File size: 25,685 Bytes
84745c1 f8571a5 84745c1 f8571a5 84745c1 f8571a5 84745c1 f8571a5 84745c1 b2ca582 84745c1 b2ca582 84745c1 b2ca582 612092f b2ca582 612092f b2ca582 612092f b2ca582 612092f b2ca582 84745c1 b2ca582 |
1 2 3 4 5 6 7 8 9 10 11 12 13 14 15 16 17 18 19 20 21 22 23 24 25 26 27 28 29 30 31 32 33 34 35 36 37 38 39 40 41 42 43 44 45 46 47 48 49 50 51 52 53 54 55 56 57 58 59 60 61 62 63 64 65 66 67 68 69 70 71 72 73 74 75 76 77 78 79 80 81 82 83 84 85 86 87 88 89 90 91 92 93 94 95 96 97 98 99 100 101 102 103 104 105 106 107 108 109 110 111 112 113 114 115 116 117 118 119 120 121 122 123 124 125 126 127 128 129 130 131 132 133 134 135 136 137 138 139 140 141 142 143 144 145 146 147 148 149 150 151 152 153 154 155 156 157 158 159 160 161 162 163 164 165 166 167 168 169 170 171 172 173 174 175 176 177 178 179 180 181 182 183 184 185 186 187 188 189 190 191 192 193 194 195 196 197 198 199 200 201 202 203 204 205 206 207 208 209 210 211 212 213 214 215 216 217 218 219 220 221 222 223 224 225 226 227 228 229 230 231 232 233 234 235 236 237 238 239 240 241 242 243 244 245 246 247 248 249 250 251 252 253 254 255 256 257 258 259 260 261 262 263 264 265 266 267 268 269 270 271 272 273 274 275 276 277 278 279 280 281 282 283 284 285 286 287 288 289 290 291 292 293 294 295 296 297 298 299 300 301 302 303 304 305 306 307 308 309 310 311 312 313 314 315 316 317 318 319 320 321 322 323 324 325 326 327 328 329 330 331 332 333 334 335 336 337 338 339 340 341 342 343 344 345 346 347 348 349 350 351 352 353 354 355 356 357 358 359 360 361 362 363 364 365 366 367 368 369 370 371 372 373 374 375 376 377 378 379 380 381 382 383 384 385 386 387 388 389 390 391 392 393 394 395 396 397 398 399 400 401 402 403 404 405 406 407 408 409 410 411 412 413 414 415 416 417 418 419 420 421 422 423 424 425 426 427 428 429 430 431 432 433 434 435 436 437 438 439 440 441 442 443 444 445 446 447 448 449 450 451 452 453 454 455 456 457 458 |
---
tags:
- image-to-text
- image-captioning
- Transformers
- vision-encoder-decoder
license: apache-2.0
widget:
- src: >-
https://huggingface.co./datasets/mishig/sample_images/resolve/main/savanna.jpg
example_title: Savanna
- src: >-
https://huggingface.co./datasets/mishig/sample_images/resolve/main/football-match.jpg
example_title: Football Match
- src: >-
https://huggingface.co./datasets/mishig/sample_images/resolve/main/airport.jpg
example_title: Airport
---
# The Illustrated Image Captioning using transformers model
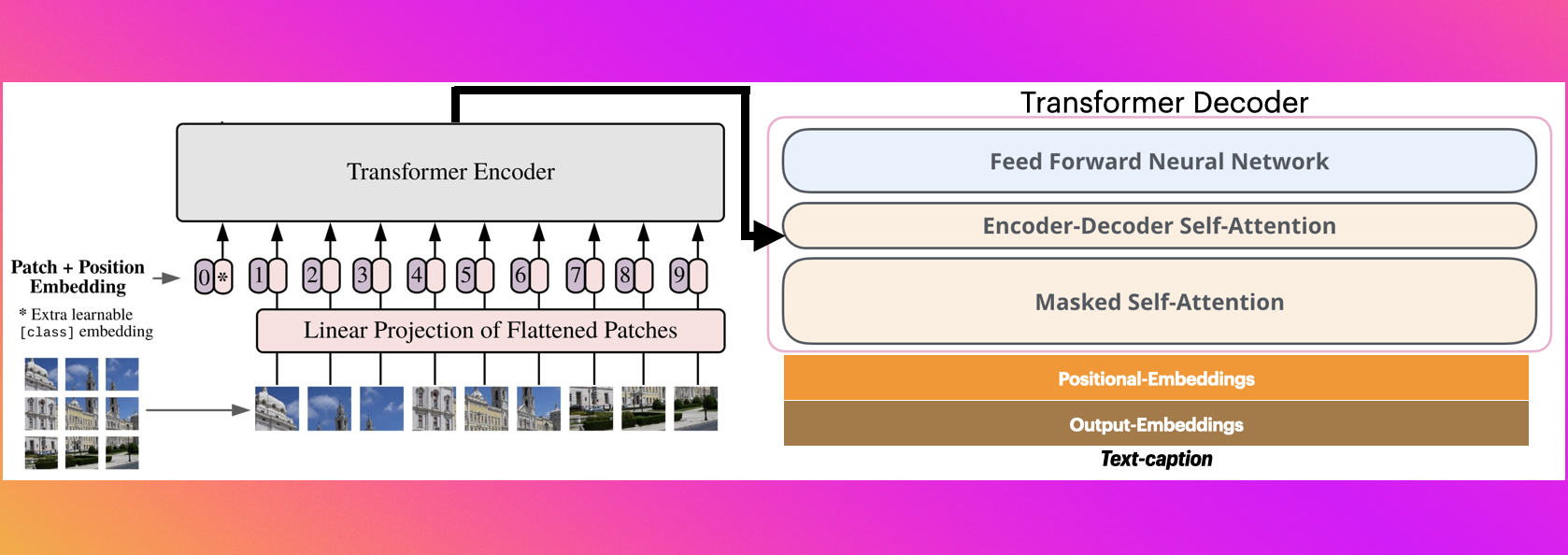
