000
value: 14.671000000000001
- type: map_at_20
value: 13.864
- type: map_at_3
value: 11.218
- type: map_at_5
value: 12.239
- type: mrr_at_1
value: 15.363636363636363
- type: mrr_at_10
value: 21.62342712842711
- type: mrr_at_100
value: 22.63936235019517
- type: mrr_at_1000
value: 22.735838806851703
- type: mrr_at_20
value: 22.175914005467874
- type: mrr_at_3
value: 19.448484848484895
- type: mrr_at_5
value: 20.589393939394007
- type: ndcg_at_1
value: 15.364
- type: ndcg_at_10
value: 17.858
- type: ndcg_at_100
value: 23.794999999999998
- type: ndcg_at_1000
value: 28.17
- type: ndcg_at_20
value: 19.901
- type: ndcg_at_3
value: 14.888000000000002
- type: ndcg_at_5
value: 15.926000000000002
- type: precision_at_1
value: 15.364
- type: precision_at_10
value: 5.024
- type: precision_at_100
value: 1.087
- type: precision_at_1000
value: 0.184
- type: precision_at_20
value: 3.293
- type: precision_at_3
value: 9.751999999999999
- type: precision_at_5
value: 7.465
- type: recall_at_1
value: 8.012
- type: recall_at_10
value: 23.233999999999998
- type: recall_at_100
value: 46.623999999999995
- type: recall_at_1000
value: 74.092
- type: recall_at_20
value: 29.854000000000003
- type: recall_at_3
value: 14.216000000000001
- type: recall_at_5
value: 17.713
task:
type: Retrieval
- dataset:
config: default
name: MTEB HellaSwag (default)
revision: a5c990205e017d10761197ccab3000936689c3ae
split: test
type: RAR-b/hellaswag
metrics:
- type: main_score
value: 16.377
- type: map_at_1
value: 8.474
- type: map_at_10
value: 13.479
- type: map_at_100
value: 14.296000000000001
- type: map_at_1000
value: 14.393
- type: map_at_20
value: 13.905000000000001
- type: map_at_3
value: 11.878
- type: map_at_5
value: 12.733
- type: mrr_at_1
value: 8.474407488548099
- type: mrr_at_10
value: 13.47926012335491
- type: mrr_at_100
value: 14.296018190032331
- type: mrr_at_1000
value: 14.39320635735857
- type: mrr_at_20
value: 13.905283977590932
- type: mrr_at_3
value: 11.878443869083188
- type: mrr_at_5
value: 12.733353249684685
- type: ndcg_at_1
value: 8.474
- type: ndcg_at_10
value: 16.377
- type: ndcg_at_100
value: 20.878
- type: ndcg_at_1000
value: 23.878
- type: ndcg_at_20
value: 17.93
- type: ndcg_at_3
value: 13.014999999999999
- type: ndcg_at_5
value: 14.557999999999998
- type: precision_at_1
value: 8.474
- type: precision_at_10
value: 2.571
- type: precision_at_100
value: 0.48
- type: precision_at_1000
value: 0.073
- type: precision_at_20
value: 1.593
- type: precision_at_3
value: 5.437
- type: precision_at_5
value: 4.013
- type: recall_at_1
value: 8.474
- type: recall_at_10
value: 25.712000000000003
- type: recall_at_100
value: 48.008
- type: recall_at_1000
value: 72.52499999999999
- type: recall_at_20
value: 31.856
- type: recall_at_3
value: 16.311
- type: recall_at_5
value: 20.066
task:
type: Retrieval
- dataset:
config: default
name: MTEB HotpotQA (default)
revision: ab518f4d6fcca38d87c25209f94beba119d02014
split: dev
type: mteb/hotpotqa
metrics:
- type: main_score
value: 49.524
- type: map_at_1
value: 27.583999999999996
- type: map_at_10
value: 40.455000000000005
- type: map_at_100
value: 41.567
- type: map_at_1000
value: 41.665
- type: map_at_20
value: 41.099000000000004
- type: map_at_3
value: 37.438
- type: map_at_5
value: 39.202999999999996
- type: mrr_at_1
value: 55.16798237561961
- type: mrr_at_10
value: 63.83496376336482
- type: mrr_at_100
value: 64.32844309604842
- type: mrr_at_1000
value: 64.35048997347738
- type: mrr_at_20
value: 64.14047945884145
- type: mrr_at_3
value: 61.93929380086919
- type: mrr_at_5
value: 63.0802888440121
- type: ndcg_at_1
value: 55.16799999999999
- type: ndcg_at_10
value: 49.524
- type: ndcg_at_100
value: 53.879
- type: ndcg_at_1000
value: 55.911
- type: ndcg_at_20
value: 51.31
- type: ndcg_at_3
value: 44.527
- type: ndcg_at_5
value: 47.102
- type: precision_at_1
value: 55.16799999999999
- type: precision_at_10
value: 10.718
- type: precision_at_100
value: 1.4160000000000001
- type: precision_at_1000
value: 0.169
- type: precision_at_20
value: 5.935
- type: precision_at_3
value: 28.26
- type: precision_at_5
value: 18.990000000000002
- type: recall_at_1
value: 27.583999999999996
- type: recall_at_10
value: 53.589
- type: recall_at_100
value: 70.782
- type: recall_at_1000
value: 84.276
- type: recall_at_20
value: 59.354
- type: recall_at_3
value: 42.39
- type: recall_at_5
value: 47.476
task:
type: Retrieval
- dataset:
config: default
name: MTEB HotpotQAHardNegatives (default)
revision: 617612fa63afcb60e3b134bed8b7216a99707c37
split: test
type: mteb/HotpotQA_test_top_250_only_w_correct-v2
metrics:
- type: main_score
value: 50.415
- type: map_at_1
value: 26.8
- type: map_at_10
value: 40.503
- type: map_at_100
value: 42.092
- type: map_at_1000
value: 42.198
- type: map_at_20
value: 41.394999999999996
- type: map_at_3
value: 36.75
- type: map_at_5
value: 38.945
- type: mrr_at_1
value: 53.6
- type: mrr_at_10
value: 63.90658730158732
- type: mrr_at_100
value: 64.46665914282829
- type: mrr_at_1000
value: 64.4775151418674
- type: mrr_at_20
value: 64.20681999545006
- type: mrr_at_3
value: 61.40000000000001
- type: mrr_at_5
value: 62.98000000000005
- type: ndcg_at_1
value: 53.6
- type: ndcg_at_10
value: 50.415
- type: ndcg_at_100
value: 56.48800000000001
- type: ndcg_at_1000
value: 58.388
- type: ndcg_at_20
value: 52.68000000000001
- type: ndcg_at_3
value: 44.165
- type: ndcg_at_5
value: 47.429
- type: precision_at_1
value: 53.6
- type: precision_at_10
value: 11.31
- type: precision_at_100
value: 1.614
- type: precision_at_1000
value: 0.186
- type: precision_at_20
value: 6.375
- type: precision_at_3
value: 28.433000000000003
- type: precision_at_5
value: 19.62
- type: recall_at_1
value: 26.8
- type: recall_at_10
value: 56.55
- type: recall_at_100
value: 80.7
- type: recall_at_1000
value: 93.05
- type: recall_at_20
value: 63.74999999999999
- type: recall_at_3
value: 42.65
- type: recall_at_5
value: 49.05
task:
type: Retrieval
- dataset:
config: default
name: MTEB LEMBNarrativeQARetrieval (default)
revision: 6e346642246bfb4928c560ee08640dc84d074e8c
split: test
type: dwzhu/LongEmbed
metrics:
- type: main_score
value: 47.11
- type: map_at_1
value: 38.176
- type: map_at_10
value: 44.11
- type: map_at_100
value: 44.885999999999996
- type: map_at_1000
value: 45.005
- type: map_at_20
value: 44.486
- type: map_at_3
value: 42.669000000000004
- type: map_at_5
value: 43.441
- type: mrr_at_1
value: 38.17590200019141
- type: mrr_at_10
value: 44.109989260003694
- type: mrr_at_100
value: 44.886475970293596
- type: mrr_at_1000
value: 45.00541901614199
- type: mrr_at_20
value: 44.48565175776022
- type: mrr_at_3
value: 42.66915494305687
- type: mrr_at_5
value: 43.44052062398311
- type: ndcg_at_1
value: 38.176
- type: ndcg_at_10
value: 47.11
- type: ndcg_at_100
value: 51.644999999999996
- type: ndcg_at_1000
value: 54.366
- type: ndcg_at_20
value: 48.475
- type: ndcg_at_3
value: 44.101
- type: ndcg_at_5
value: 45.494
- type: precision_at_1
value: 38.176
- type: precision_at_10
value: 5.6610000000000005
- type: precision_at_100
value: 0.796
- type: precision_at_1000
value: 0.1
- type: precision_at_20
value: 3.1
- type: precision_at_3
value: 16.078
- type: precision_at_5
value: 10.324
- type: recall_at_1
value: 38.176
- type: recall_at_10
value: 56.608000000000004
- type: recall_at_100
value: 79.644
- type: recall_at_1000
value: 100.0
- type: recall_at_20
value: 61.995999999999995
- type: recall_at_3
value: 48.234
- type: recall_at_5
value: 51.622
task:
type: Retrieval
- dataset:
config: default
name: MTEB LEMBNeedleRetrieval (default)
revision: 6e346642246bfb4928c560ee08640dc84d074e8c
split: test_1024
type: dwzhu/LongEmbed
metrics:
- type: main_score
value: 90.0
- type: map_at_1
value: 90.0
- type: map_at_10
value: 94.667
- type: map_at_100
value: 94.667
- type: map_at_1000
value: 94.667
- type: map_at_20
value: 94.667
- type: map_at_3
value: 94.667
- type: map_at_5
value: 94.667
- type: mrr_at_1
value: 90.0
- type: mrr_at_10
value: 94.66666666666666
- type: mrr_at_100
value: 94.66666666666666
- type: mrr_at_1000
value: 94.66666666666666
- type: mrr_at_20
value: 94.66666666666666
- type: mrr_at_3
value: 94.66666666666666
- type: mrr_at_5
value: 94.66666666666666
- type: ndcg_at_1
value: 90.0
- type: ndcg_at_10
value: 96.04700000000001
- type: ndcg_at_100
value: 96.04700000000001
- type: ndcg_at_1000
value: 96.04700000000001
- type: ndcg_at_20
value: 96.04700000000001
- type: ndcg_at_3
value: 96.04700000000001
- type: ndcg_at_5
value: 96.04700000000001
- type: precision_at_1
value: 90.0
- type: precision_at_10
value: 10.0
- type: precision_at_100
value: 1.0
- type: precision_at_1000
value: 0.1
- type: precision_at_20
value: 5.0
- type: precision_at_3
value: 33.333
- type: precision_at_5
value: 20.0
- type: recall_at_1
value: 90.0
- type: recall_at_10
value: 100.0
- type: recall_at_100
value: 100.0
- type: recall_at_1000
value: 100.0
- type: recall_at_20
value: 100.0
- type: recall_at_3
value: 100.0
- type: recall_at_5
value: 100.0
task:
type: Retrieval
- dataset:
config: default
name: MTEB LEMBNeedleRetrieval (default)
revision: 6e346642246bfb4928c560ee08640dc84d074e8c
split: test_16384
type: dwzhu/LongEmbed
metrics:
- type: main_score
value: 90.0
- type: map_at_1
value: 90.0
- type: map_at_10
value: 94.167
- type: map_at_100
value: 94.167
- type: map_at_1000
value: 94.167
- type: map_at_20
value: 94.167
- type: map_at_3
value: 93.667
- type: map_at_5
value: 94.167
- type: mrr_at_1
value: 90.0
- type: mrr_at_10
value: 94.16666666666666
- type: mrr_at_100
value: 94.16666666666666
- type: mrr_at_1000
value: 94.16666666666666
- type: mrr_at_20
value: 94.16666666666666
- type: mrr_at_3
value: 93.66666666666666
- type: mrr_at_5
value: 94.16666666666666
- type: ndcg_at_1
value: 90.0
- type: ndcg_at_10
value: 95.647
- type: ndcg_at_100
value: 95.647
- type: ndcg_at_1000
value: 95.647
- type: ndcg_at_20
value: 95.647
- type: ndcg_at_3
value: 94.786
- type: ndcg_at_5
value: 95.647
- type: precision_at_1
value: 90.0
- type: precision_at_10
value: 10.0
- type: precision_at_100
value: 1.0
- type: precision_at_1000
value: 0.1
- type: precision_at_20
value: 5.0
- type: precision_at_3
value: 32.667
- type: precision_at_5
value: 20.0
- type: recall_at_1
value: 90.0
- type: recall_at_10
value: 100.0
- type: recall_at_100
value: 100.0
- type: recall_at_1000
value: 100.0
- type: recall_at_20
value: 100.0
- type: recall_at_3
value: 98.0
- type: recall_at_5
value: 100.0
task:
type: Retrieval
- dataset:
config: default
name: MTEB LEMBNeedleRetrieval (default)
revision: 6e346642246bfb4928c560ee08640dc84d074e8c
split: test_2048
type: dwzhu/LongEmbed
metrics:
- type: main_score
value: 92.0
- type: map_at_1
value: 92.0
- type: map_at_10
value: 95.667
- type: map_at_100
value: 95.667
- type: map_at_1000
value: 95.667
- type: map_at_20
value: 95.667
- type: map_at_3
value: 95.667
- type: map_at_5
value: 95.667
- type: mrr_at_1
value: 92.0
- type: mrr_at_10
value: 95.66666666666666
- type: mrr_at_100
value: 95.66666666666666
- type: mrr_at_1000
value: 95.66666666666666
- type: mrr_at_20
value: 95.66666666666666
- type: mrr_at_3
value: 95.66666666666666
- type: mrr_at_5
value: 95.66666666666666
- type: ndcg_at_1
value: 92.0
- type: ndcg_at_10
value: 96.786
- type: ndcg_at_100
value: 96.786
- type: ndcg_at_1000
value: 96.786
- type: ndcg_at_20
value: 96.786
- type: ndcg_at_3
value: 96.786
- type: ndcg_at_5
value: 96.786
- type: precision_at_1
value: 92.0
- type: precision_at_10
value: 10.0
- type: precision_at_100
value: 1.0
- type: precision_at_1000
value: 0.1
- type: precision_at_20
value: 5.0
- type: precision_at_3
value: 33.333
- type: precision_at_5
value: 20.0
- type: recall_at_1
value: 92.0
- type: recall_at_10
value: 100.0
- type: recall_at_100
value: 100.0
- type: recall_at_1000
value: 100.0
- type: recall_at_20
value: 100.0
- type: recall_at_3
value: 100.0
- type: recall_at_5
value: 100.0
task:
type: Retrieval
- dataset:
config: default
name: MTEB LEMBNeedleRetrieval (default)
revision: 6e346642246bfb4928c560ee08640dc84d074e8c
split: test_256
type: dwzhu/LongEmbed
metrics:
- type: main_score
value: 90.0
- type: map_at_1
value: 90.0
- type: map_at_10
value: 95.0
- type: map_at_100
value: 95.0
- type: map_at_1000
value: 95.0
- type: map_at_20
value: 95.0
- type: map_at_3
value: 95.0
- type: map_at_5
value: 95.0
- type: mrr_at_1
value: 90.0
- type: mrr_at_10
value: 95.0
- type: mrr_at_100
value: 95.0
- type: mrr_at_1000
value: 95.0
- type: mrr_at_20
value: 95.0
- type: mrr_at_3
value: 95.0
- type: mrr_at_5
value: 95.0
- type: ndcg_at_1
value: 90.0
- type: ndcg_at_10
value: 96.309
- type: ndcg_at_100
value: 96.309
- type: ndcg_at_1000
value: 96.309
- type: ndcg_at_20
value: 96.309
- type: ndcg_at_3
value: 96.309
- type: ndcg_at_5
value: 96.309
- type: precision_at_1
value: 90.0
- type: precision_at_10
value: 10.0
- type: precision_at_100
value: 1.0
- type: precision_at_1000
value: 0.1
- type: precision_at_20
value: 5.0
- type: precision_at_3
value: 33.333
- type: precision_at_5
value: 20.0
- type: recall_at_1
value: 90.0
- type: recall_at_10
value: 100.0
- type: recall_at_100
value: 100.0
- type: recall_at_1000
value: 100.0
- type: recall_at_20
value: 100.0
- type: recall_at_3
value: 100.0
- type: recall_at_5
value: 100.0
task:
type: Retrieval
- dataset:
config: default
name: MTEB LEMBNeedleRetrieval (default)
revision: 6e346642246bfb4928c560ee08640dc84d074e8c
split: test_32768
type: dwzhu/LongEmbed
metrics:
- type: main_score
value: 84.0
- type: map_at_1
value: 84.0
- type: map_at_10
value: 91.167
- type: map_at_100
value: 91.167
- type: map_at_1000
value: 91.167
- type: map_at_20
value: 91.167
- type: map_at_3
value: 90.667
- type: map_at_5
value: 91.167
- type: mrr_at_1
value: 84.0
- type: mrr_at_10
value: 91.16666666666666
- type: mrr_at_100
value: 91.16666666666666
- type: mrr_at_1000
value: 91.16666666666666
- type: mrr_at_20
value: 91.16666666666666
- type: mrr_at_3
value: 90.66666666666666
- type: mrr_at_5
value: 91.16666666666666
- type: ndcg_at_1
value: 84.0
- type: ndcg_at_10
value: 93.43299999999999
- type: ndcg_at_100
value: 93.43299999999999
- type: ndcg_at_1000
value: 93.43299999999999
- type: ndcg_at_20
value: 93.43299999999999
- type: ndcg_at_3
value: 92.571
- type: ndcg_at_5
value: 93.43299999999999
- type: precision_at_1
value: 84.0
- type: precision_at_10
value: 10.0
- type: precision_at_100
value: 1.0
- type: precision_at_1000
value: 0.1
- type: precision_at_20
value: 5.0
- type: precision_at_3
value: 32.667
- type: precision_at_5
value: 20.0
- type: recall_at_1
value: 84.0
- type: recall_at_10
value: 100.0
- type: recall_at_100
value: 100.0
- type: recall_at_1000
value: 100.0
- type: recall_at_20
value: 100.0
- type: recall_at_3
value: 98.0
- type: recall_at_5
value: 100.0
task:
type: Retrieval
- dataset:
config: default
name: MTEB LEMBNeedleRetrieval (default)
revision: 6e346642246bfb4928c560ee08640dc84d074e8c
split: test_4096
type: dwzhu/LongEmbed
metrics:
- type: main_score
value: 92.0
- type: map_at_1
value: 92.0
- type: map_at_10
value: 96.0
- type: map_at_100
value: 96.0
- type: map_at_1000
value: 96.0
- type: map_at_20
value: 96.0
- type: map_at_3
value: 96.0
- type: map_at_5
value: 96.0
- type: mrr_at_1
value: 92.0
- type: mrr_at_10
value: 96.0
- type: mrr_at_100
value: 96.0
- type: mrr_at_1000
value: 96.0
- type: mrr_at_20
value: 96.0
- type: mrr_at_3
value: 96.0
- type: mrr_at_5
value: 96.0
- type: ndcg_at_1
value: 92.0
- type: ndcg_at_10
value: 97.04700000000001
- type: ndcg_at_100
value: 97.04700000000001
- type: ndcg_at_1000
value: 97.04700000000001
- type: ndcg_at_20
value: 97.04700000000001
- type: ndcg_at_3
value: 97.04700000000001
- type: ndcg_at_5
value: 97.04700000000001
- type: precision_at_1
value: 92.0
- type: precision_at_10
value: 10.0
- type: precision_at_100
value: 1.0
- type: precision_at_1000
value: 0.1
- type: precision_at_20
value: 5.0
- type: precision_at_3
value: 33.333
- type: precision_at_5
value: 20.0
- type: recall_at_1
value: 92.0
- type: recall_at_10
value: 100.0
- type: recall_at_100
value: 100.0
- type: recall_at_1000
value: 100.0
- type: recall_at_20
value: 100.0
- type: recall_at_3
value: 100.0
- type: recall_at_5
value: 100.0
task:
type: Retrieval
- dataset:
config: default
name: MTEB LEMBNeedleRetrieval (default)
revision: 6e346642246bfb4928c560ee08640dc84d074e8c
split: test_512
type: dwzhu/LongEmbed
metrics:
- type: main_score
value: 94.0
- type: map_at_1
value: 94.0
- type: map_at_10
value: 97.0
- type: map_at_100
value: 97.0
- type: map_at_1000
value: 97.0
- type: map_at_20
value: 97.0
- type: map_at_3
value: 97.0
- type: map_at_5
value: 97.0
- type: mrr_at_1
value: 94.0
- type: mrr_at_10
value: 97.0
- type: mrr_at_100
value: 97.0
- type: mrr_at_1000
value: 97.0
- type: mrr_at_20
value: 97.0
- type: mrr_at_3
value: 97.0
- type: mrr_at_5
value: 97.0
- type: ndcg_at_1
value: 94.0
- type: ndcg_at_10
value: 97.786
- type: ndcg_at_100
value: 97.786
- type: ndcg_at_1000
value: 97.786
- type: ndcg_at_20
value: 97.786
- type: ndcg_at_3
value: 97.786
- type: ndcg_at_5
value: 97.786
- type: precision_at_1
value: 94.0
- type: precision_at_10
value: 10.0
- type: precision_at_100
value: 1.0
- type: precision_at_1000
value: 0.1
- type: precision_at_20
value: 5.0
- type: precision_at_3
value: 33.333
- type: precision_at_5
value: 20.0
- type: recall_at_1
value: 94.0
- type: recall_at_10
value: 100.0
- type: recall_at_100
value: 100.0
- type: recall_at_1000
value: 100.0
- type: recall_at_20
value: 100.0
- type: recall_at_3
value: 100.0
- type: recall_at_5
value: 100.0
task:
type: Retrieval
- dataset:
config: default
name: MTEB LEMBNeedleRetrieval (default)
revision: 6e346642246bfb4928c560ee08640dc84d074e8c
split: test_8192
type: dwzhu/LongEmbed
metrics:
- type: main_score
value: 88.0
- type: map_at_1
value: 88.0
- type: map_at_10
value: 93.167
- type: map_at_100
value: 93.167
- type: map_at_1000
value: 93.167
- type: map_at_20
value: 93.167
- type: map_at_3
value: 92.667
- type: map_at_5
value: 93.167
- type: mrr_at_1
value: 88.0
- type: mrr_at_10
value: 93.16666666666666
- type: mrr_at_100
value: 93.16666666666666
- type: mrr_at_1000
value: 93.16666666666666
- type: mrr_at_20
value: 93.16666666666666
- type: mrr_at_3
value: 92.66666666666666
- type: mrr_at_5
value: 93.16666666666666
- type: ndcg_at_1
value: 88.0
- type: ndcg_at_10
value: 94.90899999999999
- type: ndcg_at_100
value: 94.90899999999999
- type: ndcg_at_1000
value: 94.90899999999999
- type: ndcg_at_20
value: 94.90899999999999
- type: ndcg_at_3
value: 94.047
- type: ndcg_at_5
value: 94.90899999999999
- type: precision_at_1
value: 88.0
- type: precision_at_10
value: 10.0
- type: precision_at_100
value: 1.0
- type: precision_at_1000
value: 0.1
- type: precision_at_20
value: 5.0
- type: precision_at_3
value: 32.667
- type: precision_at_5
value: 20.0
- type: recall_at_1
value: 88.0
- type: recall_at_10
value: 100.0
- type: recall_at_100
value: 100.0
- type: recall_at_1000
value: 100.0
- type: recall_at_20
value: 100.0
- type: recall_at_3
value: 98.0
- type: recall_at_5
value: 100.0
task:
type: Retrieval
- dataset:
config: default
name: MTEB LEMBPasskeyRetrieval (default)
revision: 6e346642246bfb4928c560ee08640dc84d074e8c
split: test_1024
type: dwzhu/LongEmbed
metrics:
- type: main_score
value: 100.0
- type: map_at_1
value: 100.0
- type: map_at_10
value: 100.0
- type: map_at_100
value: 100.0
- type: map_at_1000
value: 100.0
- type: map_at_20
value: 100.0
- type: map_at_3
value: 100.0
- type: map_at_5
value: 100.0
- type: mrr_at_1
value: 100.0
- type: mrr_at_10
value: 100.0
- type: mrr_at_100
value: 100.0
- type: mrr_at_1000
value: 100.0
- type: mrr_at_20
value: 100.0
- type: mrr_at_3
value: 100.0
- type: mrr_at_5
value: 100.0
- type: ndcg_at_1
value: 100.0
- type: ndcg_at_10
value: 100.0
- type: ndcg_at_100
value: 100.0
- type: ndcg_at_1000
value: 100.0
- type: ndcg_at_20
value: 100.0
- type: ndcg_at_3
value: 100.0
- type: ndcg_at_5
value: 100.0
- type: precision_at_1
value: 100.0
- type: precision_at_10
value: 10.0
- type: precision_at_100
value: 1.0
- type: precision_at_1000
value: 0.1
- type: precision_at_20
value: 5.0
- type: precision_at_3
value: 33.333
- type: precision_at_5
value: 20.0
- type: recall_at_1
value: 100.0
- type: recall_at_10
value: 100.0
- type: recall_at_100
value: 100.0
- type: recall_at_1000
value: 100.0
- type: recall_at_20
value: 100.0
- type: recall_at_3
value: 100.0
- type: recall_at_5
value: 100.0
task:
type: Retrieval
- dataset:
config: default
name: MTEB LEMBPasskeyRetrieval (default)
revision: 6e346642246bfb4928c560ee08640dc84d074e8c
split: test_16384
type: dwzhu/LongEmbed
metrics:
- type: main_score
value: 100.0
- type: map_at_1
value: 100.0
- type: map_at_10
value: 100.0
- type: map_at_100
value: 100.0
- type: map_at_1000
value: 100.0
- type: map_at_20
value: 100.0
- type: map_at_3
value: 100.0
- type: map_at_5
value: 100.0
- type: mrr_at_1
value: 100.0
- type: mrr_at_10
value: 100.0
- type: mrr_at_100
value: 100.0
- type: mrr_at_1000
value: 100.0
- type: mrr_at_20
value: 100.0
- type: mrr_at_3
value: 100.0
- type: mrr_at_5
value: 100.0
- type: ndcg_at_1
value: 100.0
- type: ndcg_at_10
value: 100.0
- type: ndcg_at_100
value: 100.0
- type: ndcg_at_1000
value: 100.0
- type: ndcg_at_20
value: 100.0
- type: ndcg_at_3
value: 100.0
- type: ndcg_at_5
value: 100.0
- type: precision_at_1
value: 100.0
- type: precision_at_10
value: 10.0
- type: precision_at_100
value: 1.0
- type: precision_at_1000
value: 0.1
- type: precision_at_20
value: 5.0
- type: precision_at_3
value: 33.333
- type: precision_at_5
value: 20.0
- type: recall_at_1
value: 100.0
- type: recall_at_10
value: 100.0
- type: recall_at_100
value: 100.0
- type: recall_at_1000
value: 100.0
- type: recall_at_20
value: 100.0
- type: recall_at_3
value: 100.0
- type: recall_at_5
value: 100.0
task:
type: Retrieval
- dataset:
config: default
name: MTEB LEMBPasskeyRetrieval (default)
revision: 6e346642246bfb4928c560ee08640dc84d074e8c
split: test_2048
type: dwzhu/LongEmbed
metrics:
- type: main_score
value: 100.0
- type: map_at_1
value: 100.0
- type: map_at_10
value: 100.0
- type: map_at_100
value: 100.0
- type: map_at_1000
value: 100.0
- type: map_at_20
value: 100.0
- type: map_at_3
value: 100.0
- type: map_at_5
value: 100.0
- type: mrr_at_1
value: 100.0
- type: mrr_at_10
value: 100.0
- type: mrr_at_100
value: 100.0
- type: mrr_at_1000
value: 100.0
- type: mrr_at_20
value: 100.0
- type: mrr_at_3
value: 100.0
- type: mrr_at_5
value: 100.0
- type: ndcg_at_1
value: 100.0
- type: ndcg_at_10
value: 100.0
- type: ndcg_at_100
value: 100.0
- type: ndcg_at_1000
value: 100.0
- type: ndcg_at_20
value: 100.0
- type: ndcg_at_3
value: 100.0
- type: ndcg_at_5
value: 100.0
- type: precision_at_1
value: 100.0
- type: precision_at_10
value: 10.0
- type: precision_at_100
value: 1.0
- type: precision_at_1000
value: 0.1
- type: precision_at_20
value: 5.0
- type: precision_at_3
value: 33.333
- type: precision_at_5
value: 20.0
- type: recall_at_1
value: 100.0
- type: recall_at_10
value: 100.0
- type: recall_at_100
value: 100.0
- type: recall_at_1000
value: 100.0
- type: recall_at_20
value: 100.0
- type: recall_at_3
value: 100.0
- type: recall_at_5
value: 100.0
task:
type: Retrieval
- dataset:
config: default
name: MTEB LEMBPasskeyRetrieval (default)
revision: 6e346642246bfb4928c560ee08640dc84d074e8c
split: test_256
type: dwzhu/LongEmbed
metrics:
- type: main_score
value: 100.0
- type: map_at_1
value: 100.0
- type: map_at_10
value: 100.0
- type: map_at_100
value: 100.0
- type: map_at_1000
value: 100.0
- type: map_at_20
value: 100.0
- type: map_at_3
value: 100.0
- type: map_at_5
value: 100.0
- type: mrr_at_1
value: 100.0
- type: mrr_at_10
value: 100.0
- type: mrr_at_100
value: 100.0
- type: mrr_at_1000
value: 100.0
- type: mrr_at_20
value: 100.0
- type: mrr_at_3
value: 100.0
- type: mrr_at_5
value: 100.0
- type: ndcg_at_1
value: 100.0
- type: ndcg_at_10
value: 100.0
- type: ndcg_at_100
value: 100.0
- type: ndcg_at_1000
value: 100.0
- type: ndcg_at_20
value: 100.0
- type: ndcg_at_3
value: 100.0
- type: ndcg_at_5
value: 100.0
- type: precision_at_1
value: 100.0
- type: precision_at_10
value: 10.0
- type: precision_at_100
value: 1.0
- type: precision_at_1000
value: 0.1
- type: precision_at_20
value: 5.0
- type: precision_at_3
value: 33.333
- type: precision_at_5
value: 20.0
- type: recall_at_1
value: 100.0
- type: recall_at_10
value: 100.0
- type: recall_at_100
value: 100.0
- type: recall_at_1000
value: 100.0
- type: recall_at_20
value: 100.0
- type: recall_at_3
value: 100.0
- type: recall_at_5
value: 100.0
task:
type: Retrieval
- dataset:
config: default
name: MTEB LEMBPasskeyRetrieval (default)
revision: 6e346642246bfb4928c560ee08640dc84d074e8c
split: test_32768
type: dwzhu/LongEmbed
metrics:
- type: main_score
value: 100.0
- type: map_at_1
value: 100.0
- type: map_at_10
value: 100.0
- type: map_at_100
value: 100.0
- type: map_at_1000
value: 100.0
- type: map_at_20
value: 100.0
- type: map_at_3
value: 100.0
- type: map_at_5
value: 100.0
- type: mrr_at_1
value: 100.0
- type: mrr_at_10
value: 100.0
- type: mrr_at_100
value: 100.0
- type: mrr_at_1000
value: 100.0
- type: mrr_at_20
value: 100.0
- type: mrr_at_3
value: 100.0
- type: mrr_at_5
value: 100.0
- type: ndcg_at_1
value: 100.0
- type: ndcg_at_10
value: 100.0
- type: ndcg_at_100
value: 100.0
- type: ndcg_at_1000
value: 100.0
- type: ndcg_at_20
value: 100.0
- type: ndcg_at_3
value: 100.0
- type: ndcg_at_5
value: 100.0
- type: precision_at_1
value: 100.0
- type: precision_at_10
value: 10.0
- type: precision_at_100
value: 1.0
- type: precision_at_1000
value: 0.1
- type: precision_at_20
value: 5.0
- type: precision_at_3
value: 33.333
- type: precision_at_5
value: 20.0
- type: recall_at_1
value: 100.0
- type: recall_at_10
value: 100.0
- type: recall_at_100
value: 100.0
- type: recall_at_1000
value: 100.0
- type: recall_at_20
value: 100.0
- type: recall_at_3
value: 100.0
- type: recall_at_5
value: 100.0
task:
type: Retrieval
- dataset:
config: default
name: MTEB LEMBPasskeyRetrieval (default)
revision: 6e346642246bfb4928c560ee08640dc84d074e8c
split: test_4096
type: dwzhu/LongEmbed
metrics:
- type: main_score
value: 100.0
- type: map_at_1
value: 100.0
- type: map_at_10
value: 100.0
- type: map_at_100
value: 100.0
- type: map_at_1000
value: 100.0
- type: map_at_20
value: 100.0
- type: map_at_3
value: 100.0
- type: map_at_5
value: 100.0
- type: mrr_at_1
value: 100.0
- type: mrr_at_10
value: 100.0
- type: mrr_at_100
value: 100.0
- type: mrr_at_1000
value: 100.0
- type: mrr_at_20
value: 100.0
- type: mrr_at_3
value: 100.0
- type: mrr_at_5
value: 100.0
- type: ndcg_at_1
value: 100.0
- type: ndcg_at_10
value: 100.0
- type: ndcg_at_100
value: 100.0
- type: ndcg_at_1000
value: 100.0
- type: ndcg_at_20
value: 100.0
- type: ndcg_at_3
value: 100.0
- type: ndcg_at_5
value: 100.0
- type: precision_at_1
value: 100.0
- type: precision_at_10
value: 10.0
- type: precision_at_100
value: 1.0
- type: precision_at_1000
value: 0.1
- type: precision_at_20
value: 5.0
- type: precision_at_3
value: 33.333
- type: precision_at_5
value: 20.0
- type: recall_at_1
value: 100.0
- type: recall_at_10
value: 100.0
- type: recall_at_100
value: 100.0
- type: recall_at_1000
value: 100.0
- type: recall_at_20
value: 100.0
- type: recall_at_3
value: 100.0
- type: recall_at_5
value: 100.0
task:
type: Retrieval
- dataset:
config: default
name: MTEB LEMBPasskeyRetrieval (default)
revision: 6e346642246bfb4928c560ee08640dc84d074e8c
split: test_512
type: dwzhu/LongEmbed
metrics:
- type: main_score
value: 100.0
- type: map_at_1
value: 100.0
- type: map_at_10
value: 100.0
- type: map_at_100
value: 100.0
- type: map_at_1000
value: 100.0
- type: map_at_20
value: 100.0
- type: map_at_3
value: 100.0
- type: map_at_5
value: 100.0
- type: mrr_at_1
value: 100.0
- type: mrr_at_10
value: 100.0
- type: mrr_at_100
value: 100.0
- type: mrr_at_1000
value: 100.0
- type: mrr_at_20
value: 100.0
- type: mrr_at_3
value: 100.0
- type: mrr_at_5
value: 100.0
- type: ndcg_at_1
value: 100.0
- type: ndcg_at_10
value: 100.0
- type: ndcg_at_100
value: 100.0
- type: ndcg_at_1000
value: 100.0
- type: ndcg_at_20
value: 100.0
- type: ndcg_at_3
value: 100.0
- type: ndcg_at_5
value: 100.0
- type: precision_at_1
value: 100.0
- type: precision_at_10
value: 10.0
- type: precision_at_100
value: 1.0
- type: precision_at_1000
value: 0.1
- type: precision_at_20
value: 5.0
- type: precision_at_3
value: 33.333
- type: precision_at_5
value: 20.0
- type: recall_at_1
value: 100.0
- type: recall_at_10
value: 100.0
- type: recall_at_100
value: 100.0
- type: recall_at_1000
value: 100.0
- type: recall_at_20
value: 100.0
- type: recall_at_3
value: 100.0
- type: recall_at_5
value: 100.0
task:
type: Retrieval
- dataset:
config: default
name: MTEB LEMBPasskeyRetrieval (default)
revision: 6e346642246bfb4928c560ee08640dc84d074e8c
split: test_8192
type: dwzhu/LongEmbed
metrics:
- type: main_score
value: 100.0
- type: map_at_1
value: 100.0
- type: map_at_10
value: 100.0
- type: map_at_100
value: 100.0
- type: map_at_1000
value: 100.0
- type: map_at_20
value: 100.0
- type: map_at_3
value: 100.0
- type: map_at_5
value: 100.0
- type: mrr_at_1
value: 100.0
- type: mrr_at_10
value: 100.0
- type: mrr_at_100
value: 100.0
- type: mrr_at_1000
value: 100.0
- type: mrr_at_20
value: 100.0
- type: mrr_at_3
value: 100.0
- type: mrr_at_5
value: 100.0
- type: ndcg_at_1
value: 100.0
- type: ndcg_at_10
value: 100.0
- type: ndcg_at_100
value: 100.0
- type: ndcg_at_1000
value: 100.0
- type: ndcg_at_20
value: 100.0
- type: ndcg_at_3
value: 100.0
- type: ndcg_at_5
value: 100.0
- type: precision_at_1
value: 100.0
- type: precision_at_10
value: 10.0
- type: precision_at_100
value: 1.0
- type: precision_at_1000
value: 0.1
- type: precision_at_20
value: 5.0
- type: precision_at_3
value: 33.333
- type: precision_at_5
value: 20.0
- type: recall_at_1
value: 100.0
- type: recall_at_10
value: 100.0
- type: recall_at_100
value: 100.0
- type: recall_at_1000
value: 100.0
- type: recall_at_20
value: 100.0
- type: recall_at_3
value: 100.0
- type: recall_at_5
value: 100.0
task:
type: Retrieval
- dataset:
config: default
name: MTEB LEMBQMSumRetrieval (default)
revision: 6e346642246bfb4928c560ee08640dc84d074e8c
split: test
type: dwzhu/LongEmbed
metrics:
- type: main_score
value: 29.847
- type: map_at_1
value: 18.271
- type: map_at_10
value: 25.415
- type: map_at_100
value: 27.086
- type: map_at_1000
value: 27.134000000000004
- type: map_at_20
value: 26.135
- type: map_at_3
value: 22.659000000000002
- type: map_at_5
value: 24.198
- type: mrr_at_1
value: 18.271119842829076
- type: mrr_at_10
value: 25.414678641594147
- type: mrr_at_100
value: 27.086094547163714
- type: mrr_at_1000
value: 27.13383971528746
- type: mrr_at_20
value: 26.13474777243653
- type: mrr_at_3
value: 22.658808120497696
- type: mrr_at_5
value: 24.197773411918757
- type: ndcg_at_1
value: 18.271
- type: ndcg_at_10
value: 29.847
- type: ndcg_at_100
value: 39.669
- type: ndcg_at_1000
value: 40.528999999999996
- type: ndcg_at_20
value: 32.509
- type: ndcg_at_3
value: 24.151
- type: ndcg_at_5
value: 26.927
- type: precision_at_1
value: 18.271
- type: precision_at_10
value: 4.42
- type: precision_at_100
value: 0.9400000000000001
- type: precision_at_1000
value: 0.1
- type: precision_at_20
value: 2.741
- type: precision_at_3
value: 9.496
- type: precision_at_5
value: 7.045999999999999
- type: recall_at_1
value: 18.271
- type: recall_at_10
value: 44.204
- type: recall_at_100
value: 93.975
- type: recall_at_1000
value: 100.0
- type: recall_at_20
value: 54.813
- type: recall_at_3
value: 28.487000000000002
- type: recall_at_5
value: 35.232
task:
type: Retrieval
- dataset:
config: default
name: MTEB LEMBSummScreenFDRetrieval (default)
revision: 6e346642246bfb4928c560ee08640dc84d074e8c
split: validation
type: dwzhu/LongEmbed
metrics:
- type: main_score
value: 90.19
- type: map_at_1
value: 81.548
- type: map_at_10
value: 87.57300000000001
- type: map_at_100
value: 87.703
- type: map_at_1000
value: 87.703
- type: map_at_20
value: 87.703
- type: map_at_3
value: 86.657
- type: map_at_5
value: 87.178
- type: mrr_at_1
value: 81.54761904761905
- type: mrr_at_10
value: 87.57345993953139
- type: mrr_at_100
value: 87.70306685222651
- type: mrr_at_1000
value: 87.70306685222651
- type: mrr_at_20
value: 87.70306685222651
- type: mrr_at_3
value: 86.65674603174602
- type: mrr_at_5
value: 87.17757936507935
- type: ndcg_at_1
value: 81.548
- type: ndcg_at_10
value: 90.19
- type: ndcg_at_100
value: 90.648
- type: ndcg_at_1000
value: 90.648
- type: ndcg_at_20
value: 90.648
- type: ndcg_at_3
value: 88.325
- type: ndcg_at_5
value: 89.286
- type: precision_at_1
value: 81.548
- type: precision_at_10
value: 9.821
- type: precision_at_100
value: 1.0
- type: precision_at_1000
value: 0.1
- type: precision_at_20
value: 5.0
- type: precision_at_3
value: 31.052000000000003
- type: precision_at_5
value: 19.107
- type: recall_at_1
value: 81.548
- type: recall_at_10
value: 98.214
- type: recall_at_100
value: 100.0
- type: recall_at_1000
value: 100.0
- type: recall_at_20
value: 100.0
- type: recall_at_3
value: 93.155
- type: recall_at_5
value: 95.536
task:
type: Retrieval
- dataset:
config: default
name: MTEB LEMBWikimQARetrieval (default)
revision: 6e346642246bfb4928c560ee08640dc84d074e8c
split: test
type: dwzhu/LongEmbed
metrics:
- type: main_score
value: 77.203
- type: map_at_1
value: 69.333
- type: map_at_10
value: 74.787
- type: map_at_100
value: 75.348
- type: map_at_1000
value: 75.369
- type: map_at_20
value: 75.16000000000001
- type: map_at_3
value: 73.611
- type: map_at_5
value: 74.42800000000001
- type: mrr_at_1
value: 69.33333333333334
- type: mrr_at_10
value: 74.78743386243384
- type: mrr_at_100
value: 75.34827076805841
- type: mrr_at_1000
value: 75.36876455686495
- type: mrr_at_20
value: 75.16008204758204
- type: mrr_at_3
value: 73.61111111111111
- type: mrr_at_5
value: 74.42777777777778
- type: ndcg_at_1
value: 69.333
- type: ndcg_at_10
value: 77.203
- type: ndcg_at_100
value: 79.87100000000001
- type: ndcg_at_1000
value: 80.286
- type: ndcg_at_20
value: 78.55499999999999
- type: ndcg_at_3
value: 74.917
- type: ndcg_at_5
value: 76.337
- type: precision_at_1
value: 69.333
- type: precision_at_10
value: 8.466999999999999
- type: precision_at_100
value: 0.97
- type: precision_at_1000
value: 0.1
- type: precision_at_20
value: 4.5
- type: precision_at_3
value: 26.222
- type: precision_at_5
value: 16.400000000000002
- type: recall_at_1
value: 69.333
- type: recall_at_10
value: 84.667
- type: recall_at_100
value: 97.0
- type: recall_at_1000
value: 100.0
- type: recall_at_20
value: 90.0
- type: recall_at_3
value: 78.667
- type: recall_at_5
value: 82.0
task:
type: Retrieval
- dataset:
config: default
name: MTEB LegalBenchConsumerContractsQA (default)
revision: b23590301ec94e8087e2850b21d43d4956b1cca9
split: test
type: mteb/legalbench_consumer_contracts_qa
metrics:
- type: main_score
value: 61.623000000000005
- type: map_at_1
value: 40.909
- type: map_at_10
value: 54.376999999999995
- type: map_at_100
value: 55.150999999999996
- type: map_at_1000
value: 55.150999999999996
- type: map_at_20
value: 54.881
- type: map_at_3
value: 49.916
- type: map_at_5
value: 52.883
- type: mrr_at_1
value: 41.16161616161616
- type: mrr_at_10
value: 54.502765752765725
- type: mrr_at_100
value: 55.27732053682153
- type: mrr_at_1000
value: 55.27732053682153
- type: mrr_at_20
value: 55.00715286102044
- type: mrr_at_3
value: 50.04208754208756
- type: mrr_at_5
value: 53.00925925925923
- type: ndcg_at_1
value: 40.909
- type: ndcg_at_10
value: 61.623000000000005
- type: ndcg_at_100
value: 65.08500000000001
- type: ndcg_at_1000
value: 65.08500000000001
- type: ndcg_at_20
value: 63.427
- type: ndcg_at_3
value: 52.735
- type: ndcg_at_5
value: 58.114
- type: precision_at_1
value: 40.909
- type: precision_at_10
value: 8.459999999999999
- type: precision_at_100
value: 1.0
- type: precision_at_1000
value: 0.1
- type: precision_at_20
value: 4.583
- type: precision_at_3
value: 20.286
- type: precision_at_5
value: 14.798
- type: recall_at_1
value: 40.909
- type: recall_at_10
value: 84.596
- type: recall_at_100
value: 100.0
- type: recall_at_1000
value: 100.0
- type: recall_at_20
value: 91.667
- type: recall_at_3
value: 60.858999999999995
- type: recall_at_5
value: 73.99
task:
type: Retrieval
- dataset:
config: default
name: MTEB LegalBenchCorporateLobbying (default)
revision: f69691c650464e62546d7f2a4536f8f87c891e38
split: test
type: mteb/legalbench_corporate_lobbying
metrics:
- type: main_score
value: 89.377
- type: map_at_1
value: 79.706
- type: map_at_10
value: 86.652
- type: map_at_100
value: 86.756
- type: map_at_1000
value: 86.76
- type: map_at_20
value: 86.749
- type: map_at_3
value: 85.784
- type: map_at_5
value: 86.387
- type: mrr_at_1
value: 79.70588235294119
- type: mrr_at_10
value: 86.65184407096169
- type: mrr_at_100
value: 86.75604621101796
- type: mrr_at_1000
value: 86.76035993650815
- type: mrr_at_20
value: 86.7486932698415
- type: mrr_at_3
value: 85.78431372549021
- type: mrr_at_5
value: 86.38725490196077
- type: ndcg_at_1
value: 79.706
- type: ndcg_at_10
value: 89.377
- type: ndcg_at_100
value: 89.79700000000001
- type: ndcg_at_1000
value: 89.88000000000001
- type: ndcg_at_20
value: 89.742
- type: ndcg_at_3
value: 87.63300000000001
- type: ndcg_at_5
value: 88.721
- type: precision_at_1
value: 79.706
- type: precision_at_10
value: 9.765
- type: precision_at_100
value: 0.9939999999999999
- type: precision_at_1000
value: 0.1
- type: precision_at_20
value: 4.956
- type: precision_at_3
value: 30.98
- type: precision_at_5
value: 19.118
- type: recall_at_1
value: 79.706
- type: recall_at_10
value: 97.64699999999999
- type: recall_at_100
value: 99.412
- type: recall_at_1000
value: 100.0
- type: recall_at_20
value: 99.118
- type: recall_at_3
value: 92.941
- type: recall_at_5
value: 95.588
task:
type: Retrieval
- dataset:
config: default
name: MTEB LegalSummarization (default)
revision: 3bb1a05c66872889662af04c5691c14489cebd72
split: test
type: mteb/legal_summarization
metrics:
- type: main_score
value: 54.98800000000001
- type: map_at_1
value: 37.468
- type: map_at_10
value: 48.509
- type: map_at_100
value: 49.681
- type: map_at_1000
value: 49.757
- type: map_at_20
value: 49.021
- type: map_at_3
value: 44.59
- type: map_at_5
value: 46.867999999999995
- type: mrr_at_1
value: 42.6056338028169
- type: mrr_at_10
value: 53.24223116476639
- type: mrr_at_100
value: 53.82518326740263
- type: mrr_at_1000
value: 53.86171229208665
- type: mrr_at_20
value: 53.51133505321795
- type: mrr_at_3
value: 49.76525821596244
- type: mrr_at_5
value: 51.87793427230047
- type: ndcg_at_1
value: 42.606
- type: ndcg_at_10
value: 54.98800000000001
- type: ndcg_at_100
value: 60.111000000000004
- type: ndcg_at_1000
value: 61.382000000000005
- type: ndcg_at_20
value: 56.428999999999995
- type: ndcg_at_3
value: 48.367
- type: ndcg_at_5
value: 51.72
- type: precision_at_1
value: 42.606
- type: precision_at_10
value: 9.331
- type: precision_at_100
value: 1.398
- type: precision_at_1000
value: 0.155
- type: precision_at_20
value: 5.176
- type: precision_at_3
value: 21.009
- type: precision_at_5
value: 15.211
- type: recall_at_1
value: 37.468
- type: recall_at_10
value: 69.607
- type: recall_at_100
value: 91.57
- type: recall_at_1000
value: 100.0
- type: recall_at_20
value: 74.553
- type: recall_at_3
value: 51.856
- type: recall_at_5
value: 60.309999999999995
task:
type: Retrieval
- dataset:
config: default
name: MTEB LitSearchRetrieval (default)
revision: 9573fb284a1026c998df47024b888a163f0f0e25
split: test
type: princeton-nlp/LitSearch
metrics:
- type: main_score
value: 25.226
- type: map_at_1
value: 15.326999999999998
- type: map_at_10
value: 21.394
- type: map_at_100
value: 22.343
- type: map_at_1000
value: 22.429
- type: map_at_20
value: 21.941
- type: map_at_3
value: 19.123
- type: map_at_5
value: 20.14
- type: mrr_at_1
value: 15.745393634840871
- type: mrr_at_10
value: 21.745699396453173
- type: mrr_at_100
value: 22.713350239272813
- type: mrr_at_1000
value: 22.79392349547639
- type: mrr_at_20
value: 22.30884258376597
- type: mrr_at_3
value: 19.458403126744827
- type: mrr_at_5
value: 20.538805136795084
- type: ndcg_at_1
value: 15.494
- type: ndcg_at_10
value: 25.226
- type: ndcg_at_100
value: 30.263
- type: ndcg_at_1000
value: 32.994
- type: ndcg_at_20
value: 27.183
- type: ndcg_at_3
value: 20.385
- type: ndcg_at_5
value: 22.21
- type: precision_at_1
value: 15.745000000000001
- type: precision_at_10
value: 3.987
- type: precision_at_100
value: 0.657
- type: precision_at_1000
value: 0.09
- type: precision_at_20
value: 2.404
- type: precision_at_3
value: 8.319
- type: precision_at_5
value: 5.93
- type: recall_at_1
value: 15.326999999999998
- type: recall_at_10
value: 37.968
- type: recall_at_100
value: 62.546
- type: recall_at_1000
value: 84.87700000000001
- type: recall_at_20
value: 45.739999999999995
- type: recall_at_3
value: 24.204
- type: recall_at_5
value: 28.615000000000002
task:
type: Retrieval
- dataset:
config: default
name: MTEB MSMARCO (default)
revision: c5a29a104738b98a9e76336939199e264163d4a0
split: test
type: mteb/msmarco
metrics:
- type: main_score
value: 39.988
- type: map_at_1
value: 1.055
- type: map_at_10
value: 7.149
- type: map_at_100
value: 21.816
- type: map_at_1000
value: 29.885
- type: map_at_20
value: 11.162999999999998
- type: map_at_3
value: 2.735
- type: map_at_5
value: 4.199
- type: mrr_at_1
value: 60.46511627906976
- type: mrr_at_10
value: 71.29844961240309
- type: mrr_at_100
value: 71.4438037073023
- type: mrr_at_1000
value: 71.45852257689312
- type: mrr_at_20
value: 71.29844961240309
- type: mrr_at_3
value: 69.3798449612403
- type: mrr_at_5
value: 71.00775193798448
- type: ndcg_at_1
value: 39.535
- type: ndcg_at_10
value: 39.988
- type: ndcg_at_100
value: 41.952
- type: ndcg_at_1000
value: 55.149
- type: ndcg_at_20
value: 39.861000000000004
- type: ndcg_at_3
value: 39.713
- type: ndcg_at_5
value: 40.2
- type: precision_at_1
value: 60.465
- type: precision_at_10
value: 52.791
- type: precision_at_100
value: 29.558
- type: precision_at_1000
value: 6.952999999999999
- type: precision_at_20
value: 47.209
- type: precision_at_3
value: 57.364000000000004
- type: precision_at_5
value: 56.279
- type: recall_at_1
value: 1.055
- type: recall_at_10
value: 8.778
- type: recall_at_100
value: 36.775999999999996
- type: recall_at_1000
value: 72.783
- type: recall_at_20
value: 14.529
- type: recall_at_3
value: 3.019
- type: recall_at_5
value: 4.987
task:
type: Retrieval
- dataset:
config: default
name: MTEB MSMARCOHardNegatives (default)
revision: 67c0b4f7f15946e0b15cf6cf3b8993d04cb3efc6
split: test
type: mteb/MSMARCO_test_top_250_only_w_correct-v2
metrics:
- type: main_score
value: 49.17
- type: map_at_1
value: 1.871
- type: map_at_10
value: 11.155
- type: map_at_100
value: 38.995000000000005
- type: map_at_1000
value: 58.543
- type: map_at_20
value: 16.564
- type: map_at_3
value: 5.378
- type: map_at_5
value: 7.403
- type: mrr_at_1
value: 69.76744186046511
- type: mrr_at_10
value: 78.7624584717608
- type: mrr_at_100
value: 78.97387496224707
- type: mrr_at_1000
value: 78.97387496224707
- type: mrr_at_20
value: 78.97387496224707
- type: mrr_at_3
value: 76.74418604651163
- type: mrr_at_5
value: 77.90697674418605
- type: ndcg_at_1
value: 46.899
- type: ndcg_at_10
value: 49.17
- type: ndcg_at_100
value: 62.022
- type: ndcg_at_1000
value: 76.946
- type: ndcg_at_20
value: 50.113
- type: ndcg_at_3
value: 47.809000000000005
- type: ndcg_at_5
value: 47.947
- type: precision_at_1
value: 69.767
- type: precision_at_10
value: 63.256
- type: precision_at_100
value: 46.302
- type: precision_at_1000
value: 9.419
- type: precision_at_20
value: 58.48799999999999
- type: precision_at_3
value: 69.767
- type: precision_at_5
value: 66.047
- type: recall_at_1
value: 1.871
- type: recall_at_10
value: 12.928999999999998
- type: recall_at_100
value: 60.528000000000006
- type: recall_at_1000
value: 98.18599999999999
- type: recall_at_20
value: 20.534
- type: recall_at_3
value: 5.611
- type: recall_at_5
value: 8.054
task:
type: Retrieval
- dataset:
config: default
name: MTEB MedicalQARetrieval (default)
revision: ae763399273d8b20506b80cf6f6f9a31a6a2b238
split: test
type: mteb/medical_qa
metrics:
- type: main_score
value: 47.905
- type: map_at_1
value: 23.926
- type: map_at_10
value: 39.777
- type: map_at_100
value: 40.449
- type: map_at_1000
value: 40.486
- type: map_at_20
value: 40.204
- type: map_at_3
value: 35.531
- type: map_at_5
value: 38.25
- type: mrr_at_1
value: 23.876953125
- type: mrr_at_10
value: 39.77653382316477
- type: mrr_at_100
value: 40.449022311809415
- type: mrr_at_1000
value: 40.485791901682774
- type: mrr_at_20
value: 40.20422058322255
- type: mrr_at_3
value: 35.53873697916675
- type: mrr_at_5
value: 38.25846354166688
- type: ndcg_at_1
value: 23.926
- type: ndcg_at_10
value: 47.905
- type: ndcg_at_100
value: 51.186
- type: ndcg_at_1000
value: 52.297000000000004
- type: ndcg_at_20
value: 49.445
- type: ndcg_at_3
value: 39.391
- type: ndcg_at_5
value: 44.254
- type: precision_at_1
value: 23.926
- type: precision_at_10
value: 7.349
- type: precision_at_100
value: 0.889
- type: precision_at_1000
value: 0.098
- type: precision_at_20
value: 3.977
- type: precision_at_3
value: 16.862
- type: precision_at_5
value: 12.461
- type: recall_at_1
value: 23.926
- type: recall_at_10
value: 73.48599999999999
- type: recall_at_100
value: 88.86699999999999
- type: recall_at_1000
value: 97.89999999999999
- type: recall_at_20
value: 79.541
- type: recall_at_3
value: 50.586
- type: recall_at_5
value: 62.305
task:
type: Retrieval
- dataset:
config: default
name: MTEB NFCorpus (default)
revision: ec0fa4fe99da2ff19ca1214b7966684033a58814
split: test
type: mteb/nfcorpus
metrics:
- type: main_score
value: 30.044999999999998
- type: map_at_1
value: 5.015
- type: map_at_10
value: 10.93
- type: map_at_100
value: 13.592
- type: map_at_1000
value: 14.890999999999998
- type: map_at_20
value: 12.005
- type: map_at_3
value: 8.518
- type: map_at_5
value: 9.646
- type: mrr_at_1
value: 41.17647058823529
- type: mrr_at_10
value: 49.96486313823774
- type: mrr_at_100
value: 50.73871199227761
- type: mrr_at_1000
value: 50.788364180879874
- type: mrr_at_20
value: 50.53695651632227
- type: mrr_at_3
value: 47.936016511867905
- type: mrr_at_5
value: 49.267285861713106
- type: ndcg_at_1
value: 39.164
- type: ndcg_at_10
value: 30.044999999999998
- type: ndcg_at_100
value: 27.654
- type: ndcg_at_1000
value: 36.397
- type: ndcg_at_20
value: 28.016000000000002
- type: ndcg_at_3
value: 35.476
- type: ndcg_at_5
value: 33.123999999999995
- type: precision_at_1
value: 41.176
- type: precision_at_10
value: 21.765
- type: precision_at_100
value: 7.127
- type: precision_at_1000
value: 1.9959999999999998
- type: precision_at_20
value: 16.223000000000003
- type: precision_at_3
value: 33.333
- type: precision_at_5
value: 28.421000000000003
- type: recall_at_1
value: 5.015
- type: recall_at_10
value: 14.618999999999998
- type: recall_at_100
value: 27.755000000000003
- type: recall_at_1000
value: 59.302
- type: recall_at_20
value: 17.743000000000002
- type: recall_at_3
value: 9.769
- type: recall_at_5
value: 11.912
task:
type: Retrieval
- dataset:
config: default
name: MTEB NQ (default)
revision: b774495ed302d8c44a3a7ea25c90dbce03968f31
split: test
type: mteb/nq
metrics:
- type: main_score
value: 23.105
- type: map_at_1
value: 9.2
- type: map_at_10
value: 17.564
- type: map_at_100
value: 19.226
- type: map_at_1000
value: 19.323
- type: map_at_20
value: 18.507
- type: map_at_3
value: 14.274999999999999
- type: map_at_5
value: 15.98
- type: mrr_at_1
value: 10.573580533024334
- type: mrr_at_10
value: 19.264677481653145
- type: mrr_at_100
value: 20.746572887142992
- type: mrr_at_1000
value: 20.823910481320183
- type: mrr_at_20
value: 20.119865220550533
- type: mrr_at_3
value: 16.005214368482008
- type: mrr_at_5
value: 17.75057937427577
- type: ndcg_at_1
value: 10.545
- type: ndcg_at_10
value: 23.105
- type: ndcg_at_100
value: 31.249
- type: ndcg_at_1000
value: 33.69
- type: ndcg_at_20
value: 26.334999999999997
- type: ndcg_at_3
value: 16.357
- type: ndcg_at_5
value: 19.403000000000002
- type: precision_at_1
value: 10.545
- type: precision_at_10
value: 4.565
- type: precision_at_100
value: 0.9169999999999999
- type: precision_at_1000
value: 0.11499999999999999
- type: precision_at_20
value: 3.023
- type: precision_at_3
value: 8.033999999999999
- type: precision_at_5
value: 6.524000000000001
- type: recall_at_1
value: 9.2
- type: recall_at_10
value: 38.775
- type: recall_at_100
value: 76.188
- type: recall_at_1000
value: 94.56599999999999
- type: recall_at_20
value: 50.9
- type: recall_at_3
value: 20.676
- type: recall_at_5
value: 27.810000000000002
task:
type: Retrieval
- dataset:
config: default
name: MTEB NQHardNegatives (default)
revision: d700fe4f167a5db8e6c9b03e8c26e7eaf66faf97
split: test
type: mteb/NQ_test_top_250_only_w_correct-v2
metrics:
- type: main_score
value: 25.072
- type: map_at_1
value: 9.767000000000001
- type: map_at_10
value: 18.782
- type: map_at_100
value: 20.74
- type: map_at_1000
value: 20.821
- type: map_at_20
value: 19.819
- type: map_at_3
value: 15.089
- type: map_at_5
value: 16.929
- type: mrr_at_1
value: 11.0
- type: mrr_at_10
value: 20.55162698412698
- type: mrr_at_100
value: 22.24001716934204
- type: mrr_at_1000
value: 22.296375463042438
- type: mrr_at_20
value: 21.45978809546147
- type: mrr_at_3
value: 16.683333333333337
- type: mrr_at_5
value: 18.738333333333344
- type: ndcg_at_1
value: 11.0
- type: ndcg_at_10
value: 25.072
- type: ndcg_at_100
value: 34.695
- type: ndcg_at_1000
value: 36.312
- type: ndcg_at_20
value: 28.595
- type: ndcg_at_3
value: 17.273
- type: ndcg_at_5
value: 20.663999999999998
- type: precision_at_1
value: 11.0
- type: precision_at_10
value: 5.01
- type: precision_at_100
value: 1.05
- type: precision_at_1000
value: 0.12
- type: precision_at_20
value: 3.335
- type: precision_at_3
value: 8.433
- type: precision_at_5
value: 6.959999999999999
- type: recall_at_1
value: 9.767000000000001
- type: recall_at_10
value: 43.175000000000004
- type: recall_at_100
value: 87.467
- type: recall_at_1000
value: 98.917
- type: recall_at_20
value: 56.325
- type: recall_at_3
value: 22.033
- type: recall_at_5
value: 29.975
task:
type: Retrieval
- dataset:
config: default
name: MTEB PIQA (default)
revision: bb30be7e9184e6b6b1d99bbfe1bb90a3a81842e6
split: test
type: RAR-b/piqa
metrics:
- type: main_score
value: 15.254000000000001
- type: map_at_1
value: 7.073
- type: map_at_10
value: 12.281
- type: map_at_100
value: 13.178
- type: map_at_1000
value: 13.26
- type: map_at_20
value: 12.753999999999998
- type: map_at_3
value: 10.591000000000001
- type: map_at_5
value: 11.522
- type: mrr_at_1
value: 7.127312295973885
- type: mrr_at_10
value: 12.307826830405721
- type: mrr_at_100
value: 13.205659849576772
- type: mrr_at_1000
value: 13.28739675923323
- type: mrr_at_20
value: 12.781972613283662
- type: mrr_at_3
value: 10.618425825172299
- type: mrr_at_5
value: 11.548784911135296
- type: ndcg_at_1
value: 7.073
- type: ndcg_at_10
value: 15.254000000000001
- type: ndcg_at_100
value: 20.077
- type: ndcg_at_1000
value: 22.43
- type: ndcg_at_20
value: 16.948
- type: ndcg_at_3
value: 11.741
- type: ndcg_at_5
value: 13.420000000000002
- type: precision_at_1
value: 7.073
- type: precision_at_10
value: 2.481
- type: precision_at_100
value: 0.484
- type: precision_at_1000
value: 0.067
- type: precision_at_20
value: 1.572
- type: precision_at_3
value: 5.024
- type: precision_at_5
value: 3.83
- type: recall_at_1
value: 7.073
- type: recall_at_10
value: 24.81
- type: recall_at_100
value: 48.422
- type: recall_at_1000
value: 67.35600000000001
- type: recall_at_20
value: 31.447000000000003
- type: recall_at_3
value: 15.071000000000002
- type: recall_at_5
value: 19.151
task:
type: Retrieval
- dataset:
config: default
name: MTEB Quail (default)
revision: 1851bc536f8bdab29e03e29191c4586b1d8d7c5a
split: test
type: RAR-b/quail
metrics:
- type: main_score
value: 3.605
- type: map_at_1
value: 0.882
- type: map_at_10
value: 2.467
- type: map_at_100
value: 2.9659999999999997
- type: map_at_1000
value: 3.052
- type: map_at_20
value: 2.711
- type: map_at_3
value: 1.746
- type: map_at_5
value: 2.0629999999999997
- type: mrr_at_1
value: 0.8823529411764706
- type: mrr_at_10
value: 2.4645337301587333
- type: mrr_at_100
value: 2.9670174083352596
- type: mrr_at_1000
value: 3.0527771606810092
- type: mrr_at_20
value: 2.7112556752180152
- type: mrr_at_3
value: 1.7463235294117647
- type: mrr_at_5
value: 2.0625000000000013
- type: ndcg_at_1
value: 0.882
- type: ndcg_at_10
value: 3.605
- type: ndcg_at_100
value: 6.494999999999999
- type: ndcg_at_1000
value: 9.27
- type: ndcg_at_20
value: 4.502
- type: ndcg_at_3
value: 2.0340000000000003
- type: ndcg_at_5
value: 2.614
- type: precision_at_1
value: 0.882
- type: precision_at_10
value: 0.739
- type: precision_at_100
value: 0.22
- type: precision_at_1000
value: 0.045
- type: precision_at_20
value: 0.5479999999999999
- type: precision_at_3
value: 0.9560000000000001
- type: precision_at_5
value: 0.86
- type: recall_at_1
value: 0.882
- type: recall_at_10
value: 7.39
- type: recall_at_100
value: 21.985
- type: recall_at_1000
value: 44.926
- type: recall_at_20
value: 10.956000000000001
- type: recall_at_3
value: 2.868
- type: recall_at_5
value: 4.301
task:
type: Retrieval
- dataset:
config: default
name: MTEB QuoraRetrieval (default)
revision: e4e08e0b7dbe3c8700f0daef558ff32256715259
split: dev
type: mteb/quora
metrics:
- type: main_score
value: 77.63
- type: map_at_1
value: 60.195
- type: map_at_10
value: 72.834
- type: map_at_100
value: 73.657
- type: map_at_1000
value: 73.68900000000001
- type: map_at_20
value: 73.358
- type: map_at_3
value: 69.834
- type: map_at_5
value: 71.622
- type: mrr_at_1
value: 69.12
- type: mrr_at_10
value: 76.90555555555562
- type: mrr_at_100
value: 77.22690418927783
- type: mrr_at_1000
value: 77.23378887488153
- type: mrr_at_20
value: 77.12735614892509
- type: mrr_at_3
value: 75.29666666666685
- type: mrr_at_5
value: 76.29566666666672
- type: ndcg_at_1
value: 69.06
- type: ndcg_at_10
value: 77.63
- type: ndcg_at_100
value: 80.143
- type: ndcg_at_1000
value: 80.57900000000001
- type: ndcg_at_20
value: 78.886
- type: ndcg_at_3
value: 73.735
- type: ndcg_at_5
value: 75.689
- type: precision_at_1
value: 69.06
- type: precision_at_10
value: 11.804
- type: precision_at_100
value: 1.417
- type: precision_at_1000
value: 0.151
- type: precision_at_20
value: 6.383
- type: precision_at_3
value: 32.0
- type: precision_at_5
value: 21.279999999999998
- type: recall_at_1
value: 60.195
- type: recall_at_10
value: 87.35300000000001
- type: recall_at_100
value: 97.055
- type: recall_at_1000
value: 99.669
- type: recall_at_20
value: 91.628
- type: recall_at_3
value: 76.40599999999999
- type: recall_at_5
value: 81.636
task:
type: Retrieval
- dataset:
config: default
name: MTEB QuoraRetrieval (default)
revision: e4e08e0b7dbe3c8700f0daef558ff32256715259
split: test
type: mteb/quora
metrics:
- type: main_score
value: 77.529
- type: map_at_1
value: 60.492000000000004
- type: map_at_10
value: 72.82499999999999
- type: map_at_100
value: 73.668
- type: map_at_1000
value: 73.706
- type: map_at_20
value: 73.351
- type: map_at_3
value: 69.887
- type: map_at_5
value: 71.66
- type: mrr_at_1
value: 69.59
- type: mrr_at_10
value: 76.96056349206305
- type: mrr_at_100
value: 77.29271487249463
- type: mrr_at_1000
value: 77.30133384825908
- type: mrr_at_20
value: 77.1902356844216
- type: mrr_at_3
value: 75.39499999999957
- type: mrr_at_5
value: 76.38149999999933
- type: ndcg_at_1
value: 69.61
- type: ndcg_at_10
value: 77.529
- type: ndcg_at_100
value: 80.067
- type: ndcg_at_1000
value: 80.54299999999999
- type: ndcg_at_20
value: 78.76100000000001
- type: ndcg_at_3
value: 73.786
- type: ndcg_at_5
value: 75.696
- type: precision_at_1
value: 69.61
- type: precision_at_10
value: 11.756
- type: precision_at_100
value: 1.436
- type: precision_at_1000
value: 0.154
- type: precision_at_20
value: 6.382000000000001
- type: precision_at_3
value: 31.996999999999996
- type: precision_at_5
value: 21.198
- type: recall_at_1
value: 60.492000000000004
- type: recall_at_10
value: 86.887
- type: recall_at_100
value: 96.67999999999999
- type: recall_at_1000
value: 99.438
- type: recall_at_20
value: 91.081
- type: recall_at_3
value: 76.212
- type: recall_at_5
value: 81.48100000000001
task:
type: Retrieval
- dataset:
config: default
name: MTEB QuoraRetrievalHardNegatives (default)
revision: 907a33577e9506221d3ba20f5a851b7c3f8dc6d3
split: test
type: mteb/QuoraRetrieval_test_top_250_only_w_correct-v2
metrics:
- type: main_score
value: 77.352
- type: map_at_1
value: 60.285999999999994
- type: map_at_10
value: 72.541
- type: map_at_100
value: 73.576
- type: map_at_1000
value: 73.61999999999999
- type: map_at_20
value: 73.236
- type: map_at_3
value: 69.434
- type: map_at_5
value: 71.177
- type: mrr_at_1
value: 69.6
- type: mrr_at_10
value: 76.9061111111111
- type: mrr_at_100
value: 77.25264059161483
- type: mrr_at_1000
value: 77.25961973203633
- type: mrr_at_20
value: 77.14650618344506
- type: mrr_at_3
value: 75.20000000000002
- type: mrr_at_5
value: 76.18
- type: ndcg_at_1
value: 69.6
- type: ndcg_at_10
value: 77.352
- type: ndcg_at_100
value: 80.24
- type: ndcg_at_1000
value: 80.658
- type: ndcg_at_20
value: 78.90599999999999
- type: ndcg_at_3
value: 73.534
- type: ndcg_at_5
value: 75.18599999999999
- type: precision_at_1
value: 69.6
- type: precision_at_10
value: 12.08
- type: precision_at_100
value: 1.514
- type: precision_at_1000
value: 0.16199999999999998
- type: precision_at_20
value: 6.69
- type: precision_at_3
value: 32.067
- type: precision_at_5
value: 21.279999999999998
- type: recall_at_1
value: 60.285999999999994
- type: recall_at_10
value: 86.75399999999999
- type: recall_at_100
value: 97.328
- type: recall_at_1000
value: 99.551
- type: recall_at_20
value: 91.85900000000001
- type: recall_at_3
value: 75.408
- type: recall_at_5
value: 80.353
task:
type: Retrieval
- dataset:
config: default
name: MTEB RARbCode (default)
revision: 25f7d11a7ac12dcbb8d3836eb2de682b98c825e4
split: test
type: RAR-b/humanevalpack-mbpp-pooled
metrics:
- type: main_score
value: 10.407
- type: map_at_1
value: 6.132
- type: map_at_10
value: 8.741
- type: map_at_100
value: 9.198
- type: map_at_1000
value: 9.264
- type: map_at_20
value: 8.991
- type: map_at_3
value: 7.637
- type: map_at_5
value: 8.21
- type: mrr_at_1
value: 6.132075471698113
- type: mrr_at_10
value: 8.741389637616054
- type: mrr_at_100
value: 9.19843044441297
- type: mrr_at_1000
value: 9.263870046532622
- type: mrr_at_20
value: 8.991124756997893
- type: mrr_at_3
value: 7.637017070979335
- type: mrr_at_5
value: 8.209793351302785
- type: ndcg_at_1
value: 6.132
- type: ndcg_at_10
value: 10.407
- type: ndcg_at_100
value: 12.959000000000001
- type: ndcg_at_1000
value: 14.991
- type: ndcg_at_20
value: 11.324
- type: ndcg_at_3
value: 8.117
- type: ndcg_at_5
value: 9.146
- type: precision_at_1
value: 6.132
- type: precision_at_10
value: 1.584
- type: precision_at_100
value: 0.28600000000000003
- type: precision_at_1000
value: 0.045
- type: precision_at_20
value: 0.9740000000000001
- type: precision_at_3
value: 3.167
- type: precision_at_5
value: 2.399
- type: recall_at_1
value: 6.132
- type: recall_at_10
value: 15.836
- type: recall_at_100
value: 28.571
- type: recall_at_1000
value: 45.216
- type: recall_at_20
value: 19.474
- type: recall_at_3
value: 9.501
- type: recall_at_5
value: 11.995000000000001
task:
type: Retrieval
- dataset:
config: default
name: MTEB RARbMath (default)
revision: 2393603c0221ff52f448d12dd75f0856103c6cca
split: test
type: RAR-b/math-pooled
metrics:
- type: main_score
value: 23.658
- type: map_at_1
value: 19.686999999999998
- type: map_at_10
value: 22.178
- type: map_at_100
value: 22.765
- type: map_at_1000
value: 22.844
- type: map_at_20
value: 22.462
- type: map_at_3
value: 21.29
- type: map_at_5
value: 21.787
- type: mrr_at_1
value: 19.686659281531888
- type: mrr_at_10
value: 22.177553460588754
- type: mrr_at_100
value: 22.7654510715158
- type: mrr_at_1000
value: 22.843891574167113
- type: mrr_at_20
value: 22.46217836587706
- type: mrr_at_3
value: 21.290288547765996
- type: mrr_at_5
value: 21.787202616447765
- type: ndcg_at_1
value: 19.686999999999998
- type: ndcg_at_10
value: 23.658
- type: ndcg_at_100
value: 27.0
- type: ndcg_at_1000
value: 29.509999999999998
- type: ndcg_at_20
value: 24.715
- type: ndcg_at_3
value: 21.817
- type: ndcg_at_5
value: 22.711000000000002
- type: precision_at_1
value: 19.686999999999998
- type: precision_at_10
value: 2.844
- type: precision_at_100
value: 0.45199999999999996
- type: precision_at_1000
value: 0.066
- type: precision_at_20
value: 1.633
- type: precision_at_3
value: 7.781000000000001
- type: precision_at_5
value: 5.102
- type: recall_at_1
value: 19.686999999999998
- type: recall_at_10
value: 28.438000000000002
- type: recall_at_100
value: 45.165
- type: recall_at_1000
value: 65.865
- type: recall_at_20
value: 32.663
- type: recall_at_3
value: 23.342
- type: recall_at_5
value: 25.509999999999998
task:
type: Retrieval
- dataset:
config: default
name: MTEB SCIDOCS (default)
revision: f8c2fcf00f625baaa80f62ec5bd9e1fff3b8ae88
split: test
type: mteb/scidocs
metrics:
- type: main_score
value: 13.222999999999999
- type: map_at_1
value: 2.9979999999999998
- type: map_at_10
value: 7.475
- type: map_at_100
value: 8.903
- type: map_at_1000
value: 9.16
- type: map_at_20
value: 8.136000000000001
- type: map_at_3
value: 5.329
- type: map_at_5
value: 6.411
- type: mrr_at_1
value: 14.7
- type: mrr_at_10
value: 22.86599206349206
- type: mrr_at_100
value: 24.016847471793167
- type: mrr_at_1000
value: 24.09878143285336
- type: mrr_at_20
value: 23.487873612455665
- type: mrr_at_3
value: 19.850000000000016
- type: mrr_at_5
value: 21.385000000000005
- type: ndcg_at_1
value: 14.7
- type: ndcg_at_10
value: 13.222999999999999
- type: ndcg_at_100
value: 19.725
- type: ndcg_at_1000
value: 24.723
- type: ndcg_at_20
value: 15.215
- type: ndcg_at_3
value: 12.073
- type: ndcg_at_5
value: 10.707
- type: precision_at_1
value: 14.7
- type: precision_at_10
value: 7.049999999999999
- type: precision_at_100
value: 1.6650000000000003
- type: precision_at_1000
value: 0.28600000000000003
- type: precision_at_20
value: 4.68
- type: precision_at_3
value: 11.3
- type: precision_at_5
value: 9.48
- type: recall_at_1
value: 2.9979999999999998
- type: recall_at_10
value: 14.277999999999999
- type: recall_at_100
value: 33.772000000000006
- type: recall_at_1000
value: 58.15
- type: recall_at_20
value: 18.956999999999997
- type: recall_at_3
value: 6.883
- type: recall_at_5
value: 9.613
task:
type: Retrieval
- dataset:
config: default
name: MTEB SIQA (default)
revision: 4ed8415e9dc24060deefc84be59e2db0aacbadcc
split: test
type: RAR-b/siqa
metrics:
- type: main_score
value: 0.517
- type: map_at_1
value: 0.307
- type: map_at_10
value: 0.42700000000000005
- type: map_at_100
value: 0.511
- type: map_at_1000
value: 0.5640000000000001
- type: map_at_20
value: 0.477
- type: map_at_3
value: 0.358
- type: map_at_5
value: 0.384
- type: mrr_at_1
value: 0.3070624360286591
- type: mrr_at_10
value: 0.42667868921707197
- type: mrr_at_100
value: 0.5106947806513232
- type: mrr_at_1000
value: 0.5637065102894676
- type: mrr_at_20
value: 0.476660924594987
- type: mrr_at_3
value: 0.35823950870010235
- type: mrr_at_5
value: 0.3838280450358239
- type: ndcg_at_1
value: 0.307
- type: ndcg_at_10
value: 0.517
- type: ndcg_at_100
value: 0.9570000000000001
- type: ndcg_at_1000
value: 3.83
- type: ndcg_at_20
value: 0.69
- type: ndcg_at_3
value: 0.372
- type: ndcg_at_5
value: 0.416
- type: precision_at_1
value: 0.307
- type: precision_at_10
value: 0.082
- type: precision_at_100
value: 0.03
- type: precision_at_1000
value: 0.029
- type: precision_at_20
value: 0.074
- type: precision_at_3
value: 0.136
- type: precision_at_5
value: 0.10200000000000001
- type: recall_at_1
value: 0.307
- type: recall_at_10
value: 0.819
- type: recall_at_100
value: 2.968
- type: recall_at_1000
value: 29.017
- type: recall_at_20
value: 1.484
- type: recall_at_3
value: 0.409
- type: recall_at_5
value: 0.512
task:
type: Retrieval
- dataset:
config: default
name: MTEB SciFact (default)
revision: 0228b52cf27578f30900b9e5271d331663a030d7
split: test
type: mteb/scifact
metrics:
- type: main_score
value: 59.348
- type: map_at_1
value: 45.306000000000004
- type: map_at_10
value: 54.547000000000004
- type: map_at_100
value: 55.535000000000004
- type: map_at_1000
value: 55.582
- type: map_at_20
value: 55.242000000000004
- type: map_at_3
value: 51.763000000000005
- type: map_at_5
value: 53.27499999999999
- type: mrr_at_1
value: 47.0
- type: mrr_at_10
value: 55.67632275132276
- type: mrr_at_100
value: 56.50056008171798
- type: mrr_at_1000
value: 56.54270500751058
- type: mrr_at_20
value: 56.277193298903846
- type: mrr_at_3
value: 53.22222222222223
- type: mrr_at_5
value: 54.70555555555556
- type: ndcg_at_1
value: 47.0
- type: ndcg_at_10
value: 59.348
- type: ndcg_at_100
value: 63.42100000000001
- type: ndcg_at_1000
value: 64.534
- type: ndcg_at_20
value: 61.622
- type: ndcg_at_3
value: 54.117000000000004
- type: ndcg_at_5
value: 56.669000000000004
- type: precision_at_1
value: 47.0
- type: precision_at_10
value: 8.1
- type: precision_at_100
value: 1.027
- type: precision_at_1000
value: 0.11199999999999999
- type: precision_at_20
value: 4.583
- type: precision_at_3
value: 21.221999999999998
- type: precision_at_5
value: 14.2
- type: recall_at_1
value: 45.306000000000004
- type: recall_at_10
value: 72.95
- type: recall_at_100
value: 90.533
- type: recall_at_1000
value: 99.1
- type: recall_at_20
value: 81.389
- type: recall_at_3
value: 58.9
- type: recall_at_5
value: 65.261
task:
type: Retrieval
- dataset:
config: default
name: MTEB SciFact (default)
revision: 0228b52cf27578f30900b9e5271d331663a030d7
split: train
type: mteb/scifact
metrics:
- type: main_score
value: 61.812999999999995
- type: map_at_1
value: 45.328
- type: map_at_10
value: 56.464000000000006
- type: map_at_100
value: 57.282
- type: map_at_1000
value: 57.312
- type: map_at_20
value: 57.019
- type: map_at_3
value: 53.447
- type: map_at_5
value: 55.452999999999996
- type: mrr_at_1
value: 47.83683559950556
- type: mrr_at_10
value: 58.03147134420309
- type: mrr_at_100
value: 58.65513617901087
- type: mrr_at_1000
value: 58.680986977449564
- type: mrr_at_20
value: 58.47209594120791
- type: mrr_at_3
value: 55.418211784095575
- type: mrr_at_5
value: 57.222908941079474
- type: ndcg_at_1
value: 47.837
- type: ndcg_at_10
value: 61.812999999999995
- type: ndcg_at_100
value: 65.254
- type: ndcg_at_1000
value: 66.116
- type: ndcg_at_20
value: 63.634
- type: ndcg_at_3
value: 56.239
- type: ndcg_at_5
value: 59.550000000000004
- type: precision_at_1
value: 47.837
- type: precision_at_10
value: 8.554
- type: precision_at_100
value: 1.043
- type: precision_at_1000
value: 0.11199999999999999
- type: precision_at_20
value: 4.697
- type: precision_at_3
value: 22.579
- type: precision_at_5
value: 15.476
- type: recall_at_1
value: 45.328
- type: recall_at_10
value: 76.667
- type: recall_at_100
value: 91.801
- type: recall_at_1000
value: 98.578
- type: recall_at_20
value: 83.531
- type: recall_at_3
value: 61.988
- type: recall_at_5
value: 69.868
task:
type: Retrieval
- dataset:
config: default
name: MTEB SpartQA (default)
revision: 9ab3ca3ccdd0d43f9cd6d346a363935d127f4f45
split: test
type: RAR-b/spartqa
metrics:
- type: main_score
value: 15.065999999999999
- type: map_at_1
value: 2.94
- type: map_at_10
value: 9.815999999999999
- type: map_at_100
value: 11.514000000000001
- type: map_at_1000
value: 11.578
- type: map_at_20
value: 11.016
- type: map_at_3
value: 6.734999999999999
- type: map_at_5
value: 8.337
- type: mrr_at_1
value: 5.982192543127435
- type: mrr_at_10
value: 13.466734681258858
- type: mrr_at_100
value: 15.23447840689604
- type: mrr_at_1000
value: 15.301367917746896
- type: mrr_at_20
value: 14.71801235668512
- type: mrr_at_3
value: 9.98423298089408
- type: mrr_at_5
value: 11.894360971990297
- type: ndcg_at_1
value: 5.982
- type: ndcg_at_10
value: 15.065999999999999
- type: ndcg_at_100
value: 22.867
- type: ndcg_at_1000
value: 24.808
- type: ndcg_at_20
value: 19.363
- type: ndcg_at_3
value: 8.397
- type: ndcg_at_5
value: 11.453000000000001
- type: precision_at_1
value: 5.982
- type: precision_at_10
value: 4.413
- type: precision_at_100
value: 0.9809999999999999
- type: precision_at_1000
value: 0.123
- type: precision_at_20
value: 3.415
- type: precision_at_3
value: 5.9270000000000005
- type: precision_at_5
value: 5.659
- type: recall_at_1
value: 2.94
- type: recall_at_10
value: 26.951999999999998
- type: recall_at_100
value: 58.11500000000001
- type: recall_at_1000
value: 71.777
- type: recall_at_20
value: 42.042
- type: recall_at_3
value: 9.915000000000001
- type: recall_at_5
value: 16.814999999999998
task:
type: Retrieval
- dataset:
config: default
name: MTEB StackOverflowQA (default)
revision: db8f169f3894c14a00251061f957b2063eef2bd5
split: test
type: CoIR-Retrieval/stackoverflow-qa
metrics:
- type: main_score
value: 55.907
- type: map_at_1
value: 46.991
- type: map_at_10
value: 52.763000000000005
- type: map_at_100
value: 53.386
- type: map_at_1000
value: 53.432
- type: map_at_20
value: 53.141000000000005
- type: map_at_3
value: 51.044999999999995
- type: map_at_5
value: 51.98500000000001
- type: mrr_at_1
value: 46.99097291875627
- type: mrr_at_10
value: 52.76291175112643
- type: mrr_at_100
value: 53.386278433480506
- type: mrr_at_1000
value: 53.431881088094414
- type: mrr_at_20
value: 53.140779558381865
- type: mrr_at_3
value: 51.04480106987634
- type: mrr_at_5
value: 51.985122032765055
- type: ndcg_at_1
value: 46.991
- type: ndcg_at_10
value: 55.907
- type: ndcg_at_100
value: 59.019
- type: ndcg_at_1000
value: 60.416000000000004
- type: ndcg_at_20
value: 57.269999999999996
- type: ndcg_at_3
value: 52.337
- type: ndcg_at_5
value: 54.053
- type: precision_at_1
value: 46.991
- type: precision_at_10
value: 6.595
- type: precision_at_100
value: 0.807
- type: precision_at_1000
value: 0.092
- type: precision_at_20
value: 3.566
- type: precision_at_3
value: 18.689
- type: precision_at_5
value: 12.056000000000001
- type: recall_at_1
value: 46.991
- type: recall_at_10
value: 65.948
- type: recall_at_100
value: 80.692
- type: recall_at_1000
value: 92.07600000000001
- type: recall_at_20
value: 71.314
- type: recall_at_3
value: 56.068
- type: recall_at_5
value: 60.281
task:
type: Retrieval
- dataset:
config: default
name: MTEB SyntheticText2SQL (default)
revision: 686b87296c3a0191b5d9415a00526c62db9fce09
split: test
type: CoIR-Retrieval/synthetic-text2sql
metrics:
- type: main_score
value: 35.068
- type: map_at_1
value: 2.547
- type: map_at_10
value: 26.267000000000003
- type: map_at_100
value: 27.162999999999997
- type: map_at_1000
value: 27.229
- type: map_at_20
value: 26.789
- type: map_at_3
value: 23.717
- type: map_at_5
value: 25.237
- type: mrr_at_1
value: 21.329687232951635
- type: mrr_at_10
value: 36.61716352922974
- type: mrr_at_100
value: 37.472046007482554
- type: mrr_at_1000
value: 37.53687081095924
- type: mrr_at_20
value: 37.10337925231122
- type: mrr_at_3
value: 34.30752577906894
- type: mrr_at_5
value: 35.66712242921432
- type: ndcg_at_1
value: 2.547
- type: ndcg_at_10
value: 35.068
- type: ndcg_at_100
value: 39.708
- type: ndcg_at_1000
value: 41.454
- type: ndcg_at_20
value: 36.943
- type: ndcg_at_3
value: 29.837000000000003
- type: ndcg_at_5
value: 32.583
- type: precision_at_1
value: 2.547
- type: precision_at_10
value: 6.165
- type: precision_at_100
value: 0.84
- type: precision_at_1000
value: 0.098
- type: precision_at_20
value: 3.451
- type: precision_at_3
value: 15.769
- type: precision_at_5
value: 10.798
- type: recall_at_1
value: 2.547
- type: recall_at_10
value: 61.648
- type: recall_at_100
value: 84.03699999999999
- type: recall_at_1000
value: 97.77799999999999
- type: recall_at_20
value: 69.014
- type: recall_at_3
value: 47.308
- type: recall_at_5
value: 53.991
task:
type: Retrieval
- dataset:
config: default
name: MTEB TRECCOVID (default)
revision: bb9466bac8153a0349341eb1b22e06409e78ef4e
split: test
type: mteb/trec-covid
metrics:
- type: main_score
value: 44.603
- type: map_at_1
value: 0.128
- type: map_at_10
value: 1.012
- type: map_at_100
value: 4.585999999999999
- type: map_at_1000
value: 11.622
- type: map_at_20
value: 1.611
- type: map_at_3
value: 0.35500000000000004
- type: map_at_5
value: 0.586
- type: mrr_at_1
value: 50.0
- type: mrr_at_10
value: 61.15555555555555
- type: mrr_at_100
value: 61.673274833274824
- type: mrr_at_1000
value: 61.70384154456443
- type: mrr_at_20
value: 61.43174603174602
- type: mrr_at_3
value: 59.33333333333333
- type: mrr_at_5
value: 60.73333333333333
- type: ndcg_at_1
value: 45.0
- type: ndcg_at_10
value: 44.603
- type: ndcg_at_100
value: 32.218
- type: ndcg_at_1000
value: 28.721999999999998
- type: ndcg_at_20
value: 40.752
- type: ndcg_at_3
value: 45.641999999999996
- type: ndcg_at_5
value: 45.903
- type: precision_at_1
value: 50.0
- type: precision_at_10
value: 48.4
- type: precision_at_100
value: 33.339999999999996
- type: precision_at_1000
value: 13.794
- type: precision_at_20
value: 43.3
- type: precision_at_3
value: 51.333
- type: precision_at_5
value: 51.6
- type: recall_at_1
value: 0.128
- type: recall_at_10
value: 1.226
- type: recall_at_100
value: 7.185999999999999
- type: recall_at_1000
value: 27.279999999999998
- type: recall_at_20
value: 2.088
- type: recall_at_3
value: 0.40299999999999997
- type: recall_at_5
value: 0.69
task:
type: Retrieval
- dataset:
config: default
name: MTEB TempReasonL1 (default)
revision: 9097e99aa8c9d827189c65f2e11bfe756af439f6
split: test
type: RAR-b/TempReason-l1
metrics:
- type: main_score
value: 1.8399999999999999
- type: map_at_1
value: 0.0
- type: map_at_10
value: 1.0370000000000001
- type: map_at_100
value: 1.275
- type: map_at_1000
value: 1.322
- type: map_at_20
value: 1.16
- type: map_at_3
value: 0.512
- type: map_at_5
value: 0.767
- type: mrr_at_1
value: 0.0
- type: mrr_at_10
value: 1.036884920634922
- type: mrr_at_100
value: 1.274587207544468
- type: mrr_at_1000
value: 1.3215562619414125
- type: mrr_at_20
value: 1.1597194843769947
- type: mrr_at_3
value: 0.5125
- type: mrr_at_5
value: 0.7674999999999993
- type: ndcg_at_1
value: 0.0
- type: ndcg_at_10
value: 1.8399999999999999
- type: ndcg_at_100
value: 3.206
- type: ndcg_at_1000
value: 4.7940000000000005
- type: ndcg_at_20
value: 2.2800000000000002
- type: ndcg_at_3
value: 0.7000000000000001
- type: ndcg_at_5
value: 1.167
- type: precision_at_1
value: 0.0
- type: precision_at_10
value: 0.45199999999999996
- type: precision_at_100
value: 0.11399999999999999
- type: precision_at_1000
value: 0.025
- type: precision_at_20
value: 0.313
- type: precision_at_3
value: 0.41700000000000004
- type: precision_at_5
value: 0.48
- type: recall_at_1
value: 0.0
- type: recall_at_10
value: 4.5249999999999995
- type: recall_at_100
value: 11.450000000000001
- type: recall_at_1000
value: 24.75
- type: recall_at_20
value: 6.25
- type: recall_at_3
value: 1.25
- type: recall_at_5
value: 2.4
task:
type: Retrieval
- dataset:
config: default
name: MTEB TempReasonL2Context (default)
revision: f2dc4764024ae93cc42d9c09bc53a31da1af84b2
split: test
type: RAR-b/TempReason-l2-context
metrics:
- type: main_score
value: 10.853
- type: map_at_1
value: 4.317
- type: map_at_10
value: 8.261000000000001
- type: map_at_100
value: 9.324
- type: map_at_1000
value: 9.443
- type: map_at_20
value: 8.738999999999999
- type: map_at_3
value: 6.658
- type: map_at_5
value: 7.475
- type: mrr_at_1
value: 4.317213266629609
- type: mrr_at_10
value: 8.260541570713873
- type: mrr_at_100
value: 9.324279575140654
- type: mrr_at_1000
value: 9.44289907211348
- type: mrr_at_20
value: 8.738944029983301
- type: mrr_at_3
value: 6.658019887591863
- type: mrr_at_5
value: 7.475140510159946
- type: ndcg_at_1
value: 4.317
- type: ndcg_at_10
value: 10.853
- type: ndcg_at_100
value: 17.087
- type: ndcg_at_1000
value: 20.593
- type: ndcg_at_20
value: 12.598999999999998
- type: ndcg_at_3
value: 7.467
- type: ndcg_at_5
value: 8.931000000000001
- type: precision_at_1
value: 4.317
- type: precision_at_10
value: 1.934
- type: precision_at_100
value: 0.51
- type: precision_at_1000
value: 0.079
- type: precision_at_20
value: 1.313
- type: precision_at_3
value: 3.273
- type: precision_at_5
value: 2.672
- type: recall_at_1
value: 4.317
- type: recall_at_10
value: 19.344
- type: recall_at_100
value: 50.973
- type: recall_at_1000
value: 79.35900000000001
- type: recall_at_20
value: 26.255
- type: recall_at_3
value: 9.82
- type: recall_at_5
value: 13.358999999999998
task:
type: Retrieval
- dataset:
config: default
name: MTEB TempReasonL2Fact (default)
revision: 13758bcf978613b249d0de4d0840f57815122bdf
split: test
type: RAR-b/TempReason-l2-fact
metrics:
- type: main_score
value: 6.4430000000000005
- type: map_at_1
value: 1.538
- type: map_at_10
value: 4.381
- type: map_at_100
value: 5.583
- type: map_at_1000
value: 5.755
- type: map_at_20
value: 4.888
- type: map_at_3
value: 3.042
- type: map_at_5
value: 3.6929999999999996
- type: mrr_at_1
value: 1.5378914211599035
- type: mrr_at_10
value: 4.380615627141467
- type: mrr_at_100
value: 5.582481340163152
- type: mrr_at_1000
value: 5.7543358690140085
- type: mrr_at_20
value: 4.888341522384855
- type: mrr_at_3
value: 3.041813353097401
- type: mrr_at_5
value: 3.693101105552468
- type: ndcg_at_1
value: 1.538
- type: ndcg_at_10
value: 6.4430000000000005
- type: ndcg_at_100
value: 14.17
- type: ndcg_at_1000
value: 18.682000000000002
- type: ndcg_at_20
value: 8.291
- type: ndcg_at_3
value: 3.5709999999999997
- type: ndcg_at_5
value: 4.749
- type: precision_at_1
value: 1.538
- type: precision_at_10
value: 1.329
- type: precision_at_100
value: 0.541
- type: precision_at_1000
value: 0.09
- type: precision_at_20
value: 1.0290000000000001
- type: precision_at_3
value: 1.7049999999999998
- type: precision_at_5
value: 1.5970000000000002
- type: recall_at_1
value: 1.538
- type: recall_at_10
value: 13.285
- type: recall_at_100
value: 54.14099999999999
- type: recall_at_1000
value: 89.642
- type: recall_at_20
value: 20.586
- type: recall_at_3
value: 5.114
- type: recall_at_5
value: 7.986
task:
type: Retrieval
- dataset:
config: default
name: MTEB TempReasonL2Pure (default)
revision: 27668949b97bfb178901e0cf047cbee805305dc1
split: test
type: RAR-b/TempReason-l2-pure
metrics:
- type: main_score
value: 0.211
- type: map_at_1
value: 0.055999999999999994
- type: map_at_10
value: 0.14100000000000001
- type: map_at_100
value: 0.259
- type: map_at_1000
value: 0.337
- type: map_at_20
value: 0.179
- type: map_at_3
value: 0.10200000000000001
- type: map_at_5
value: 0.11
- type: mrr_at_1
value: 0.055586436909394105
- type: mrr_at_10
value: 0.14145865869045413
- type: mrr_at_100
value: 0.2592058422890182
- type: mrr_at_1000
value: 0.33682005085387395
- type: mrr_at_20
value: 0.17880657618592516
- type: mrr_at_3
value: 0.10190846766722254
- type: mrr_at_5
value: 0.11024643320363164
- type: ndcg_at_1
value: 0.055999999999999994
- type: ndcg_at_10
value: 0.211
- type: ndcg_at_100
value: 1.088
- type: ndcg_at_1000
value: 3.7859999999999996
- type: ndcg_at_20
value: 0.356
- type: ndcg_at_3
value: 0.11800000000000001
- type: ndcg_at_5
value: 0.134
- type: precision_at_1
value: 0.055999999999999994
- type: precision_at_10
value: 0.044000000000000004
- type: precision_at_100
value: 0.053
- type: precision_at_1000
value: 0.027999999999999997
- type: precision_at_20
value: 0.052
- type: precision_at_3
value: 0.055999999999999994
- type: precision_at_5
value: 0.041
- type: recall_at_1
value: 0.055999999999999994
- type: recall_at_10
value: 0.445
- type: recall_at_100
value: 5.299
- type: recall_at_1000
value: 27.979
- type: recall_at_20
value: 1.038
- type: recall_at_3
value: 0.167
- type: recall_at_5
value: 0.20400000000000001
task:
type: Retrieval
- dataset:
config: default
name: MTEB TempReasonL3Context (default)
revision: 3c42539652de3d787cecfb897d3b20905e5c7250
split: test
type: RAR-b/TempReason-l3-context
metrics:
- type: main_score
value: 8.985999999999999
- type: map_at_1
value: 2.915
- type: map_at_10
value: 6.546
- type: map_at_100
value: 7.591
- type: map_at_1000
value: 7.71
- type: map_at_20
value: 7.015000000000001
- type: map_at_3
value: 5.065
- type: map_at_5
value: 5.779999999999999
- type: mrr_at_1
value: 2.914595571622232
- type: mrr_at_10
value: 6.546229351810003
- type: mrr_at_100
value: 7.590639752125733
- type: mrr_at_1000
value: 7.7100662080438696
- type: mrr_at_20
value: 7.014767909905029
- type: mrr_at_3
value: 5.064768790480501
- type: mrr_at_5
value: 5.7798614249133955
- type: ndcg_at_1
value: 2.915
- type: ndcg_at_10
value: 8.985999999999999
- type: ndcg_at_100
value: 15.088
- type: ndcg_at_1000
value: 18.618000000000002
- type: ndcg_at_20
value: 10.708
- type: ndcg_at_3
value: 5.825
- type: ndcg_at_5
value: 7.116
- type: precision_at_1
value: 2.915
- type: precision_at_10
value: 1.699
- type: precision_at_100
value: 0.479
- type: precision_at_1000
value: 0.076
- type: precision_at_20
value: 1.192
- type: precision_at_3
value: 2.681
- type: precision_at_5
value: 2.237
- type: recall_at_1
value: 2.915
- type: recall_at_10
value: 16.991
- type: recall_at_100
value: 47.876000000000005
- type: recall_at_1000
value: 76.48
- type: recall_at_20
value: 23.836
- type: recall_at_3
value: 8.043
- type: recall_at_5
value: 11.184
task:
type: Retrieval
- dataset:
config: default
name: MTEB TempReasonL3Fact (default)
revision: 4b70e90197901da24f3cfcd51d27111292878680
split: test
type: RAR-b/TempReason-l3-fact
metrics:
- type: main_score
value: 6.9239999999999995
- type: map_at_1
value: 0.9490000000000001
- type: map_at_10
value: 4.482
- type: map_at_100
value: 5.673
- type: map_at_1000
value: 5.831
- type: map_at_20
value: 4.97
- type: map_at_3
value: 2.862
- type: map_at_5
value: 3.673
- type: mrr_at_1
value: 0.9489380930863082
- type: mrr_at_10
value: 4.4841269841269815
- type: mrr_at_100
value: 5.672705016400986
- type: mrr_at_1000
value: 5.831192204277269
- type: mrr_at_20
value: 4.970528777573662
- type: mrr_at_3
value: 2.8618767886729892
- type: mrr_at_5
value: 3.6729929206205796
- type: ndcg_at_1
value: 0.9490000000000001
- type: ndcg_at_10
value: 6.9239999999999995
- type: ndcg_at_100
value: 14.408000000000001
- type: ndcg_at_1000
value: 18.734
- type: ndcg_at_20
value: 8.698
- type: ndcg_at_3
value: 3.512
- type: ndcg_at_5
value: 4.978
- type: precision_at_1
value: 0.9490000000000001
- type: precision_at_10
value: 1.496
- type: precision_at_100
value: 0.541
- type: precision_at_1000
value: 0.08800000000000001
- type: precision_at_20
value: 1.098
- type: precision_at_3
value: 1.7999999999999998
- type: precision_at_5
value: 1.794
- type: recall_at_1
value: 0.9490000000000001
- type: recall_at_10
value: 14.957
- type: recall_at_100
value: 54.089
- type: recall_at_1000
value: 88.47699999999999
- type: recall_at_20
value: 21.961
- type: recall_at_3
value: 5.4
- type: recall_at_5
value: 8.97
task:
type: Retrieval
- dataset:
config: default
name: MTEB TempReasonL3Pure (default)
revision: 68fba138e7e63daccecfbdad0a9d2714e56e34ff
split: test
type: RAR-b/TempReason-l3-pure
metrics:
- type: main_score
value: 3.9510000000000005
- type: map_at_1
value: 0.045
- type: map_at_10
value: 2.464
- type: map_at_100
value: 3.159
- type: map_at_1000
value: 3.2649999999999997
- type: map_at_20
value: 2.7439999999999998
- type: map_at_3
value: 1.5779999999999998
- type: map_at_5
value: 2.075
- type: mrr_at_1
value: 0.045187528242205156
- type: mrr_at_10
value: 2.4634465173326463
- type: mrr_at_100
value: 3.1587507355194098
- type: mrr_at_1000
value: 3.2645570477304884
- type: mrr_at_20
value: 2.7446874866280555
- type: mrr_at_3
value: 1.5777978611236638
- type: mrr_at_5
value: 2.07486067178792
- type: ndcg_at_1
value: 0.045
- type: ndcg_at_10
value: 3.9510000000000005
- type: ndcg_at_100
value: 8.116
- type: ndcg_at_1000
value: 11.599
- type: ndcg_at_20
value: 4.977
- type: ndcg_at_3
value: 2.11
- type: ndcg_at_5
value: 3.005
- type: precision_at_1
value: 0.045
- type: precision_at_10
value: 0.877
- type: precision_at_100
value: 0.3
- type: precision_at_1000
value: 0.059000000000000004
- type: precision_at_20
value: 0.642
- type: precision_at_3
value: 1.22
- type: precision_at_5
value: 1.166
- type: recall_at_1
value: 0.045
- type: recall_at_10
value: 8.766
- type: recall_at_100
value: 30.005
- type: recall_at_1000
value: 58.925000000000004
- type: recall_at_20
value: 12.833
- type: recall_at_3
value: 3.66
- type: recall_at_5
value: 5.829
task:
type: Retrieval
- dataset:
config: default
name: MTEB TopiOCQAHardNegatives (default)
revision: b4cc09fb8bb3a9e0ce0f94dc69c96397a2a47c18
split: validation
type: mteb/TopiOCQA_validation_top_250_only_w_correct-v2
metrics:
- type: main_score
value: 10.59
- type: map_at_1
value: 4.3
- type: map_at_10
value: 8.134
- type: map_at_100
value: 8.967
- type: map_at_1000
value: 9.154
- type: map_at_20
value: 8.498999999999999
- type: map_at_3
value: 6.550000000000001
- type: map_at_5
value: 7.385
- type: mrr_at_1
value: 4.3
- type: mrr_at_10
value: 8.133968253968261
- type: mrr_at_100
value: 8.966515392145464
- type: mrr_at_1000
value: 9.153606984319149
- type: mrr_at_20
value: 8.49921878517081
- type: mrr_at_3
value: 6.550000000000002
- type: mrr_at_5
value: 7.385000000000004
- type: ndcg_at_1
value: 4.3
- type: ndcg_at_10
value: 10.59
- type: ndcg_at_100
value: 15.728
- type: ndcg_at_1000
value: 21.025
- type: ndcg_at_20
value: 11.944
- type: ndcg_at_3
value: 7.282
- type: ndcg_at_5
value: 8.797
- type: precision_at_1
value: 4.3
- type: precision_at_10
value: 1.8599999999999999
- type: precision_at_100
value: 0.45199999999999996
- type: precision_at_1000
value: 0.087
- type: precision_at_20
value: 1.2
- type: precision_at_3
value: 3.1329999999999996
- type: precision_at_5
value: 2.62
- type: recall_at_1
value: 4.3
- type: recall_at_10
value: 18.6
- type: recall_at_100
value: 45.2
- type: recall_at_1000
value: 87.4
- type: recall_at_20
value: 24.0
- type: recall_at_3
value: 9.4
- type: recall_at_5
value: 13.100000000000001
task:
type: Retrieval
- dataset:
config: default
name: MTEB Touche2020 (default)
revision: a34f9a33db75fa0cbb21bb5cfc3dae8dc8bec93f
split: test
type: mteb/touche2020
metrics:
- type: main_score
value: 22.398
- type: map_at_1
value: 2.067
- type: map_at_10
value: 8.579
- type: map_at_100
value: 15.012
- type: map_at_1000
value: 16.706
- type: map_at_20
value: 10.653
- type: map_at_3
value: 3.909
- type: map_at_5
value: 6.077
- type: mrr_at_1
value: 28.57142857142857
- type: mrr_at_10
value: 43.01830255911888
- type: mrr_at_100
value: 43.96547082263703
- type: mrr_at_1000
value: 43.96547082263703
- type: mrr_at_20
value: 43.71604198403339
- type: mrr_at_3
value: 37.41496598639456
- type: mrr_at_5
value: 41.496598639455776
- type: ndcg_at_1
value: 24.490000000000002
- type: ndcg_at_10
value: 22.398
- type: ndcg_at_100
value: 36.604
- type: ndcg_at_1000
value: 48.111
- type: ndcg_at_20
value: 23.369999999999997
- type: ndcg_at_3
value: 21.378
- type: ndcg_at_5
value: 23.685000000000002
- type: precision_at_1
value: 28.571
- type: precision_at_10
value: 21.224
- type: precision_at_100
value: 8.408
- type: precision_at_1000
value: 1.59
- type: precision_at_20
value: 16.735
- type: precision_at_3
value: 23.128999999999998
- type: precision_at_5
value: 26.122
- type: recall_at_1
value: 2.067
- type: recall_at_10
value: 15.182
- type: recall_at_100
value: 50.768
- type: recall_at_1000
value: 86.29299999999999
- type: recall_at_20
value: 22.32
- type: recall_at_3
value: 4.865
- type: recall_at_5
value: 9.24
task:
type: Retrieval
- dataset:
config: default
name: MTEB Touche2020Retrieval.v3 (default)
revision: 431886eaecc48f067a3975b70d0949ea2862463c
split: test
type: mteb/webis-touche2020-v3
metrics:
- type: main_score
value: 52.575
- type: map_at_1
value: 2.026
- type: map_at_10
value: 15.136
- type: map_at_100
value: 31.539
- type: map_at_1000
value: 34.672
- type: map_at_20
value: 21.477
- type: map_at_3
value: 5.931
- type: map_at_5
value: 9.476999999999999
- type: mrr_at_1
value: 63.26530612244898
- type: mrr_at_10
value: 77.57045675413023
- type: mrr_at_100
value: 77.75598551108757
- type: mrr_at_1000
value: 77.75598551108757
- type: mrr_at_20
value: 77.75598551108757
- type: mrr_at_3
value: 75.85034013605441
- type: mrr_at_5
value: 77.27891156462586
- type: ndcg_at_1
value: 54.081999999999994
- type: ndcg_at_10
value: 52.575
- type: ndcg_at_100
value: 55.051
- type: ndcg_at_1000
value: 67.027
- type: ndcg_at_20
value: 46.561
- type: ndcg_at_3
value: 58.48799999999999
- type: ndcg_at_5
value: 57.115
- type: precision_at_1
value: 63.26500000000001
- type: precision_at_10
value: 56.531
- type: precision_at_100
value: 18.898
- type: precision_at_1000
value: 3.084
- type: precision_at_20
value: 44.082
- type: precision_at_3
value: 68.027
- type: precision_at_5
value: 65.714
- type: recall_at_1
value: 2.026
- type: recall_at_10
value: 19.494
- type: recall_at_100
value: 59.349
- type: recall_at_1000
value: 89.84
- type: recall_at_20
value: 29.953000000000003
- type: recall_at_3
value: 6.819999999999999
- type: recall_at_5
value: 11.386000000000001
task:
type: Retrieval
- dataset:
config: default
name: MTEB WinoGrande (default)
revision: f74c094f321077cf909ddfb8bccc1b5912a4ac28
split: test
type: RAR-b/winogrande
metrics:
- type: main_score
value: 61.800999999999995
- type: map_at_1
value: 29.044999999999998
- type: map_at_10
value: 51.514
- type: map_at_100
value: 51.896
- type: map_at_1000
value: 51.897000000000006
- type: map_at_20
value: 51.842
- type: map_at_3
value: 46.803
- type: map_at_5
value: 49.957
- type: mrr_at_1
value: 29.36069455406472
- type: mrr_at_10
value: 51.617619423460056
- type: mrr_at_100
value: 52.01415572431666
- type: mrr_at_1000
value: 52.014274589675495
- type: mrr_at_20
value: 51.95996098874236
- type: mrr_at_3
value: 46.86924493554325
- type: mrr_at_5
value: 50.04209418574064
- type: ndcg_at_1
value: 29.044999999999998
- type: ndcg_at_10
value: 61.800999999999995
- type: ndcg_at_100
value: 63.315
- type: ndcg_at_1000
value: 63.324000000000005
- type: ndcg_at_20
value: 62.986
- type: ndcg_at_3
value: 52.525
- type: ndcg_at_5
value: 58.160999999999994
- type: precision_at_1
value: 29.044999999999998
- type: precision_at_10
value: 9.361
- type: precision_at_100
value: 0.9990000000000001
- type: precision_at_1000
value: 0.1
- type: precision_at_20
value: 4.913
- type: precision_at_3
value: 23.02
- type: precision_at_5
value: 16.527
- type: recall_at_1
value: 29.044999999999998
- type: recall_at_10
value: 93.607
- type: recall_at_100
value: 99.921
- type: recall_at_1000
value: 100.0
- type: recall_at_20
value: 98.264
- type: recall_at_3
value: 69.06099999999999
- type: recall_at_5
value: 82.636
task:
type: Retrieval
---
# Static Embeddings with BERT uncased tokenizer finetuned on various datasets
This is a [sentence-transformers](https://www.SBERT.net) model trained on the [gooaq](https://huggingface.co./datasets/sentence-transformers/gooaq), [msmarco](https://huggingface.co./datasets/sentence-transformers/msmarco-co-condenser-margin-mse-sym-mnrl-mean-v1), [squad](https://huggingface.co./datasets/sentence-transformers/squad), [s2orc](https://huggingface.co./datasets/sentence-transformers/s2orc), [allnli](https://huggingface.co./datasets/sentence-transformers/all-nli), [paq](https://huggingface.co./datasets/sentence-transformers/paq), [trivia_qa](https://huggingface.co./datasets/sentence-transformers/trivia-qa), [msmarco_10m](https://huggingface.co./datasets/bclavie/msmarco-10m-triplets), [swim_ir](https://huggingface.co./datasets/nthakur/swim-ir-monolingual), [pubmedqa](https://huggingface.co./datasets/sentence-transformers/pubmedqa), [miracl](https://huggingface.co./datasets/sentence-transformers/miracl), [mldr](https://huggingface.co./datasets/sentence-transformers/mldr) and [mr_tydi](https://huggingface.co./datasets/sentence-transformers/mr-tydi) datasets. It maps sentences & paragraphs to a 1024-dimensional dense vector space and is designed to be used for semantic search.
Read our [Static Embeddings blogpost](https://huggingface.co./blog/static-embeddings) to learn more about this model and how it was trained.
* **0 Active Parameters:** This model does not use any active parameters, instead consisting exclusively of averaging pre-computed token embeddings.
* **100x to 400x faster:** On CPU, this model is 100x to 400x faster than common options like [all-mpnet-base-v2](https://huggingface.co./sentence-transformers/all-mpnet-base-v2). On GPU, it's 10x to 25x faster.
* **Matryoshka:** This model was trained with a [Matryoshka loss](https://huggingface.co./blog/matryoshka), allowing you to truncate the embeddings for faster retrieval at minimal performance costs.
* **Evaluations:** See [Evaluations](#evaluation) for details on performance on NanoBEIR, embedding speed, and Matryoshka dimensionality truncation. In short, this model is **87.4%** as performant as the commonly used [`all-mpnet-base-v2`](https://huggingface.co./sentence-transformers/all-mpnet-base-v2).
* **Training Script:** See [train.py](train.py) for the training script used to train this model from scratch.
See [`static-similarity-mrl-multilingual-v1`](https://huggingface.co./sentence-transformers/static-similarity-mrl-multilingual-v1) for a general-purpose multilingual static embedding model. It's been trained for semantic textual similarity, paraphrase mining, text classification, clustering, and more.
## Model Details
### Model Description
- **Model Type:** Sentence Transformer
- **Maximum Sequence Length:** inf tokens
- **Output Dimensionality:** 1024 tokens
- **Similarity Function:** Cosine Similarity
- **Training Datasets:**
- [gooaq](https://huggingface.co./datasets/sentence-transformers/gooaq)
- [msmarco](https://huggingface.co./datasets/sentence-transformers/msmarco-co-condenser-margin-mse-sym-mnrl-mean-v1)
- [squad](https://huggingface.co./datasets/sentence-transformers/squad)
- [s2orc](https://huggingface.co./datasets/sentence-transformers/s2orc)
- [allnli](https://huggingface.co./datasets/sentence-transformers/all-nli)
- [paq](https://huggingface.co./datasets/sentence-transformers/paq)
- [trivia_qa](https://huggingface.co./datasets/sentence-transformers/trivia-qa)
- [msmarco_10m](https://huggingface.co./datasets/bclavie/msmarco-10m-triplets)
- [swim_ir](https://huggingface.co./datasets/nthakur/swim-ir-monolingual)
- [pubmedqa](https://huggingface.co./datasets/sentence-transformers/pubmedqa)
- [miracl](https://huggingface.co./datasets/sentence-transformers/miracl)
- [mldr](https://huggingface.co./datasets/sentence-transformers/mldr)
- [mr_tydi](https://huggingface.co./datasets/sentence-transformers/mr-tydi)
- **Language:** en
- **License:** apache-2.0
### Model Sources
- **Documentation:** [Sentence Transformers Documentation](https://sbert.net)
- **Repository:** [Sentence Transformers on GitHub](https://github.com/UKPLab/sentence-transformers)
- **Hugging Face:** [Sentence Transformers on Hugging Face](https://huggingface.co./models?library=sentence-transformers)
### Full Model Architecture
```
SentenceTransformer(
(0): StaticEmbedding(
(embedding): EmbeddingBag(30522, 1024, mode='mean')
)
)
```
## Usage
### Direct Usage (Sentence Transformers)
First install the Sentence Transformers library:
```bash
pip install -U sentence-transformers
```
Then you can load this model and run inference.
```python
from sentence_transformers import SentenceTransformer
# Download from the 🤗 Hub
model = SentenceTransformer("tomaarsen/static-retrieval-mrl-en-v1")
# Run inference
sentences = [
'Gadofosveset-enhanced MR angiography of carotid arteries: does steady-state imaging improve accuracy of first-pass imaging?',
'To evaluate the diagnostic accuracy of gadofosveset-enhanced magnetic resonance (MR) angiography in the assessment of carotid artery stenosis, with digital subtraction angiography (DSA) as the reference standard, and to determine the value of reading first-pass, steady-state, and "combined" (first-pass plus steady-state) MR angiograms.',
'In a longitudinal study we investigated in vivo alterations of CVO during neuroinflammation, applying Gadofluorine M- (Gf) enhanced magnetic resonance imaging (MRI) in experimental autoimmune encephalomyelitis, an animal model of multiple sclerosis. SJL/J mice were monitored by Gadopentate dimeglumine- (Gd-DTPA) and Gf-enhanced MRI after adoptive transfer of proteolipid-protein-specific T cells. Mean Gf intensity ratios were calculated individually for different CVO and correlated to the clinical disease course. Subsequently, the tissue distribution of fluorescence-labeled Gf as well as the extent of cellular inflammation was assessed in corresponding histological slices.',
]
embeddings = model.encode(sentences)
print(embeddings.shape)
# [3, 1024]
# Get the similarity scores for the embeddings
similarities = model.similarity(embeddings, embeddings)
print(similarities.shape)
# [3, 3]
```
This model was trained with Matryoshka loss, allowing this model to be used with lower dimensionalities with minimal performance loss (See [Matryoshka Evaluations](#matryoshka-evaluations) for evaluations).
Notably, a lower dimensionality allows for much faster and cheaper information retrieval. You can specify a lower dimensionality with the `truncate_dim` argument when initializing the Sentence Transformer model:
```python
from sentence_transformers import SentenceTransformer
model = SentenceTransformer("tomaarsen/static-retrieval-mrl-en-v1", truncate_dim=256)
embeddings = model.encode([
"what is the difference between chronological order and spatial order?",
"can lavender grow indoors?"
])
print(embeddings.shape)
# => (2, 256)
```
## Evaluation
### Metrics
#### Information Retrieval
* Datasets: `NanoClimateFEVER`, `NanoDBPedia`, `NanoFEVER`, `NanoFiQA2018`, `NanoHotpotQA`, `NanoMSMARCO`, `NanoNFCorpus`, `NanoNQ`, `NanoQuoraRetrieval`, `NanoSCIDOCS`, `NanoArguAna`, `NanoSciFact` and `NanoTouche2020`
* Evaluated with [InformationRetrievalEvaluator
](https://sbert.net/docs/package_reference/sentence_transformer/evaluation.html#sentence_transformers.evaluation.InformationRetrievalEvaluator)
| Metric | NanoClimateFEVER | NanoDBPedia | NanoFEVER | NanoFiQA2018 | NanoHotpotQA | NanoMSMARCO | NanoNFCorpus | NanoNQ | NanoQuoraRetrieval | NanoSCIDOCS | NanoArguAna | NanoSciFact | NanoTouche2020 |
|:--------------------|:-----------------|:------------|:-----------|:-------------|:-------------|:------------|:-------------|:-----------|:-------------------|:------------|:------------|:------------|:---------------|
| cosine_accuracy@1 | 0.32 | 0.7 | 0.46 | 0.28 | 0.64 | 0.18 | 0.42 | 0.24 | 0.8 | 0.28 | 0.1 | 0.52 | 0.5714 |
| cosine_accuracy@3 | 0.52 | 0.84 | 0.8 | 0.44 | 0.82 | 0.42 | 0.56 | 0.44 | 0.96 | 0.48 | 0.46 | 0.6 | 0.898 |
| cosine_accuracy@5 | 0.6 | 0.9 | 0.84 | 0.54 | 0.86 | 0.5 | 0.62 | 0.58 | 1.0 | 0.54 | 0.56 | 0.62 | 0.9796 |
| cosine_accuracy@10 | 0.78 | 0.94 | 0.94 | 0.64 | 0.96 | 0.66 | 0.72 | 0.7 | 1.0 | 0.7 | 0.74 | 0.76 | 1.0 |
| cosine_precision@1 | 0.32 | 0.7 | 0.46 | 0.28 | 0.64 | 0.18 | 0.42 | 0.24 | 0.8 | 0.28 | 0.1 | 0.52 | 0.5714 |
| cosine_precision@3 | 0.1933 | 0.5867 | 0.2667 | 0.1933 | 0.3733 | 0.14 | 0.3733 | 0.1467 | 0.3867 | 0.2267 | 0.1533 | 0.2067 | 0.6054 |
| cosine_precision@5 | 0.14 | 0.544 | 0.18 | 0.16 | 0.26 | 0.1 | 0.32 | 0.124 | 0.248 | 0.188 | 0.112 | 0.132 | 0.6204 |
| cosine_precision@10 | 0.104 | 0.452 | 0.1 | 0.104 | 0.148 | 0.066 | 0.244 | 0.076 | 0.13 | 0.14 | 0.074 | 0.084 | 0.5306 |
| cosine_recall@1 | 0.1467 | 0.0805 | 0.4367 | 0.1519 | 0.32 | 0.18 | 0.0428 | 0.24 | 0.7107 | 0.0597 | 0.1 | 0.485 | 0.0398 |
| cosine_recall@3 | 0.239 | 0.1605 | 0.7467 | 0.2983 | 0.56 | 0.42 | 0.0984 | 0.43 | 0.9253 | 0.1417 | 0.46 | 0.57 | 0.1236 |
| cosine_recall@5 | 0.279 | 0.218 | 0.8033 | 0.3793 | 0.65 | 0.5 | 0.1196 | 0.58 | 0.9627 | 0.1947 | 0.56 | 0.595 | 0.2095 |
| cosine_recall@10 | 0.4197 | 0.3143 | 0.9033 | 0.4838 | 0.74 | 0.66 | 0.1389 | 0.69 | 0.9793 | 0.2887 | 0.74 | 0.75 | 0.337 |
| **cosine_ndcg@10** | **0.3309** | **0.5681** | **0.6922** | **0.3651** | **0.6547** | **0.4041** | **0.3242** | **0.4534** | **0.8951** | **0.2643** | **0.4078** | **0.6111** | **0.5703** |
| cosine_mrr@10 | 0.4453 | 0.7854 | 0.6397 | 0.3915 | 0.7485 | 0.3245 | 0.5041 | 0.3764 | 0.88 | 0.3998 | 0.3034 | 0.5837 | 0.744 |
| cosine_map@100 | 0.2598 | 0.4335 | 0.6205 | 0.3024 | 0.5798 | 0.3389 | 0.1449 | 0.389 | 0.8594 | 0.205 | 0.3151 | 0.5683 | 0.447 |
#### Nano BEIR
* Dataset: `NanoBEIR_mean`
* Evaluated with [NanoBEIREvaluator
](https://sbert.net/docs/package_reference/sentence_transformer/evaluation.html#sentence_transformers.evaluation.NanoBEIREvaluator)
| Metric | Value |
|:--------------------|:-----------|
| cosine_accuracy@1 | 0.424 |
| cosine_accuracy@3 | 0.6337 |
| cosine_accuracy@5 | 0.703 |
| cosine_accuracy@10 | 0.8108 |
| cosine_precision@1 | 0.424 |
| cosine_precision@3 | 0.2963 |
| cosine_precision@5 | 0.2406 |
| cosine_precision@10 | 0.1733 |
| cosine_recall@1 | 0.2303 |
| cosine_recall@3 | 0.398 |
| cosine_recall@5 | 0.4655 |
| cosine_recall@10 | 0.5727 |
| **cosine_ndcg@10** | **0.5032** |
| cosine_mrr@10 | 0.5482 |
| cosine_map@100 | 0.4203 |
We've evaluated [sentence-transformers/static-retrieval-mrl-en-v1](https://huggingface.co./sentence-transformers/static-retrieval-mrl-en-v1) on NanoBEIR and plotted it against the inference speed computed on a RTX 3090 and i7-13700K. For the inference speed tests, we calculated the number of computed query embeddings of the [GooAQ dataset](https://huggingface.co./datasets/sentence-transformers/gooaq) per second, either on CPU or GPU.
We evaluate against 3 types of models:
1. Attention-based dense embedding models, e.g. traditional Sentence Transformer models like [`all-mpnet-base-v2`](https://huggingface.co./sentence-transformers/all-mpnet-base-v2), [`bge-base-en-v1.5`](https://huggingface.co./BAAI/bge-base-en-v1.5), and [`gte-large-en-v1.5`](https://huggingface.co./Alibaba-NLP/gte-large-en-v1.5).
2. Static Embedding-based models, e.g. [`static-retrieval-mrl-en-v1`](https://huggingface.co./sentence-transformers/static-retrieval-mrl-en-v1), [`potion-base-8M`](https://huggingface.co./minishlab/potion-base-8M), [`M2V_base_output`](https://huggingface.co./minishlab/M2V_base_output), and [`glove.6B.300d`](https://huggingface.co./sentence-transformers/average_word_embeddings_glove.6B.300d).
3. Sparse bag-of-words model, BM25, often a strong baseline.
Click to expand BM25 implementation details
I relied on the highly efficient [bm25s](https://github.com/xhluca/bm25s) implementation, using `model.get_scores()` on tokens after tokenization and stemming with the English `PyStemmer`.
> **NOTE:** Many of the attention-based dense embedding models are finetuned on the training splits of the (Nano)BEIR evaluation datasets. This gives the models an unfair advantage in this benchmark and can result in lower downstream performance on real retrieval tasks.
>
> [static-retrieval-mrl-en-v1](https://huggingface.co./sentence-transformers/static-retrieval-mrl-en-v1) is purposefully not trained on any of these datasets.
##### GPU
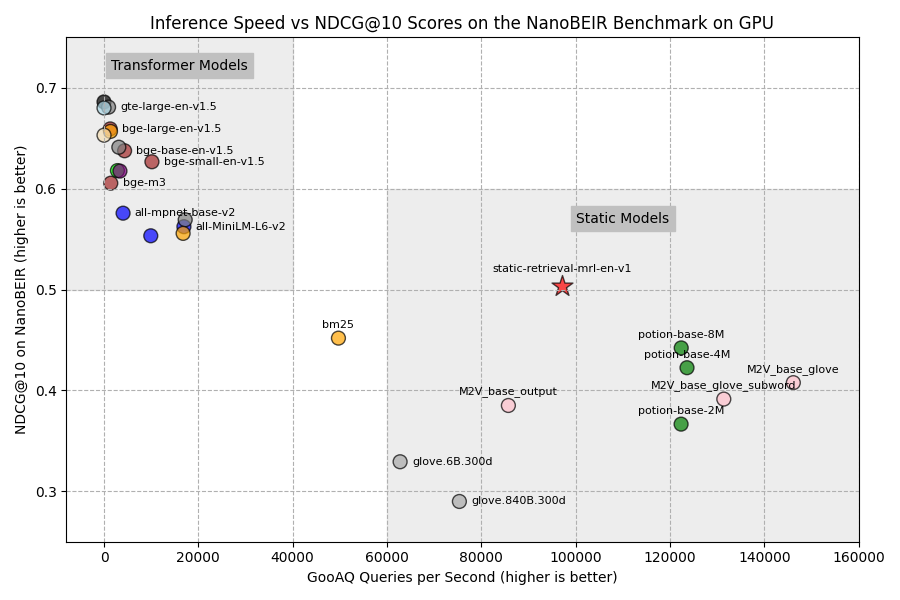
##### CPU
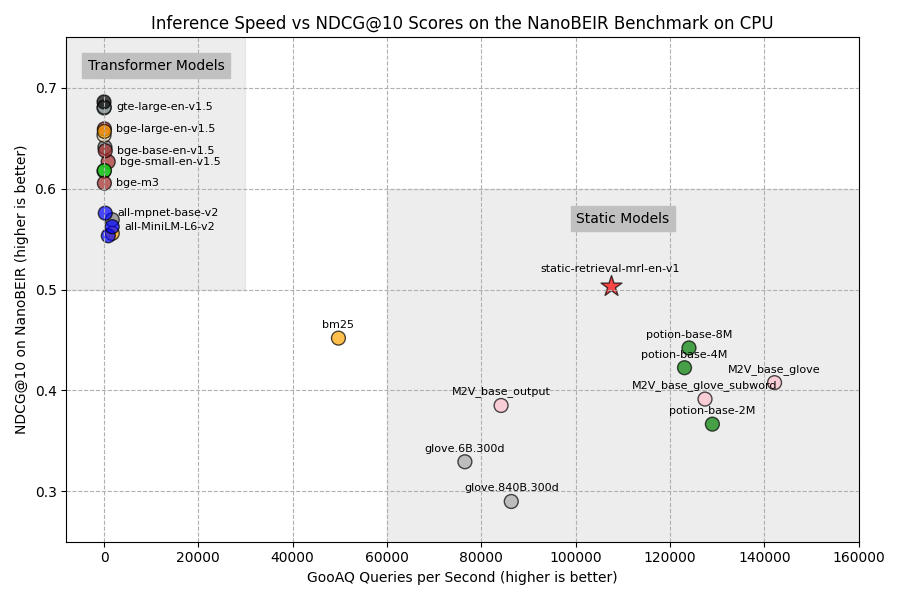
We can draw some notable conclusions from these figures:
1. [`static-retrieval-mrl-en-v1`](https://huggingface.co./sentence-transformers/static-retrieval-mrl-en-v1) outperforms all other Static Embedding models.
2. [`static-retrieval-mrl-en-v1`](https://huggingface.co./sentence-transformers/static-retrieval-mrl-en-v1) is the only Static Embedding model to outperform BM25.
3. [`static-retrieval-mrl-en-v1`](https://huggingface.co./sentence-transformers/static-retrieval-mrl-en-v1) is
* **87.4%** as performant as the commonly used [`all-mpnet-base-v2`](https://huggingface.co./sentence-transformers/all-mpnet-base-v2),
* **24x** faster on GPU,
* **397x** faster on CPU.
4. [`static-retrieval-mrl-en-v1`](https://huggingface.co./sentence-transformers/static-retrieval-mrl-en-v1) is quicker on CPU than on GPU: This model can run extraordinarily quickly everywhere, including consumer-grade PCs, tiny servers, phones, or in-browser.
#### Matryoshka Evaluations
We experimented with the results on NanoBEIR performance when we performed Matryoshka-style dimensionality reduction by truncating the output embeddings to a lower dimensionality.
| Dimensionality | NanoBEIR_mean | NanoArguAna | NanoClimateFEVER | NanoDBPedia | NanoFEVER | NanoFiQA2018 | NanoHotpotQA | NanoMSMARCO | NanoNFCorpus | NanoNQ | NanoQuoraRetrieval | NanoSCIDOCS | NanoSciFact | NanoTouche2020 |
|----------------|---------------|-------------|------------------|-------------|-----------|--------------|--------------|-------------|--------------|--------|--------------------|-------------|-------------|----------------|
| 1024 | **0.5031** | 0.4077 | 0.3308 | 0.5681 | 0.6921 | 0.3651 | 0.6547 | 0.4040 | 0.3241 | 0.4533 | 0.8950 | 0.2642 | 0.6111 | 0.5702 |
| 512 | **0.4957** | 0.3878 | 0.3360 | 0.5626 | 0.6945 | 0.3517 | 0.6280 | 0.3892 | 0.3206 | 0.4505 | 0.8986 | 0.2657 | 0.5953 | 0.5635 |
| 256 | **0.4819** | 0.3855 | 0.3203 | 0.5407 | 0.6734 | 0.3518 | 0.6027 | 0.4144 | 0.2860 | 0.4254 | 0.8948 | 0.2466 | 0.5620 | 0.5605 |
| 128 | **0.4622** | 0.4001 | 0.2982 | 0.5266 | 0.6273 | 0.3188 | 0.5606 | 0.4025 | 0.2693 | 0.4021 | 0.8930 | 0.2283 | 0.5447 | 0.5368 |
| 64 | **0.4176** | 0.3424 | 0.2809 | 0.5022 | 0.5480 | 0.2831 | 0.4680 | 0.3739 | 0.2153 | 0.3845 | 0.8525 | 0.1680 | 0.5045 | 0.5050 |
| 32 | **0.3532** | 0.2866 | 0.1870 | 0.4292 | 0.4193 | 0.2292 | 0.3602 | 0.3587 | 0.1444 | 0.3525 | 0.8325 | 0.1525 | 0.3983 | 0.4408 |
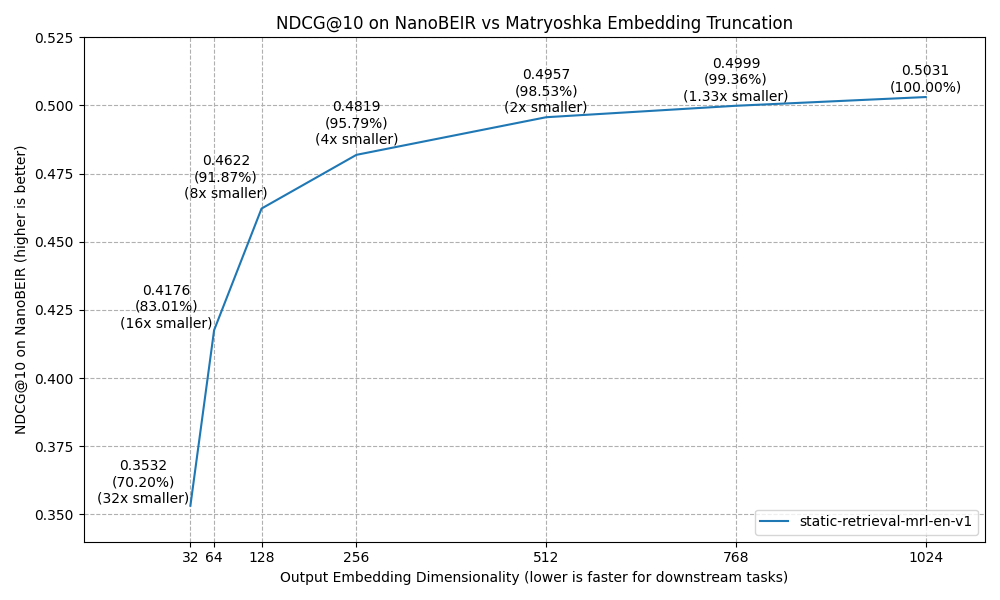
These findings show that reducing the dimensionality by e.g. 2x only has a 1.47% reduction in performance (0.5031 NDCG@10 vs 0.4957 NDCG@10), while realistically resulting in a 2x speedup in retrieval speed.
## Training Details
### Training Datasets
gooaq
* Dataset: [gooaq](https://huggingface.co./datasets/sentence-transformers/gooaq) at [b089f72](https://huggingface.co./datasets/sentence-transformers/gooaq/tree/b089f728748a068b7bc5234e5bcf5b25e3c8279c)
* Size: 3,012,496 training samples
* Columns: question
and answer
* Approximate statistics based on the first 1000 samples:
| | question | answer |
|:--------|:-----------------------------------------------------------------------------------------------|:-------------------------------------------------------------------------------------------------|
| type | string | string |
| details | - min: 18 characters
- mean: 43.23 characters
- max: 96 characters
| - min: 55 characters
- mean: 253.36 characters
- max: 371 characters
|
* Samples:
| question | answer |
|:-----------------------------------------------------------------------------------|:----------------------------------------------------------------------------------------------------------------------------------------------------------------------------------------------------------------------------------------------------------------------------------------------------------------------|
| what is the difference between broilers and layers?
| An egg laying poultry is called egger or layer whereas broilers are reared for obtaining meat. So a layer should be able to produce more number of large sized eggs, without growing too much. On the other hand, a broiler should yield more meat and hence should be able to grow well.
|
| what is the difference between chronological order and spatial order?
| As a writer, you should always remember that unlike chronological order and the other organizational methods for data, spatial order does not take into account the time. Spatial order is primarily focused on the location. All it does is take into account the location of objects and not the time.
|
| is kamagra same as viagra?
| Kamagra is thought to contain the same active ingredient as Viagra, sildenafil citrate. In theory, it should work in much the same way as Viagra, taking about 45 minutes to take effect, and lasting for around 4-6 hours. However, this will vary from person to person.
|
* Loss: [MatryoshkaLoss
](https://sbert.net/docs/package_reference/sentence_transformer/losses.html#matryoshkaloss) with these parameters:
```json
{
"loss": "MultipleNegativesRankingLoss",
"matryoshka_dims": [
1024,
512,
256,
128,
64,
32
],
"matryoshka_weights": [
1,
1,
1,
1,
1,
1
],
"n_dims_per_step": -1
}
```
msmarco
* Dataset: [msmarco](https://huggingface.co./datasets/sentence-transformers/msmarco-co-condenser-margin-mse-sym-mnrl-mean-v1) at [84ed2d3](https://huggingface.co./datasets/sentence-transformers/msmarco-co-condenser-margin-mse-sym-mnrl-mean-v1/tree/84ed2d35626f617d890bd493b4d6db69a741e0e2)
* Size: 502,939 training samples
* Columns: query
, positive
, and negative
* Approximate statistics based on the first 1000 samples:
| | query | positive | negative |
|:--------|:------------------------------------------------------------------------------------------------|:--------------------------------------------------------------------------------------------------|:-------------------------------------------------------------------------------------------------|
| type | string | string | string |
| details | - min: 11 characters
- mean: 33.26 characters
- max: 197 characters
| - min: 96 characters
- mean: 356.24 characters
- max: 1006 characters
| - min: 68 characters
- mean: 327.52 characters
- max: 995 characters
|
* Samples:
| query | positive | negative |
|:---------------------------------------------|:---------------------------------------------------------------------------------------------------------------------------------------------------------------------------------------------------------------------------------------------------------------------------------------------------------------------------------------|:-----------------------------------------------------------------------------------------------------------------------------------------------------------------------------------------------------------------------------------------------------------------------------------------------------------------------------------------------|
| when was the sullivan acts
| Sullivan Act Tim Sullivan, a major Irish criminal passed the Sullivan Act in 1911 to help his constituents rob strangers or to help them against Italian incomers. That is the crux of story that goes with a very early gun control law.
| Sullivan Act Tim Sullivan, a major Irish criminal passed the Sullivan Act in 1911 to help his constituents rob strangers or to help them against Italian incomers. That is the crux of story that goes with a very early gun control law.
|
| can lavender grow indoors
| Growing Lavender Indoors. People ALWAYS ask if you can grow lavender indoors. Well, you can, but most Lavender does best outside. Here is our winter experiment to show you what it would look like. This is one of our 4 Lavender Babies from Fall 2010. Our test specimen is L. x intermedia 'Grosso'.
| Lavender can be grown indoors with a bit of effort to keep it in the conditions it loves to thrive. First off begin with choosing a variety that is better able to tolerate the conditions inside a home. To successfully grow Lavender indoors you need to create optimal growing conditions which is hard to do inside a house.
|
| what kind of barley do you malt
| Barley is a wonderfully versatile cereal grain with a rich nutlike flavor and an appealing chewy, pasta-like consistency. Its appearance resembles wheat berries, although it is slightly lighter in color. Sprouted barley is naturally high in maltose, a sugar that serves as the basis for both malt syrup sweetener.
| Specialty grains that can be used in this way are usually barley, malted or unmalted, that has been treated differently at the malting company. Crystal malt is one of the specialty grains. It is available in a whole range of colors, from 20 to 120 Lovibond. Crystal malt is malted barley that is heated while wet.
|
* Loss: [MatryoshkaLoss
](https://sbert.net/docs/package_reference/sentence_transformer/losses.html#matryoshkaloss) with these parameters:
```json
{
"loss": "MultipleNegativesRankingLoss",
"matryoshka_dims": [
1024,
512,
256,
128,
64,
32
],
"matryoshka_weights": [
1,
1,
1,
1,
1,
1
],
"n_dims_per_step": -1
}
```
squad
* Dataset: [squad](https://huggingface.co./datasets/sentence-transformers/squad) at [d84c8c2](https://huggingface.co./datasets/sentence-transformers/squad/tree/d84c8c2ef64693264c890bb242d2e73fc0a46c40)
* Size: 87,599 training samples
* Columns: question
and answer
* Approximate statistics based on the first 1000 samples:
| | question | answer |
|:--------|:------------------------------------------------------------------------------------------------|:---------------------------------------------------------------------------------------------------|
| type | string | string |
| details | - min: 14 characters
- mean: 59.66 characters
- max: 150 characters
| - min: 156 characters
- mean: 769.53 characters
- max: 3706 characters
|
* Samples:
| question | answer |
|:-------------------------------------------------------------------------|:---------------------------------------------------------------------------------------------------------------------------------------------------------------------------------------------------------------------------------------------------------------------------------------------------------------------------------------------------------------------------------------------------------------------------------------------------------------------------------------------------------------------------------------------------------------------------------------------------------------------------------------------------------------------------------------------------------------------------------------------------------------------------------------------------------------------------------------------------------------------------------------------------------------------------------------------------------------------------------------------------------------------------------------------------------|
| What did Business Insider call San Diego in 2013?
| San Diego was ranked as the 20th-safest city in America in 2013 by Business Insider. According to Forbes magazine, San Diego was the ninth-safest city in the top 10 list of safest cities in the U.S. in 2010. Like most major cities, San Diego had a declining crime rate from 1990 to 2000. Crime in San Diego increased in the early 2000s. In 2004, San Diego had the sixth lowest crime rate of any U.S. city with over half a million residents. From 2002 to 2006, the crime rate overall dropped 0.8%, though not evenly by category. While violent crime decreased 12.4% during this period, property crime increased 1.1%. Total property crimes per 100,000 people were lower than the national average in 2008.
|
| What did the Spanish call this region?
| The name Montana comes from the Spanish word Montaña, meaning "mountain", or more broadly, "mountainous country". Montaña del Norte was the name given by early Spanish explorers to the entire mountainous region of the west. The name Montana was added to a bill by the United States House Committee on Territories, which was chaired at the time by Rep. James Ashley of Ohio, for the territory that would become Idaho Territory. The name was successfully changed by Representatives Henry Wilson (Massachusetts) and Benjamin F. Harding (Oregon), who complained that Montana had "no meaning". When Ashley presented a bill to establish a temporary government in 1864 for a new territory to be carved out of Idaho, he again chose Montana Territory. This time Rep. Samuel Cox, also of Ohio, objected to the name. Cox complained that the name was a misnomer given that most of the territory was not mountainous and that a Native American name would be more appropriate than a Spanish one. Other names such as...
|
| Small missiles were designed that could be mounted on what?
| As this process continued, the missile found itself being used for more and more of the roles formerly filled by guns. First to go were the large weapons, replaced by equally large missile systems of much higher performance. Smaller missiles soon followed, eventually becoming small enough to be mounted on armored cars and tank chassis. These started replacing, or at least supplanting, similar gun-based SPAAG systems in the 1960s, and by the 1990s had replaced almost all such systems in modern armies. Man-portable missiles, MANPADs as they are known today, were introduced in the 1960s and have supplanted or even replaced even the smallest guns in most advanced armies.
|
* Loss: [MatryoshkaLoss
](https://sbert.net/docs/package_reference/sentence_transformer/losses.html#matryoshkaloss) with these parameters:
```json
{
"loss": "MultipleNegativesRankingLoss",
"matryoshka_dims": [
1024,
512,
256,
128,
64,
32
],
"matryoshka_weights": [
1,
1,
1,
1,
1,
1
],
"n_dims_per_step": -1
}
```
s2orc
* Dataset: [s2orc](https://huggingface.co./datasets/sentence-transformers/s2orc) at [8cfc394](https://huggingface.co./datasets/sentence-transformers/s2orc/tree/8cfc394e83b2ebfcf38f90b508aea383df742439)
* Size: 90,000 training samples
* Columns: title
and abstract
* Approximate statistics based on the first 1000 samples:
| | title | abstract |
|:--------|:------------------------------------------------------------------------------------------------|:--------------------------------------------------------------------------------------------------|
| type | string | string |
| details | - min: 31 characters
- mean: 80.02 characters
- max: 185 characters
| - min: 84 characters
- mean: 635.31 characters
- max: 1023 characters
|
* Samples:
| title | abstract |
|:----------------------------------------------------------------------------------------------------------------------|:--------------------------------------------------------------------------------------------------------------------------------------------------------------------------------------------------------------------------------------------------------------------------------------------------------------------------------------------------------------------------------------------------------------------------------------------------------------------------------------------------------------------------------------------------------------------------------------------------------------------------------------------------------------------------------------------------------------------------------------------------------------------------------------------------------------------------------------------------------------------------------------------------------|
| Modeling Method of Flow Diversion of the Three Outlets in Jingjiang Reach Under Unsteady Flow Conditions
| The Yangtze River Flood Protection Physical Model is built under the financial support of World Bank loan.Based on theoretical analysis and experimental study,a modeling method of flow diversion of the three outlets in Jingjiang Reach under unsteady flow conditions was established for the model.Validation tests under both steady and unsteady flow conditions manifested that with this modeling method,the experimental flow diversion proves to be consistent with that of the prototype and therefore meets the requirements for precision.Being validated,this modeling method has been applied to Yangtze River Flood Protection Physical Model to study the flood routing features in Jingjiang reach.
|
| Enlightening on medical administration by clinical governance in British
| Medical quality and safety were the responsibilities of medical system in view of British clinical governance. Medical regulation institutes were considered to be built and be authorized regulation rights. British medical administration was introduced and its enlightening in China was mentioned.
|
| APPLICATION OF A FUZZY MULTI-CRITERIA DECISION-MAKING MODEL FOR SHIPPING COMPANY PERFORMANCE EVALUATION
| Combining fuzzy set theory, Analytic Hierarchy Process (AHP) and concept of entropy, a fuzzy Multiple Criteria Decision-Making (MCDM) model for shipping company performance evaluation is proposed. First, the AHP is used to construct subjective weights for all criteria and sub-criteria. Then, linguistic values characterized by triangular fuzzy numbers and trapezoidal fuzzy numbers are used to denote the evaluation values of all alternatives with respect to various subjective and objective criteria. Finally, the aggregation fuzzy assessment of different shipping companies is ranked to determine the best selection. Utilizing this fuzzy MCDM model, the decision-maker's fuzzy assessment and the trade-off between various evaluations criteria can be taken into account in the aggregation process, thus ensuring more effective and accurate decision-making.
|
* Loss: [MatryoshkaLoss
](https://sbert.net/docs/package_reference/sentence_transformer/losses.html#matryoshkaloss) with these parameters:
```json
{
"loss": "MultipleNegativesRankingLoss",
"matryoshka_dims": [
1024,
512,
256,
128,
64,
32
],
"matryoshka_weights": [
1,
1,
1,
1,
1,
1
],
"n_dims_per_step": -1
}
```
allnli
* Dataset: [allnli](https://huggingface.co./datasets/sentence-transformers/all-nli) at [d482672](https://huggingface.co./datasets/sentence-transformers/all-nli/tree/d482672c8e74ce18da116f430137434ba2e52fab)
* Size: 557,850 training samples
* Columns: anchor
, positive
, and negative
* Approximate statistics based on the first 1000 samples:
| | anchor | positive | negative |
|:--------|:------------------------------------------------------------------------------------------------|:------------------------------------------------------------------------------------------------|:------------------------------------------------------------------------------------------------|
| type | string | string | string |
| details | - min: 18 characters
- mean: 34.88 characters
- max: 193 characters
| - min: 15 characters
- mean: 46.49 characters
- max: 181 characters
| - min: 16 characters
- mean: 50.47 characters
- max: 204 characters
|
* Samples:
| anchor | positive | negative |
|:---------------------------------------------------------------------------|:-------------------------------------------------|:-----------------------------------------------------------|
| A person on a horse jumps over a broken down airplane.
| A person is outdoors, on a horse.
| A person is at a diner, ordering an omelette.
|
| Children smiling and waving at camera
| There are children present
| The kids are frowning
|
| A boy is jumping on skateboard in the middle of a red bridge.
| The boy does a skateboarding trick.
| The boy skates down the sidewalk.
|
* Loss: [MatryoshkaLoss
](https://sbert.net/docs/package_reference/sentence_transformer/losses.html#matryoshkaloss) with these parameters:
```json
{
"loss": "MultipleNegativesRankingLoss",
"matryoshka_dims": [
1024,
512,
256,
128,
64,
32
],
"matryoshka_weights": [
1,
1,
1,
1,
1,
1
],
"n_dims_per_step": -1
}
```
paq
* Dataset: [paq](https://huggingface.co./datasets/sentence-transformers/paq) at [74601d8](https://huggingface.co./datasets/sentence-transformers/paq/tree/74601d8d731019bc9c627ffc4271cdd640e1e748)
* Size: 64,371,441 training samples
* Columns: query
and answer
* Approximate statistics based on the first 1000 samples:
| | query | answer |
|:--------|:------------------------------------------------------------------------------------------------|:--------------------------------------------------------------------------------------------------|
| type | string | string |
| details | - min: 25 characters
- mean: 50.56 characters
- max: 104 characters
| - min: 509 characters
- mean: 620.96 characters
- max: 773 characters
|
* Samples:
| query | answer |
|:----------------------------------------------------------------------------|:--------------------------------------------------------------------------------------------------------------------------------------------------------------------------------------------------------------------------------------------------------------------------------------------------------------------------------------------------------------------------------------------------------------------------------------------------------------------------------------------------------------------------------------------------------------------------------------------------------------------------------------------------------------------------------------------------------------------------------------------------------------------------|
| in veetla visheshanga ganesh is the husband of
| Veetla Visheshanga a song which reminds Ganga's memory. She is actually not Ganga but Gowri and her lover is the groom named Ganesh. When both were about to marry they were stopped by some goons because of which Gowri fell from the mountain but survived with injuries. Gopal who found the truth brought Ganesh to unite them. Gopal insists Gowri to marry Ganesh as both of them are lovers to which Gowri unwillingly accepts. But while Ganesh tries to tie the Mangal Sutra, Gowri stops him and she goes to Gopal saying that he may not need her but she needs him
|
| when did simon property group became a publicly traded company
| of the S&P 100. Simon Property Group has been the subject of several lawsuits and investigations regarding civil rights and discrimination. Simon Property Group was formed in 1993 when the majority of the shopping center interests of Melvin Simon & Associates became a publicly traded company. Melvin Simon & Associates, owned by brothers Melvin Simon and Herbert Simon, was founded in 1960 in Indianapolis, Indiana, and had long been one of the top shopping center developers in the United States. In 1996, Simon DeBartolo Group was created when Simon Property merged with former rival DeBartolo Realty Corp. This was shortly
|
| what was the nationality of antoine faivre
| Theosophy (Boehmian) below. "Theosophy": The scholar of esotericism Wouter Hanegraaff described Christian theosophy as "one of the major currents in the history of Western esotericism". Christian theosophy is an under-researched area; a general history of it has never been written. The French scholar Antoine Faivre had a specific interest in the theosophers and illuminists of the eighteenth and nineteenth centuries. He wrote his doctoral thesis on Karl von Eckartshausen and Christian theosophy. Scholars of esotericism have argued that Faivre's definition of Western esotericism relies on his own specialist focus on Christian theosophy, Renaissance Hermeticism, and Romantic "Naturphilosophie" and therefore creates an "ideal"
|
* Loss: [MatryoshkaLoss
](https://sbert.net/docs/package_reference/sentence_transformer/losses.html#matryoshkaloss) with these parameters:
```json
{
"loss": "MultipleNegativesRankingLoss",
"matryoshka_dims": [
1024,
512,
256,
128,
64,
32
],
"matryoshka_weights": [
1,
1,
1,
1,
1,
1
],
"n_dims_per_step": -1
}
```
trivia
_qa
* Dataset: [trivia_qa](https://huggingface.co./datasets/sentence-transformers/trivia-qa) at [a7c36e3](https://huggingface.co./datasets/sentence-transformers/trivia-qa/tree/a7c36e3c8c8c01526bc094d79bf80d4c848b0ad0)
* Size: 73,346 training samples
* Columns: query
and answer
* Approximate statistics based on the first 1000 samples:
| | query | answer |
|:--------|:------------------------------------------------------------------------------------------------|:----------------------------------------------------------------------------------------------------|
| type | string | string |
| details | - min: 21 characters
- mean: 76.91 characters
- max: 455 characters
| - min: 136 characters
- mean: 3273.89 characters
- max: 4096 characters
|
* Samples:
| query | answer |
|:--------------------------------------------------------------------------------|:---------------------------------------------------------------------------------------------------------------------------------------------------------------------------------------------------------------------------------------------------------------------------------------------------------------------------------------------------------------------------------------------------------------------------------------------------------------------------------------------------------------------------------------------------------------------------------------------------------------------------------------------------------------------------------------------------------------------------------------------------------------------------------------------------------------------------------------------------------------------------------------------------------------------------------------------------------------------------------------------------------------------------------------------------------|
| What type of rock is formed by the solidification of molten magma?
| igneous rock - Dictionary Definition : Vocabulary.com igneous rock n rock formed by the solidification of molten magma Types: a rare type of peridotite that sometimes contains diamonds; found in South Africa and Siberia Type of: material consisting of the aggregate of minerals like those making up the Earth's crust Word Family Usage Examples Sign up, it's free! Whether you're a student, an educator, or a life-long learner, Vocabulary.com can put you on the path to systematic vocabulary improvement.
|
| Which river flows through the town of Shrewsbury?
| River Severn | river, Wales and England, United Kingdom | Britannica.com river, Wales and England, United Kingdom Written By: Wales River Severn, Welsh Hafren, Britain’s longest river from source to tidal waters—about 180 miles (290 km) long, with the Severn estuary adding some 40 miles (64 km) to its total length. The Severn rises near the River Wye on the northeastern slopes of Plynlimon (Welsh: Pumlumon), Wales , and follows a semicircular course basically southward to the Bristol Channel and the Atlantic Ocean . It drains an area of 4,350 square miles (11,266 square km) with an average discharge at Bewdley of 2,170 cubic feet (61.5 cubic m) per second. River Severn at Shrewsbury, Shropshire, Eng. Chris Bayley The river’s course is at first southeasterly, descending from an elevation of 2,000 feet (600 m) at its source to 500 feet (150 m) at the Welsh town of Llanidloes. There it turns sharply northeastward, following the Vale of Powys past Newtown and Welshpool . At Llanymynech the...
|
| Which band's name was inspired by a novel by Herman Hesse?
| 23 Band Names Inspired by Literature :: Books :: Lists :: Paste 23 Band Names Inspired by Literature By Wyndham Wyeth | April 24, 2011 | 10:52pm Share Tweet Submit Pin At Paste, we look for “Signs of Life” in all forms of art. And while we value each artform for its unique merits, it’s always a treat when they overlap. So we decided to take a look at bands that derived their names from literature. The works that inspired several of the entries are probably obvious, but a few of them will most certainly surprise you. It may also surprise you to see which genres favor the written word. (Who knew metalheads were such scholars?) Photo by Max Blau New Jersey punks Titus Andronicus take their name from the greatest wordsmith of them all, William Shakespeare . Titus Andronicus is thought to be the famous playwright’s first tragedy. It is also his bloodiest and most violent work. 2. The Doors Source: The Doors of Perception by Aldous Huxley When The Doors formed in 1965, they decided to na...
|
* Loss: [MatryoshkaLoss
](https://sbert.net/docs/package_reference/sentence_transformer/losses.html#matryoshkaloss) with these parameters:
```json
{
"loss": "MultipleNegativesRankingLoss",
"matryoshka_dims": [
1024,
512,
256,
128,
64,
32
],
"matryoshka_weights": [
1,
1,
1,
1,
1,
1
],
"n_dims_per_step": -1
}
```
msmarco_10m
* Dataset: [msmarco_10m](https://huggingface.co./datasets/bclavie/msmarco-10m-triplets) at [8c5139a](https://huggingface.co./datasets/bclavie/msmarco-10m-triplets/tree/8c5139a245a5997992605792faa49ec12a6eb5f2)
* Size: 10,000,000 training samples
* Columns: query
, positive
, and negative
* Approximate statistics based on the first 1000 samples:
| | query | positive | negative |
|:--------|:------------------------------------------------------------------------------------------------|:-------------------------------------------------------------------------------------------------|:--------------------------------------------------------------------------------------------------|
| type | string | string | string |
| details | - min: 10 characters
- mean: 33.47 characters
- max: 158 characters
| - min: 53 characters
- mean: 353.76 characters
- max: 948 characters
| - min: 67 characters
- mean: 343.74 characters
- max: 1063 characters
|
* Samples:
| query | positive | negative |
|:-------------------------------------------------|:--------------------------------------------------------------------------------------------------------------------------------------------------------------------------------------------------------------------------------------------------------------------------------------------------------------------------------------------------------------------|:-----------------------------------------------------------------------------------------------------------------------------------------------------------------------------------------------------------------------------------------------------------------------------------------------------------------------------------------------------------------------------------------------------------------------------------------------------------------------------------------------------------------------|
| what is ged equivalent
| A GED is equivalent to a high school diploma however colleges do not look at it the same. A huge part of high school is commitment and dedication, therefore if you choose to drop out of high school you will probably not get accepted into any top colleges. However, you could always start at a community college and work your way up. Good Luck
| If you are not far along in your Army career, education is an especially good way to boost your chances of promotion. Continuing your education at any level can earn you points. For completing your GED or bachelor's degree, you earn 10 points.
|
| foods that help with diverticulitis
| Diet for Diverticulitis. Gradually you can ease back into a regular diet. Your doctor may advise you to start with low-fiber foods (white bread, meat, poultry, fish, eggs, and dairy products) before introducing high-fiber foods. Fiber softens and adds bulk to stools, helping them pass more easily through the colon.
| During an attack of diverticulitis, your doctor may recommend a clear liquid diet or a low-fiber diet. This helps the area of infection to heal. Foods allowed on a clear-liquid diet include: 1 Plain water.iverticulitis occurs when small, bulging pouches (diverticula) in your colon become infected and inflamed â causing severe abdominal pain, nausea, and fever. The treatment of a diverticulitis attack will depend on the severity of the symptoms and whether this is your first attack.
|
| calories burned in turbo kick class
| The American Council on Exercise did a study to find out how many calories are burned during a turbo kick class. The study looked at 15 women in a turbo kick class who weighed about 135 lbs and found that they burned between 6.45 to 8.3 calories a minute. This comes out to about 350 to 450 calories an hour.
| Popular Calories Burned Searches: 1 Calories Burned For Intervals of run/walk or walk/jog: 6 mph or slower (> 10 minutes per mile) 2 Calories Burned For Walking: 3.5 mph (17 minutes per mile) 3 Calories Burned For Walking: 4 mph (15 minutes per mile) Calories Burned For Walking: 4.6 mph (13 minutes per mile)
|
* Loss: [MatryoshkaLoss
](https://sbert.net/docs/package_reference/sentence_transformer/losses.html#matryoshkaloss) with these parameters:
```json
{
"loss": "MultipleNegativesRankingLoss",
"matryoshka_dims": [
1024,
512,
256,
128,
64,
32
],
"matryoshka_weights": [
1,
1,
1,
1,
1,
1
],
"n_dims_per_step": -1
}
```
swim_ir
* Dataset: [swim_ir](https://huggingface.co./datasets/nthakur/swim-ir-monolingual) at [834c20f](https://huggingface.co./datasets/nthakur/swim-ir-monolingual/tree/834c20f0ceef6a68e029fb4447d17d20bb0288c3)
* Size: 501,538 training samples
* Columns: query
and text
* Approximate statistics based on the first 1000 samples:
| | query | text |
|:--------|:-----------------------------------------------------------------------------------------------|:--------------------------------------------------------------------------------------------------|
| type | string | string |
| details | - min: 0 characters
- mean: 59.98 characters
- max: 189 characters
| - min: 208 characters
- mean: 525.9 characters
- max: 2743 characters
|
* Samples:
| query | text |
|:------------------------------------------------------------------------------------------------------------|:------------------------------------------------------------------------------------------------------------------------------------------------------------------------------------------------------------------------------------------------------------------------------------------------------------------------------------------------------------------------------------------------------------------------------------------------------------------------------------------------------------------------------------------------------------------------------------------------------------------------------------------------------------------------------------------------------------------------------------------------------------------------------------------------------|
| How many blocked kicks did Williams have in his second year at Bowling Green State University?
| Williams accepted a football scholarship from Bowling Green State University, where he became one of the best special teams players in school history. As a redshirt freshman, he was a wide receiver on the scout team. The next year, he played mainly on special teams and had 3 blocked kicks.
|
| How many town councils are there in the metropolitan borough?
| Horwich, Westhoughton and Blackrod are now constituted as civil parishes. There are three town councils in the metropolitan borough, Westhoughton Town Council, Horwich Town Council and Blackrod Town Council. The rest of the metropolitan borough, Bolton, Farnworth, Kearsley, Little Lever, and South Turton, have remained unparished areas since 1974.
|
| What is the name of the person selected to lead BART’s 296-member police force?
| In 2009, the hiring of two independent organizations reviewed BART's policies and procedures in the process of overseeing the BART Police. The two independent firms investigated the matters of BART Police Shooting of Oscar Grant and were charged with making recommendations to the board. Ward Allen formulated and chaired BART's first Police Department Review Committee, and as a result, BART made sweeping changes on many security measures, as well as corrected and implemented several policies and procedures. BPD Review Committee has led to the re-training of all officers on use of force, diversity re-training and other issues. Ward Allen hired Kenton Rainey, the person selected to lead BART’s 296-member police force, to take command as Chief of Police.
|
* Loss: [MatryoshkaLoss
](https://sbert.net/docs/package_reference/sentence_transformer/losses.html#matryoshkaloss) with these parameters:
```json
{
"loss": "MultipleNegativesRankingLoss",
"matryoshka_dims": [
1024,
512,
256,
128,
64,
32
],
"matryoshka_weights": [
1,
1,
1,
1,
1,
1
],
"n_dims_per_step": -1
}
```
pubmedqa
* Dataset: [pubmedqa](https://huggingface.co./datasets/sentence-transformers/pubmedqa) at [a1ef0b5](https://huggingface.co./datasets/sentence-transformers/pubmedqa/tree/a1ef0b513b16ed490e807ac11da40e436d3a54c3)
* Size: 1,660 training samples
* Columns: anchor
, positive
, negative_1
, negative_2
, negative_3
, negative_4
, negative_5
, negative_6
, negative_7
, negative_8
, negative_9
, negative_10
, negative_11
, negative_12
, negative_13
, negative_14
, negative_15
, negative_16
, negative_17
, negative_18
, negative_19
, and negative_20
* Approximate statistics based on the first 1000 samples:
| | anchor | positive | negative_1 | negative_2 | negative_3 | negative_4 | negative_5 | negative_6 | negative_7 | negative_8 | negative_9 | negative_10 | negative_11 | negative_12 | negative_13 | negative_14 | negative_15 | negative_16 | negative_17 | negative_18 | negative_19 | negative_20 |
|:--------|:------------------------------------------------------------------------------------------------|:-------------------------------------------------------------------------------------------------|:-------------------------------------------------------------------------------------------------|:-------------------------------------------------------------------------------------------------|:--------------------------------------------------------------------------------------------------|:--------------------------------------------------------------------------------------------------|:--------------------------------------------------------------------------------------------------|:--------------------------------------------------------------------------------------------------|:--------------------------------------------------------------------------------------------------|:--------------------------------------------------------------------------------------------------|:--------------------------------------------------------------------------------------------------|:--------------------------------------------------------------------------------------------------|:-------------------------------------------------------------------------------------------------|:--------------------------------------------------------------------------------------------------|:--------------------------------------------------------------------------------------------------|:--------------------------------------------------------------------------------------------------|:--------------------------------------------------------------------------------------------------|:--------------------------------------------------------------------------------------------------|:--------------------------------------------------------------------------------------------------|:--------------------------------------------------------------------------------------------------|:--------------------------------------------------------------------------------------------------|:--------------------------------------------------------------------------------------------------|
| type | string | string | string | string | string | string | string | string | string | string | string | string | string | string | string | string | string | string | string | string | string | string |
| details | - min: 25 characters
- mean: 94.06 characters
- max: 213 characters
| - min: 5 characters
- mean: 409.42 characters
- max: 1582 characters
| - min: 5 characters
- mean: 325.57 characters
- max: 1300 characters
| - min: 17 characters
- mean: 299.5 characters
- max: 1352 characters
| - min: 21 characters
- mean: 317.37 characters
- max: 1590 characters
| - min: 13 characters
- mean: 334.43 characters
- max: 1536 characters
| - min: 13 characters
- mean: 335.49 characters
- max: 1247 characters
| - min: 13 characters
- mean: 336.16 characters
- max: 1383 characters
| - min: 14 characters
- mean: 319.98 characters
- max: 1501 characters
| - min: 16 characters
- mean: 337.33 characters
- max: 1493 characters
| - min: 15 characters
- mean: 324.82 characters
- max: 1058 characters
| - min: 13 characters
- mean: 336.76 characters
- max: 1457 characters
| - min: 10 characters
- mean: 355.4 characters
- max: 1748 characters
| - min: 13 characters
- mean: 344.26 characters
- max: 1705 characters
| - min: 16 characters
- mean: 335.11 characters
- max: 1593 characters
| - min: 18 characters
- mean: 353.83 characters
- max: 1374 characters
| - min: 13 characters
- mean: 328.01 characters
- max: 1755 characters
| - min: 12 characters
- mean: 337.74 characters
- max: 1579 characters
| - min: 16 characters
- mean: 336.94 characters
- max: 1325 characters
| - min: 15 characters
- mean: 319.49 characters
- max: 1410 characters
| - min: 12 characters
- mean: 340.91 characters
- max: 1680 characters
| - min: 20 characters
- mean: 330.34 characters
- max: 1509 characters
|
* Samples:
| anchor | positive | negative_1 | negative_2 | negative_3 | negative_4 | negative_5 | negative_6 | negative_7 | negative_8 | negative_9 | negative_10 | negative_11 | negative_12 | negative_13 | negative_14 | negative_15 | negative_16 | negative_17 | negative_18 | negative_19 | negative_20 |
|:--------------------------------------------------------------------------------------------------------------------------|:---------------------------------------------------------------------------------------------------------------------------------------------------------------------------------------------------------------------------------------------------------------------------------------------------------------------------------------------------------------------------------------------------------------------------------------------------------------------------------------------------------------------------------------------------------------------------------------------------------------------------------------------------------------------------------------------------------------------------------------------------------------------------------------------------------------------------------------------------------------------------------------------------------------------------------------------------------------------------------------------------------------------------------------------------------|:---------------------------------------------------------------------------------------------------------------------------------------------------------------------------------------------------------------------------------------------------------------------------------------------------------------------------------------------------------------------------------------------------------------------------------------------------------------------------------------------------------------------------------|:----------------------------------------------------------------------------------------------------------------------------------------------------------------------------------------------------------------------------------------------------------------------------------------------------------------------------------------------------------|:---------------------------------------------------------------------------------------------------------------------------------------------------------------------------------------------------------------------------------------------------------------------------------------------------------------------------------------------------------------------------------------------------------------------------------------------------|:---------------------------------------------------------------------------------------------------------------------------------------------------------------------------------------------------------------------------------------------------------------------------------------------------------------------------------------------------------------------------------------|:------------------------------------------------------------------------------------------------------------------------------------------------------------------------------------------------------------------------------------------------|:--------------------------------------------------------------------------------------------------------------------------------------------------------------------------------------------------------------------------------------------------------------------------------------------------------------------------------------------------------------------------------------------------------------------------------------------------------------------------------------------------------------------------------------------------------------------------------------------------------------------------------------------------------------------------------------------------------------------------------------------------------------------------------------------------------------------------------------------------------------------------------------------------------------------------------------------------------------------------------------------------------------------------------|:--------------------------------------------------------------------------------------------------------------------------------------------------------------------------------------------------------------------------------------------------------------------------------------------------------------------------------------------------------------------------------------------------------------------------|:------------------------------------------------------------------------------------------------------------------------------------------------------------------------------------------------------------------------------------------------------------------------------------------------------------------------------------------------------------------------------------------------|:------------------------------------------------------------------------------------------------------------------------------------------------------------------------------------------------------------------------------------------------------------------------------------------------------------------------------------------------------------------------------------------------------------------------------------------------------------------------------------------------------------------------------------------------------------------------------------------------------------------------------------------------------------------------------------------------------------------------------------------------------------------------------------------------------------------------------------------------------------------------|:-----------------------------------------------------------------------------------------------------------------------------------------------------------------------------------------------------------------------------------------------------------------------------------------------------------------------------------------------------------|:-----------------------------------------------------------------------------------------------------------------------------------------------------------------------------------------------------------------------------------------------------------------------------------------------------------------------------------------------------|:---------------------------------------------------------------------------------------------------------------------------------------------------------------------------------------------------------------------------------------------------------------------------------------------------------------------------------------------------------------------------------------------------------------------------------------------------------------------------------------------------------------------------|:-----------------------------------------------------------------------------------------------------------------------------------------------------------------------------------------------------------------------------------------------------------------------------|:---------------------------------------------------------------------------------------------------------------------------------------------------------------------------------------------------------------------------------------------------------------------------------------------------------------------------------------------------------------------------------------------------------------------------------------------------------------------------------------------------------------------------------------------------------------------------------------------------------------------------------------------------------------------------------------------|:-----------------------------------------------------------------------------------------------------------------------------------------------------------------------------------------------------------------------------------------------------------------------------------------------------------------------------------------------------------------------------------------------------------------------|:---------------------------------------------------------------------------------------------------------------------------------------------------------------------------------------------------------------------------------------------------------------------------------------------------------------------------------------------------------------------------------------------------------------------------------------------------------------------------------------------------------------------------------------------------------------------------------------------------------------------------------------------------------------------------------------------------------------------------------------------------------|:----------------------------------------------------------------------------------------------------------------------------------------------------------------------------------------------------------------------------------------------------------------------------------------------------------------------------------------------------------------------------------------------------------------------------------------------------------------------------------------------------------------------------------------------------------------|:--------------------------------------------------------------------------------------------------------------------------------------------------------------------------------------------------------------------------------------------------------------------------------------------------------------------------------------------------------------------------------------------------------------------|:------------------------------------------------------------------------------------------------------------------------------------------------------------------------------------------------------------------------------------------------|:------------------------------------------------------------------------------------------------------------------------------------------------------------------------------------------------------------------------------------------------------------------------------------------------------------------------------------------------------------------------------------------------------------------------------------|
| Visceral adipose tissue area measurement at a single level: can it represent visceral adipose tissue volume?
| Measurement of visceral adipose tissue (VAT) needs to be accurate and sensitive to change for risk monitoring. The purpose of this study is to determine the CT slice location where VAT area can best reflect changes in VAT volume and body weight.
| 46 patients with psoriasis and 46 sex- and age-matched control patients were included in this study. The abdominal fat area [visceral fat area (VFA), subcutaneous fat area (SFA) and total fat area (TFA)] at the level of the umbilicus was evaluated by computed tomography.
| A retrospective review of CRC patients who received adjuvant chemotherapy at a single center during the period 2006-2009 identified from a prospectively maintained database. Visceral adiposity was determined by measuring visceral fat area (VFA) on preoperative staging CT. All patients were followed up to study completion or death.
| A total of 1941 participants without known cardiovascular disease were enrolled from the Korean Genome and Epidemiology Study. Visceral fat area (VFA) was assessed by computed tomography. Appendicular skeletal muscle mass (ASM) was estimated by dual-energy X-ray absorptiometry and was used as a percentage of body weight (ASM/Wt). LV structure and function were assessed by tissue Doppler imaging (TDI) echocardiography.
| One hundred and forty nonobese patients (BMI <25 kg/m2) were enrolled. EFV and visceral fat area were measured by MDCT. Patients were classified according to the plaque components (noncalcified, mixed and calcified) and severity of CAD. Inflammatory biomarkers were also measured, and compared with each CT parameter.
| The blood gas level in each pulmonary vein (PV) was measured in supine subjects with diverse body mass index (BMI) values, to determine whether there was a regional insufficiency in gas exchange depending on the subject's BMI.
| Magnetic resonance imaging (MRI) of 163 patients with cholecystolithiasis and 163 non-cholecystolithiasis control subjects admitted to our institution between March 2011 and September 2013 were included in this cross-sectional evaluation. There were 98 women and 65 men in cholecystolithiasis group with an average age of 57±16 years (range 25-86 years). There were 87 women and 76 men in the control group with an average age of 41±16 years (range 14-77 years). Visceral adipose tissue (VAT), abdominal subcutaneous adipose tissue (SAT) and total abdominal adipose tissue (TAT) of all the subjects at navel level were measured on abdominal MRI. According to the visceral adipose area (cut-off point VAT = 100 cm2), study subjects were divided into 1) increased accumulation of intra-abdominal fat and 2) normal distribution of intra-abdominal fat. Logistic regression was used to assess the association of fat with the presence of cholecystolithiasis, adjusted for age and sex.
| We reviewed the medical records of 106 patients undergoing LA or LESS-A at our institution. Total fat area (TFA) and visceral fat area (VFA) were measured at the level of the L4 vertebra by computed tomography. To categorize the type of obesity, the VFA/TFA ratio was calculated. Multiple logistic regression analyses were performed to identify independent predictors of prolonged operative time.
| The weight gain was 5.2% greater in rats exposed to fructose than in controls (P = 0.042). Total and visceral adipose tissue volumes were 5.2 cm3 (P = 0.017) and 3.1 cm3 (P = 0.019) greater, respectively, while lean tissue volumes did not differ. The level of triglycerides and apolipoprotein A-I was higher (P = 0.034, P = 0.005, respectively) in fructose-exposed rats.
| A total of 1593 middle-aged to older patients participated in this cross-sectional study. Brachial-to-ankle pulse wave velocity (baPWV) was measured as an index of arterial stiffness. Second PP (PP2) at the second peak of radial SBP was used to estimate central PP. Radial augmentation index was calculated as PP2/PP. Thigh muscle cross-sectional area and abdominal visceral fat area were quantified by computed tomography. Patients were classified as sarcopenic if their hand grip strength or skeletal muscle mass (measured by bioelectrical impedance) was more than 1 SD lower than the mean of those in a reference group aged below 50 years, or in the lowest 20% of the studied population. Visceral obesity was defined as visceral fat area greater than 100 cm.
| Visceral adiposity is linked with sleep-disordered breathing (SDB) (called Syndrome Z), and both correlate with coronary artery disease (CAD). The aim of the present study was to determine the significance of excess visceral fat, SDB and circulating levels of biomarkers in CAD in Japanese men.
| There are no published studies on the impact of visceral adipose tissue (VAT) change on outcomes of restorative proctocolectomy and ileal pouch-anal anastomosis (IPAA). The aim of this historic cohort study was to evaluate the impact of excessive VAT gain on the outcomes of inflammatory bowel disease (IBD) patients with IPAA.
| A subgroup of 46 men (n = 20, aged 29.1-33.4 years) and women (n = 26, aged 29.1-33.8 years) were recruited from an ongoing population study at our institution. Anthropometric variables including weight, height, and waist circumference were measured using standard procedures, and body mass index was calculated (kg/m(2)). Visceral adipose tissue (VAT) was measured with magnetic resonance imaging. Plasma apolipoproteins, lipids, glucose, and insulin were measured after an overnight fasting.
| Our aim was to describe adipose tissue content and distribution in ALS patients.
| This was a cross-sectional study of 140 Japanese patients with type 2 diabetes (mean age 65 ± 11 year; 44.6% women). Visceral fat area (VFA; cm(2) ) and liver attenuation index (LAI) were assessed by abdominal computed tomography. The patients were divided into four groups by VFA and body mass index (BMI; kg/m(2) ) as follows: BMI <25 kg/m(2) and VFA <100 cm(2) (OB[-]VA[-]), BMI ≥25 kg/m(2) and VFA <100 cm(2) (OB[+]VA[-]), BMI <25 kg/m(2) and VFA ≥100 cm(2) (OB[-]VA[+]), and BMI ≥25 kg/m(2) and VFA ≥100 cm(2) (OB[+]VA[+]). Multivariate linear regression and logistic regression analysis were carried out to determine the impact of OB(-)VA(+) on LAI.
| Epicardial adipose tissue represents visceral adiposity, the early detection of which could be helpful for assessing subclinical target organ damage. Although previous studies have reported a relationship between epicardial fat thickness (EFT) and carotid intima-media thickness, there have been no studies detailing the relationship between EFT and brachial-ankle pulse wave velocity (baPWV).
| Higher adiponectin levels were associated with lower risk of diabetes (P < 0.001). Visceral fat was the only adiposity measure associated with diabetes after adjusting for BMI (odds ratio 3.0 [2.1-4.3] in women and 1.3 [1.0-1.6] in men, P < 0.001 between-sex comparison). Adipocytokines attenuated the association between visceral fat and diabetes for both sexes but more strongly in men (women 2.3 [1.5-3.3], men 1.1 [0.9-1.4]). In men, adiponectin, IL-6, and PAI-1 remained independently associated with diabetes after adjusting for fat depots; in women, adiponectin was the only independently associated adipocytokine. Controlling for insulin, HDL, triglycerides, and blood pressure did not change these results.
| Ninety obesity patients and 95 non-obesity Uygur individuals were enrolled in this study. CD68 levels in abdominal subcutaneous and omental adipose tissues were detected by immunohistochemistry. The cytokine expression levels of adiponectin (APMI) and visfatin in serum were measured by enzyme-linked immunosorbent assay. Infection of 3T3-L1 cells with Ad36 was performed. Real-time PCR was performed to determine expression levels of APMI and Visfatin genes in the 3T3-L1 preadipocytes infected with Ad36.
| Serum CEA levels correlated with visceral fat area, fasting glucose, and triglyceride levels after adjusting for age and BMI. The mean visceral fat area increased significantly with the increasing CEA tirtiles. In a step-wise multiple regression analysis, age (β = 0.26, p<0.01) and visceral fat area (β = 0.19, p = 0.03) were identified as explanatory variables for serum CEA level.
| The visceral adiposity index (VAI) has proved to be a marker of visceral adipose dysfunction, strongly associated with insulin sensitivity in both the general and specific populations of patients at metabolic risk.
| Visceral adiposity is associated with hepatic steatosis, inflammation, and fibrosis in non-alcoholic fatty liver disease (NAFLD). The visceral adiposity index (VAI), a novel marker of visceral fat distribution and dysfunction, has been correlated with histology in hepatitis C. We assessed the ability of VAI to predict disease severity in NAFLD and hence its role as a non-invasive marker of liver damage.
|
| Do general practitioner hospitals reduce the utilisation of general hospital beds?
| Observational study comparing the total rates of admissions and of occupied bed days in general hospitals between populations with and without access to GP hospitals. Comparisons were also made separately for diagnoses commonly encountered in GP hospitals.
| To study the prevalence of GERD comorbidities in a tertiary care hospital.
| The population studied was a sample of 10% of the patients 65 years or older registered with a general practitioner contributing to the General Practice Research Database between 1988 and 1996.
| Tertiary University Hospitals.
| University hospital and district general hospital.
| Inpatient rehabilitation facilities.
| Inpatient rehabilitation facilities.
| General medical service at a teaching hospital.
| To examine the hypothesis that nursing homes responding to these changes in demand shifted the balance of resources from hotel to clinical activities.
| Outpatient practices of general practitioners in the United Kingdom who contribute to the General Practice Research Database.
| Hospital rehabilitation programs.
| Surgical department of a large district general hospital.
| Hospital-based case-control study.
| Patients' homes.
| the relationship between proximity to death and the amount of care provided by general practitioners (GPs) is largely unknown.
| Public hospital, primary care clinic.
| A teaching hospital and a district general hospital.
| District General Hospital in the UK.
| Academic general internal medicine practice.
| A hospital-based study was conducted.
| To evaluate the impact of participation in a trial on General Practitioners management and patient behaviour.
|
| "Occult" posttraumatic lesions of the knee: can magnetic resonance substitute for diagnostic arthroscopy?
| We identified three types of occult post-traumatic injuries by morpho-topographic and signal intensity patterns: bone bruises (no. 25), subchondral (no. 33) and osteochondral (no. 35) injuries. Arthroscopy depicted 45 osteochondral and 19 chondral injuries. A bone bruise was defined as a typical subcortical area of signal loss, with various shapes, on T1-weighted images and of increased signal intensity on T2-weighted and FIR images. The cortical bone and articular cartilage were normal in all cases, while osteochondral injuries exhibited associated bone and cartilage damage with the same abnormal MR signal intensity. Sprain was the mechanism of injury in 52 cases, bruise in 12 and stress in 6. In 52 sprains (30 in valgus), the injury site was the lateral compartment in 92.3% of cases (100% in valgus), associated with meniscal damage in 73% of cases (90% in valgus) and with ligament injury in 90.4% (100% in valgus). In 12 bruises, the injury site was the lateral compartment in 58.3% of...
| Preoperative range of motion (ROM) has been regarded as one of the most important factors in predicting postoperative ROM following total knee arthroplasty (TKA). Mobile-bearing TKA designs have been suggested to possibly improve the knee kinematics compared to fixed-bearing designs. The purpose of this study was to examine the difference in postoperative flexion as a function of preoperative flexion in a consecutive series of TKAs done using a posterior-stabilized rotating-platform prosthesis.
| To assess the association of underlying diagnosis with outcomes after revision total knee arthroplasty (TKA).
| We identified 37 knees diagnosed with osteoarthritis with a preoperative knee flexion ≥120° but a 12-month postoperative range of motion (ROM) ≤110°. A random sample of 111 patients (1:3) from the same database, whose knees had a preoperative and 12-month postoperative ROM ≥120°, based on a diagnosis of primary osteoarthritis and no previous open knee surgery, were selected as the controls.
| This study reports a series of patients operated on by anterior cruciate ligament (ACL) reconstruction combined with valgus high tibial osteotomy (HTO) for chronic anterior knee instability associated with medial tibiofemoral osteoarthritis. It was hypothesized that the combined surgery would enable return to sport, stabilize the knee and relieve medial pain.
| To determine the prevalence and factors associated with knee osteoarthritis (OA) defined by magnetic resonance imaging (MRI) and specific OA features on MRI 1 year after anterior cruciate ligament reconstruction (ACLR).
| In anterior ankle arthroscopy, the anterior working area (AWA) is restricted by the presence of the dorsalis pedis artery (DPA) and tendons. Pseudoaneurysms caused by iatrogenic damage to the DPA are difficult to identify intraoperatively. In knee arthroscopy, risk of popliteal artery damage is reduced in the flexed position [1]. This study investigates how DPA movement is affected by dorsiflexion and plantarflexion with the aim of identifying the positions providing the greatest AWA.
| To investigate whether sex affects the trajectory of functional recovery after total knee arthroplasty (TKA).
| To determine whether magnetic resonance imaging (MRI) evidence of tendinopathy in early rheumatoid arthritis (RA) could be used to predict the course of tendon involvement in later disease and specifically the risk of tendon rupture.
| With the advent of MRI (Magnetic Resonance Imaging), Synovial lesions around knee are being more and more easily detected. Synovial lesions of knee present with boggy swelling, effusion, pain, and restriction of motion. Differential diagnoses of such lesions include pigmented villonodular synovitis, synovial lipoma, synovial chondromatosis, rheumatoid arthritis, synovial hemangioma, amyloid arthropathy, xanthomata and lipoma arborescens. CT and MRI often help in diagnosis of such lesions. MRI of Lipoma Arborescens has been regarded to have characteristic diagnostic appearance - it includes a synovial mass with frond-like architecture and fat signal intensity on all pulse sequences. Sometimes Lipoma Arborescens can present in conjunction with inflammatory arthritis. Synovectomy is often curative for such conditions.
| Joint trauma can lead to a spectrum of acute lesions, including cartilage degradation, ligament or meniscus tears, and synovitis, all potentially associated with osteoarthritis (OA). This study was undertaken to generate and validate a murine model of knee joint trauma following noninvasive controlled injurious compression in vivo.
| To determine which subregions of the knee joint have a high prevalence of pre-radiographic osteoarthritic changes, i.e., cartilage damage and osteophytes that can only be detected by magnetic resonance imaging (MRI), in radiographically normal knees.
| 78 of initially 84 patients (80 of 86 knees) were clinically and radiographically reassessed 5 (5.1-5.9) years after conventional, image-based, and image-free total knee arthroplasty. The methodology was identical to that used preoperatively and at 2 years, including the Knee Society score (KSS) and the functional score (FS), and AP and true lateral standard radiographs.
| In adult patients with trauma, an increase in the thickness of the retropharyngeal soft tissues is commonly used as a potential indicator of occult injury, but no studies have examined this parameter using computed tomography (CT) as a screening modality.
| To evaluate the thickness of cartilage at the posterior aspect of the medial and lateral condyle in Osteoarthritis (OA) knees compared to non-OA knees using computed tomography arthrography (CTA).
| Total knee arthroplasty (TKA) successfully alleviates pain from knee osteoarthritis; but deficits in function can persist long term. Despite these well-known deficits, there is little evidence supporting the use of rehabilitation interventions following TKA.
| The anterior intermeniscal ligament of the knee is at risk during knee arthroscopy, anterior cruciate ligament reconstruction, and tibial nail insertion.
| It has been previously demonstrated that radiographic severity of arthritis predicts outcome following knee replacement. In certain circumstances, patients may undergo arthroplasty without severe radiographic disease. An example may be the patient with significant chondral damage unsuccessfully treated with arthroscopy. This patient may proceed to joint replacement when their radiographs would not normally merit such intervention. We investigated whether these findings were also applicable to total ankle replacements (TARs).
| There is an increasing body of evidence that magnetic resonance imaging-occult tissue damage is an important component of primary progressive multiple sclerosis (PPMS) pathology. Proton magnetic resonance spectroscopy (1H-MRS) can be used to measure in vivo whole-brain N-acetylaspartate (WBNAA) concentrations, the decrease of whose levels is considered a marker of neuronal-axonal injury.
| Meniscectomy and articular cartilage damage have been found to increase the prevalence of osteoarthritis after anterior cruciate ligament reconstruction, but the effect of knee range of motion has not been extensively studied.
| Patients 50 years and older with knee osteoarthritis who underwent arthroscopy between 1998 and 2010 were retrospectively identified and an annual arthroscopy rate was calculated from 1998 through 2002 and from 2006 through 2010. Patients who underwent knee arthroplasty within 2 years of arthroscopy during each period were identified, and a 2-year conversion to arthroplasty rate was calculated.
|
* Loss: [MatryoshkaLoss
](https://sbert.net/docs/package_reference/sentence_transformer/losses.html#matryoshkaloss) with these parameters:
```json
{
"loss": "MultipleNegativesRankingLoss",
"matryoshka_dims": [
1024,
512,
256,
128,
64,
32
],
"matryoshka_weights": [
1,
1,
1,
1,
1,
1
],
"n_dims_per_step": -1
}
```
miracl
* Dataset: [miracl](https://huggingface.co./datasets/sentence-transformers/miracl) at [07e2b62](https://huggingface.co./datasets/sentence-transformers/miracl/tree/07e2b629250bf4185f4c87f640fac15949b8aa73)
* Size: 789,900 training samples
* Columns: anchor
, positive
, and negative
* Approximate statistics based on the first 1000 samples:
| | anchor | positive | negative |
|:--------|:------------------------------------------------------------------------------------------------|:--------------------------------------------------------------------------------------------------|:--------------------------------------------------------------------------------------------------|
| type | string | string | string |
| details | - min: 11 characters
- mean: 39.23 characters
- max: 129 characters
| - min: 72 characters
- mean: 745.86 characters
- max: 4292 characters
| - min: 18 characters
- mean: 649.52 characters
- max: 3570 characters
|
* Samples:
| anchor | positive | negative |
|:-------------------------------------------------------|:------------------------------------------------------------------------------------------------------------------------------------------------------------------------------------------------------------------------------------------------------------------------------------------------------------------------------------------------------------------------------------------------------------------------------------------------------------------------------------------------------------------------------------------------------------------------------------------------------------------------------------------------------------------------------------------------------------------------------------------------------------------------------------------------------------------------------------------------------------------------------------------------------------------------------------------------------------------------------------------------------------------------------------------------------------|:------------------------------------------------------------------------------------------------------------------------------------------------------------------------------------------------------------------------------------------------------------------------------------------------------------------------------------------------------------------------------------------------------------------------------------------------------------------------------------------------------------------------------------------------------------------------------------------------------------------------------------------------------------------------------------------------------------------------------------------------------------------------------------------------------------------------------------------------------------------------------------------------------------------------------------------------------------------------------------------------------------------------------------------------------------|
| Who created The Walking Dead comic books?
| Days Gone Bye (The Walking Dead)
Robert Kirkman, the creator of the eponymous series of comic books, considered the idea of creating a television show based on the comic series, but did not move forward. Frank Darabont expressed interest in developing the series for television. In January 2010, AMC formally announced that it had ordered a pilot for a possible series adapted from "The Walking Dead" comic book. In the announcement, the executives stated that Darabont would serve as writer, director, and an executive producer alongside Gale Anne Hurd.
| Living Dead
The Walking Dead
|
| When was the first car invented?
| Car
In 1879, Benz was granted a patent for his first engine, which had been designed in 1878. Many of his other inventions made the use of the internal combustion engine feasible for powering a vehicle. His first "Motorwagen" was built in 1885 in Mannheim, Germany. He was awarded the patent for its invention as of his application on 29 January 1886 (under the auspices of his major company, Benz & Cie., which was founded in 1883). Benz began promotion of the vehicle on 3 July 1886, and about 25 Benz vehicles were sold between 1888 and 1893, when his first four-wheeler was introduced along with a model intended for affordability. They also were powered with four-stroke engines of his own design. Emile Roger of France, already producing Benz engines under license, now added the Benz car to his line of products. Because France was more open to the early cars, initially more were built and sold in France through Roger than Benz sold in Germany. In August 1888 Bertha Benz, the wife of Karl B...
| Elwood Haynes
In 1905, three years after the Apperson brothers split from Haynes, Haynes-Apperson was renamed the Haynes Automobile Company and Haynes launched a series of publicity campaigns. A parade of 2,000 cars was organized in New York City during 1908 and Haynes, whom many recognized as the inventor of the American automobile, led the parade down Broadway riding in the "Pioneer". He was followed by ten Haynes cars, a model from each year to display the advancement in technology. On his way to the parade, Haynes was unaware of the city's newly established speeding laws and was arrested for driving too fast—in a car with a top speed of 15 mph (17 km/h)—and taken to jail. He was soon able to see a magistrate who released him after learning that he was Elwood Haynes and had come to lead the parade. The celebration was intended to be a ten-year commemoration of the invention of the automobile, although earlier self-vehicles dated back nearly twenty years in Europe. Haynes donated the...
|
| How many doctors are in Doctor Who?
| The Doctor (Doctor Who)
The Doctor is the title character in the long-running BBC science fiction television programme "Doctor Who". Since the show's inception in 1963, the character has been portrayed by thirteen lead actors. In the programme, "the Doctor" is the alias assumed by a centuries-old alien—a Time Lord from the planet Gallifrey—who travels through space and time in the TARDIS, frequently with companions. The transition to each succeeding actor is explained within the show's narrative through the plot device of "regeneration", a biological function of the Time Lord race that allows a change of cellular structure and appearance with recovery following a potentially fatal injury.
| Sixth Doctor
The Sixth Doctor is an incarnation of the Doctor, the protagonist of the BBC science fiction television series "Doctor Who". He is portrayed by Colin Baker. Although his televisual time on the series was comparatively brief and turbulent, Baker has continued as the Sixth Doctor in Big Finish's range of original "Doctor Who" audio adventures. Within the series' narrative, the Doctor is a centuries-old Time Lord alien from the planet Gallifrey who travels in time and space in his TARDIS, frequently with companions. At the end of life, the Doctor can regenerate his body; in doing so, his physical appearance and personality change. Baker portrays the sixth such incarnation, an arrogant, flamboyant character in brightly coloured, mismatched clothes whose brash, often patronising personality set him apart from all his previous incarnations.
|
* Loss: [MatryoshkaLoss
](https://sbert.net/docs/package_reference/sentence_transformer/losses.html#matryoshkaloss) with these parameters:
```json
{
"loss": "MultipleNegativesRankingLoss",
"matryoshka_dims": [
1024,
512,
256,
128,
64,
32
],
"matryoshka_weights": [
1,
1,
1,
1,
1,
1
],
"n_dims_per_step": -1
}
```
mldr
* Dataset: [mldr](https://huggingface.co./datasets/sentence-transformers/mldr) at [40ad767](https://huggingface.co./datasets/sentence-transformers/mldr/tree/40ad7672817ebee49e00dd25aed00e1c401881d6)
* Size: 200,000 training samples
* Columns: anchor
, positive
, and negative
* Approximate statistics based on the first 1000 samples:
| | anchor | positive | negative |
|:--------|:------------------------------------------------------------------------------------------------|:--------------------------------------------------------------------------------------------------------|:--------------------------------------------------------------------------------------------------------|
| type | string | string | string |
| details | - min: 17 characters
- mean: 65.31 characters
- max: 210 characters
| - min: 2432 characters
- mean: 20354.29 characters
- max: 123500 characters
| - min: 3035 characters
- mean: 16236.77 characters
- max: 166364 characters
|
* Samples:
| anchor | positive | negative |
|:-----------------------------------------------------------------------------------------------------------------|:---------------------------------------------------------------------------------------------------------------------------------------------------------------------------------------------------------------------------------------------------------------------------------------------------------------------------------------------------------------------------------------------------------------------------------------------------------------------------------------------------------------------------------------------------------------------------------------------------------------------------------------------------------------------------------------------------------------------------------------------------------------------------------------------------------------------------------------------------------------------------------------------------------------------------------------------------------------------------------------------------------------------------------------------------------------------------------|:---------------------------------------------------------------------------------------------------------------------------------------------------------------------------------------------------------------------------------------------------------------------------------------------------------------------------------------------------------------------------------------------------------------------------------------------------------------------------------------------------------------------------------------------------------------------------------------------------------------------------------------------------------------------------------------------------------------------------------------------------------------------------------------------------------------------------------------------------------------------------------------------------------------------------------------------------------------------------------------------------------------------------------------------------------------------------|
| When was the art museum in Santa Barbara first opened to the public?
| The Santa Barbara Museum of Art (SBMA) is an art museum located in downtown Santa Barbara, California.
Founded in 1941, it is home to both permanent and special collections, the former of which includes Asian, American, and European art that spans 4,000 years from ancient to modern.
History
The Santa Barbara Museum of Art opened to the public on June 5, 1941, in a building that was at one time the Santa Barbara Post Office (1914–1932). The idea for an art museum first came from the local artist Colin Campbell Cooper when he learned that the post office was going to be sold. In a letter to the editor published in the Santa Barbara News-Press in July 1937, Cooper proposed that the impressive Italianate structure should be transformed into a museum. After gaining momentum in town and with the support of local businesses, politicians and art collectors the Santa Barbara Museum of Art was officially established just four years after Cooper's letter was published. The renowned Chicago arc...
| The Knott's Berry Farm amusement park in Orange County, California, originated from a berry farm owned by Walter Knott (1889–1981). In the 1920s, Knott and his wife, Cordelia, sold berries, berry preserves and pies from a roadside stand beside State Route 39, near the small town of Buena Park.
In 1932, on a visit to Rudolph Boysen's farm in nearby Anaheim, Walter Knott was introduced to a new hybrid berry of a blackberry, a red raspberry, and a loganberry cross-bred by Boysen, who gave Walter his last six wilted berry-hybrid plants. Walter planted and cultivated them, then the family sold the berries at their roadside stand. When people asked what kind they were, he called them "boysenberries".
In 1934, to make ends meet, Knott's wife Cordelia (1890–1974) reluctantly began serving fried chicken dinners on their wedding china. For dessert, Knott's signature Boysenberry Pie was also served to guests dining in the small tea room. As Southern California developed, Highway 39 became the ma...
|
| What is the objective of Opération Chammal?
| Opération Chammal is a French military operation in Iraq and Syria in an attempt to contain the expansion of the Islamic State of Iraq and the Levant and to support the Iraqi Army. Its name comes from the Shamal (Chammal in French), a northwesterly wind that blows over Iraq and the Persian Gulf states.
Airstrikes over Iraq started 19 September 2014 and airstrikes over Syria started by the end of September 2015. The French operation is limited to airstrikes; French president François Hollande has reiterated that no ground troops would be used in the conflict. Additionally, the French frigate has joined the United States Navy's Commander Task Force 50 (CTF 50) as an escort.
On 14 November 2015, ISIL claimed that the attacks that took place in Paris the previous day were retaliation for Opération Chammal. In response, French forces increased their attacks against ISIL in Syria.
Background
On 10 June 2014, the terrorist group of the Islamic State of Iraq and the Levant and several ot...
| CMA CGM S.A. is a French container transportation and shipping company. It is the world’s 3rd largest container shipping company, using 257 shipping routes between 420 ports in 160 different countries. Its headquarters are in Marseille, France and its North American headquarters are in Norfolk, Virginia, United States.
The name is an acronym of two predecessor companies, Compagnie Maritime d'Affrètement (CMA) and Compagnie Générale Maritime (CGM), which translate as "Maritime Freighting Company" and "General Maritime Company".
History
The history of CMA CGM can be traced back to the middle of the 19th century, when two major French shipping lines were created, respectively Messageries Maritimes (MM) in 1851 and Compagnie Générale Maritime (CGM) in 1855, soon renamed Compagnie Générale Transatlantique in 1861. Both companies were created partly with the backing of the French State, through the award of mail contracts to various destinations, French colonies and overseas territories a...
|
| What was the reason for Williams-Franklin's decision to become a vegan during her time as a player?
| Taj McWilliams-Franklin (born October 20, 1970) is a former American professional women's basketball player.
A two-time WNBA champion with the Detroit Shock and Minnesota Lynx and six-time all-star, McWilliams-Franklin's professional career has spanned three decades, and began before the WNBA was founded. She retired from the WNBA after the 2012 season.
College years
After attending T. W. Josey High School in Augusta, Georgia, McWilliams-Franklin attended Georgia State University in 1989 and played on the school's basketball team for one season. However, she had become pregnant during her senior year in high school, and after the coach who recruited her to Georgia State was let go, the incoming staff told her "school was no place for kids." McWilliams-Franklin moved to Austin, Texas, where a friend connected her with St. Edward's University coach Dave McKey. She enrolled at St. Edwards as a Rhetoric major.
While at St. Edward's, she set school records and individual achievements, in...
| Spider-Woman (Gwendolyne Maxine Stacy; colloquial: "Spider-Gwen" or "Ghost-Spider") is a superhero appearing in American comic books published by Marvel Comics. She was created by Jason Latour and Robbi Rodriguez. The character debuted in Edge of Spider-Verse issue #2 as part of the 2014–15 "Spider-Verse" comic book storyline, leading to the ongoing series Spider-Gwen that began in 2015.
Spider-Woman is a variant of Spider-Man and an alternate-universe version of Gwen Stacy. She lives on Earth-65, where Gwen Stacy is bitten by a radioactive spider and becomes a superhero instead of Peter Parker becoming Spider-Man. The character's various enemies include Earth-65 versions of Matt Murdock and Frank Castle. Gwen Stacy's Spider-Woman harbors much of Peter's personality and conflicts along with his powers and abilities.
Spider-Woman was met with positive reviews from critics, with them applauding her design—cited as a popular choice for cosplay—and a feminist perspective. For promotion, ...
|
* Loss: [MatryoshkaLoss
](https://sbert.net/docs/package_reference/sentence_transformer/losses.html#matryoshkaloss) with these parameters:
```json
{
"loss": "MultipleNegativesRankingLoss",
"matryoshka_dims": [
1024,
512,
256,
128,
64,
32
],
"matryoshka_weights": [
1,
1,
1,
1,
1,
1
],
"n_dims_per_step": -1
}
```
mr_tydi
* Dataset: [mr_tydi](https://huggingface.co./datasets/sentence-transformers/mr-tydi) at [abbdf55](https://huggingface.co./datasets/sentence-transformers/mr-tydi/tree/abbdf55c630352da943f779610c3ce6268118351)
* Size: 354,700 training samples
* Columns: anchor
, positive
, and negative
* Approximate statistics based on the first 1000 samples:
| | anchor | positive | negative |
|:--------|:-----------------------------------------------------------------------------------------------|:--------------------------------------------------------------------------------------------------|:--------------------------------------------------------------------------------------------------|
| type | string | string | string |
| details | - min: 12 characters
- mean: 38.85 characters
- max: 95 characters
| - min: 64 characters
- mean: 645.85 characters
- max: 4067 characters
| - min: 21 characters
- mean: 626.85 characters
- max: 2870 characters
|
* Samples:
| anchor | positive | negative |
|:----------------------------------------------------------|:------------------------------------------------------------------------------------------------------------------------------------------------------------------------------------------------------------------------------------------------------------------------------------------------------------------------------------------------------------------------------------------------------------------------------------------------------------------------------------------------------------------------------------------------------------------------------------------------------------------------------------------------------------------------------------------------------------------------------------------------------------------------------------------------------------------------------------------------------------------------------------------------------------------------------------------------------------------------------------------------------------------------------------------------------------|:------------------------------------------------------------------------------------------------------------------------------------------------------------------------------------------------------------------------------------------------------------------------------------------------------------------------------------------------------------------------------------------------------------------------------------------------------------------------------------------------------------------------------------------------------------------------------------------------------------------------------------------------------------------------------------------------------------------------------------------------------------------------------------------------------------------------------------------------------------------------------------------------------------------------------------------------------------------------------------------------------------------------------------------------------------|
| Is amnesia real?
| Amnesia
Amnesia is a deficit in memory caused by brain damage, disease, or psychological trauma.[1] Amnesia can also be caused temporarily by the use of various sedatives and hypnotic drugs. The memory can be either wholly or partially lost due to the extent of damage that was caused.[2] There are two main types of amnesia: retrograde amnesia and anterograde amnesia. Retrograde amnesia is the inability to retrieve information that was acquired before a particular date, usually the date of an accident or operation.[3] In some cases the memory loss can extend back decades, while in others the person may lose only a few months of memory. Anterograde amnesia is the inability to transfer new information from the short-term store into the long-term store. People with this type of amnesia cannot remember things for long periods of time. These two types are not mutually exclusive; both can occur simultaneously.
| Amnesia
Head trauma is a very broad range as it deals with any kind of injury or active action toward the brain which might cause amnesia. Retrograde and anterograde amnesia is more often seen from events like this, an exact example of a cause of the two would be electroconvulsive therapy, which would cause both briefly for the receiving patient. Traumatic events are more subjective. What is traumatic is dependent on what the person finds to be traumatic. Regardless, a traumatic event is an event where something so distressing occurs that the mind chooses to forget rather than deal with the stress. A common example of amnesia that is caused by traumatic events is dissociative amnesia, which occurs when the person forgets an event that has deeply disturbed them.[8] An example would be a person forgetting a fatal and graphic car accident involving their loved ones. Physical deficiencies are different from head trauma because physical deficiencies lean more toward passive physical issues.
|
| What is the largest naval base in the world?
| Naval Station Norfolk
Naval Station Norfolk, is a United States Navy base in Norfolk, Virginia. It supports naval forces in the United States Fleet Forces Command,[1] those operating in the Atlantic Ocean, Mediterranean Sea, and the Indian Ocean. The installation occupies about 4 miles (6.4km) of waterfront space and 11 miles (18km) of pier and wharf space of the Hampton Roads peninsula known as Sewell's Point. It is the world's largest naval station, with the largest concentration of U.S. Navy forces through 75 ships alongside 14 piers and with 134 aircraft and 11 aircraft hangars at the adjacently operated Chambers Field and [2] Port Services controls more than 3,100 ships' movements annually as they arrive and depart their berths.
| Clark Air Base
Clark Air Base was arguably the most urbanized military facility in history and was the largest American base overseas. At its peak around 1990, it had a permanent population of 15,000. It had a base exchange, a large commissary, a small shopping arcade, a branch department store, cafeterias, teen centers, a hotel, miniature golf, riding stables, zoo, and other concessions.
|
| What is the power of the Red Lantern?
| Red Lantern Corps
In Final Crisis: Rage of the Red Lanterns, Atrocitus is shown in a flashback as having apparently formed a central power battery by using the blood of the other Inversions in blood magic rituals. The battery stands before a great lake of blood from which he forms his red power ring (crystallized by his anger), as well as other rings and batteries used to form the Red Lantern Corps. Harnessing the red light of rage, he sends his rings out into the universe; however, upon accepting the rings, his recruits' hearts are rendered useless. Their blood spoils from within, forcing them to expel the violently flammable and corrosive material from their mouths. Additionally, the Red Lanterns are reduced to an almost animalistic state, with only Atrocitus appearing to be in full control of himself. Once Atrocitus assembles a sufficient force, he leads them on a mission to capture Sinestro (who is being transferred to Korugar for his execution). Coincidentally, the Sinestro Corps ...
| Green Lantern in other media
John Stewart is a member of the Justice League in the "Justice League" animated series. In this series, Stewart's ring was initially constrained to permitting him to fly, generating a protective force field, creating walls, and firing energy blasts; this limitation was established as being due to Stewart's mindset, not an inherent limitation of the ring itself (the series' version of Stewart is a former U.S. Marine, not an architect). After being berated by Katma Tui for his unimaginative use of the ring, Stewart has learned to generate complex tools (to defuse a bomb in one instance) and weapons. (He was also shown to be more creative when transformed into a child in the episode "Kids Stuff".) In a development not seen in any other version of the Green Lantern mythos, Stewart's eyes glow green when wearing his charged power ring. The glow fades when the ring runs out of power. The series has been inconsistent about the ring's effectiveness against yellow; ...
|
* Loss: [MatryoshkaLoss
](https://sbert.net/docs/package_reference/sentence_transformer/losses.html#matryoshkaloss) with these parameters:
```json
{
"loss": "MultipleNegativesRankingLoss",
"matryoshka_dims": [
1024,
512,
256,
128,
64,
32
],
"matryoshka_weights": [
1,
1,
1,
1,
1,
1
],
"n_dims_per_step": -1
}
```
### Evaluation Datasets
gooaq
* Dataset: [gooaq](https://huggingface.co./datasets/sentence-transformers/gooaq) at [b089f72](https://huggingface.co./datasets/sentence-transformers/gooaq/tree/b089f728748a068b7bc5234e5bcf5b25e3c8279c)
* Size: 3,012,496 evaluation samples
* Columns: question
and answer
* Approximate statistics based on the first 1000 samples:
| | question | answer |
|:--------|:-----------------------------------------------------------------------------------------------|:-------------------------------------------------------------------------------------------------|
| type | string | string |
| details | - min: 18 characters
- mean: 43.17 characters
- max: 98 characters
| - min: 51 characters
- mean: 254.12 characters
- max: 360 characters
|
* Samples:
| question | answer |
|:-----------------------------------------------------------------------------|:-------------------------------------------------------------------------------------------------------------------------------------------------------------------------------------------------------------------------------------------------------------------------------------------------------------------------------------------|
| how do i program my directv remote with my tv?
| ['Press MENU on your remote.', 'Select Settings & Help > Settings > Remote Control > Program Remote.', 'Choose the device (TV, audio, DVD) you wish to program. ... ', 'Follow the on-screen prompts to complete programming.']
|
| are rodrigues fruit bats nocturnal?
| Before its numbers were threatened by habitat destruction, storms, and hunting, some of those groups could number 500 or more members. Sunrise, sunset. Rodrigues fruit bats are most active at dawn, at dusk, and at night.
|
| why does your heart rate increase during exercise bbc bitesize?
| During exercise there is an increase in physical activity and muscle cells respire more than they do when the body is at rest. The heart rate increases during exercise. The rate and depth of breathing increases - this makes sure that more oxygen is absorbed into the blood, and more carbon dioxide is removed from it.
|
* Loss: [MatryoshkaLoss
](https://sbert.net/docs/package_reference/sentence_transformer/losses.html#matryoshkaloss) with these parameters:
```json
{
"loss": "MultipleNegativesRankingLoss",
"matryoshka_dims": [
1024,
512,
256,
128,
64,
32
],
"matryoshka_weights": [
1,
1,
1,
1,
1,
1
],
"n_dims_per_step": -1
}
```
msmarco
* Dataset: [msmarco](https://huggingface.co./datasets/sentence-transformers/msmarco-co-condenser-margin-mse-sym-mnrl-mean-v1) at [84ed2d3](https://huggingface.co./datasets/sentence-transformers/msmarco-co-condenser-margin-mse-sym-mnrl-mean-v1/tree/84ed2d35626f617d890bd493b4d6db69a741e0e2)
* Size: 502,939 evaluation samples
* Columns: query
, positive
, and negative
* Approximate statistics based on the first 1000 samples:
| | query | positive | negative |
|:--------|:------------------------------------------------------------------------------------------------|:-------------------------------------------------------------------------------------------------|:-------------------------------------------------------------------------------------------------|
| type | string | string | string |
| details | - min: 10 characters
- mean: 33.36 characters
- max: 137 characters
| - min: 67 characters
- mean: 347.87 characters
- max: 906 characters
| - min: 57 characters
- mean: 318.18 characters
- max: 906 characters
|
* Samples:
| query | positive | negative |
|:-------------------------------------------------|:-------------------------------------------------------------------------------------------------------------------------------------------------------------------------------------------------------------------------------------------------------------------------------------------------------------------------------------------|:------------------------------------------------------------------------------------------------------------------------------------------------------------------------------------------------------------------------------------------------------------------------------------------------------------------------------------------|
| is cabinet refacing worth the cost?
| Fans of refacing say this mini-makeover can give a kitchen a whole new look at a much lower cost than installing all-new cabinets. Cabinet refacing can save up to 50 percent compared to the cost of replacing, says Cheryl Catalano, owner of Kitchen Solvers, a cabinet refacing franchise in Napierville, Illinois. From.
| Most cabinet refacing projects cost about $4,000 to $10,000. The price varies based on the materials you select and the size and configuration of your kitchen. Wood veneer doors, for example, will cost less than solid wood doors.
|
| is the fovea ethmoidalis a bone
| Ethmoid bone/fovea ethmoidalis. The medial portion of the ethmoid bone is a cruciate membranous bone composed of the crista galli, cribriform plate, and perpendicular ethmoidal plate. The crista is a thick piece of bone, shaped like a âcock's comb,â that projects intracranially and attaches to the falx cerebri.
| Ethmoid bone/fovea ethmoidalis. The medial portion of the ethmoid bone is a cruciate membranous bone composed of the crista galli, cribriform plate, and perpendicular ethmoidal plate. The crista is a thick piece of bone, shaped like a âcock's comb,â that projects intracranially and attaches to the falx cerebri.
|
| average pitches per inning
| The likelihood of a pitcher completing nine innings if he throws an average of 14 pitches or less per inning is reinforced by the totals of the 89 games in which pitchers did actually complete nine innings of work.
| The likelihood of a pitcher completing nine innings if he throws an average of 14 pitches or less per inning is reinforced by the totals of the 89 games in which pitchers did actually complete nine innings of work.
|
* Loss: [MatryoshkaLoss
](https://sbert.net/docs/package_reference/sentence_transformer/losses.html#matryoshkaloss) with these parameters:
```json
{
"loss": "MultipleNegativesRankingLoss",
"matryoshka_dims": [
1024,
512,
256,
128,
64,
32
],
"matryoshka_weights": [
1,
1,
1,
1,
1,
1
],
"n_dims_per_step": -1
}
```
squad
* Dataset: [squad](https://huggingface.co./datasets/sentence-transformers/squad) at [d84c8c2](https://huggingface.co./datasets/sentence-transformers/squad/tree/d84c8c2ef64693264c890bb242d2e73fc0a46c40)
* Size: 87,599 evaluation samples
* Columns: question
and answer
* Approximate statistics based on the first 1000 samples:
| | question | answer |
|:--------|:-----------------------------------------------------------------------------------------------|:---------------------------------------------------------------------------------------------------|
| type | string | string |
| details | - min: 1 characters
- mean: 60.25 characters
- max: 161 characters
| - min: 152 characters
- mean: 761.88 characters
- max: 2525 characters
|
* Samples:
| question | answer |
|:----------------------------------------------------------------------------------------------------------|:---------------------------------------------------------------------------------------------------------------------------------------------------------------------------------------------------------------------------------------------------------------------------------------------------------------------------------------------------------------------------------------------------------------------------------------------------------------------------------------------------------------------------------------------------------------------------------------------------------------------------------------------------------------------------------------------------------------------------------------------------------------------------------------------------------------------------------------------------------------------------------------------------------------------------------------------------------------------------------------------------------------------------------------------------------|
| When did the Russian Empire begin to question the existence of the Ottoman Empire?
| In 1853 the Russian Empire on behalf of the Slavic Balkan states began to question the very existence of the Ottoman Empire. The result was the Crimean War, 1853–1856, in which the British Empire and the French Empire supported the Ottoman Empire in its struggle against the incursions of the Russian Empire. Eventually, the Ottoman Empire lost control of the Balkan region.
|
| How would one describe the control of universities before nation-states in the 17th century?
| The propagation of universities was not necessarily a steady progression, as the 17th century was rife with events that adversely affected university expansion. Many wars, and especially the Thirty Years' War, disrupted the university landscape throughout Europe at different times. War, plague, famine, regicide, and changes in religious power and structure often adversely affected the societies that provided support for universities. Internal strife within the universities themselves, such as student brawling and absentee professors, acted to destabilize these institutions as well. Universities were also reluctant to give up older curricula, and the continued reliance on the works of Aristotle defied contemporary advancements in science and the arts. This era was also affected by the rise of the nation-state. As universities increasingly came under state control, or formed under the auspices of the state, the faculty governance model (begun by the University of Paris) became more and m...
|
| When did Jewish law recognize copyright?
| The concept's origins can potentially be traced back further. Jewish law includes several considerations whose effects are similar to those of modern intellectual property laws, though the notion of intellectual creations as property does not seem to exist – notably the principle of Hasagat Ge'vul (unfair encroachment) was used to justify limited-term publisher (but not author) copyright in the 16th century. In 500 BCE, the government of the Greek state of Sybaris offered one year's patent "to all who should discover any new refinement in luxury".
|
* Loss: [MatryoshkaLoss
](https://sbert.net/docs/package_reference/sentence_transformer/losses.html#matryoshkaloss) with these parameters:
```json
{
"loss": "MultipleNegativesRankingLoss",
"matryoshka_dims": [
1024,
512,
256,
128,
64,
32
],
"matryoshka_weights": [
1,
1,
1,
1,
1,
1
],
"n_dims_per_step": -1
}
```
s2orc
* Dataset: [s2orc](https://huggingface.co./datasets/sentence-transformers/s2orc) at [8cfc394](https://huggingface.co./datasets/sentence-transformers/s2orc/tree/8cfc394e83b2ebfcf38f90b508aea383df742439)
* Size: 10,000 evaluation samples
* Columns: title
and abstract
* Approximate statistics based on the first 1000 samples:
| | title | abstract |
|:--------|:------------------------------------------------------------------------------------------------|:--------------------------------------------------------------------------------------------------|
| type | string | string |
| details | - min: 31 characters
- mean: 80.04 characters
- max: 198 characters
| - min: 96 characters
- mean: 653.93 characters
- max: 1023 characters
|
* Samples:
| title | abstract |
|:-------------------------------------------------------------------------------------------------------------|:---------------------------------------------------------------------------------------------------------------------------------------------------------------------------------------------------------------------------------------------------------------------------------------------------------------------------------------------------------------------------------------------------------------------------------------------------------------------------------------------------------------------------------------------------------------------------------------------------------------------------------------------------------------------------------------------------------------------------------------------------------------------------------------------------------------------------------------------------------------------------------------------------------------------------------------------------------------------------------------------------------------------------------------------------------|
| Screen Printing Ink Film Thickness Analysis of the Passive RFID Tag Antenna
| The relationship between the screen mesh and the theoretical and practical ink film thickness was analyzed based on the main influencing factors of the ink film thickness by screen printing.A calculation model for the ink thickness was established based on the screen under static and compressive deformation.The relation curve between the screen mesh and the ink film thickness was fitted and the suitable printing craft parameter was chosen to print two kinds of RFID tag antennas.The fluctuation of the antenna resistance was analyzed to demonstrate the reliability of the passive RFID tag antenna manufactured by screen printing technology.
|
| Subclinical organ damage and cardiovascular risk prediction
| AbstractTraditional cardiovascular risk factors have poor prognostic value for individuals and screening for subclinical organ damage has been recommended in hypertension in recent guidelines. The aim of this review was to investigate the clinical impact of the additive prognostic information provided by measuring subclinical organ damage. We have (i) reviewed recent studies linking markers of subclinical organ damage in the heart, blood vessels and kidney to cardiovascular risk; (ii) discussed the evidence for improvement in cardiovascular risk prediction using markers of subclinical organ damage; (iii) investigated which and how many markers to measure and (iv) finally discussed whether measuring subclinical organ damage provided benefits beyond risk prediction. In conclusion, more studies and if possible randomized studies are needed to investigate (i) the importance of markers of subclinical organ damage for risk discrimination, calibration and reclassification; and (ii) the econom...
|
| A Novel Approach to Simulate Climate Change Impacts on Vascular Epiphytes: Case Study in Taiwan
| In the wet tropics, epiphytes form a conspicuous layer in the forest canopy, support abundant coexisting biota, and are known to have a critical influence on forest hydrology and nutrient cycling. Since canopy-dwelling plants have no vascular connection to the ground or their host plants, they are likely more sensitive to environmental changes than their soil-rooted counterparts, subsequently regarded as one of the groups most vulnerable to global climate change. Epiphytes have adapted to life in highly dynamic forest canopies by producing many, mostly wind-dispersed, seeds or spores. Consequently, epiphytes should colonize trees rapidly, which, in addition to atmospheric sensitivity and short life cycles, make epiphytes suitable climate change indicators. In this study, we assess the impact of climate change on Taiwanese epiphytes using a modeling approach.
|
* Loss: [MatryoshkaLoss
](https://sbert.net/docs/package_reference/sentence_transformer/losses.html#matryoshkaloss) with these parameters:
```json
{
"loss": "MultipleNegativesRankingLoss",
"matryoshka_dims": [
1024,
512,
256,
128,
64,
32
],
"matryoshka_weights": [
1,
1,
1,
1,
1,
1
],
"n_dims_per_step": -1
}
```
allnli
* Dataset: [allnli](https://huggingface.co./datasets/sentence-transformers/all-nli) at [d482672](https://huggingface.co./datasets/sentence-transformers/all-nli/tree/d482672c8e74ce18da116f430137434ba2e52fab)
* Size: 6,584 evaluation samples
* Columns: anchor
, positive
, and negative
* Approximate statistics based on the first 1000 samples:
| | anchor | positive | negative |
|:--------|:------------------------------------------------------------------------------------------------|:------------------------------------------------------------------------------------------------|:------------------------------------------------------------------------------------------------|
| type | string | string | string |
| details | - min: 15 characters
- mean: 72.82 characters
- max: 300 characters
| - min: 12 characters
- mean: 34.11 characters
- max: 126 characters
| - min: 11 characters
- mean: 36.38 characters
- max: 121 characters
|
* Samples:
| anchor | positive | negative |
|:-------------------------------------------------------------------------------------------------------------------------------------------------------------------------------|:------------------------------------------------------------|:--------------------------------------------------------|
| Two women are embracing while holding to go packages.
| Two woman are holding packages.
| The men are fighting outside a deli.
|
| Two young children in blue jerseys, one with the number 9 and one with the number 2 are standing on wooden steps in a bathroom and washing their hands in a sink.
| Two kids in numbered jerseys wash their hands.
| Two kids in jackets walk to school.
|
| A man selling donuts to a customer during a world exhibition event held in the city of Angeles
| A man selling donuts to a customer.
| A woman drinks her coffee in a small cafe.
|
* Loss: [MatryoshkaLoss
](https://sbert.net/docs/package_reference/sentence_transformer/losses.html#matryoshkaloss) with these parameters:
```json
{
"loss": "MultipleNegativesRankingLoss",
"matryoshka_dims": [
1024,
512,
256,
128,
64,
32
],
"matryoshka_weights": [
1,
1,
1,
1,
1,
1
],
"n_dims_per_step": -1
}
```
paq
* Dataset: [paq](https://huggingface.co./datasets/sentence-transformers/paq) at [74601d8](https://huggingface.co./datasets/sentence-transformers/paq/tree/74601d8d731019bc9c627ffc4271cdd640e1e748)
* Size: 64,371,441 evaluation samples
* Columns: query
and answer
* Approximate statistics based on the first 1000 samples:
| | query | answer |
|:--------|:-----------------------------------------------------------------------------------------------|:--------------------------------------------------------------------------------------------------|
| type | string | string |
| details | - min: 25 characters
- mean: 51.3 characters
- max: 108 characters
| - min: 504 characters
- mean: 623.09 characters
- max: 835 characters
|
* Samples:
| query | answer |
|:---------------------------------------------------------------|:-----------------------------------------------------------------------------------------------------------------------------------------------------------------------------------------------------------------------------------------------------------------------------------------------------------------------------------------------------------------------------------------------------------------------------------------------------------------------------------------------------------------------------------------------------------------------------------------------------------------------------|
| when did season 3 of the voice brasil start
| The Voice Brasil (season 3) The third season of "The Voice Brasil", premiered on Rede Globo on September 18, 2014 in the 10:30 p.m. (BRT/AMT) slot immediately following the primetime telenovela "Império". The 22- and 24-year-old sertanejo duo Danilo Reis e Rafael won the competition on December 25, 2014 with 43% of the votes cast. This marked Lulu Santos' first win as a coach, the first stolen artist to win a Brazilian season of "The Voice", and the first time in any "The Voice" franchise that a duo won the competition. Online applications for "The Voice Brasil" were open on
|
| when did the little ranger first come out
| Gang" theme song was an instrumental medley of "London Bridge", "Here We Go Round the Mulberry Bush" and "The Farmer in the Dell". It remained in use until the series ended in 1944. The Little Ranger The Little Ranger is a 1938 "Our Gang" short comedy film directed by Gordon Douglas. It was the 169th short in the "Our Gang" series, and the first produced by Metro-Goldwyn-Mayer, who purchased the rights to the series from creator Hal Roach. Snubbed by his girlfriend Darla, Alfalfa accepts the invitation of tomboyish Muggsy to attend the local picture show. While watching the adventures
|
| what is the name of rachel's sister in ninjaaiden
| her among ten female characters who have never been featured on their games' cover arts, Samir Torres of VentureBeat wrote that while "Team Ninja sexualy exploits all of their female characters, yet Rachel somehow got axed from every modern "Ninja Gaiden" box art." Rachel (Ninja Gaiden) In 2004's "Ninja Gaiden", Rachel is a fiend hunter whom the game's protagonist Ryu Hayabusa meets in the Holy Vigoor Empire, where she is on a mission to destroy the fiends, as well as find her missing sister, Alma, who has become a Greater Fiend. Soon after they first meet, she is captured but
|
* Loss: [MatryoshkaLoss
](https://sbert.net/docs/package_reference/sentence_transformer/losses.html#matryoshkaloss) with these parameters:
```json
{
"loss": "MultipleNegativesRankingLoss",
"matryoshka_dims": [
1024,
512,
256,
128,
64,
32
],
"matryoshka_weights": [
1,
1,
1,
1,
1,
1
],
"n_dims_per_step": -1
}
```
trivia_qa
* Dataset: [trivia_qa](https://huggingface.co./datasets/sentence-transformers/trivia-qa) at [a7c36e3](https://huggingface.co./datasets/sentence-transformers/trivia-qa/tree/a7c36e3c8c8c01526bc094d79bf80d4c848b0ad0)
* Size: 73,346 evaluation samples
* Columns: query
and answer
* Approximate statistics based on the first 1000 samples:
| | query | answer |
|:--------|:------------------------------------------------------------------------------------------------|:----------------------------------------------------------------------------------------------------|
| type | string | string |
| details | - min: 26 characters
- mean: 77.62 characters
- max: 258 characters
| - min: 135 characters
- mean: 3169.71 characters
- max: 4096 characters
|
* Samples:
| query | answer |
|:-----------------------------------------------------------------------------------------------------|:---------------------------------------------------------------------------------------------------------------------------------------------------------------------------------------------------------------------------------------------------------------------------------------------------------------------------------------------------------------------------------------------------------------------------------------------------------------------------------------------------------------------------------------------------------------------------------------------------------------------------------------------------------------------------------------------------------------------------------------------------------------------------------------------------------------------------------------------------------------------------------------------------------------------------------------------------------------------------------------------------------------------------------------------------------|
| In which country is 'Ninety Mile Beach'?
| Ninety (90) Mile Beach, Gippsland, Victoria - Tourism Australia Gippsland Find travel information on Ninety Mile Beach, one of the longest uninterrupted beaches in the world, located outside of Melbourne at Gippsland Lakes. Ninety Mile Beach, located in the Gippsland region on Victoria's south-eastern coastline, is one of the longest uninterrupted beaches in the world. Stand on the beach and watch the beach disappear into the salty sea spray in the distance. You might find that your footprints are the only ones in the sand that day. This is one of the most natural and unspoilt beaches in the world and is ideal for activities from beach fishing and swimming to walking, whale and dolphin-spotting or just lazing in the sun. Sun, sand and lush national parks all create the perfect holiday environment. Victoria's Ninety Mile Beach is a 90-mile long stretch of pristine golden sand that separates the Gippsland Lakes from Bass Strait. Stretching as far as the eye can see it is one of the most ...
|
| What country gets nearly 75% of its electricity from nuclear power?
| Nuclear Power in France | French Nuclear Energy - World Nuclear Association Nuclear Power in France (Updated November 2016) France derives about 75% of its electricity from nuclear energy, due to a long-standing policy based on energy security. This share may be reduced to 50% by 2025. France is the world's largest net exporter of electricity due to its very low cost of generation, and gains over €3 billion per year from this. France has been very active in developing nuclear technology. Reactors and especially fuel products and services are a significant export. About 17% of France's electricity is from recycled nuclear fuel. In 2014 French electricity generation was 541 TWh gross. Consumption in 2012 was 454 TWh – 6600 kWh per person. Winter demand varies by 2300 MWe per degree C. Over the last decade France has exported up to 70 billion kWh net each year and EdF expects exports to continue at 55-70 TWh/yr. In 2014 they were principally to Italy, UK, Switzerland, and Belgium, as ...
|
| Which Spaniard led an expedition which reached Tenochtitlan, the Aztec capital in 1519?
| The Spanish Conquest (1519-1521) : Mexico History History | See all articles tagged history The Spanish Conquest (1519-1521) Tweet April 21, 1519--the year Ce Acatl (One Reed) by Aztec reckoning-- marked the opening of a short but decisive chapter in Mexico's history. On that day a fleet of 11 Spanish galleons sailing along the eastern gulf coast dropped anchor just off the wind-swept beach on the island of San Juan de Ulúa. Under the command of the wily, daring Hernán Cortés, the vessels bore 550 Spanish soldiers and sailors, as well as 16 horses, the first of the species to tread the American continent. The party disembarked to set up camp on the dunes behind the beach. In a friendly reception from the native Totonac Indians, greetings and gifts were exchanged. Cognizant of the existence of a great inland Empire, Cortés promptly dispatched a message requesting an audience with Aztec ruler Moctezuma II . (The term "Aztec" will be used throughout, although some historians prefer the ...
|
* Loss: [MatryoshkaLoss
](https://sbert.net/docs/package_reference/sentence_transformer/losses.html#matryoshkaloss) with these parameters:
```json
{
"loss": "MultipleNegativesRankingLoss",
"matryoshka_dims": [
1024,
512,
256,
128,
64,
32
],
"matryoshka_weights": [
1,
1,
1,
1,
1,
1
],
"n_dims_per_step": -1
}
```
msmarco_10m
* Dataset: [msmarco_10m](https://huggingface.co./datasets/bclavie/msmarco-10m-triplets) at [8c5139a](https://huggingface.co./datasets/bclavie/msmarco-10m-triplets/tree/8c5139a245a5997992605792faa49ec12a6eb5f2)
* Size: 10,000,000 evaluation samples
* Columns: query
, positive
, and negative
* Approximate statistics based on the first 1000 samples:
| | query | positive | negative |
|:--------|:------------------------------------------------------------------------------------------------|:--------------------------------------------------------------------------------------------------|:-------------------------------------------------------------------------------------------------|
| type | string | string | string |
| details | - min: 10 characters
- mean: 33.98 characters
- max: 131 characters
| - min: 56 characters
- mean: 353.39 characters
- max: 1029 characters
| - min: 85 characters
- mean: 339.79 characters
- max: 983 characters
|
* Samples:
| query | positive | negative |
|:----------------------------------------------------------|:-------------------------------------------------------------------------------------------------------------------------------------------------------------------------------------------------------------------------------------------------------------------------------------------------------------------------------------------------------------------------------------------------------------------------------------------------------------------------------------------------------|:-------------------------------------------------------------------------------------------------------------------------------------------------------------------------------------------------------------------------------------------------------------------------------------------------------------------------------------------------------------------------------|
| what does a dental hygienist do
| During a dental appointment, a hygienist typically removes soft and hard deposits from a patient's teeth; examines the gums and teeth to discern the presence of disease or oral abnormality; and strips the teeth of calculus (tartar), stains and plaque. dental hygienist takes on a somewhat academic role as well; he or she educates dental patients on how to establish and maintain suitable oral hygiene, often with the aid of teeth models to give the patient a visual sense.
| Michelly in Tennessee said: Can anyone tell me how much you get paid hourly working as a dental hygienist. I make $36 dollars an hour and I just graduated from school. Should I be making more. Try to visit this link www.payscale.com/research/US/Job=Dental_Hygienist/Hourly_Rate so you will know how much dental hygienist usually get based on experience.
|
| average annual temperature by florida county
| Lake County Weather. The average temperature of Lake County is 70.90°F, which is about the same as the Florida average temperature of 71.80°F and is much higher than the national average temperature of 54.45°F. Historical Weather. Heating Cost Index, #29.
| average rn salary in fl the average annual salary for a registered nurse in the state of florida in 2011 was $ 64020 the average does fluctuate throughout the state with median rn salaries at their highest in metro areas
|
| what does amortization mean
| What is 'Amortization'. Amortization is the paying off of debt with a fixed repayment schedule in regular installments over a period of time for example with a mortgage or a car loan.
| First off, your EBIT is the same as your operating profit, but you can also calculate it by subtracting interest and tax from net income: $100,000 / ($10,000 + $25,000) = $65,000 To get EBITDA, you need to add back in depreciation and amortization:
|
* Loss: [MatryoshkaLoss
](https://sbert.net/docs/package_reference/sentence_transformer/losses.html#matryoshkaloss) with these parameters:
```json
{
"loss": "MultipleNegativesRankingLoss",
"matryoshka_dims": [
1024,
512,
256,
128,
64,
32
],
"matryoshka_weights": [
1,
1,
1,
1,
1,
1
],
"n_dims_per_step": -1
}
```
swim_ir
* Dataset: [swim_ir](https://huggingface.co./datasets/nthakur/swim-ir-monolingual) at [834c20f](https://huggingface.co./datasets/nthakur/swim-ir-monolingual/tree/834c20f0ceef6a68e029fb4447d17d20bb0288c3)
* Size: 501,538 evaluation samples
* Columns: query
and text
* Approximate statistics based on the first 1000 samples:
| | query | text |
|:--------|:-----------------------------------------------------------------------------------------------|:---------------------------------------------------------------------------------------------------|
| type | string | string |
| details | - min: 4 characters
- mean: 59.74 characters
- max: 165 characters
| - min: 206 characters
- mean: 522.53 characters
- max: 3079 characters
|
* Samples:
| query | text |
|:------------------------------------------------------------------------|:-----------------------------------------------------------------------------------------------------------------------------------------------------------------------------------------------------------------------------------------------------------------------------------------------------------------------------------------------------------------------------------------------------------------------------------------------------------------------------------------------------------------------------------------------------------------------------------------------------------------------------------------------------------|
| Where was he born?
| He was born in Brownsville, Edmonson County, Kentucky, March 28, 1890; attended the public schools, Western Kentucky State Teachers College at Bowling Green, and the law department of the University of Kentucky at Lexington; was admitted to the bar in 1915 and commenced practice in Brownsville, Ky.; county judge of Edmonson County, Ky., 1916-1918.
|
| What was Channon's National Service?
| Channon completed his National Service in the Royal Horse Guards (the Blues) from 1955 to 1956, serving in Cyprus during the 1956 Cyprus emergency. In London, he was a member of the set around Princess Margaret, and then attended Christ Church, Oxford from 1956. He was president of the Oxford University Conservative Association.
|
| What is the role of Immunoglobulin A in the immune system?
| Immunoglobulin heavy constant alpha 1 is a immunoglobulin gene with symbol "IGHA1". It encodes a constant (C) segment of Immunoglobulin A heavy chain. Immunoglobulin A is an antibody that plays a critical role in immune function in the mucous membranes. IgA shows the same typical structure of other antibody classes, with two heavy chains and two light chains, and four distinct domains: one variable region, and three variable regions. As a major class of immunoglobulin in body secretions, IgA plays a role in defending against infection, as well as preventing the access of foreign antigens to the immunologic system.
|
* Loss: [MatryoshkaLoss
](https://sbert.net/docs/package_reference/sentence_transformer/losses.html#matryoshkaloss) with these parameters:
```json
{
"loss": "MultipleNegativesRankingLoss",
"matryoshka_dims": [
1024,
512,
256,
128,
64,
32
],
"matryoshka_weights": [
1,
1,
1,
1,
1,
1
],
"n_dims_per_step": -1
}
```
pubmedqa
* Dataset: [pubmedqa](https://huggingface.co./datasets/sentence-transformers/pubmedqa) at [a1ef0b5](https://huggingface.co./datasets/sentence-transformers/pubmedqa/tree/a1ef0b513b16ed490e807ac11da40e436d3a54c3)
* Size: 1,660 evaluation samples
* Columns: anchor
, positive
, negative_1
, negative_2
, negative_3
, negative_4
, negative_5
, negative_6
, negative_7
, negative_8
, negative_9
, negative_10
, negative_11
, negative_12
, negative_13
, negative_14
, negative_15
, negative_16
, negative_17
, negative_18
, negative_19
, and negative_20
* Approximate statistics based on the first 1000 samples:
| | anchor | positive | negative_1 | negative_2 | negative_3 | negative_4 | negative_5 | negative_6 | negative_7 | negative_8 | negative_9 | negative_10 | negative_11 | negative_12 | negative_13 | negative_14 | negative_15 | negative_16 | negative_17 | negative_18 | negative_19 | negative_20 |
|:--------|:------------------------------------------------------------------------------------------------|:--------------------------------------------------------------------------------------------------|:-------------------------------------------------------------------------------------------------|:--------------------------------------------------------------------------------------------------|:--------------------------------------------------------------------------------------------------|:--------------------------------------------------------------------------------------------------|:--------------------------------------------------------------------------------------------------|:-------------------------------------------------------------------------------------------------|:--------------------------------------------------------------------------------------------------|:-------------------------------------------------------------------------------------------------|:-------------------------------------------------------------------------------------------------|:-------------------------------------------------------------------------------------------------|:--------------------------------------------------------------------------------------------------|:--------------------------------------------------------------------------------------------------|:--------------------------------------------------------------------------------------------------|:-------------------------------------------------------------------------------------------------|:--------------------------------------------------------------------------------------------------|:--------------------------------------------------------------------------------------------------|:--------------------------------------------------------------------------------------------------|:------------------------------------------------------------------------------------------------|:--------------------------------------------------------------------------------------------------|:--------------------------------------------------------------------------------------------------|
| type | string | string | string | string | string | string | string | string | string | string | string | string | string | string | string | string | string | string | string | string | string | string |
| details | - min: 38 characters
- mean: 88.92 characters
- max: 140 characters
| - min: 12 characters
- mean: 372.72 characters
- max: 1113 characters
| - min: 26 characters
- mean: 371.1 characters
- max: 1185 characters
| - min: 20 characters
- mean: 326.89 characters
- max: 1084 characters
| - min: 23 characters
- mean: 334.62 characters
- max: 1477 characters
| - min: 13 characters
- mean: 347.64 characters
- max: 1310 characters
| - min: 13 characters
- mean: 337.96 characters
- max: 1221 characters
| - min: 13 characters
- mean: 303.39 characters
- max: 953 characters
| - min: 14 characters
- mean: 328.25 characters
- max: 1168 characters
| - min: 16 characters
- mean: 318.59 characters
- max: 989 characters
| - min: 18 characters
- mean: 284.81 characters
- max: 853 characters
| - min: 13 characters
- mean: 333.89 characters
- max: 875 characters
| - min: 26 characters
- mean: 327.33 characters
- max: 1041 characters
| - min: 19 characters
- mean: 354.74 characters
- max: 1705 characters
| - min: 16 characters
- mean: 334.33 characters
- max: 1593 characters
| - min: 18 characters
- mean: 346.0 characters
- max: 1374 characters
| - min: 13 characters
- mean: 367.52 characters
- max: 1625 characters
| - min: 17 characters
- mean: 357.96 characters
- max: 1126 characters
| - min: 23 characters
- mean: 322.84 characters
- max: 1060 characters
| - min: 25 characters
- mean: 312.1 characters
- max: 805 characters
| - min: 22 characters
- mean: 362.97 characters
- max: 1002 characters
| - min: 22 characters
- mean: 328.62 characters
- max: 1310 characters
|
* Samples:
| anchor | positive | negative_1 | negative_2 | negative_3 | negative_4 | negative_5 | negative_6 | negative_7 | negative_8 | negative_9 | negative_10 | negative_11 | negative_12 | negative_13 | negative_14 | negative_15 | negative_16 | negative_17 | negative_18 | negative_19 | negative_20 |
|:--------------------------------------------------------------------------------------------------------|:------------------------------------------------------------------------------------------------------------------------------------------------------------------------------------------------------------------------------------------------------------------------------------------------------------------------------------------------------------------------------------------------------------------------------------------------------------------------------------------------------------------------------------------------------------------------------------------------------------------------------------------------------------------------------------------------------------------------------------------------------------------------------------------------------|:------------------------------------------------------------------------------------------------------------------------------------------------------------------------------------------------------------------------------------------------------------------------------------------------------------------------------------------------------------------------------------------------------------------------------------------------------------------------------------------------------------------------------------------------------------------------------------------------------------------------------------------------------------------------------------------------------------------------------------------------------------------------------------------|:---------------------------------------------------------------------------------------------------------------------------------------------------------------------------------------------------------------------------------------------------------------------------------------------------------------------------------------------------------------------------------------------------------------------------------------------------------------------------------------------------------------------------------------------------------------------------------------------------------------------------------------------------------------------------------------------------------------------------------------------------------------------------|:---------------------------------------------------------------------------------------------------------------------------------------------------------------------------------------------------------------------------------------------------------------------------------------------------------------------------------------------------------------------------------------------------------------------------------------------------------------------------------------------------------------------------------------------------------------------------------------------------------------------|:------------------------------------------------------------------------------------------------------------------------------------------|:-------------------------------------------------------------------------------------------------------------------------------------------------------------------------------------------------------------------------------------------------------------------------------------------------------------------------------------------------------------------------------------------------------------------------------------------------------------------------------------------------------------------------------|:-------------------------------------------------------------------------------------------------------------------------------------------------------------------------------------------------------------------------------------------------------------------------------------------------------------------------------------------------------------------------------------------------------------------------------------------------------------------------------------------------------------------------------------------------------------------------|:-------------------------------------------------------------------------------------------------------------------------------------------------------------------------------------------------------------------------------------------------------------------------------------------------------------------------------------------------------------------------------------------------------------------------------------------------------------------------------------------------------------------------------------------------------------------------------------------------------------------------------------------------------------------------------------------------|:------------------------------------------------------------------------------------------------------------------------------------------------------------------------------------------------------------------------------------------------------------------------------------------------------------------------------|:-----------------------------------------------------------------------------------------------------------------------------------------------------------------------------------------------------------------------------------------------------------------------------------------------------------------------------------------------------|:-------------------------------------------------------------------------------------------------------------------------------------------------------------------------------------------------------------------------------------------------------------------------------------------------------------------------------------------------------------------------------------------------------------------------------------------------------------------------------------------------------------------------------------------------------|:---------------------------------------------------------------------------------------------------------------------------------------------------------------------------------------------------------------------------------------------------------------------------------------------------------------------------------------------------------------------------------------------------------------------------------------------------------------------------------------------------------------------------------------------------------------------------------------------------------------------------------------------------------------------------------------------------------------------------------------------------------------------------------------------------------------------------------------------------------------------------------------------------------------------------------------------------------------------------|:--------------------------------------------------------------------------------------------------------------------------------------------------------------------------------------------------------------------------------------------------------------------------------------------------------------------------------------------------------------------------------------------------------------------------------------------------------------------------------------------------------------------------------------------------|:----------------------------------------------------------------------------------------------------------------------------------------------------------------------------------------------------------------------------------------------------------------------------------------------------------------------------------------------------------------------------------------------------------------------------------------|:----------------------------------------------------------------------------------------------------------------------------------------------------------------------------------------------------------------------------------|:----------------------------------------------------------------------------------------------------------------------------------------------------------------------------------------------------------------------------------------------------------------------------------------------------------------------------------------------------------------------------------|:---------------------------------------------------------------------------------------------------------------------------------------------------------------------------------------------------------------------------------------------------------------------------------------------------------------------------------------------------------------------------------------------------------------------------------------------------------------------------------------------------------------------------------------------------------------------------------------------------------------------------------------------------------------------------------------------------------------------------------------------------------------------------------------------------------------------------------------------------------------------------------------------------------------------------------------------------------------------------------------------------------------------------------------------------------|:------------------------------------------------------------------------------------------------------------------------------------------------------------------------------------------------------------------------------------------------------------------------------------------------------------------------------------------------------------------------------------------------------------------------------------------------------------------------------------------------------------------------------------------------------------------------------------------------------------------------------------------------------------------------------|:-------------------------------------------------------------------------------------------------------------------------------------------------------------------------------------------------------------------------------------------------------------------------------------------------------------------------------------------------------------------------------------------------------------------------------------------------------------------------------------------------------------------------------------------------------------------------------------------------------------------------|:-----------------------------------------------------------------------------------------------------------------------------------------------------------------------------------------------------------------------------------------------------------------------------------------------------------------------------------------------------------------------------------------------------------------------------------------------------------------------------------------------------------------------------------------------------------|:--------------------------------------------------------------------------------------------------------------------------------------------------------------------------------------------------------------------------------------------------------------------------------------------------------------------------------------------------------------------------------------------------------------------------------------------------------------------------------------------------------------------------------------------------------------------------------------------------------------------------------------------------|
| Chronic functional somatic symptoms: a single syndrome?
| Observational study, with a comparison control group.
| Nasal nitric oxide (NO) and olfactory function are decreased in patients with chronic inflammatory sinonasal disease, suggesting a link between these two parameters. The aim of the study was to investigate nasal NO levels in patients with olfactory dysfunction due to different causes.
| Fibromyalgia (FM) is a worldwide diffuse musculoskeletal chronic pain condition that affects up to 5% of the general population. Many symptoms associated with mitochondrial diseases are reported in patients with FM such as exercise intolerance, fatigue, myopathy and mitochondrial dysfunction. In this study, we report a mutation in cytochrome b gene of mitochondrial DNA (mtDNA) in a family with FM with inflammasome complex activation.
| Chronic fatigue syndrome (CFS) has an uncertain pathogenesis. Allergies have been suggested as one cause.
| Patient's self-reported symptoms on a structured case history questionnaire.
| Chronic multisymptom illness (reporting at least one symptom in at least two of the following symptom constructs: general fatigue; mood and cognition problems; and musculoskeletal discomfort) was assessed, differentiating by potential burn pit exposure, among deployers who completed 2004 and 2007 Millennium Cohort questionnaires.
| functional decline and death.
| Olfactory loss is a debilitating symptom of chronic rhinosinusitis (CRS). The pathophysiology of inflammatory olfactory dysfunction likely involves both conductive and sensorineural components. To study the interaction of CRS-associated inflammatory cytokines with the olfactory epithelium (OE), a transgenic mouse model was developed that allows temporally-controlled local gene expression. Interferon-gamma (IFN-γ) is a prototypical T helper 1 (Th1) cytokine linked to nonpolypoid CRS (CRSsNP), as well as sinonasal viral and bacterial infections. In this study, the effects of chronic IFN-γ expression on olfactory histology and function were investigated.
| Monosomy 1p36 syndrome is the most commonly observed subtelomeric deletion syndrome. Patients with this syndrome typically have common clinical features, such as intellectual disability, epilepsy, and characteristic craniofacial features.
| Migraine is frequently accompanied by symptoms consistent with functional gastrointestinal disorders (FGIDs). This study evaluated the prevalence of functional gastrointestinal symptoms and assessed the symptoms' relationship with the concomitant functional symptoms of anxiety, depression, and headache-related disability.
| The 2003 Canadian Consensus Criteria for chronic fatigue syndrome (CFS) are often assumed to suggest low-grade systemic inflammation, but have never been formally validated. This study explored the content validity of the Criteria in a sample of adolescents with CFS selected according to a wide case definition.
| Irritable pouch syndrome (IPS) is a functional disorder in patients with ileal pouch-anal anastomosis (IPAA), which presents with symptoms in the absence of structural abnormalities of the pouch. Thus, it resembles other functional disorders, such as irritable bowel syndrome characterized by visceral hypersensitivity in the presence of normal rectal biomechanics. The aim was to assess pouch biomechanics and perception of balloon distension in different groups of subjects with IPAA and to correlate the findings with clinical features.
| Fibromyalgia (FM) and chronic fatigue syndrome (CFS) frequently overlap clinically and have been considered variants of one common disorder. We have recently shown that CFS is associated with a short corrected electrocardiographic QT interval (QTc). In the present study, we evaluated whether FM and CFS can be distinguished by QTc.
| Single case with clinical follow-up over 2 years.
| Fibromyalgia syndrome (FMS) is a disease of unknown pathogenesis characterized by chronic musculoskeletal pain. FMS has been also associated with altered endocrinological responses, but findings are inconsistent.
| Primary Sjögren's syndrome (pSS) is a systemic rheumatic disease in which gastrointestinal (GI) symptoms are common. Faecal calprotectin (FC) is a non-invasive biomarker that has been suggested to discriminate organic intestinal disease from functional disorders. The purpose of this study was to explore the usefulness of FC testing in patients with pSS.
| An inactive lifestyle has been associated with functional somatic symptoms (FSS), but findings are contradictory. Moreover, mediating factors in this relationship are unclear. We examined whether low physical activity was related to FSS in adolescents, and whether this association was mediated by low physical fitness.
| Nephrotic syndrome is a common kidney disease in both children and adults that is characterized by dramatic structural changes in the actin-rich foot processes of glomerular podocytes. Although glucocorticoids are the primary treatment for nephrotic syndrome, neither the target cell nor mechanism of action of glucocorticoids in nephrotic syndrome is known. For the last 30 years glucocorticoids have been presumed to act by reducing the release of soluble mediators of disease by circulating lymphocytes. In contrast, we hypothesized that glucocorticoids exert their beneficial effects in nephrotic syndrome by direct action on podocytes.
| Chronic sclerosing sialadenitis is a fibroinflammatory disease of the salivary glands, characteristically of the submandibular gland. One prior Asian study proposed that chronic sclerosing sialadenitis is a part of the spectrum of IgG4-associated disease. This association has not been confirmed in Western populations. We therefore, investigated the relationship between IgG4 and chronic sclerosing sialadenitis, and compared the histomorphologic features of this condition with those of chronic sialadenitis-not otherwise specified, Sjögren syndrome, and lymphoepithelial sialadenitis.
| Presence of GI symptoms.
| Disrupted-in schizophrenia 1 (DISC1), identified in a pedigree with a familial psychosis with the chromosome translocation (1:11), is a putative susceptibility gene for psychoses such as schizophrenia and major depressive disorder (MDD). Patients with chronic fatigue syndrome (CFS) report having continuous severe fatigue and many overlapping symptoms with MDD; however, the mechanism and effective treatment of CFS are still unclear. We focused on the overlapping symptoms between CFS and MDD and performed an association study of the functional single-nucleotide polymorphism (SNP) in the DISC1 gene with CFS.
|
| Does sonographic needle guidance affect the clinical outcome of intraarticular injections?
| In total, 148 painful joints were randomized to IA triamcinolone acetonide injection by conventional palpation-guided anatomic injection or sonographic image-guided injection enhanced with a one-handed control syringe (the reciprocating device). A one-needle, 2-syringe technique was used, where the first syringe was used to introduce the needle, aspirate any effusion, and anesthetize and dilate the IA space with lidocaine. After IA placement and synovial space dilation were confirmed, a syringe exchange was performed, and corticosteroid was injected with the second syringe through the indwelling IA needle. Baseline pain, procedural pain, pain at outcome (2 weeks), and changes in pain scores were measured with a 0-10 cm visual analog pain scale (VAS).
| We used a blinded, longitudinal observational design of effectiveness in an effort to determine the accuracy of intra-articular injections and the effect of that accuracy on pain and functional outcomes in patients with various shoulder pathologies.
| Data from standardized procedure notes and postprocedure chest radiographs were extracted and individually reviewed to verify the presence of pneumothorax or misplacement, and any intervention performed for either complication. The overall success rate of ultrasound-guided right internal jugular vein central venous catheter placement was 96.9% with an average of 1.3 attempts. There was only one pneumothorax (0.1% [95% CI, 0-0.4%]), and the rate of catheter misplacement requiring repositioning or replacement was 1.0% (95% CI, 0.6-1.7%). There were no arterial placements found on chest radiographs. Multivariate regression analysis showed no correlation between high-risk patient characteristics and composite complication rate.
| Real-time ultrasound-guided techniques allow for improved cannulation of the internal jugular vein and femoral vein for hemodialysis; however, these techniques require extra sterilization procedures, specialized probes, or needle guides. A simpler ultrasound vessel localization method was performed to investigate whether this alternative approach would aid in the cannulation of the femoral vein for patients in whom temporary angioaccess was required for hemodialysis.
| To determine whether duration or venue of intravenous antibiotic administration affect lung function.
| Needle electromyography (NEE) would be more valuable if it could predict outcomes after lumbar epidural steroid injections (LESIs) in lumbosacral radiculopathy (LSR).
| Among patients with Wilkes stage III and IV disease undergoing arthroscopic lysis and lavage, does the use of an intra-articular injection of sodium hyaluronate (SH), when compared with Ringer lavage, result in better postoperative pain control and temporomandibular joint (TMJ) function?
| Intraoperative MR imaging and sonography are used for navigation during neurosurgical procedures. The purpose of this experimental study was to evaluate the potential of high-resolution sonography using superparamagnetic iron oxide (SPIO) particles as a contrast medium to delineate brain tumors and to relate these findings with those of MR imaging.
| Academic general internal medicine practice.
| To evaluate how guidance on water-intake impacts the degree of nocturia.
| Intra-articular knee injections are commonly performed in clinical practice for treating various knee joint disorders such as osteoarthritis and rheumatoid arthritis. When selecting the portal for injection, not only intra-articular needle accuracy but also procedural pain should be taken into consideration. The purpose of this study was to determine whether injection through anterolateral portal provokes less pain and provides better pain relief compared to superolateral portal.
| Ultrasound guidance reduces the required local anesthetic volume for successful peripheral nerve blockade, but it is unclear whether this impacts postoperative analgesia. This prospective, randomized, observer-blinded study tested the hypothesis that a low-volume ultrasound-guided ankle block would provide similar analgesia after foot surgery compared with a conventional-volume surface landmark technique.
| The purpose of this study was to evaluate how intravascular ultrasound-determined thickness and reflectivity of the inner echogenic layer of coronary artery plaque is affected by changes in collagen, elastin, proteoglycan, calcium and lipid content in the intima and media.
| The anesthetic requirement is decreased in animals with head injury, but there are no data regarding the effect of intracranial tumor on the potency for intravenous anesthetics. The authors compared the quantal dose-response curves for propofol in patients having large (> or = 30 mm, mass effect) brain tumor with those having smaller (< 30 mm) lesions and with control patients undergoing noncranial surgery.
| To develop digital multimedia instruction on intraoral suturing.
| To evaluate a long term efficiency of a deep sclerectomy with T-Flux implant on intraocular pressure
| In bilateral cochlear implant users, electrodes mapped to the same frequency range in each ear may stimulate different places in each cochlea due to an insertion depth difference of electrode arrays. This interaural place of stimulation mismatch can lead to problems with auditory image fusion and sensitivity to binaural cues, which may explain the large localization errors seen in many patients. Previous work has shown that interaural place of stimulation mismatch can lead to off-centered auditory images being perceived even though interaural time and level differences (ITD and ILD, respectively) were zero. Large interaural mismatches reduced the ability to use ITDs for auditory image lateralization. In contrast, lateralization with ILDs was still possible but the mapping of ILDs to spatial locations was distorted. This study extends the previous work by systematically investigating the effect of interaural place of stimulation mismatch on ITD and ILD sensitivity directly and examining...
| Mathematical modeling and use of real world clinical inputs.
| The use of ultrasonography in regional anesthetic blocks has rapidly evolved over the past few years. It has been speculated that ultrasound guidance might increase success rates and reduce complications. The aim of our study is to compare the success rate and quality of interscalene brachial plexus blocks performed either with direct ultrasound visualization or with the aid of nerve stimulation to guide needle placement.
| Intra-operative nerve monitoring (IONM) of the recurrent laryngeal nerve (RLN) during thyroid and parathyroid surgery is thought to aid in identification and dissection of the RLN. While utilization of IONM is increasing, one area of variability in its application is the assessment of adequate endotracheal tube electrode placement for IONM during the case. The main objective of this study is to assess the overall success of utilizing respiratory variation to confirm proper endotracheal tube placement for RLN monitoring.
| Time-dependent development of intracochlear impedances was investigated in 4 different groups of adult patients up to 4 years after implantation. Additionally, during rehabilitation period just after first fitting, impedances before and after stimulation were measured as to investigate the influence of electrical stimulation on the impedances. Results from standard Nucleus 24 Contour (control), standard Nucleus 24 Contour with intraoperative application of steroids, iridium-coated Nucleus 24 Contour, and iridium-coated Nucleus 24 Contour with intraoperative application of steroids were compared.
|
| Does type 1 diabetes mellitus affect Achilles tendon response to a 10 km run?
| Participants were 7 individuals with T1DM and 10 controls. All regularly ran distances greater than 5 km and VISA-A scores indicated good tendon function (T1DM = 94 ± 11, control = 94 ± 10). There were no diabetic complications and HbA1c was 8.7 ± 2.6 mmol/mol for T1DM and 5.3 ± 0.4 mmol/mol for control groups. Baseline tendon structure was similar in T1DM and control groups - UTC echo-types (I-IV) and anterior-posterior thickness were all p > 0.05. No response to load was seen in either T1DM or control group over the 4-days post exercise.
| The inability to produce insulin endogenously precipitates the clinical symptoms of type 1 diabetes mellitus. However, the dynamic trajectory of beta cell destruction following onset remains unclear. Using model-based inference, the severity of beta cell destruction at onset decreases with age where, on average, a 40% reduction in beta cell mass was sufficient to precipitate clinical symptoms at 20 years of age. While plasma C-peptide provides a surrogate measure of endogenous insulin production post-onset, it is unclear as to whether plasma C-peptide represents changes in beta cell mass or beta cell function. The objective of this paper was to determine the relationship between beta cell mass and endogenous insulin production post-onset.
| Seven healthy individuals with type 1 diabetes were tested on two separate occasions, during which either a 30-min MOD or IHE protocol was performed. MOD consisted of continuous exercise at 40% Vo(2peak), while the IHE protocol involved a combination of continuous exercise at 40% Vo(2peak) interspersed with 4-s sprints performed every 2 min to simulate the activity patterns of team sports.
| It is unclear how genetic type 1 diabetes mellitus (DM) influences infarct size when blood glucose is tightly controlled. The aim of this study was to determine the effect of genetic type 1 DM, as occurs in BB rats, on infarct size after transient unilateral middle cerebral artery occlusion (MCAO) in male and female rats. In addition, studies suggest that male type 1 DM rats have a higher incidence of end-organ complications than do females. A second aim of this study was to determine the effect of chronic 17beta-estradiol (E(2)) administration on infarct size in male BB rats.
| Studies on bone mineral characteristics in children with type 1 diabetes mellitus (T1DM) have generated conflicting results.
| It has recently been reported that the risk of developing nephropathy in patients with insulin dependent (type 1) diabetes mellitus is strongly associated with synergism between poor glycaemic control and carriage of the hypertension associated angiotensin II (type 1) receptor C1166 allele. The same report also revealed an increase in risk of nephropathy in diabetic patients carrying a specific angiotensin II (type 1) receptor haplotype, i.e. C1166/140-bp microsatellite allele (major allele).
| The angiotensin II (type 1) (AT1) receptor mediates many biological effects of the renin-angiotensin system (RAS), leading to remodelling of cardiac tissue. The present study was designed to analyse changes in the function and expression of the AT1 receptor as principal effector of the RAS in myocardium from type 2 diabetic patients compared with non-diabetic myocardium as control. In addition, we determined the effect of treatment with ACE inhibitors or AT1 receptor blockers on expression levels of the receptor in diabetic patients.
| Type 2 diabetes mellitus increases the risk of atherosclerotic cardiovascular disease. Antioxidative properties of high density lipoprotein (HDL) are important for atheroprotection. This study investigated whether the antioxidative functionality of HDL is altered in type 2 diabetes mellitus and aimed to identify potential determinants of this parameter.
| Life-long insulin is the standard treatment for type 1 diabetes mellitus (T1DM). The role of traditional Chinese medicine (TCM) in T1DM is still not clear. The aim of this study is to explore the prescription pattern of TCM and its impact on the risk of diabetic ketoacidosis (DKA) in patients with T1DM.
| In type 1 diabetes, lung diffusing capacity for carbon monoxide (DL(CO)) may be impaired, and insulin has been shown to be beneficial in cases in which near-normal metabolic control is achieved. An influence of insulin, per se, on the alveolar-capillary membrane conductance is unexplored. We aimed at testing this possibility.
| aIMT, but not cIMT, was significantly greater in the children with type 1 diabetes mellitus than in control subjects (P < .001). In children with type 1 diabetes mellitus, aIMT correlated with glycosylated hemoglobin (r = 0.31, P = .01) and was independently associated with age (beta = 0.38, P = .001) and low-density lipoprotein cholesterol level (beta = 0.38, P = .001). Vascular function (GTN) was worse in children with type 1 diabetes mellitus who had an aIMT >95th percentile, as defined with the control subjects.
| Type 1 diabetes mellitus (T1DM) is caused by specific destruction of the pancreatic beta cells in the islets of Langerhans. Increased sensitivity to cytokines, in particular to interleukin-1beta (IL-1beta) seems to be an acquired trait during beta-cell maturation. In response to cytokines both protective and deleterious mechanisms are induced in beta cells, and when the deleterious prevail, T1DM develops. The aims of this study were to identify perturbation in protein patterns (PiPP) associated with beta-cell maturation, and compare these changes to previous analyses of IL-1beta exposed rat islets. For this purpose, proteome analyses were carried out using a cell-line, which matures from a glucagon-producing pre-beta-cell phenotype (NHI-glu) to an insulin-producing beta-cell phenotype (NHI-ins). We have previously shown that this maturation is accompanied by acquired sensitivity to the toxic effects of IL-1beta.
| Type 1 diabetes mellitus is a T-cell-mediated autoimmune disease that leads to a major loss of insulin-secreting beta cells. The further decline of beta-cell function after clinical onset might be prevented by treatment with CD3 monoclonal antibodies, as suggested by the results of a phase 1 study. To provide proof of this therapeutic principle at the metabolic level, we initiated a phase 2 placebo-controlled trial with a humanized antibody, an aglycosylated human IgG1 antibody directed against CD3 (ChAglyCD3).
| Type 2 diabetes mellitus (T2DM) affects approximately 10% of Americans, while 79 million Americans are estimated to have glucose intolerance or prediabetes (pre-DM). The present study was designed to determine whether obese patients with pre-DM or T2DM would lose weight as effectively as obese normoglycemic patients, in a medically supervised high-protein, low-calorie-weight management program.
| We have previously shown that long-term type 1 diabetes affects the structural organization, contractile apparatus and extracellular matrix (ECM) of the myometrium during early pregnancy in mice.
| In Achilles injured participants, there was a significant difference between soleus and lateral gastrocnemius offset times during running compared to the asymptomatic controls (p<0.05). There were no significant differences in triceps surae muscle activity between the footwear only and footwear and orthoses condition in the Achilles injured participants.
| Nine adolescents with type 1 diabetes mellitus (five males, four females, aged 16 +/- 1.8 yr, diabetes duration 8.2 +/- 4.1 yr, hemoglobin A1c 7.8 +/- 0.8%, mean +/- SD) were subjected on two different occasions to a rest or 45 min of exercise at 95% of their lactate threshold. Insulin was administered iv at a rate based on their usual insulin dose, with similar plasma insulin levels for both studies (82.1 +/- 19.0, exercise vs. 82.7 +/- 16.4 pmol/liter, rest). Glucose was infused to maintain euglycemia for 18 h.
| Type 1 diabetes mellitus (T1DM) is influenced by genetic as well as environmental factors. Its incidence has risen considerably since the 1950s.
| Insulin resistance has been associated with hypertension and with renal complications in patients with type 1 diabetes mellitus. Causal relationships have not been fully explained.
| Diabetes mellitus (DM) is a common metabolic disease among the middle-aged and older population, which leads to an increase of stroke incidence and poor stroke recovery. The present study was designed to investigate the impact of DM on brain damage and on ischemic brain repair after stroke in aging animals.
| Type 1 diabetes (T1D) is an autoimmune disease with multiple susceptibility genes. The aim of this study was to determine whether combining IDDM1/HLA and IDDM2/ insulin( INS) 5' variable number of tandem repeat locus (VNTR) genotypes improves T1D risk assessment.
|
* Loss: [MatryoshkaLoss
](https://sbert.net/docs/package_reference/sentence_transformer/losses.html#matryoshkaloss) with these parameters:
```json
{
"loss": "MultipleNegativesRankingLoss",
"matryoshka_dims": [
1024,
512,
256,
128,
64,
32
],
"matryoshka_weights": [
1,
1,
1,
1,
1,
1
],
"n_dims_per_step": -1
}
```
miracl
* Dataset: [miracl](https://huggingface.co./datasets/sentence-transformers/miracl) at [07e2b62](https://huggingface.co./datasets/sentence-transformers/miracl/tree/07e2b629250bf4185f4c87f640fac15949b8aa73)
* Size: 789,900 evaluation samples
* Columns: anchor
, positive
, and negative
* Approximate statistics based on the first 1000 samples:
| | anchor | positive | negative |
|:--------|:-----------------------------------------------------------------------------------------------|:--------------------------------------------------------------------------------------------------|:--------------------------------------------------------------------------------------------------|
| type | string | string | string |
| details | - min: 11 characters
- mean: 38.08 characters
- max: 86 characters
| - min: 93 characters
- mean: 746.58 characters
- max: 3851 characters
| - min: 18 characters
- mean: 648.12 characters
- max: 2554 characters
|
* Samples:
| anchor | positive | negative |
|:------------------------------------------------------|:------------------------------------------------------------------------------------------------------------------------------------------------------------------------------------------------------------------------------------------------------------------------------------------------------------------------------------------------------------------------------------------------------------------------------------------------------------------------------------------------------------------------------------------------------------------------------------------------------------------------------------------------------------------------------------------------------------------------------------------------------------------------------------------------------------------------------------------------------------------------------------------------------------------------------------------------------------------------------------------------------------------------------------------------------------|:--------------------------------------------------------------------------------------------------------------------------------------------------------------------------------------------------------------------------------------------------------------------------------------------------------------------------------------------------------------------------------------------------------------------------------------------------------------------------------------------------------------------------------------------------------------------------------------------------------------------------------------------------------------------------------------------------------------------------------------------------------------------------------------------------------------------------------------------------------------------------------------------------------------------------------|
| What was Paul Heyman's first production?
| Paul Heyman
On May 23, 2005, Heyman returned in a segment with Vince McMahon and Eric Bischoff announcing ECW One Night Stand, with Heyman in charge. On the May 22 episode of "Raw", Heyman appeared as ECW Representative promoting "One Night Stand". On May 25, 2006 it was announced that ECW would relaunch, as a third WWE brand. Heyman was in charge of the new brand on-camera but had minimal creative input off-camera as well. On the May 29 episode of "Raw", during a face-off with Mick Foley, Heyman announced that he was granted a draft pick from both Raw and SmackDown! by Vince McMahon. His Raw draft pick was former ECW wrestler (and Money in the Bank contract holder) Rob Van Dam, and his SmackDown! draft pick was Kurt Angle. Heyman predicted that Van Dam would defeat John Cena at "One Night Stand" for the WWE Championship and then declare himself the new ECW World Heavyweight Champion.
| Jay Wolpert
Wolpert left Goodson-Todman to form his own production company, and his first game show was the 1979 series "Whew!" for CBS. Wolpert produced the series with Burt Sugarman for most of its run. "Whew!" was canceled in 1980 and Wolpert did not return to television with a series until January 1983, despite shooting several pilots in the interim. On January 3, 1983, Wolpert's "Hit Man" debuted on NBC with Peter Tomarken as its host. "Hit Man" lasted thirteen weeks on the air.
|
| When was Locke born?
| Adria Locke Langley
Locke was born in Iowa, 1899, as the youngest of three children. When she was young her family moved to Stanton, Nebraska and that is where she grew up. Her father William Locke, was president of the Omaha livestock market and a Quaker. He had certain ideas of what a woman should be. Because of this Adria's grandfather, Thomas Glendenning, took over in teaching her what would later be a great social consciousness.
| Keith Locke
Locke was born and grew up in Christchurch, to Jack and Elsie Locke, prominent lifelong political activists for a wide variety of causes. Their four children were brought up in this environment and followed their parents into a life of activism, (as well as Keith, his sister Maire Leadbeater is a well-known activist and former city councillor for Auckland City Council). His father Jack was under surveillance during the 1951 New Zealand waterfront dispute.
Former Prime Minister Robert Muldoon is said to have described the Lockes as the most "notorious Communist family in New Zealand". The Lockes lived in the Avon Loop area of the Christchurch Central City and were very active in the community notably organising Avon River clean-ups and native tree planting and arguing against development of the area, and in favour of retaining the character of the area.
|
| How do plants get blight?
| Bacterial blight of soybean
Bacterial blight of soybeans can enter leaves through wounds or natural openings such as stomata. After gaining entrance to the host leaves, "Pseudomonas syringae pv. glycinea" multiplies in the leaf intercellular fluid. The pathogen must then overcome the plants defenses. "Pseudomonas syringae pv. glycinea" accomplishes this by using the type three secretion system to inject a variety of pathogenicity effector proteins (Hrp proteins) into the plant cell cytoplasm. These proteins act by interfering with effector-triggered immunity and producing phytohormones/toxins that suppress plant defenses. The expression of these virulence factors depends on the environmental conditions at the time of infection (see "environment section). Furthermore, expression of virulence factors will only take place when a sufficiently large population of bacteria is present, which is determined through quorum sensing. When successful, the common symptoms of bacterial blight will be...
| Gummy stem blight
Gummy Stem Blight is a cucurbit-rot disease caused by the fungal plant pathogen "Didymella bryoniae" (anamorph "Phoma cucurbitacearum").
Gummy Stem Blight can affect a host at any stage of growth in its development and affects all parts of the host including leaves, stems and fruits. Symptoms generally consist of circular dark tan lesions that blight the leaf, water soaked leaves, stem cankers, and gummy brown ooze that exudes from cankers, giving it the name Gummy Stem Blight. Gummy Stem Blight reduces yields of edible cucurbits by devastating the vines and leaves and rotting the fruits.
|
* Loss: [MatryoshkaLoss
](https://sbert.net/docs/package_reference/sentence_transformer/losses.html#matryoshkaloss) with these parameters:
```json
{
"loss": "MultipleNegativesRankingLoss",
"matryoshka_dims": [
1024,
512,
256,
128,
64,
32
],
"matryoshka_weights": [
1,
1,
1,
1,
1,
1
],
"n_dims_per_step": -1
}
```
mldr
* Dataset: [mldr](https://huggingface.co./datasets/sentence-transformers/mldr) at [40ad767](https://huggingface.co./datasets/sentence-transformers/mldr/tree/40ad7672817ebee49e00dd25aed00e1c401881d6)
* Size: 200,000 evaluation samples
* Columns: anchor
, positive
, and negative
* Approximate statistics based on the first 1000 samples:
| | anchor | positive | negative |
|:--------|:------------------------------------------------------------------------------------------------|:--------------------------------------------------------------------------------------------------------|:--------------------------------------------------------------------------------------------------------|
| type | string | string | string |
| details | - min: 16 characters
- mean: 66.52 characters
- max: 527 characters
| - min: 3223 characters
- mean: 20386.58 characters
- max: 131268 characters
| - min: 3611 characters
- mean: 15772.93 characters
- max: 145135 characters
|
* Samples:
| anchor | positive | negative |
|:-------------------------------------------------------------------------------------------------------------------------------|:---------------------------------------------------------------------------------------------------------------------------------------------------------------------------------------------------------------------------------------------------------------------------------------------------------------------------------------------------------------------------------------------------------------------------------------------------------------------------------------------------------------------------------------------------------------------------------------------------------------------------------------------------------------------------------------------------------------------------------------------------------------------------------------------------------------------------------------------------------------------------------------------------------------------------------------------------------------------------------------------------------------------------------------------------------------------------------------------------------|:---------------------------------------------------------------------------------------------------------------------------------------------------------------------------------------------------------------------------------------------------------------------------------------------------------------------------------------------------------------------------------------------------------------------------------------------------------------------------------------------------------------------------------------------------------------------------------------------------------------------------------------------------------------------------------------------------------------------------------------------------------------------------------------------------------------------------------------------------------------------------------------------------------------------------------------------------------------------------------------------------------------------------------------------------------------------|
| What is an Aboriginal reserve and how was it created?
| An Aboriginal reserve, also called simply reserve, was a government-sanctioned settlement for Aboriginal Australians, created under various state and federal legislation. Along with missions and other institutions, they were used from the 19th century to the 1960s to keep Aboriginal people separate from the white Australian population, for various reasons perceived by the government of the day. The Aboriginal reserve laws gave governments much power over all aspects of Aboriginal people’s lives.
Protectors of Aborigines and (later) Aboriginal Protection Boards were appointed to look after the interests of the Aboriginal people.
History
Aboriginal reserves were used from the nineteenth century to keep Aboriginal people separate from the white Australian population, often ostensibly for their protection.
Protectors of Aborigines had been appointed from as early as 1836 in South Australia (with Matthew Moorhouse as the first permanent appointment as Chief Protector in 1839), with the G...
| The Nature Reserve () is a nature reserve located in the municipalities of Sorsele and Storuman in Västerbotten County of Swedish Lapland. It is the largest natural reserve in Sweden and one of the largest protected areas in Europe, totaling 562,772 ha (approx. 5,628 km2).
Most of the reserve is made up of several Scandinavian Mountains, the main ones being Artfjället, Norra Storfjället, Ammarfjället and Björkfjället. Most of the landscapes of the Swedish mountains are represented. This ranges from the pronounced alpine character of Norra Storfjället, which includes the highlight of the reserve, the Norra Sytertoppen (1,768 m), to the plateau and plains near the base of the mountains. The differences in elevation highlight the diversity of rocks in the mountains. Among the mountains are the valleys and waterways of the Ume River drainage basin. This includes a portion of the Vindel River, after which the reserve is named. Towards the east, the elevation decreases and the mountains gi...
|
| What is the English translation of the song "Bosanac"?
| This is a list of Bosnian patriotic songs.
{| class="wikitable sortable"
|+
!Title!!English translation!!Lyricist!!Composer!!Arranger!!Year!!scope="col" class="unsortable"|Description
|-
|"Bosanac"||'Bosnian Man'||||||||style="text-align:center"|1984||song sung by Bosnian singer Lepa Brena and her band Slatki Greh on their 1984 album Bato, Bato
|-
|"Bosanac"||'Bosnian [Man]'||||||||style="text-align:center"|1991||folk song sung by singer Enes Begović
|-
|"Bosanac"||'Bosnian [Man]'||Mirko Šenkovski||Mirko Šenkovski||Mirko Šenkovski||style="text-align:center"|2012||turbo-folk song sung by singer Elvira Rahić and DJ Deny
|-
|"Bosna"||'Bosnia'||||||||||song about the Bosnia and Herzegovina national basketball team, also used when the KK Bosna team plays games
|-
|"Bosna će vječno živjeti u nama"||'Bosnia Will Live in Us Forever'||Besim Spahić||Besim Spahić||Besim Spahić||||song sung by Bosnian musician and scientist
|-
|"Bosna ima jedna samo"||'Bosnia [There] Is One [And] Only'||Enver Ša...
| "Quiéreme mucho" is a criolla-bolero composed in 1911 by Gonzalo Roig with lyrics by Ramón Gollury and Agustín Rodríguez. The song was inspired by Roig's wife, Blanca Becerra, and premiered in Havana in 1911 without much success. In 1917, it was included in the sainete El servicio militar obligatorio and performed by Becerra and Rafael Llorens to critical acclaim. Roig published and sold the rights to the song in 1921, and the first recording was made in the United States by singer Tito Schipa in 1923. The English version, "Yours", was published in 1931 in the United States. It featured lyrics in English written by Albert Gamse and Jack Sherr. Both versions have been extensively recorded and arranged by different musicians, becoming Latin music standards.
Composition
"Quiéreme mucho" was composed by Gonzalo Roig at 21 years of age in 1911, before he had finished his music studies. He wrote the melody and played it on his piano, without making any further arrangements. Roig had been co...
|
| Who were the representatives that declined to run for reelection to the Vermont House of Representatives in 2016?
| Selene Colburn is an American politician currently serving in the Vermont House of Representatives from the Chittenden-6-4 district since 2017 as a member of the Vermont Progressive Party. Prior to her tenure in the State House she served on the city council in Burlington, Vermont. She is the first female chair of the House Progressive Caucus.
Colburn was born in Burlington, and educated at Burlington High School, Bennington College, and Simmons University. She became active in politics in her youth when she joined anti-war demonstrations.
Colburn was first elected to office with her election to the Burlington city council in the 2014 election and she won reelection in the 2015 and 2017 elections. She was elected to the state house alongside Brian Cina in the 2016 election with the nominations of the Progressive and Democratic parties and was reelected in the 2018 and 2020 elections. She was selected to serve as assistant chair of the Vermont Progressive Party's caucus in the state h...
| The 2016 United States Senate election in Florida was held November 8, 2016 to elect a member of the United States Senate to represent the State of Florida, concurrently with the 2016 U.S. presidential election, as well as other elections to the United States Senate in other states and elections to the United States House of Representatives and various state and local elections. The primary elections for both the Republicans and Democrats took place on August 30, 2016.
Incumbent Republican Senator Marco Rubio ran for another term but faced well-funded Republican primary opposition after initially announcing he would not seek re-election to his Senate seat. He had openly considered whether to seek re-election or run for president in 2016. He stated in April 2014 that he would not run for both the Senate and president in 2016, as Florida law prohibits a candidate from simultaneously appearing twice on a ballot, but did not rule out running for either office.
However, in April 2015, Ru...
|
* Loss: [MatryoshkaLoss
](https://sbert.net/docs/package_reference/sentence_transformer/losses.html#matryoshkaloss) with these parameters:
```json
{
"loss": "MultipleNegativesRankingLoss",
"matryoshka_dims": [
1024,
512,
256,
128,
64,
32
],
"matryoshka_weights": [
1,
1,
1,
1,
1,
1
],
"n_dims_per_step": -1
}
```
mr_tydi
* Dataset: [mr_tydi](https://huggingface.co./datasets/sentence-transformers/mr-tydi) at [abbdf55](https://huggingface.co./datasets/sentence-transformers/mr-tydi/tree/abbdf55c630352da943f779610c3ce6268118351)
* Size: 354,700 evaluation samples
* Columns: anchor
, positive
, and negative
* Approximate statistics based on the first 1000 samples:
| | anchor | positive | negative |
|:--------|:-----------------------------------------------------------------------------------------------|:--------------------------------------------------------------------------------------------------|:--------------------------------------------------------------------------------------------------|
| type | string | string | string |
| details | - min: 13 characters
- mean: 38.6 characters
- max: 101 characters
| - min: 71 characters
- mean: 667.4 characters
- max: 21297 characters
| - min: 10 characters
- mean: 619.16 characters
- max: 3134 characters
|
* Samples:
| anchor | positive | negative |
|:-----------------------------------------------------------------|:--------------------------------------------------------------------------------------------------------------------------------------------------------------------------------------------------------------------------------------------------------------------------------------------------------------------------------------------------------------------------------------------------------------------------------------------------------------------------------------------------------------------------------------------------------------------------------------------------------------------------------------------------------------------------------------------------------------------------------------------------------------------------------------------------------------------------|:---------------------------------------------------------------------------------------------------------------------------------------------------------------------------------------------------------------------------------------------------------------------------------------------------------------------------------------------------------------------------------------------------------------------------------------------------------------------------------------------------------------------------------------------------------------------------------------------------------------------------------------------------------------------------------------------------------------------------------------------------------------------------------------------------------------------------------------------------------------------------------------------------------------------------------------------------------------------------------------------------------------------------------------------------------------|
| What is the largest city in Sardinia?
| Sardinia
Sardinia is politically a region of Italy, whose official name is Regione Autonoma della Sardegna / Regione Autònoma de Sardigna (Autonomous Region of Sardinia),[3] and enjoys some degree of domestic autonomy granted by a specific Statute.[4] It is divided into four provinces and a metropolitan city, with Cagliari being the region's capital and its largest city as well. Sardinia's indigenous language and the other minority languages (Sassarese, Corsican Gallurese, Algherese Catalan and Ligurian Tabarchino) spoken on the island are recognized by the regional law and enjoy "equal dignity" with Italian.[5]
| History of Cagliari
Caralis (or Karales) was the capital of the Roman province of Sardinia and Corsica and was elevated to the rank of "Municipium", a result of the civil war between Julius Caesar and Pompey when Caesar himself granted this status in gratitude to the city for its fidelity during the bloody war. All Caralitani obtained Roman citizenship and were enrolled in the tribe Quirina. The territory of the city included the campidano plain, likely becoming Sanluri.
With about 20,000 inhabitants Caralis was the largest and most populous city of the island and the most important of the western Mediterranean basin of the Republic, and later of the Roman Empire. The city was equipped with important road links to the main towns of the island such as Sulki, with the coastal road and with that running through the valley of the Cixerri, Olbia and Tibula along the east coast, and Turris and Tibula along the road modeled on the current "Carlo Felice", and finally a road through the centre ...
|
| What activism did Katherine Schmidt participate in?
| Katherine Schmidt
Katherine Schmidt (February 6, 1899 – April 18, 1978) was an American artist and art activist. Early in her career the figure studies, landscapes, and still lifes she painted drew praise for their "purity and clarity of color," "sound draftsmanship," and "individual choice of subject and its handling."[3] During the 1930s she was known mainly for the quality of her still life paintings which showed, one critic said, "impeccable artistry."[4] At the end of her career, in the 1960s and 1970s, she produced specialized and highly disciplined still lifes of objects such as dead leaves and pieces of crumpled paper, which, said a critic, approached a "magical realism."[5] As an art activist she helped promote the rights of artists for fair remuneration.[6]
| Caroline Katzenstein
Caroline Katzenstein (1888–1968) was an American suffragist, activist, advocate for equal rights, insurance agent, and author. She was active in the local Philadelphia suffragist movement through the Pennsylvania branch of the National American Woman Suffrage Association and the Equal Franchise Society of Philadelphia. She played a role in the formation of the Congressional Union for Women Suffrage, which later became the National Women's Party. Katzenstein was also active in the movement for equal rights, serving on the Women's Joint Legislative Committee with Alice Paul, and championing the cause for the Equal Rights Amendment. She was the author of "Lifting the Curtain: the State and National Woman Suffrage Campaigns in Pennsylvania as I Saw Them" (1955).
|
| Who made the first wristwatch?
| History of watches
From the beginning, wristwatches were almost exclusively worn by women, while men used pocketwatches up until the early 20th century. The concept of the wristwatch goes back to the production of the very earliest watches in the 16th century. Some people say the world's first wristwatch was created by Abraham-Louis Breguet for Caroline Murat, Queen of Naples, in 1810.[21][22][23][24][25] Elizabeth I of England received a wristwatch from Robert Dudley in 1571, described as an arm watch. By the mid nineteenth century, most watchmakers produced a range of wristwatches, often marketed as bracelets, for women.[26]
| Rolex
In 1908, Wilsdorf registered the trademark "Rolex" and opened an office in La Chaux-de-Fonds, Switzerland.[14][15] The book The Best of Time: Rolex Wristwatches: An Unauthorized History by Jeffrey P. Hess and James Dowling says that the name was just made up.[16] One story, never confirmed by Wilsdorf, recounts that the name came from the French phrase horlogerie exquise, meaning "exquisite clockwork"[9] or as a contraction of "horological excellence". Wilsdorf was said to want his watch brand's name to be easily pronounceable in any language.[17] He also thought that the name "Rolex" was onomatopoeic, sounding like a watch being wound. It is easily pronounceable in many languages and, as all its upper-case letters have the same size, can be written symmetrically. It was also short enough to fit on the face of a watch.[17]
|
* Loss: [MatryoshkaLoss
](https://sbert.net/docs/package_reference/sentence_transformer/losses.html#matryoshkaloss) with these parameters:
```json
{
"loss": "MultipleNegativesRankingLoss",
"matryoshka_dims": [
1024,
512,
256,
128,
64,
32
],
"matryoshka_weights": [
1,
1,
1,
1,
1,
1
],
"n_dims_per_step": -1
}
```
### Training Hyperparameters
#### Non-Default Hyperparameters
- `eval_strategy`: steps
- `per_device_train_batch_size`: 2048
- `per_device_eval_batch_size`: 2048
- `learning_rate`: 0.2
- `num_train_epochs`: 1
- `warmup_ratio`: 0.1
- `bf16`: True
- `batch_sampler`: no_duplicates
#### All Hyperparameters
Click to expand
- `overwrite_output_dir`: False
- `do_predict`: False
- `eval_strategy`: steps
- `prediction_loss_only`: True
- `per_device_train_batch_size`: 2048
- `per_device_eval_batch_size`: 2048
- `per_gpu_train_batch_size`: None
- `per_gpu_eval_batch_size`: None
- `gradient_accumulation_steps`: 1
- `eval_accumulation_steps`: None
- `torch_empty_cache_steps`: None
- `learning_rate`: 0.2
- `weight_decay`: 0.0
- `adam_beta1`: 0.9
- `adam_beta2`: 0.999
- `adam_epsilon`: 1e-08
- `max_grad_norm`: 1.0
- `num_train_epochs`: 1
- `max_steps`: -1
- `lr_scheduler_type`: linear
- `lr_scheduler_kwargs`: {}
- `warmup_ratio`: 0.1
- `warmup_steps`: 0
- `log_level`: passive
- `log_level_replica`: warning
- `log_on_each_node`: True
- `logging_nan_inf_filter`: True
- `save_safetensors`: True
- `save_on_each_node`: False
- `save_only_model`: False
- `restore_callback_states_from_checkpoint`: False
- `no_cuda`: False
- `use_cpu`: False
- `use_mps_device`: False
- `seed`: 42
- `data_seed`: None
- `jit_mode_eval`: False
- `use_ipex`: False
- `bf16`: True
- `fp16`: False
- `fp16_opt_level`: O1
- `half_precision_backend`: auto
- `bf16_full_eval`: False
- `fp16_full_eval`: False
- `tf32`: None
- `local_rank`: 0
- `ddp_backend`: None
- `tpu_num_cores`: None
- `tpu_metrics_debug`: False
- `debug`: []
- `dataloader_drop_last`: False
- `dataloader_num_workers`: 0
- `dataloader_prefetch_factor`: None
- `past_index`: -1
- `disable_tqdm`: False
- `remove_unused_columns`: True
- `label_names`: None
- `load_best_model_at_end`: False
- `ignore_data_skip`: False
- `fsdp`: []
- `fsdp_min_num_params`: 0
- `fsdp_config`: {'min_num_params': 0, 'xla': False, 'xla_fsdp_v2': False, 'xla_fsdp_grad_ckpt': False}
- `fsdp_transformer_layer_cls_to_wrap`: None
- `accelerator_config`: {'split_batches': False, 'dispatch_batches': None, 'even_batches': True, 'use_seedable_sampler': True, 'non_blocking': False, 'gradient_accumulation_kwargs': None}
- `deepspeed`: None
- `label_smoothing_factor`: 0.0
- `optim`: adamw_torch
- `optim_args`: None
- `adafactor`: False
- `group_by_length`: False
- `length_column_name`: length
- `ddp_find_unused_parameters`: None
- `ddp_bucket_cap_mb`: None
- `ddp_broadcast_buffers`: False
- `dataloader_pin_memory`: True
- `dataloader_persistent_workers`: False
- `skip_memory_metrics`: True
- `use_legacy_prediction_loop`: False
- `push_to_hub`: False
- `resume_from_checkpoint`: None
- `hub_model_id`: None
- `hub_strategy`: every_save
- `hub_private_repo`: False
- `hub_always_push`: False
- `gradient_checkpointing`: False
- `gradient_checkpointing_kwargs`: None
- `include_inputs_for_metrics`: False
- `eval_do_concat_batches`: True
- `fp16_backend`: auto
- `push_to_hub_model_id`: None
- `push_to_hub_organization`: None
- `mp_parameters`:
- `auto_find_batch_size`: False
- `full_determinism`: False
- `torchdynamo`: None
- `ray_scope`: last
- `ddp_timeout`: 1800
- `torch_compile`: False
- `torch_compile_backend`: None
- `torch_compile_mode`: None
- `dispatch_batches`: None
- `split_batches`: None
- `include_tokens_per_second`: False
- `include_num_input_tokens_seen`: False
- `neftune_noise_alpha`: None
- `optim_target_modules`: None
- `batch_eval_metrics`: False
- `eval_on_start`: False
- `use_liger_kernel`: False
- `eval_use_gather_object`: False
- `batch_sampler`: no_duplicates
- `multi_dataset_batch_sampler`: proportional
### Training Logs
Click to expand
| Epoch | Step | Training Loss | gooaq loss | msmarco loss | squad loss | s2orc loss | allnli loss | paq loss | trivia qa loss | msmarco 10m loss | swim ir loss | pubmedqa loss | miracl loss | mldr loss | mr tydi loss | NanoClimateFEVER_cosine_ndcg@10 | NanoDBPedia_cosine_ndcg@10 | NanoFEVER_cosine_ndcg@10 | NanoFiQA2018_cosine_ndcg@10 | NanoHotpotQA_cosine_ndcg@10 | NanoMSMARCO_cosine_ndcg@10 | NanoNFCorpus_cosine_ndcg@10 | NanoNQ_cosine_ndcg@10 | NanoQuoraRetrieval_cosine_ndcg@10 | NanoSCIDOCS_cosine_ndcg@10 | NanoArguAna_cosine_ndcg@10 | NanoSciFact_cosine_ndcg@10 | NanoTouche2020_cosine_ndcg@10 | NanoBEIR_mean_cosine_ndcg@10 |
|:------:|:-----:|:-------------:|:----------:|:------------:|:----------:|:----------:|:-----------:|:--------:|:--------------:|:----------------:|:------------:|:-------------:|:-----------:|:---------:|:------------:|:-------------------------------:|:--------------------------:|:------------------------:|:---------------------------:|:---------------------------:|:--------------------------:|:---------------------------:|:---------------------:|:---------------------------------:|:--------------------------:|:--------------------------:|:--------------------------:|:-----------------------------:|:----------------------------:|
| 0 | 0 | - | - | - | - | - | - | - | - | - | - | - | - | - | - | 0.0741 | 0.3518 | 0.2118 | 0.0793 | 0.3538 | 0.3200 | 0.1954 | 0.1589 | 0.6759 | 0.1532 | 0.0945 | 0.4296 | 0.1455 | 0.2495 |
| 0.0000 | 1 | 32.2074 | - | - | - | - | - | - | - | - | - | - | - | - | - | - | - | - | - | - | - | - | - | - | - | - | - | - | - |
| 0.0064 | 250 | 22.7851 | 8.3992 | 17.7191 | 17.6791 | 16.6296 | 18.7913 | 12.1404 | 18.9992 | 12.1891 | 11.6795 | 26.3440 | 8.5795 | 19.3571 | 9.5985 | 0.2366 | 0.5129 | 0.6004 | 0.1960 | 0.6334 | 0.3941 | 0.2713 | 0.3392 | 0.7977 | 0.2416 | 0.3819 | 0.5448 | 0.4679 | 0.4321 |
| 0.0127 | 500 | 9.6296 | 4.6987 | 13.6254 | 11.7605 | 12.5290 | 17.2038 | 6.9342 | 12.0873 | 7.3539 | 9.1374 | 23.1663 | 4.2482 | 14.5991 | 3.3365 | 0.2929 | 0.5509 | 0.6529 | 0.2890 | 0.6495 | 0.4244 | 0.2873 | 0.3690 | 0.8830 | 0.2373 | 0.3815 | 0.5802 | 0.5422 | 0.4723 |
| 0.0191 | 750 | 6.7008 | 3.6302 | 11.7061 | 10.1299 | 11.2366 | 15.1612 | 5.5833 | 10.6967 | 5.7074 | 8.8117 | 23.2404 | 3.5115 | 12.5734 | 2.4346 | 0.3101 | 0.5565 | 0.6684 | 0.3406 | 0.6354 | 0.4111 | 0.2972 | 0.3894 | 0.8611 | 0.2513 | 0.3613 | 0.5840 | 0.5578 | 0.4788 |
| 0.0255 | 1000 | 5.8282 | 2.9789 | 11.2050 | 9.5095 | 10.6029 | 14.7717 | 5.0173 | 9.6170 | 5.1146 | 9.0596 | 22.3746 | 3.0912 | 12.2982 | 2.2626 | 0.3066 | 0.5514 | 0.6654 | 0.3252 | 0.6390 | 0.4139 | 0.2917 | 0.4168 | 0.8678 | 0.2590 | 0.3884 | 0.6214 | 0.5614 | 0.4852 |
| 0.0318 | 1250 | 5.3975 | 2.8335 | 11.0393 | 9.3407 | 9.6014 | 14.5350 | 4.8262 | 9.4577 | 4.9009 | 8.9271 | 21.8053 | 3.2513 | 12.2634 | 1.6880 | 0.3090 | 0.5449 | 0.6607 | 0.3432 | 0.6243 | 0.4145 | 0.3026 | 0.4392 | 0.8801 | 0.2608 | 0.3760 | 0.6102 | 0.5768 | 0.4879 |
| 0.0382 | 1500 | 5.3077 | 2.7030 | 10.6366 | 8.9914 | 9.8588 | 14.6669 | 4.6253 | 9.3728 | 4.5863 | 9.1788 | 22.0617 | 2.8378 | 10.9618 | 1.8702 | 0.3240 | 0.5421 | 0.7026 | 0.3507 | 0.6227 | 0.4134 | 0.3150 | 0.3996 | 0.8776 | 0.2493 | 0.3625 | 0.6120 | 0.5642 | 0.4874 |
| 0.0445 | 1750 | 4.9354 | 2.6691 | 10.6339 | 8.9606 | 9.7095 | 14.9174 | 4.5880 | 9.3114 | 4.1786 | 8.2898 | 22.8332 | 2.6850 | 11.3781 | 1.6352 | 0.3092 | 0.5591 | 0.6615 | 0.3253 | 0.6363 | 0.3926 | 0.3165 | 0.4057 | 0.9019 | 0.2600 | 0.3685 | 0.6030 | 0.5563 | 0.4843 |
| 0.0509 | 2000 | 4.8017 | 2.5867 | 10.0547 | 8.8155 | 9.6765 | 14.7973 | 4.3931 | 9.2721 | 4.0193 | 7.7955 | 23.4468 | 1.9884 | 11.4315 | 1.8009 | 0.3274 | 0.5615 | 0.7024 | 0.3531 | 0.6481 | 0.3959 | 0.3134 | 0.4183 | 0.8849 | 0.2505 | 0.3694 | 0.5991 | 0.5664 | 0.4916 |
| 0.0573 | 2250 | 4.8193 | 2.4974 | 9.9855 | 8.8389 | 9.6763 | 14.4220 | 4.3112 | 9.1019 | 4.0176 | 8.4064 | 22.7034 | 2.7534 | 11.5256 | 2.3585 | 0.3149 | 0.5392 | 0.6689 | 0.3344 | 0.6495 | 0.4080 | 0.3058 | 0.3953 | 0.8857 | 0.2588 | 0.3426 | 0.5986 | 0.5756 | 0.4829 |
| 0.0636 | 2500 | 4.8773 | 2.7116 | 10.2180 | 8.6935 | 9.7664 | 14.3161 | 4.2722 | 8.9829 | 4.2454 | 9.1911 | 22.8367 | 2.6666 | 11.6110 | 2.0147 | 0.3377 | 0.5455 | 0.6547 | 0.3130 | 0.6396 | 0.4259 | 0.3256 | 0.4226 | 0.8825 | 0.2491 | 0.3908 | 0.5852 | 0.5656 | 0.4875 |
| 0.0700 | 2750 | 4.5856 | 2.7758 | 9.9754 | 8.6197 | 9.6282 | 14.4828 | 4.1534 | 8.8766 | 4.2312 | 9.5281 | 21.9368 | 2.9119 | 9.7259 | 1.9405 | 0.3384 | 0.5763 | 0.6646 | 0.3068 | 0.6740 | 0.4195 | 0.3155 | 0.4301 | 0.8809 | 0.2366 | 0.3965 | 0.5963 | 0.5714 | 0.4928 |
| 0.0764 | 3000 | 4.3725 | 2.5993 | 10.1291 | 8.7361 | 8.9502 | 14.8227 | 4.1163 | 8.8371 | 4.1014 | 9.2259 | 23.4047 | 3.2715 | 10.3051 | 2.3604 | 0.3040 | 0.5703 | 0.6836 | 0.3006 | 0.6355 | 0.3993 | 0.3318 | 0.4236 | 0.9001 | 0.2579 | 0.3966 | 0.5902 | 0.5770 | 0.4900 |
| 0.0827 | 3250 | 4.4409 | 2.5753 | 10.0879 | 8.5131 | 8.7106 | 14.8015 | 4.0560 | 8.8296 | 4.1868 | 9.3069 | 22.5793 | 2.3810 | 8.6639 | 2.0435 | 0.3147 | 0.5806 | 0.6766 | 0.3342 | 0.6293 | 0.4134 | 0.3208 | 0.4089 | 0.8834 | 0.2656 | 0.3784 | 0.6119 | 0.5731 | 0.4916 |
| 0.0891 | 3500 | 4.6192 | 2.4352 | 9.9932 | 8.5716 | 9.2016 | 14.1559 | 4.0585 | 8.9413 | 3.8278 | 8.6089 | 22.9941 | 2.5541 | 9.4271 | 1.7271 | 0.3052 | 0.5531 | 0.6921 | 0.3284 | 0.6391 | 0.4027 | 0.3288 | 0.4235 | 0.8938 | 0.2565 | 0.3928 | 0.5848 | 0.5741 | 0.4904 |
| 0.0955 | 3750 | 4.4805 | 2.5370 | 10.0723 | 8.4652 | 8.8024 | 14.4678 | 4.0045 | 8.8487 | 3.6855 | 8.4129 | 22.5177 | 2.5961 | 9.1362 | 1.6572 | 0.2996 | 0.5669 | 0.7051 | 0.3007 | 0.6433 | 0.3822 | 0.3127 | 0.4419 | 0.8853 | 0.2741 | 0.3696 | 0.5911 | 0.5796 | 0.4886 |
| 0.1018 | 4000 | 4.3246 | 2.4130 | 10.0235 | 8.4203 | 9.1794 | 14.4445 | 3.9667 | 8.8021 | 3.6692 | 8.0637 | 22.1590 | 2.2690 | 9.5487 | 1.4625 | 0.2987 | 0.5659 | 0.6905 | 0.3103 | 0.6280 | 0.4004 | 0.3025 | 0.4273 | 0.9026 | 0.2728 | 0.3710 | 0.6004 | 0.5746 | 0.4881 |
| 0.1082 | 4250 | 4.547 | 2.2318 | 10.0773 | 8.4970 | 8.6540 | 13.9845 | 3.9893 | 8.7805 | 3.4480 | 8.1038 | 21.2066 | 2.4344 | 9.2932 | 1.4761 | 0.3037 | 0.5760 | 0.7118 | 0.3239 | 0.6135 | 0.4056 | 0.3170 | 0.4323 | 0.8860 | 0.2620 | 0.3887 | 0.6211 | 0.5824 | 0.4941 |
| 0.1145 | 4500 | 4.3008 | 2.3243 | 9.8733 | 8.5042 | 9.0158 | 14.0935 | 3.9014 | 8.8306 | 3.5557 | 8.4240 | 21.2823 | 2.6280 | 9.4869 | 1.8310 | 0.3076 | 0.5788 | 0.6867 | 0.3187 | 0.6190 | 0.3936 | 0.3181 | 0.4160 | 0.8895 | 0.2564 | 0.3960 | 0.6148 | 0.5736 | 0.4899 |
| 0.1209 | 4750 | 4.2386 | 2.4259 | 9.8799 | 8.3964 | 9.1116 | 13.9412 | 3.8572 | 8.7955 | 3.6524 | 9.6881 | 21.3812 | 2.2282 | 8.9280 | 1.5408 | 0.3393 | 0.5783 | 0.7190 | 0.3137 | 0.6239 | 0.3953 | 0.3044 | 0.4231 | 0.8768 | 0.2636 | 0.3828 | 0.6043 | 0.5662 | 0.4916 |
| 0.1273 | 5000 | 4.141 | 2.4005 | 9.9973 | 8.2741 | 9.1627 | 14.4273 | 3.7931 | 8.7825 | 3.6856 | 9.0001 | 21.6595 | 2.2374 | 9.2771 | 1.4845 | 0.3243 | 0.5705 | 0.6858 | 0.3304 | 0.6328 | 0.3888 | 0.3145 | 0.4096 | 0.8775 | 0.2492 | 0.3769 | 0.6001 | 0.5600 | 0.4862 |
| 0.1336 | 5250 | 4.3221 | 2.4200 | 9.8792 | 8.2559 | 9.0431 | 13.9564 | 3.8055 | 8.5773 | 3.6137 | 8.1900 | 21.6272 | 2.2271 | 8.1229 | 1.6308 | 0.3207 | 0.5876 | 0.6945 | 0.3449 | 0.6232 | 0.4072 | 0.3011 | 0.4084 | 0.8894 | 0.2557 | 0.3668 | 0.5905 | 0.5582 | 0.4883 |
| 0.1400 | 5500 | 4.2121 | 2.3857 | 10.1277 | 8.3257 | 9.0878 | 13.8545 | 3.7696 | 8.6034 | 3.5613 | 8.7845 | 21.5562 | 2.3611 | 7.5145 | 1.9243 | 0.3160 | 0.5683 | 0.7024 | 0.3382 | 0.6244 | 0.4038 | 0.3075 | 0.4316 | 0.8784 | 0.2603 | 0.3876 | 0.5866 | 0.5650 | 0.4900 |
| 0.1464 | 5750 | 4.1071 | 2.4265 | 9.9555 | 8.0947 | 9.1289 | 14.0017 | 3.7337 | 8.6306 | 3.4562 | 8.3132 | 21.7894 | 2.1157 | 8.1967 | 1.5567 | 0.3317 | 0.5604 | 0.7101 | 0.3645 | 0.6460 | 0.3857 | 0.2987 | 0.4236 | 0.8830 | 0.2535 | 0.3867 | 0.5767 | 0.5612 | 0.4909 |
| 0.1527 | 6000 | 4.1189 | 2.3586 | 10.0799 | 8.0905 | 9.0291 | 14.0232 | 3.7064 | 8.5220 | 3.4742 | 8.3858 | 21.5903 | 2.1663 | 7.6242 | 1.4405 | 0.3264 | 0.5614 | 0.6825 | 0.3668 | 0.6296 | 0.3972 | 0.2863 | 0.4296 | 0.8869 | 0.2482 | 0.3809 | 0.6004 | 0.5556 | 0.4886 |
| 0.1591 | 6250 | 4.0873 | 2.2906 | 9.9813 | 8.1351 | 8.5907 | 13.8665 | 3.7028 | 8.5648 | 3.5042 | 8.1623 | 21.6688 | 2.2940 | 7.6652 | 1.5228 | 0.3444 | 0.5666 | 0.7035 | 0.3415 | 0.6188 | 0.3992 | 0.2989 | 0.4318 | 0.8816 | 0.2504 | 0.4014 | 0.6042 | 0.5637 | 0.4928 |
| 0.1654 | 6500 | 3.9586 | 2.2378 | 9.9318 | 8.0887 | 8.7977 | 14.1260 | 3.6614 | 8.5028 | 3.3178 | 8.3118 | 21.5718 | 2.2074 | 8.0905 | 1.7266 | 0.3299 | 0.5828 | 0.6994 | 0.3505 | 0.6375 | 0.3988 | 0.3225 | 0.4173 | 0.8891 | 0.2448 | 0.3930 | 0.6034 | 0.5614 | 0.4946 |
| 0.1718 | 6750 | 4.1981 | 2.2863 | 9.5475 | 7.6881 | 8.5462 | 13.6929 | 3.6704 | 8.6660 | 3.3401 | 8.9262 | 21.8933 | 2.0578 | 8.4832 | 1.6796 | 0.3456 | 0.5600 | 0.6983 | 0.3549 | 0.6445 | 0.3844 | 0.3139 | 0.4263 | 0.8952 | 0.2626 | 0.3877 | 0.5820 | 0.5597 | 0.4935 |
| 0.1782 | 7000 | 4.0528 | 2.3292 | 9.5129 | 7.8273 | 8.7743 | 13.6930 | 3.6284 | 8.6346 | 3.3430 | 8.5204 | 21.3129 | 2.3350 | 8.8695 | 1.9034 | 0.3457 | 0.5673 | 0.6850 | 0.3274 | 0.6321 | 0.3981 | 0.3171 | 0.4252 | 0.8830 | 0.2643 | 0.3901 | 0.5888 | 0.5590 | 0.4910 |
| 0.1845 | 7250 | 4.0547 | 2.2386 | 9.5373 | 7.9214 | 8.7896 | 13.6151 | 3.6172 | 8.5316 | 3.3128 | 9.3566 | 21.4568 | 2.3743 | 9.1696 | 1.7235 | 0.3528 | 0.5597 | 0.6931 | 0.3369 | 0.6327 | 0.3951 | 0.3111 | 0.4368 | 0.8787 | 0.2552 | 0.3758 | 0.5911 | 0.5515 | 0.4900 |
| 0.1909 | 7500 | 4.3005 | 2.2273 | 9.4397 | 7.9013 | 8.8606 | 13.3847 | 3.6401 | 8.4134 | 3.2583 | 8.3415 | 21.4206 | 2.4573 | 9.2348 | 1.4832 | 0.3557 | 0.5642 | 0.7145 | 0.3380 | 0.6412 | 0.3772 | 0.3085 | 0.4278 | 0.8792 | 0.2522 | 0.3738 | 0.5843 | 0.5587 | 0.4904 |
| 0.1973 | 7750 | 4.0054 | 2.2277 | 9.4653 | 7.9297 | 8.5999 | 13.6106 | 3.6049 | 8.3861 | 3.2335 | 9.3198 | 21.6595 | 2.4730 | 8.7335 | 1.6145 | 0.3396 | 0.5535 | 0.6901 | 0.3556 | 0.6311 | 0.3867 | 0.3182 | 0.4308 | 0.8692 | 0.2590 | 0.3654 | 0.5925 | 0.5558 | 0.4883 |
| 0.2036 | 8000 | 3.8426 | 2.2970 | 9.4352 | 7.9532 | 8.6501 | 13.9004 | 3.5835 | 8.3664 | 3.2109 | 8.4302 | 21.0340 | 2.1047 | 9.0103 | 1.1751 | 0.3420 | 0.5695 | 0.6868 | 0.3746 | 0.6434 | 0.4042 | 0.3193 | 0.4259 | 0.8847 | 0.2623 | 0.3785 | 0.5945 | 0.5702 | 0.4966 |
| 0.2100 | 8250 | 3.9404 | 2.2417 | 9.5349 | 7.8978 | 8.6899 | 13.8131 | 3.5697 | 8.3664 | 3.1548 | 8.6003 | 21.6214 | 2.0881 | 9.1829 | 0.9559 | 0.3306 | 0.5651 | 0.6959 | 0.3448 | 0.6455 | 0.3857 | 0.3123 | 0.4431 | 0.9009 | 0.2580 | 0.3981 | 0.6073 | 0.5748 | 0.4971 |
| 0.2164 | 8500 | 3.9522 | 2.2103 | 9.6575 | 7.9030 | 8.3617 | 14.0083 | 3.5433 | 8.3198 | 3.2148 | 8.5004 | 20.8166 | 2.3194 | 8.0428 | 1.2475 | 0.3343 | 0.5688 | 0.7054 | 0.3411 | 0.6625 | 0.3919 | 0.3148 | 0.4213 | 0.8965 | 0.2665 | 0.3454 | 0.6133 | 0.5782 | 0.4954 |
| 0.2227 | 8750 | 3.9665 | 2.1659 | 9.7216 | 7.8772 | 8.6394 | 13.9406 | 3.5289 | 8.3360 | 3.1863 | 8.7835 | 21.2033 | 2.1874 | 8.4683 | 1.2399 | 0.3231 | 0.5715 | 0.6794 | 0.3378 | 0.6684 | 0.3966 | 0.3171 | 0.4048 | 0.8887 | 0.2660 | 0.3461 | 0.6094 | 0.5559 | 0.4896 |
| 0.2291 | 9000 | 4.0217 | 2.1042 | 9.6765 | 7.8951 | 8.4255 | 13.8092 | 3.5314 | 8.3896 | 3.1097 | 8.0204 | 21.4246 | 2.0600 | 8.7244 | 1.3343 | 0.3218 | 0.5696 | 0.6931 | 0.3569 | 0.6654 | 0.3978 | 0.3159 | 0.4193 | 0.9000 | 0.2827 | 0.3750 | 0.5890 | 0.5796 | 0.4974 |
| 0.2354 | 9250 | 4.0008 | 2.0865 | 9.4154 | 7.9689 | 8.5298 | 13.6352 | 3.5371 | 8.4191 | 3.0414 | 8.4828 | 21.5173 | 1.9966 | 7.6465 | 1.1097 | 0.3282 | 0.5675 | 0.6934 | 0.3476 | 0.6559 | 0.3907 | 0.3272 | 0.4132 | 0.9038 | 0.2712 | 0.3891 | 0.5951 | 0.5716 | 0.4965 |
| 0.2418 | 9500 | 3.8041 | 2.0969 | 9.4478 | 7.9720 | 8.6298 | 13.7493 | 3.5003 | 8.4702 | 3.0939 | 8.5108 | 21.6929 | 1.9457 | 7.9947 | 1.2784 | 0.3291 | 0.5655 | 0.6915 | 0.3533 | 0.6495 | 0.3949 | 0.3291 | 0.4313 | 0.9007 | 0.2641 | 0.3910 | 0.5838 | 0.5710 | 0.4965 |
| 0.2482 | 9750 | 3.9483 | 2.1627 | 9.5085 | 7.8994 | 8.7048 | 13.4591 | 3.4941 | 8.3342 | 3.1202 | 8.7011 | 20.9101 | 1.8594 | 7.8214 | 1.1181 | 0.3312 | 0.5665 | 0.6870 | 0.3608 | 0.6530 | 0.4038 | 0.3267 | 0.4511 | 0.8973 | 0.2613 | 0.4063 | 0.5832 | 0.5660 | 0.4996 |
| 0.2545 | 10000 | 3.9843 | 2.1229 | 9.4348 | 7.9006 | 8.2377 | 13.5086 | 3.4905 | 8.2574 | 3.0114 | 8.3314 | 20.5157 | 1.9033 | 6.7485 | 1.1358 | 0.3365 | 0.5704 | 0.6858 | 0.3616 | 0.6561 | 0.3953 | 0.3263 | 0.4322 | 0.8833 | 0.2690 | 0.3995 | 0.6004 | 0.5691 | 0.4989 |
| 0.2609 | 10250 | 3.8779 | 2.1442 | 9.4343 | 7.9411 | 8.4677 | 13.5196 | 3.4558 | 8.2314 | 3.0592 | 8.6126 | 20.3879 | 1.9378 | 6.8287 | 1.1270 | 0.3192 | 0.5688 | 0.7051 | 0.3669 | 0.6577 | 0.3913 | 0.3167 | 0.4479 | 0.8978 | 0.2619 | 0.4030 | 0.5966 | 0.5699 | 0.5002 |
| 0.2673 | 10500 | 3.8708 | 2.1487 | 9.6030 | 7.9352 | 8.4657 | 13.3417 | 3.4574 | 8.3005 | 3.0167 | 8.4265 | 20.5354 | 1.9044 | 6.9217 | 1.1079 | 0.3255 | 0.5730 | 0.7020 | 0.3445 | 0.6527 | 0.3861 | 0.3178 | 0.4322 | 0.8859 | 0.2644 | 0.3997 | 0.5969 | 0.5739 | 0.4965 |
| 0.2736 | 10750 | 3.8153 | 2.1486 | 9.2440 | 7.8432 | 8.5271 | 13.4366 | 3.4702 | 8.3222 | 2.9680 | 8.4780 | 20.6855 | 1.8790 | 6.6261 | 1.0838 | 0.3240 | 0.5727 | 0.6970 | 0.3487 | 0.6546 | 0.3897 | 0.3294 | 0.4421 | 0.8908 | 0.2548 | 0.3802 | 0.6028 | 0.5744 | 0.4970 |
| 0.2800 | 11000 | 3.9693 | 2.1542 | 9.4072 | 7.8122 | 8.6226 | 13.1083 | 3.4426 | 8.2961 | 3.0086 | 8.6255 | 20.5001 | 1.9112 | 6.6305 | 1.1554 | 0.3273 | 0.5631 | 0.6912 | 0.3502 | 0.6431 | 0.4012 | 0.3173 | 0.4422 | 0.8834 | 0.2740 | 0.3829 | 0.6117 | 0.5745 | 0.4971 |
| 0.2864 | 11250 | 3.7596 | 2.1288 | 9.4115 | 7.8479 | 8.6295 | 13.1814 | 3.4282 | 8.2609 | 2.9531 | 8.6024 | 20.7827 | 1.8339 | 7.0503 | 1.0273 | 0.3301 | 0.5714 | 0.6814 | 0.3457 | 0.6447 | 0.3962 | 0.3142 | 0.4545 | 0.8751 | 0.2687 | 0.3827 | 0.5921 | 0.5492 | 0.4928 |
| 0.2927 | 11500 | 3.7377 | 2.1764 | 9.2284 | 7.7482 | 8.6753 | 13.2556 | 3.4186 | 8.2092 | 2.9631 | 8.2251 | 20.9522 | 1.8887 | 6.8783 | 1.1493 | 0.3184 | 0.5710 | 0.6896 | 0.3620 | 0.6411 | 0.4016 | 0.3141 | 0.4598 | 0.8871 | 0.2660 | 0.4035 | 0.5946 | 0.5680 | 0.4982 |
| 0.2991 | 11750 | 3.645 | 2.1757 | 9.2386 | 7.7988 | 8.4091 | 13.3105 | 3.3986 | 8.1770 | 3.0392 | 8.3319 | 20.8279 | 1.8464 | 7.2340 | 1.2369 | 0.3066 | 0.5680 | 0.6938 | 0.3413 | 0.6498 | 0.4035 | 0.3119 | 0.4692 | 0.8776 | 0.2697 | 0.4018 | 0.5937 | 0.5725 | 0.4969 |
| 0.3054 | 12000 | 3.8302 | 2.1730 | 9.2542 | 7.8031 | 8.3773 | 13.3083 | 3.4245 | 8.2024 | 2.9451 | 8.4451 | 20.3890 | 1.8992 | 7.1868 | 1.3149 | 0.3022 | 0.5639 | 0.7047 | 0.3580 | 0.6585 | 0.3953 | 0.3193 | 0.4512 | 0.8757 | 0.2667 | 0.4016 | 0.5815 | 0.5653 | 0.4957 |
| 0.3118 | 12250 | 3.7341 | 2.1580 | 9.1449 | 7.7487 | 8.2782 | 13.4871 | 3.4325 | 8.1531 | 2.8524 | 8.0765 | 20.4420 | 1.8084 | 7.4004 | 1.1942 | 0.3217 | 0.5648 | 0.7022 | 0.3658 | 0.6597 | 0.4010 | 0.3204 | 0.4470 | 0.8778 | 0.2668 | 0.4062 | 0.5841 | 0.5764 | 0.4995 |
| 0.3182 | 12500 | 3.6937 | 2.2003 | 9.0298 | 7.7776 | 8.3088 | 13.3345 | 3.4198 | 8.0772 | 2.8139 | 8.7163 | 20.4754 | 1.8802 | 7.2714 | 1.2016 | 0.3086 | 0.5676 | 0.6930 | 0.3609 | 0.6532 | 0.4067 | 0.3228 | 0.4341 | 0.8737 | 0.2723 | 0.4014 | 0.5783 | 0.5799 | 0.4964 |
| 0.3245 | 12750 | 3.6917 | 2.1808 | 9.0285 | 7.7770 | 8.3062 | 13.5737 | 3.3953 | 8.1291 | 2.8232 | 8.3426 | 20.9837 | 1.9420 | 7.1199 | 1.2568 | 0.3288 | 0.5620 | 0.6855 | 0.3512 | 0.6575 | 0.4069 | 0.3309 | 0.4372 | 0.8906 | 0.2709 | 0.4106 | 0.5928 | 0.5874 | 0.5009 |
| 0.3309 | 13000 | 3.6376 | 2.1621 | 9.0474 | 7.7871 | 8.1721 | 13.4874 | 3.3904 | 8.1507 | 2.8109 | 8.5607 | 21.0805 | 1.9734 | 7.0553 | 1.3466 | 0.3250 | 0.5686 | 0.6850 | 0.3520 | 0.6630 | 0.4044 | 0.3258 | 0.4424 | 0.8666 | 0.2684 | 0.4038 | 0.5769 | 0.5725 | 0.4965 |
| 0.3373 | 13250 | 3.7786 | 2.1146 | 9.1181 | 7.7333 | 8.2758 | 13.4782 | 3.3906 | 8.2021 | 2.8320 | 8.3097 | 21.1471 | 1.8529 | 7.3608 | 1.2242 | 0.3282 | 0.5649 | 0.6997 | 0.3761 | 0.6680 | 0.4097 | 0.3242 | 0.4143 | 0.8873 | 0.2784 | 0.3958 | 0.5956 | 0.5745 | 0.5013 |
| 0.3436 | 13500 | 3.8654 | 2.2053 | 9.0632 | 7.6973 | 8.4055 | 13.2312 | 3.3747 | 8.1627 | 2.8245 | 8.4075 | 20.2899 | 1.7553 | 7.1383 | 1.1577 | 0.3316 | 0.5672 | 0.6693 | 0.3901 | 0.6695 | 0.4017 | 0.3213 | 0.4138 | 0.8953 | 0.2703 | 0.4023 | 0.5856 | 0.5821 | 0.5000 |
| 0.3500 | 13750 | 3.7545 | 2.1424 | 9.0522 | 7.6998 | 8.3319 | 13.5322 | 3.3625 | 8.1303 | 2.8320 | 8.1860 | 20.4538 | 1.7997 | 7.0770 | 1.2512 | 0.3385 | 0.5654 | 0.6937 | 0.3856 | 0.6659 | 0.4025 | 0.3225 | 0.4156 | 0.8994 | 0.2675 | 0.4113 | 0.5886 | 0.5770 | 0.5026 |
| 0.3564 | 14000 | 3.715 | 2.1826 | 8.9396 | 7.6755 | 8.3111 | 13.3344 | 3.3331 | 8.1973 | 2.8760 | 8.8218 | 20.6306 | 1.9014 | 7.3386 | 1.2366 | 0.3256 | 0.5675 | 0.6903 | 0.3846 | 0.6706 | 0.4032 | 0.3300 | 0.4426 | 0.8876 | 0.2647 | 0.4082 | 0.5874 | 0.5770 | 0.5030 |
| 0.3627 | 14250 | 3.6348 | 2.1393 | 9.0032 | 7.7843 | 8.3942 | 13.2654 | 3.3368 | 8.1124 | 2.8874 | 8.5999 | 20.8261 | 1.8395 | 7.5311 | 1.1380 | 0.3339 | 0.5642 | 0.7175 | 0.3780 | 0.6600 | 0.3965 | 0.3229 | 0.4305 | 0.8915 | 0.2714 | 0.3914 | 0.5816 | 0.5732 | 0.5009 |
| 0.3691 | 14500 | 3.604 | 2.1709 | 8.9648 | 7.7166 | 8.4281 | 13.5507 | 3.3138 | 8.1362 | 2.8989 | 8.3940 | 20.6827 | 1.9298 | 7.3468 | 1.2705 | 0.3416 | 0.5594 | 0.7198 | 0.3776 | 0.6605 | 0.3965 | 0.3295 | 0.4341 | 0.8838 | 0.2632 | 0.3995 | 0.5884 | 0.5693 | 0.5018 |
| 0.3754 | 14750 | 3.5398 | 2.1451 | 9.0195 | 7.7407 | 8.4766 | 13.6169 | 3.2839 | 8.1386 | 2.9178 | 8.2585 | 21.1674 | 1.9484 | 7.5143 | 1.2747 | 0.3418 | 0.5592 | 0.6971 | 0.3763 | 0.6539 | 0.4060 | 0.3264 | 0.4214 | 0.8805 | 0.2524 | 0.3855 | 0.5872 | 0.5735 | 0.4970 |
| 0.3818 | 15000 | 3.7153 | 2.1038 | 8.9847 | 7.7279 | 8.2667 | 13.4033 | 3.2862 | 8.1232 | 2.8999 | 8.3224 | 20.9361 | 1.9569 | 7.3440 | 1.3036 | 0.3407 | 0.5687 | 0.7066 | 0.3497 | 0.6532 | 0.4043 | 0.3317 | 0.4300 | 0.8830 | 0.2564 | 0.3884 | 0.6013 | 0.5752 | 0.4992 |
| 0.3882 | 15250 | 3.752 | 2.0765 | 9.0293 | 7.7275 | 8.3168 | 13.2782 | 3.3105 | 8.0286 | 2.8404 | 8.2745 | 20.9582 | 1.7465 | 7.5436 | 1.2770 | 0.3313 | 0.5710 | 0.6945 | 0.3742 | 0.6699 | 0.4022 | 0.3327 | 0.4277 | 0.8903 | 0.2640 | 0.3872 | 0.5821 | 0.5662 | 0.4995 |
| 0.3945 | 15500 | 3.7794 | 2.0409 | 9.0264 | 7.7501 | 8.3903 | 13.3169 | 3.3058 | 8.0128 | 2.8463 | 8.5307 | 21.5663 | 1.7179 | 7.7448 | 1.1827 | 0.3286 | 0.5684 | 0.6961 | 0.3692 | 0.6743 | 0.4031 | 0.3267 | 0.4267 | 0.8927 | 0.2549 | 0.3777 | 0.5810 | 0.5686 | 0.4975 |
| 0.4009 | 15750 | 3.7444 | 2.0122 | 9.0629 | 7.7541 | 8.0134 | 13.2091 | 3.2956 | 8.0839 | 2.8528 | 8.1722 | 20.9378 | 1.7628 | 7.8655 | 1.2965 | 0.3282 | 0.5744 | 0.6839 | 0.3807 | 0.6644 | 0.4032 | 0.3277 | 0.4451 | 0.8896 | 0.2706 | 0.3916 | 0.5851 | 0.5685 | 0.5010 |
| 0.4073 | 16000 | 3.7817 | 2.0448 | 9.1787 | 7.7705 | 8.0529 | 13.1694 | 3.3128 | 8.1419 | 2.8104 | 8.2099 | 21.0454 | 1.7436 | 7.2934 | 1.2463 | 0.3137 | 0.5640 | 0.6884 | 0.3692 | 0.6600 | 0.3978 | 0.3215 | 0.4314 | 0.8937 | 0.2719 | 0.4094 | 0.6031 | 0.5697 | 0.4995 |
| 0.4136 | 16250 | 3.7293 | 2.0586 | 9.2379 | 7.7514 | 8.1877 | 13.1981 | 3.2983 | 8.0763 | 2.8564 | 8.6500 | 20.9279 | 1.8403 | 7.2051 | 1.1732 | 0.3405 | 0.5648 | 0.6944 | 0.3620 | 0.6614 | 0.3953 | 0.3286 | 0.4245 | 0.8921 | 0.2698 | 0.3946 | 0.5915 | 0.5770 | 0.4997 |
| 0.4200 | 16500 | 3.6243 | 2.0477 | 9.1718 | 7.6943 | 7.9493 | 13.3019 | 3.2908 | 8.0963 | 2.8306 | 8.7436 | 20.6790 | 1.8745 | 7.4356 | 1.1781 | 0.3272 | 0.5657 | 0.6944 | 0.3726 | 0.6848 | 0.3974 | 0.3318 | 0.4274 | 0.8939 | 0.2636 | 0.3948 | 0.5950 | 0.5746 | 0.5018 |
| 0.4263 | 16750 | 3.5071 | 2.0483 | 9.2054 | 7.7004 | 8.1887 | 13.3662 | 3.2727 | 7.9229 | 2.8256 | 8.2771 | 21.1584 | 1.8469 | 7.6476 | 1.2131 | 0.3318 | 0.5660 | 0.7000 | 0.3801 | 0.6902 | 0.3938 | 0.3224 | 0.4191 | 0.8969 | 0.2643 | 0.4052 | 0.6106 | 0.5684 | 0.5038 |
| 0.4327 | 17000 | 3.6337 | 2.0383 | 9.1228 | 7.7337 | 8.2262 | 13.2250 | 3.2714 | 7.9983 | 2.7662 | 8.4949 | 20.8407 | 1.8184 | 7.7876 | 1.2807 | 0.3251 | 0.5595 | 0.6957 | 0.3897 | 0.6697 | 0.3933 | 0.3123 | 0.4334 | 0.8934 | 0.2663 | 0.3935 | 0.5931 | 0.5700 | 0.4996 |
| 0.4391 | 17250 | 3.5075 | 2.0327 | 8.9777 | 7.7194 | 8.2392 | 13.4002 | 3.2678 | 7.9239 | 2.7551 | 8.2470 | 21.1674 | 1.7744 | 7.9402 | 1.3115 | 0.3418 | 0.5595 | 0.7123 | 0.3790 | 0.6625 | 0.3978 | 0.3174 | 0.4232 | 0.8866 | 0.2683 | 0.3926 | 0.5876 | 0.5764 | 0.5004 |
| 0.4454 | 17500 | 3.6595 | 2.0419 | 8.9509 | 7.6536 | 8.3099 | 13.3537 | 3.2766 | 7.9939 | 2.7604 | 8.3880 | 20.8993 | 1.8358 | 7.6156 | 1.2238 | 0.3311 | 0.5643 | 0.7049 | 0.3564 | 0.6686 | 0.3932 | 0.3099 | 0.4350 | 0.8872 | 0.2687 | 0.3826 | 0.5854 | 0.5665 | 0.4964 |
| 0.4518 | 17750 | 3.5743 | 2.0049 | 9.0019 | 7.6693 | 8.3489 | 13.3261 | 3.2758 | 8.0051 | 2.7881 | 8.4247 | 20.8115 | 1.8714 | 7.7491 | 1.1884 | 0.3341 | 0.5614 | 0.6972 | 0.3571 | 0.6625 | 0.3893 | 0.3036 | 0.4368 | 0.8911 | 0.2625 | 0.3861 | 0.5734 | 0.5681 | 0.4941 |
| 0.4582 | 18000 | 3.6038 | 1.9810 | 9.0106 | 7.7162 | 8.3584 | 13.1195 | 3.2674 | 8.0266 | 2.8184 | 8.4383 | 20.5199 | 1.8854 | 7.8663 | 1.1762 | 0.3318 | 0.5583 | 0.7086 | 0.3591 | 0.6509 | 0.3878 | 0.3137 | 0.4247 | 0.8831 | 0.2601 | 0.3921 | 0.5978 | 0.5648 | 0.4948 |
| 0.4645 | 18250 | 3.6903 | 2.0005 | 9.0187 | 7.6743 | 8.4280 | 13.0108 | 3.2733 | 7.8845 | 2.7810 | 8.3511 | 20.1457 | 1.7802 | 8.0015 | 1.0885 | 0.3407 | 0.5657 | 0.7033 | 0.3626 | 0.6644 | 0.3841 | 0.3299 | 0.4358 | 0.8844 | 0.2642 | 0.3904 | 0.5871 | 0.5632 | 0.4981 |
| 0.4709 | 18500 | 3.7208 | 1.9972 | 9.1020 | 7.6472 | 8.1589 | 13.0717 | 3.2601 | 8.0039 | 2.7673 | 8.3361 | 20.0231 | 1.8054 | 7.7381 | 1.1832 | 0.3328 | 0.5652 | 0.7043 | 0.3473 | 0.6693 | 0.3810 | 0.3313 | 0.4293 | 0.8770 | 0.2633 | 0.3946 | 0.5914 | 0.5634 | 0.4962 |
| 0.4773 | 18750 | 3.6357 | 2.0069 | 9.1473 | 7.6843 | 8.2110 | 13.1578 | 3.2540 | 7.9856 | 2.7390 | 8.6913 | 20.3263 | 1.8252 | 7.9545 | 1.0354 | 0.3285 | 0.5631 | 0.7093 | 0.3648 | 0.6685 | 0.3842 | 0.3285 | 0.4361 | 0.8918 | 0.2744 | 0.4065 | 0.5814 | 0.5610 | 0.4998 |
| 0.4836 | 19000 | 3.5737 | 1.9755 | 9.1397 | 7.6784 | 8.2604 | 13.3462 | 3.2391 | 7.9876 | 2.7643 | 8.4540 | 20.2047 | 1.7528 | 7.6572 | 1.0906 | 0.3284 | 0.5631 | 0.7083 | 0.3657 | 0.6660 | 0.3795 | 0.3186 | 0.4393 | 0.8876 | 0.2613 | 0.4056 | 0.5835 | 0.5637 | 0.4977 |
| 0.4900 | 19250 | 3.5325 | 2.0232 | 9.1987 | 7.6517 | 8.2727 | 13.1941 | 3.2276 | 7.9841 | 2.7238 | 8.4698 | 19.9076 | 1.8015 | 7.2144 | 1.1134 | 0.3337 | 0.5635 | 0.7076 | 0.3570 | 0.6572 | 0.3891 | 0.3297 | 0.4360 | 0.8864 | 0.2689 | 0.3997 | 0.5813 | 0.5608 | 0.4978 |
| 0.4963 | 19500 | 3.4782 | 2.0033 | 9.1235 | 7.7026 | 8.3383 | 13.1817 | 3.2308 | 8.0122 | 2.6934 | 8.4448 | 19.7121 | 1.7192 | 6.9417 | 0.9934 | 0.3222 | 0.5656 | 0.7009 | 0.3562 | 0.6654 | 0.3792 | 0.3292 | 0.4544 | 0.8803 | 0.2703 | 0.4027 | 0.5892 | 0.5611 | 0.4982 |
| 0.5027 | 19750 | 3.7141 | 1.9830 | 9.2166 | 7.6724 | 8.3188 | 13.1514 | 3.2465 | 8.0979 | 2.7005 | 8.1956 | 19.8289 | 1.6910 | 7.1995 | 1.0668 | 0.3323 | 0.5632 | 0.7209 | 0.3442 | 0.6745 | 0.3864 | 0.3287 | 0.4255 | 0.8828 | 0.2751 | 0.4097 | 0.5785 | 0.5597 | 0.4986 |
| 0.5091 | 20000 | 3.7058 | 1.9625 | 9.1729 | 7.6554 | 8.3323 | 13.0269 | 3.2368 | 8.0075 | 2.7159 | 8.6974 | 20.1650 | 1.6862 | 7.3387 | 1.1706 | 0.3414 | 0.5637 | 0.7214 | 0.3498 | 0.6745 | 0.3817 | 0.3309 | 0.4290 | 0.8861 | 0.2711 | 0.3861 | 0.5854 | 0.5757 | 0.4998 |
| 0.5154 | 20250 | 3.502 | 1.9983 | 9.1304 | 7.6354 | 8.3832 | 13.2376 | 3.2262 | 7.9229 | 2.7119 | 8.7638 | 20.0759 | 1.6633 | 6.9686 | 1.1490 | 0.3296 | 0.5703 | 0.7116 | 0.3409 | 0.6717 | 0.3847 | 0.3294 | 0.4198 | 0.8859 | 0.2655 | 0.3924 | 0.5836 | 0.5647 | 0.4962 |
| 0.5218 | 20500 | 3.6424 | 1.9867 | 9.0935 | 7.6470 | 8.4280 | 13.0866 | 3.2338 | 7.9608 | 2.7225 | 8.4571 | 20.1605 | 1.6184 | 6.8877 | 1.1519 | 0.3377 | 0.5682 | 0.7128 | 0.3479 | 0.6646 | 0.3900 | 0.3255 | 0.4408 | 0.8863 | 0.2655 | 0.4184 | 0.5862 | 0.5627 | 0.5005 |
| 0.5282 | 20750 | 3.6085 | 1.9589 | 9.1559 | 7.6282 | 8.3675 | 13.1289 | 3.2393 | 8.0050 | 2.7199 | 8.3705 | 20.4945 | 1.6721 | 6.8649 | 1.2063 | 0.3328 | 0.5719 | 0.7054 | 0.3526 | 0.6755 | 0.3974 | 0.3280 | 0.4204 | 0.8926 | 0.2686 | 0.4145 | 0.5783 | 0.5553 | 0.4995 |
| 0.5345 | 21000 | 3.5763 | 1.9392 | 9.1139 | 7.6917 | 8.2745 | 13.3345 | 3.2333 | 7.9848 | 2.7010 | 8.4815 | 20.5463 | 1.7049 | 7.0751 | 1.1276 | 0.3251 | 0.5710 | 0.7128 | 0.3561 | 0.6642 | 0.4022 | 0.3225 | 0.4394 | 0.8997 | 0.2737 | 0.4106 | 0.5732 | 0.5592 | 0.5008 |
| 0.5409 | 21250 | 3.5401 | 1.9744 | 9.0583 | 7.5955 | 8.2868 | 13.2877 | 3.2309 | 7.9686 | 2.6641 | 8.2829 | 20.3974 | 1.6843 | 6.9287 | 1.0128 | 0.3322 | 0.5692 | 0.7117 | 0.3348 | 0.6715 | 0.3834 | 0.3269 | 0.4372 | 0.8869 | 0.2662 | 0.4097 | 0.5797 | 0.5587 | 0.4975 |
| 0.5473 | 21500 | 3.489 | 1.9417 | 8.9543 | 7.6468 | 8.3612 | 13.3847 | 3.2314 | 7.9631 | 2.6635 | 8.4673 | 20.5367 | 1.7459 | 6.7037 | 1.0989 | 0.3328 | 0.5741 | 0.7097 | 0.3508 | 0.6660 | 0.3916 | 0.3277 | 0.4391 | 0.8860 | 0.2632 | 0.4100 | 0.5910 | 0.5578 | 0.5000 |
| 0.5536 | 21750 | 3.555 | 1.9533 | 8.9916 | 7.6360 | 8.3586 | 13.3764 | 3.2284 | 7.9575 | 2.7214 | 8.3429 | 20.5891 | 1.7569 | 6.6890 | 1.1157 | 0.3339 | 0.5744 | 0.7114 | 0.3392 | 0.6636 | 0.3964 | 0.3259 | 0.4504 | 0.8925 | 0.2651 | 0.4031 | 0.5792 | 0.5646 | 0.5000 |
| 0.5600 | 22000 | 3.586 | 1.9301 | 8.9806 | 7.6738 | 8.3535 | 13.2453 | 3.2316 | 7.9946 | 2.6907 | 8.2869 | 20.5606 | 1.6573 | 6.8912 | 1.1068 | 0.3239 | 0.5788 | 0.7121 | 0.3537 | 0.6589 | 0.4023 | 0.3243 | 0.4499 | 0.8891 | 0.2644 | 0.4083 | 0.5802 | 0.5616 | 0.5006 |
| 0.5663 | 22250 | 3.5084 | 1.9406 | 9.0040 | 7.6810 | 8.3815 | 13.1792 | 3.2167 | 7.9700 | 2.7316 | 8.6409 | 20.6425 | 1.6145 | 6.8099 | 1.1404 | 0.3174 | 0.5772 | 0.7177 | 0.3519 | 0.6613 | 0.3950 | 0.3324 | 0.4362 | 0.8894 | 0.2648 | 0.4173 | 0.5857 | 0.5626 | 0.5007 |
| 0.5727 | 22500 | 3.5095 | 1.9202 | 8.9619 | 7.6717 | 8.3825 | 13.1359 | 3.2147 | 7.9776 | 2.7114 | 8.2541 | 20.5107 | 1.6855 | 6.9227 | 1.1935 | 0.3335 | 0.5726 | 0.7134 | 0.3574 | 0.6578 | 0.3959 | 0.3262 | 0.4401 | 0.8968 | 0.2604 | 0.4105 | 0.5826 | 0.5620 | 0.5007 |
| 0.5791 | 22750 | 3.5059 | 1.9225 | 8.9956 | 7.6775 | 8.3968 | 13.0329 | 3.2114 | 7.9836 | 2.7107 | 8.4843 | 20.6793 | 1.6821 | 7.0987 | 1.0579 | 0.3273 | 0.5703 | 0.7118 | 0.3689 | 0.6490 | 0.3850 | 0.3257 | 0.4320 | 0.8824 | 0.2622 | 0.4119 | 0.5898 | 0.5605 | 0.4982 |
| 0.5854 | 23000 | 3.4047 | 1.9716 | 9.0017 | 7.6435 | 8.4379 | 13.0467 | 3.1957 | 7.9843 | 2.7017 | 8.5995 | 20.8783 | 1.5818 | 7.1997 | 1.0067 | 0.3272 | 0.5718 | 0.7132 | 0.3728 | 0.6544 | 0.3913 | 0.3255 | 0.4377 | 0.8869 | 0.2583 | 0.4107 | 0.5916 | 0.5539 | 0.4996 |
| 0.5918 | 23250 | 3.4732 | 1.9518 | 8.9827 | 7.6143 | 8.3925 | 13.2800 | 3.1877 | 7.9616 | 2.7122 | 8.5013 | 21.0376 | 1.6291 | 7.0831 | 1.0816 | 0.3316 | 0.5689 | 0.7129 | 0.3705 | 0.6536 | 0.3847 | 0.3211 | 0.4495 | 0.8844 | 0.2622 | 0.4141 | 0.5916 | 0.5551 | 0.5000 |
| 0.5982 | 23500 | 3.4271 | 1.9688 | 9.0092 | 7.5830 | 8.3763 | 13.2765 | 3.1857 | 7.9625 | 2.6794 | 8.4899 | 20.8080 | 1.6519 | 7.1604 | 1.1423 | 0.3346 | 0.5716 | 0.7054 | 0.3637 | 0.6562 | 0.3844 | 0.3249 | 0.4346 | 0.8912 | 0.2577 | 0.4009 | 0.5872 | 0.5697 | 0.4986 |
| 0.6045 | 23750 | 3.4701 | 2.0238 | 9.0036 | 7.5173 | 8.4058 | 13.2881 | 3.1791 | 7.9275 | 2.7149 | 8.8465 | 20.6630 | 1.7025 | 7.2286 | 1.1973 | 0.3308 | 0.5670 | 0.7040 | 0.3754 | 0.6609 | 0.3936 | 0.3252 | 0.4475 | 0.8948 | 0.2542 | 0.4062 | 0.5877 | 0.5717 | 0.5015 |
| 0.6109 | 24000 | 3.6199 | 2.0084 | 8.9869 | 7.5146 | 8.3790 | 13.1350 | 3.1771 | 7.9137 | 2.7032 | 8.5424 | 20.5441 | 1.7652 | 6.8017 | 1.1617 | 0.3224 | 0.5656 | 0.7045 | 0.3643 | 0.6643 | 0.3864 | 0.3174 | 0.4577 | 0.8873 | 0.2571 | 0.3735 | 0.5939 | 0.5611 | 0.4966 |
| 0.6173 | 24250 | 3.408 | 2.0137 | 9.0355 | 7.5305 | 8.3597 | 13.1604 | 3.1735 | 7.9201 | 2.7078 | 9.0787 | 20.5226 | 1.7341 | 6.7525 | 1.0237 | 0.3310 | 0.5676 | 0.7061 | 0.3643 | 0.6602 | 0.3995 | 0.3240 | 0.4588 | 0.8936 | 0.2572 | 0.3868 | 0.5928 | 0.5718 | 0.5011 |
| 0.6236 | 24500 | 3.4651 | 1.9564 | 8.9636 | 7.5635 | 8.3731 | 13.3261 | 3.1737 | 7.9529 | 2.6638 | 8.5231 | 20.4787 | 1.7792 | 6.7317 | 1.0516 | 0.3291 | 0.5670 | 0.7060 | 0.3572 | 0.6594 | 0.3982 | 0.3253 | 0.4400 | 0.8917 | 0.2577 | 0.3886 | 0.5951 | 0.5662 | 0.4986 |
| 0.6300 | 24750 | 3.6988 | 1.9668 | 8.9583 | 7.5875 | 8.4316 | 12.9644 | 3.1769 | 7.9801 | 2.6750 | 8.5780 | 20.3883 | 1.7045 | 6.9010 | 1.0783 | 0.3300 | 0.5668 | 0.7040 | 0.3653 | 0.6550 | 0.3957 | 0.3293 | 0.4570 | 0.8878 | 0.2621 | 0.3816 | 0.5932 | 0.5665 | 0.4996 |
| 0.6363 | 25000 | 3.4365 | 1.9782 | 8.9306 | 7.5829 | 8.4123 | 13.0937 | 3.1640 | 7.9870 | 2.6806 | 8.6281 | 20.2045 | 1.7244 | 6.9685 | 1.0365 | 0.3289 | 0.5651 | 0.6956 | 0.3703 | 0.6494 | 0.4025 | 0.3294 | 0.4511 | 0.8841 | 0.2634 | 0.3894 | 0.5970 | 0.5605 | 0.4990 |
| 0.6427 | 25250 | 3.6097 | 1.9653 | 8.9386 | 7.5722 | 8.4185 | 13.0207 | 3.1629 | 7.9910 | 2.6867 | 8.7326 | 20.2092 | 1.7321 | 6.8303 | 1.0569 | 0.3273 | 0.5643 | 0.7024 | 0.3587 | 0.6534 | 0.4018 | 0.3282 | 0.4401 | 0.8872 | 0.2614 | 0.3860 | 0.5966 | 0.5703 | 0.4983 |
| 0.6491 | 25500 | 3.5379 | 1.9518 | 8.9189 | 7.5241 | 8.4156 | 13.0924 | 3.1557 | 7.9747 | 2.6468 | 8.6005 | 20.3848 | 1.7474 | 6.9006 | 1.0193 | 0.3186 | 0.5692 | 0.7032 | 0.3581 | 0.6429 | 0.4068 | 0.3288 | 0.4426 | 0.8937 | 0.2584 | 0.4055 | 0.5963 | 0.5667 | 0.4993 |
| 0.6554 | 25750 | 3.6223 | 1.9609 | 8.9459 | 7.5554 | 8.4227 | 12.8817 | 3.1483 | 7.9813 | 2.6559 | 8.8423 | 20.3294 | 1.6381 | 6.8535 | 1.0140 | 0.3252 | 0.5722 | 0.7104 | 0.3689 | 0.6554 | 0.4087 | 0.3291 | 0.4293 | 0.8894 | 0.2632 | 0.3940 | 0.5995 | 0.5702 | 0.5012 |
| 0.6618 | 26000 | 3.402 | 1.9602 | 8.9366 | 7.5236 | 8.2409 | 12.9294 | 3.1424 | 7.9619 | 2.6323 | 8.6640 | 20.2959 | 1.6627 | 6.7331 | 1.0115 | 0.3276 | 0.5676 | 0.7031 | 0.3652 | 0.6493 | 0.4030 | 0.3263 | 0.4298 | 0.8830 | 0.2655 | 0.4030 | 0.6017 | 0.5724 | 0.4998 |
| 0.6682 | 26250 | 3.4876 | 1.9929 | 8.9621 | 7.4947 | 8.1977 | 12.8814 | 3.1350 | 7.8663 | 2.6554 | 8.5629 | 20.3156 | 1.7019 | 6.7602 | 0.9582 | 0.3253 | 0.5639 | 0.7127 | 0.3621 | 0.6544 | 0.4146 | 0.3278 | 0.4350 | 0.8895 | 0.2613 | 0.4056 | 0.5997 | 0.5635 | 0.5012 |
| 0.6745 | 26500 | 3.4301 | 1.9752 | 8.9474 | 7.5246 | 8.1379 | 12.7941 | 3.1369 | 7.8999 | 2.6256 | 8.5223 | 20.4417 | 1.6627 | 6.7999 | 0.9626 | 0.3232 | 0.5661 | 0.7094 | 0.3719 | 0.6474 | 0.4077 | 0.3299 | 0.4533 | 0.8849 | 0.2620 | 0.3993 | 0.6008 | 0.5665 | 0.5017 |
| 0.6809 | 26750 | 3.482 | 1.9646 | 8.9353 | 7.5199 | 8.2066 | 12.6512 | 3.1284 | 7.9080 | 2.6464 | 8.6207 | 20.4063 | 1.6852 | 6.7490 | 1.0089 | 0.3172 | 0.5689 | 0.7121 | 0.3734 | 0.6461 | 0.4089 | 0.3287 | 0.4516 | 0.8854 | 0.2601 | 0.3965 | 0.5993 | 0.5634 | 0.5009 |
| 0.6873 | 27000 | 3.5073 | 1.9431 | 8.9478 | 7.5188 | 8.0549 | 12.6486 | 3.1310 | 7.9241 | 2.6508 | 8.5172 | 20.4459 | 1.6855 | 6.8390 | 1.0012 | 0.3222 | 0.5705 | 0.6936 | 0.3675 | 0.6511 | 0.3970 | 0.3286 | 0.4467 | 0.8875 | 0.2587 | 0.3986 | 0.6177 | 0.5690 | 0.5007 |
| 0.6936 | 27250 | 3.5565 | 1.9438 | 8.9610 | 7.5044 | 8.0383 | 12.4879 | 3.1277 | 7.9219 | 2.6321 | 8.4003 | 20.6229 | 1.7421 | 6.7256 | 1.0533 | 0.3327 | 0.5737 | 0.6955 | 0.3653 | 0.6486 | 0.4067 | 0.3277 | 0.4368 | 0.8901 | 0.2589 | 0.3877 | 0.6135 | 0.5696 | 0.5005 |
| 0.7000 | 27500 | 3.4506 | 1.9639 | 8.9673 | 7.4644 | 8.1455 | 12.4523 | 3.1171 | 7.9159 | 2.6772 | 8.5339 | 20.7734 | 1.7690 | 6.6677 | 1.0708 | 0.3260 | 0.5730 | 0.6960 | 0.3562 | 0.6419 | 0.4053 | 0.3310 | 0.4395 | 0.8871 | 0.2594 | 0.4010 | 0.6155 | 0.5679 | 0.5000 |
| 0.7063 | 27750 | 3.4875 | 1.9177 | 8.9276 | 7.4754 | 8.1375 | 12.5163 | 3.1315 | 7.8697 | 2.6247 | 8.4426 | 20.4950 | 1.7047 | 6.6303 | 1.0030 | 0.3244 | 0.5690 | 0.6887 | 0.3499 | 0.6471 | 0.4057 | 0.3309 | 0.4464 | 0.8893 | 0.2570 | 0.4038 | 0.6038 | 0.5701 | 0.4989 |
| 0.7127 | 28000 | 3.5298 | 1.8939 | 8.9022 | 7.4889 | 8.1322 | 12.5461 | 3.1409 | 7.8621 | 2.5963 | 8.4173 | 20.4948 | 1.7078 | 6.4516 | 0.9874 | 0.3380 | 0.5721 | 0.6934 | 0.3631 | 0.6439 | 0.4046 | 0.3272 | 0.4486 | 0.8893 | 0.2593 | 0.4020 | 0.6017 | 0.5687 | 0.5009 |
| 0.7191 | 28250 | 3.3329 | 1.8970 | 8.9172 | 7.5001 | 8.1758 | 12.5515 | 3.1333 | 7.9075 | 2.5928 | 8.5025 | 20.3447 | 1.6879 | 6.5795 | 0.9658 | 0.3247 | 0.5729 | 0.7000 | 0.3710 | 0.6468 | 0.4069 | 0.3336 | 0.4564 | 0.8933 | 0.2637 | 0.4105 | 0.6012 | 0.5749 | 0.5043 |
| 0.7254 | 28500 | 3.3897 | 1.8966 | 8.9038 | 7.5265 | 8.2199 | 12.6173 | 3.1244 | 7.8882 | 2.5974 | 8.3728 | 20.3628 | 1.6804 | 6.6887 | 0.9733 | 0.3278 | 0.5750 | 0.7001 | 0.3645 | 0.6528 | 0.4145 | 0.3310 | 0.4669 | 0.8964 | 0.2638 | 0.4032 | 0.6086 | 0.5713 | 0.5058 |
| 0.7318 | 28750 | 3.4588 | 1.8934 | 8.8948 | 7.5026 | 8.2374 | 12.4866 | 3.1287 | 7.8941 | 2.5811 | 8.3781 | 20.4334 | 1.7109 | 6.6195 | 0.9699 | 0.3251 | 0.5707 | 0.7083 | 0.3702 | 0.6411 | 0.4086 | 0.3252 | 0.4553 | 0.8935 | 0.2641 | 0.4068 | 0.6063 | 0.5592 | 0.5026 |
| 0.7382 | 29000 | 3.3675 | 1.8959 | 8.8925 | 7.5043 | 8.2628 | 12.6063 | 3.1174 | 7.9132 | 2.5908 | 8.2436 | 20.3771 | 1.6740 | 6.7151 | 0.9895 | 0.3262 | 0.5725 | 0.7069 | 0.3694 | 0.6495 | 0.4063 | 0.3169 | 0.4622 | 0.8962 | 0.2609 | 0.4124 | 0.6101 | 0.5635 | 0.5041 |
| 0.7445 | 29250 | 3.3886 | 1.8976 | 8.9215 | 7.4975 | 8.2401 | 12.4978 | 3.1127 | 7.8615 | 2.5814 | 8.2715 | 20.4379 | 1.6740 | 6.7705 | 0.9492 | 0.3269 | 0.5683 | 0.7061 | 0.3760 | 0.6554 | 0.4089 | 0.3209 | 0.4508 | 0.8982 | 0.2658 | 0.3982 | 0.6053 | 0.5668 | 0.5037 |
| 0.7509 | 29500 | 3.4826 | 1.8855 | 8.9320 | 7.5047 | 8.2470 | 12.5056 | 3.1168 | 7.8967 | 2.5742 | 8.3762 | 20.4917 | 1.7122 | 6.3650 | 0.9882 | 0.3380 | 0.5681 | 0.7061 | 0.3727 | 0.6521 | 0.4071 | 0.3222 | 0.4771 | 0.8963 | 0.2701 | 0.3943 | 0.6010 | 0.5624 | 0.5052 |
| 0.7572 | 29750 | 3.4268 | 1.8725 | 8.9332 | 7.5158 | 8.2481 | 12.4708 | 3.1146 | 7.9010 | 2.5617 | 8.2478 | 20.3435 | 1.6860 | 6.4246 | 0.9801 | 0.3331 | 0.5691 | 0.7061 | 0.3767 | 0.6523 | 0.4068 | 0.3195 | 0.4589 | 0.9097 | 0.2627 | 0.4113 | 0.6059 | 0.5641 | 0.5059 |
| 0.7636 | 30000 | 3.2621 | 1.8813 | 8.9125 | 7.5203 | 8.2736 | 12.5555 | 3.1094 | 7.9083 | 2.5488 | 8.3690 | 20.3392 | 1.7015 | 6.5219 | 0.9901 | 0.3366 | 0.5686 | 0.6941 | 0.3696 | 0.6465 | 0.4062 | 0.3228 | 0.4570 | 0.8967 | 0.2657 | 0.3975 | 0.6054 | 0.5702 | 0.5028 |
| 0.7700 | 30250 | 3.3289 | 1.8893 | 8.9211 | 7.5322 | 8.1753 | 12.4890 | 3.1063 | 7.9272 | 2.5320 | 8.4628 | 20.3169 | 1.6841 | 6.5986 | 0.9798 | 0.3302 | 0.5719 | 0.6944 | 0.3745 | 0.6471 | 0.4007 | 0.3172 | 0.4741 | 0.9046 | 0.2685 | 0.4126 | 0.6014 | 0.5666 | 0.5049 |
| 0.7763 | 30500 | 3.5363 | 1.8836 | 8.8909 | 7.5323 | 8.2197 | 12.4000 | 3.1064 | 7.9088 | 2.5494 | 8.3271 | 20.3110 | 1.7132 | 6.4502 | 0.9974 | 0.3298 | 0.5705 | 0.6995 | 0.3671 | 0.6511 | 0.4057 | 0.3204 | 0.4560 | 0.8949 | 0.2613 | 0.4153 | 0.6011 | 0.5738 | 0.5036 |
| 0.7827 | 30750 | 3.3557 | 1.8824 | 8.9002 | 7.5249 | 8.2047 | 12.4618 | 3.1055 | 7.9265 | 2.5501 | 8.2708 | 20.2254 | 1.7222 | 6.4927 | 0.9813 | 0.3333 | 0.5678 | 0.6926 | 0.3781 | 0.6575 | 0.4005 | 0.3225 | 0.4497 | 0.8991 | 0.2649 | 0.4018 | 0.6051 | 0.5698 | 0.5033 |
| 0.7891 | 31000 | 3.4481 | 1.8725 | 8.9077 | 7.5043 | 8.2095 | 12.5197 | 3.1095 | 7.9124 | 2.5216 | 8.1396 | 20.0618 | 1.6962 | 6.4808 | 0.9764 | 0.3321 | 0.5691 | 0.6941 | 0.3650 | 0.6464 | 0.4013 | 0.3239 | 0.4522 | 0.9035 | 0.2657 | 0.4058 | 0.6041 | 0.5639 | 0.5021 |
| 0.7954 | 31250 | 3.3888 | 1.8596 | 8.9249 | 7.5248 | 8.2429 | 12.4622 | 3.1054 | 7.9247 | 2.5351 | 8.3011 | 19.9880 | 1.7062 | 6.5598 | 0.9628 | 0.3428 | 0.5694 | 0.7008 | 0.3761 | 0.6564 | 0.4013 | 0.3223 | 0.4589 | 0.9024 | 0.2633 | 0.4111 | 0.6052 | 0.5670 | 0.5059 |
| 0.8018 | 31500 | 3.3989 | 1.8662 | 8.9299 | 7.5206 | 8.1957 | 12.4667 | 3.1043 | 7.9089 | 2.5465 | 8.2149 | 19.8897 | 1.6830 | 6.6131 | 0.9204 | 0.3452 | 0.5658 | 0.7002 | 0.3709 | 0.6570 | 0.4004 | 0.3233 | 0.4588 | 0.9015 | 0.2619 | 0.4044 | 0.6015 | 0.5654 | 0.5043 |
| 0.8082 | 31750 | 3.3603 | 1.8671 | 8.9158 | 7.5135 | 8.1945 | 12.5201 | 3.1009 | 7.9130 | 2.5539 | 8.3876 | 19.9818 | 1.6685 | 6.6359 | 0.9307 | 0.3516 | 0.5659 | 0.6928 | 0.3767 | 0.6534 | 0.4004 | 0.3245 | 0.4627 | 0.9021 | 0.2619 | 0.4252 | 0.6019 | 0.5699 | 0.5068 |
| 0.8145 | 32000 | 3.3783 | 1.8588 | 8.8993 | 7.4994 | 8.2058 | 12.5200 | 3.0980 | 7.8902 | 2.5448 | 8.3444 | 19.9960 | 1.6815 | 6.7156 | 0.9509 | 0.3358 | 0.5665 | 0.7012 | 0.3759 | 0.6551 | 0.4002 | 0.3259 | 0.4505 | 0.8960 | 0.2621 | 0.4113 | 0.6059 | 0.5716 | 0.5045 |
| 0.8209 | 32250 | 3.4379 | 1.8693 | 8.8896 | 7.4639 | 8.0939 | 12.5840 | 3.0894 | 7.8559 | 2.5590 | 8.4227 | 19.9565 | 1.6855 | 6.6835 | 0.9650 | 0.3433 | 0.5663 | 0.7004 | 0.3717 | 0.6527 | 0.4076 | 0.3255 | 0.4491 | 0.8990 | 0.2643 | 0.4127 | 0.5984 | 0.5741 | 0.5050 |
| 0.8272 | 32500 | 3.4962 | 1.8838 | 8.8634 | 7.4636 | 8.1354 | 12.5144 | 3.0866 | 7.8561 | 2.5410 | 8.4169 | 20.0794 | 1.6625 | 6.6897 | 0.9849 | 0.3461 | 0.5678 | 0.7024 | 0.3808 | 0.6564 | 0.4083 | 0.3200 | 0.4531 | 0.9015 | 0.2622 | 0.4116 | 0.6061 | 0.5685 | 0.5065 |
| 0.8336 | 32750 | 3.4927 | 1.8696 | 8.8655 | 7.4274 | 8.0888 | 12.5431 | 3.0874 | 7.8320 | 2.5324 | 8.3181 | 20.0095 | 1.6403 | 6.7182 | 0.9837 | 0.3439 | 0.5657 | 0.7078 | 0.3771 | 0.6580 | 0.4078 | 0.3210 | 0.4543 | 0.9087 | 0.2625 | 0.4184 | 0.6095 | 0.5691 | 0.5080 |
| 0.8400 | 33000 | 3.33 | 1.8743 | 8.8776 | 7.4438 | 8.1127 | 12.5571 | 3.0793 | 7.8474 | 2.5423 | 8.3195 | 20.1064 | 1.6546 | 6.7761 | 1.0118 | 0.3400 | 0.5684 | 0.6938 | 0.3760 | 0.6560 | 0.4077 | 0.3248 | 0.4586 | 0.8965 | 0.2613 | 0.4085 | 0.6094 | 0.5704 | 0.5055 |
| 0.8463 | 33250 | 3.3431 | 1.8687 | 8.8074 | 7.4577 | 8.1313 | 12.5392 | 3.0816 | 7.8629 | 2.5306 | 8.3183 | 20.1216 | 1.6653 | 6.8390 | 1.0199 | 0.3233 | 0.5685 | 0.7031 | 0.3770 | 0.6493 | 0.4075 | 0.3243 | 0.4586 | 0.9015 | 0.2600 | 0.4068 | 0.6050 | 0.5659 | 0.5039 |
| 0.8527 | 33500 | 3.4455 | 1.8618 | 8.8108 | 7.4587 | 8.1421 | 12.5672 | 3.0800 | 7.8533 | 2.5364 | 8.2589 | 20.0766 | 1.6537 | 6.8825 | 1.0161 | 0.3346 | 0.5647 | 0.7003 | 0.3761 | 0.6523 | 0.4075 | 0.3239 | 0.4598 | 0.9088 | 0.2641 | 0.4134 | 0.5976 | 0.5702 | 0.5056 |
| 0.8591 | 33750 | 3.3189 | 1.8578 | 8.8046 | 7.4684 | 8.1488 | 12.6076 | 3.0817 | 7.8637 | 2.5249 | 8.2008 | 20.1733 | 1.6486 | 6.9228 | 1.0004 | 0.3428 | 0.5684 | 0.7019 | 0.3782 | 0.6483 | 0.4049 | 0.3229 | 0.4525 | 0.9039 | 0.2641 | 0.4088 | 0.6114 | 0.5669 | 0.5058 |
| 0.8654 | 34000 | 3.3815 | 1.8587 | 8.7921 | 7.4675 | 8.0968 | 12.6359 | 3.0800 | 7.8680 | 2.5266 | 8.3492 | 20.1037 | 1.6352 | 6.8485 | 1.0061 | 0.3412 | 0.5655 | 0.6945 | 0.3806 | 0.6510 | 0.4061 | 0.3178 | 0.4583 | 0.9085 | 0.2640 | 0.4078 | 0.6105 | 0.5689 | 0.5058 |
| 0.8718 | 34250 | 3.3381 | 1.8586 | 8.7828 | 7.4672 | 8.1115 | 12.6341 | 3.0783 | 7.8743 | 2.5275 | 8.3363 | 20.0616 | 1.6445 | 6.8898 | 1.0146 | 0.3255 | 0.5645 | 0.6941 | 0.3784 | 0.6488 | 0.4027 | 0.3190 | 0.4518 | 0.9039 | 0.2637 | 0.4079 | 0.6115 | 0.5730 | 0.5034 |
| 0.8782 | 34500 | 3.3992 | 1.8597 | 8.7906 | 7.4658 | 8.1316 | 12.6647 | 3.0781 | 7.8643 | 2.5249 | 8.3280 | 20.0237 | 1.6348 | 6.8277 | 1.0203 | 0.3283 | 0.5645 | 0.6925 | 0.3744 | 0.6525 | 0.4027 | 0.3250 | 0.4593 | 0.9040 | 0.2625 | 0.4097 | 0.6121 | 0.5676 | 0.5042 |
| 0.8845 | 34750 | 3.2951 | 1.8608 | 8.8033 | 7.4729 | 8.1063 | 12.6896 | 3.0719 | 7.8671 | 2.5243 | 8.3368 | 20.0587 | 1.6455 | 6.7907 | 1.0106 | 0.3274 | 0.5651 | 0.6925 | 0.3738 | 0.6527 | 0.4045 | 0.3219 | 0.4592 | 0.9041 | 0.2619 | 0.3985 | 0.6120 | 0.5679 | 0.5032 |
| 0.8909 | 35000 | 3.262 | 1.8677 | 8.8024 | 7.4317 | 8.1170 | 12.7225 | 3.0671 | 7.8519 | 2.5303 | 8.4655 | 20.1156 | 1.6405 | 6.7997 | 1.0141 | 0.3288 | 0.5667 | 0.6935 | 0.3738 | 0.6554 | 0.4049 | 0.3196 | 0.4532 | 0.9041 | 0.2626 | 0.4116 | 0.6123 | 0.5653 | 0.5040 |
| 0.8972 | 35250 | 3.3218 | 1.8698 | 8.7992 | 7.4495 | 8.1390 | 12.7198 | 3.0627 | 7.8528 | 2.5399 | 8.4549 | 20.1652 | 1.6288 | 6.7857 | 1.0261 | 0.3328 | 0.5666 | 0.6951 | 0.3686 | 0.6538 | 0.4043 | 0.3248 | 0.4596 | 0.9042 | 0.2646 | 0.4097 | 0.6123 | 0.5725 | 0.5053 |
| 0.9036 | 35500 | 3.3529 | 1.8633 | 8.7964 | 7.4339 | 8.1347 | 12.7295 | 3.0657 | 7.8262 | 2.5256 | 8.4119 | 20.1363 | 1.6165 | 6.7987 | 1.0260 | 0.3268 | 0.5660 | 0.6951 | 0.3695 | 0.6543 | 0.4042 | 0.3232 | 0.4511 | 0.9068 | 0.2666 | 0.4047 | 0.6112 | 0.5701 | 0.5038 |
| 0.9100 | 35750 | 3.2205 | 1.8626 | 8.7751 | 7.4335 | 8.1346 | 12.7532 | 3.0654 | 7.8318 | 2.5077 | 8.3883 | 20.0738 | 1.5839 | 6.7819 | 1.0282 | 0.3291 | 0.5659 | 0.6951 | 0.3702 | 0.6530 | 0.4045 | 0.3241 | 0.4493 | 0.9067 | 0.2622 | 0.4192 | 0.6112 | 0.5710 | 0.5047 |
| 0.9163 | 36000 | 3.3671 | 1.8521 | 8.7693 | 7.4500 | 8.1155 | 12.8041 | 3.0671 | 7.8451 | 2.4994 | 8.3053 | 20.0666 | 1.5984 | 6.7696 | 1.0377 | 0.3383 | 0.5667 | 0.6946 | 0.3739 | 0.6536 | 0.4049 | 0.3262 | 0.4457 | 0.9065 | 0.2625 | 0.4143 | 0.6112 | 0.5719 | 0.5054 |
| 0.9227 | 36250 | 3.4074 | 1.8555 | 8.7683 | 7.4607 | 8.1465 | 12.7545 | 3.0621 | 7.8488 | 2.5080 | 8.3816 | 20.0420 | 1.6043 | 6.7991 | 1.0539 | 0.3367 | 0.5685 | 0.6935 | 0.3714 | 0.6523 | 0.4068 | 0.3237 | 0.4471 | 0.9065 | 0.2645 | 0.4176 | 0.6110 | 0.5692 | 0.5053 |
| 0.9291 | 36500 | 3.4806 | 1.8543 | 8.7833 | 7.4584 | 8.1070 | 12.7444 | 3.0605 | 7.8475 | 2.5166 | 8.3809 | 19.3340 | 1.6181 | 6.7663 | 1.0555 | 0.3271 | 0.5693 | 0.6935 | 0.3733 | 0.6560 | 0.3990 | 0.3229 | 0.4530 | 0.9065 | 0.2618 | 0.4105 | 0.6129 | 0.5654 | 0.5039 |
| 0.9354 | 36750 | 3.4848 | 1.8558 | 8.7967 | 7.4512 | 8.1175 | 12.6960 | 3.0616 | 7.8420 | 2.5137 | 8.4267 | 19.4008 | 1.6181 | 6.7376 | 1.0629 | 0.3284 | 0.5697 | 0.6922 | 0.3723 | 0.6530 | 0.4048 | 0.3234 | 0.4534 | 0.9065 | 0.2641 | 0.4088 | 0.6115 | 0.5635 | 0.5040 |
| 0.9418 | 37000 | 3.1947 | 1.8574 | 8.8026 | 7.4555 | 8.1222 | 12.7155 | 3.0591 | 7.8391 | 2.5180 | 8.4551 | 19.4094 | 1.6134 | 6.7200 | 1.0657 | 0.3318 | 0.5681 | 0.6922 | 0.3711 | 0.6550 | 0.4043 | 0.3234 | 0.4534 | 0.9037 | 0.2645 | 0.4090 | 0.6113 | 0.5685 | 0.5043 |
| 0.9482 | 37250 | 3.3557 | 1.8569 | 8.7972 | 7.4535 | 8.1200 | 12.7427 | 3.0585 | 7.8460 | 2.5167 | 8.5049 | 19.4395 | 1.6141 | 6.7080 | 1.0700 | 0.3308 | 0.5699 | 0.6930 | 0.3708 | 0.6513 | 0.4048 | 0.3247 | 0.4589 | 0.9039 | 0.2645 | 0.4087 | 0.6113 | 0.5677 | 0.5046 |
| 0.9545 | 37500 | 3.369 | 1.8580 | 8.7958 | 7.4558 | 8.1193 | 12.7407 | 3.0579 | 7.8509 | 2.5139 | 8.5231 | 19.4691 | 1.6139 | 6.7228 | 1.0696 | 0.3388 | 0.5683 | 0.6922 | 0.3696 | 0.6556 | 0.4050 | 0.3236 | 0.4460 | 0.8965 | 0.2624 | 0.4145 | 0.6113 | 0.5682 | 0.5040 |
| 0.9609 | 37750 | 3.398 | 1.8564 | 8.7955 | 7.4567 | 8.1174 | 12.7478 | 3.0589 | 7.8415 | 2.5086 | 8.5030 | 19.4719 | 1.5989 | 6.7117 | 1.0689 | 0.3381 | 0.5678 | 0.6922 | 0.3652 | 0.6544 | 0.4049 | 0.3234 | 0.4460 | 0.9039 | 0.2599 | 0.3998 | 0.6113 | 0.5658 | 0.5025 |
| 0.9672 | 38000 | 3.3699 | 1.8534 | 8.8011 | 7.4604 | 8.1135 | 12.7689 | 3.0574 | 7.8412 | 2.5097 | 8.4866 | 19.4973 | 1.5966 | 6.7309 | 1.0655 | 0.3372 | 0.5680 | 0.6922 | 0.3651 | 0.6553 | 0.4038 | 0.3224 | 0.4560 | 0.9025 | 0.2675 | 0.4061 | 0.6111 | 0.5712 | 0.5045 |
| 0.9736 | 38250 | 3.4483 | 1.8540 | 8.8045 | 7.4506 | 8.0942 | 12.7725 | 3.0569 | 7.8379 | 2.5135 | 8.4817 | 19.5038 | 1.5995 | 6.7289 | 1.0693 | 0.3378 | 0.5682 | 0.6922 | 0.3724 | 0.6550 | 0.4038 | 0.3243 | 0.4534 | 0.9025 | 0.2645 | 0.4058 | 0.6111 | 0.5710 | 0.5048 |
| 0.9800 | 38500 | 3.254 | 1.8546 | 8.8026 | 7.4530 | 8.1004 | 12.7796 | 3.0559 | 7.8378 | 2.5086 | 8.4538 | 19.5061 | 1.5997 | 6.7390 | 1.0724 | 0.3381 | 0.5681 | 0.6922 | 0.3647 | 0.6547 | 0.4041 | 0.3242 | 0.4534 | 0.8951 | 0.2642 | 0.4064 | 0.6111 | 0.5677 | 0.5034 |
| 0.9863 | 38750 | 3.2759 | 1.8549 | 8.8024 | 7.4545 | 8.0996 | 12.7875 | 3.0562 | 7.8400 | 2.5054 | 8.4401 | 19.5192 | 1.6018 | 6.7433 | 1.0737 | 0.3382 | 0.5681 | 0.6922 | 0.3647 | 0.6547 | 0.4041 | 0.3241 | 0.4534 | 0.8951 | 0.2642 | 0.4078 | 0.6111 | 0.5689 | 0.5036 |
| 0.9927 | 39000 | 3.3273 | 1.8548 | 8.8017 | 7.4536 | 8.0908 | 12.7885 | 3.0557 | 7.8396 | 2.5052 | 8.4335 | 19.5169 | 1.6002 | 6.7439 | 1.0716 | 0.3382 | 0.5681 | 0.6922 | 0.3647 | 0.6547 | 0.4041 | 0.3242 | 0.4534 | 0.8951 | 0.2641 | 0.4078 | 0.6111 | 0.5703 | 0.5037 |
| 0.9991 | 39250 | 3.3902 | 1.8540 | 8.8010 | 7.4543 | 8.0872 | 12.7890 | 3.0561 | 7.8412 | 2.5040 | 8.3945 | 19.5165 | 1.5997 | 6.7462 | 1.0718 | 0.3309 | 0.5681 | 0.6922 | 0.3651 | 0.6547 | 0.4041 | 0.3242 | 0.4534 | 0.8951 | 0.2643 | 0.4078 | 0.6111 | 0.5703 | 0.5032 |
| 1.0 | 39287 | - | - | - | - | - | - | - | - | - | - | - | - | - | - | 0.3309 | 0.5681 | 0.6922 | 0.3651 | 0.6547 | 0.4041 | 0.3242 | 0.4534 | 0.8951 | 0.2643 | 0.4078 | 0.6111 | 0.5703 | 0.5032 |
### Environmental Impact
Carbon emissions were measured using [CodeCarbon](https://github.com/mlco2/codecarbon).
- **Energy Consumed**: 2.611 kWh
- **Carbon Emitted**: 1.015 kg of CO2
- **Hours Used**: 17.883 hours
### Training Hardware
- **On Cloud**: No
- **GPU Model**: 1 x NVIDIA GeForce RTX 3090
- **CPU Model**: 13th Gen Intel(R) Core(TM) i7-13700K
- **RAM Size**: 31.78 GB
### Framework Versions
- Python: 3.11.6
- Sentence Transformers: 3.3.0.dev0
- Transformers: 4.45.2
- PyTorch: 2.5.0.dev20240807+cu121
- Accelerate: 1.0.0
- Datasets: 2.20.0
- Tokenizers: 0.20.1-dev.0
## Citation
### BibTeX
#### Sentence Transformers
```bibtex
@inproceedings{reimers-2019-sentence-bert,
title = "Sentence-BERT: Sentence Embeddings using Siamese BERT-Networks",
author = "Reimers, Nils and Gurevych, Iryna",
booktitle = "Proceedings of the 2019 Conference on Empirical Methods in Natural Language Processing",
month = "11",
year = "2019",
publisher = "Association for Computational Linguistics",
url = "https://arxiv.org/abs/1908.10084",
}
```
#### MatryoshkaLoss
```bibtex
@misc{kusupati2024matryoshka,
title={Matryoshka Representation Learning},
author={Aditya Kusupati and Gantavya Bhatt and Aniket Rege and Matthew Wallingford and Aditya Sinha and Vivek Ramanujan and William Howard-Snyder and Kaifeng Chen and Sham Kakade and Prateek Jain and Ali Farhadi},
year={2024},
eprint={2205.13147},
archivePrefix={arXiv},
primaryClass={cs.LG}
}
```
#### MultipleNegativesRankingLoss
```bibtex
@misc{henderson2017efficient,
title={Efficient Natural Language Response Suggestion for Smart Reply},
author={Matthew Henderson and Rami Al-Rfou and Brian Strope and Yun-hsuan Sung and Laszlo Lukacs and Ruiqi Guo and Sanjiv Kumar and Balint Miklos and Ray Kurzweil},
year={2017},
eprint={1705.00652},
archivePrefix={arXiv},
primaryClass={cs.CL}
}
```