# Table of Contents
- [1. Introduction](#1-introduction)
- [2. Dataset Used](#2-dataset-used)
- [3. Installation](#3-installation)
- [4. Models and Technologies Used](#4-models-and-technologies-used)
- [5. Steps for Code Explanation](#5-steps-for-code-explanation)
- [6. Results and Analysis](#6-results-and-analysis)
- [7. Evaluation Metrics](#7-evaluation-metrics)
- [8. References](#8-references)
## 1. Introduction
This repository, Image captioning is a challenging problem that involves generating human-like descriptions for images. By utilizing Vision Transformers, this project aims to achieve improved image understanding and caption generation. The combination of computer vision and Transformers has shown promising results in various natural language processing tasks, and this project explores their application to image captioning.
## 2. Dataset Used
### About MS COCO dataset
The Microsoft **C**ommon **O**bjects in **CO**ntext (MS COCO) dataset is a large-scale dataset for scene understanding. The dataset is commonly used to train and benchmark object detection, segmentation, and captioning algorithms.

You can read more about the dataset on the [website](http://cocodataset.org/#home), [research paper](https://arxiv.org/pdf/1405.0312.pdf), or Appendix section at the end of this page.
## 3. Installation
### Install COCO API
1. Clone this repo: https://github.com/cocodataset/cocoapi
```
git clone https://github.com/cocodataset/cocoapi.git
```
2. Setup the coco API (also described in the readme [here](https://github.com/cocodataset/cocoapi))
```
cd cocoapi/PythonAPI
make
cd ..
```
3. Download some specific data from here: http://cocodataset.org/#download (described below)
* Under **Annotations**, download:
* **2017 Train/Val annotations [241MB]** (extract captions_train2017.json and captions_val2017.json, and place at locations cocoapi/annotations/captions_train2017.json and cocoapi/annotations/captions_val2017.json, respectively)
* **2017 Testing Image info [1MB]** (extract image_info_test2017.json and place at location cocoapi/annotations/image_info_test2017.json)
* Under **Images**, download:
* **2017 Train images [83K/13GB]** (extract the train2017 folder and place at location cocoapi/images/train2017/)
* **2017 Val images [41K/6GB]** (extract the val2017 folder and place at location cocoapi/images/val2017/)
* **2017 Test images [41K/6GB]** (extract the test2017 folder and place at location cocoapi/images/test2017/)
## 3. Installation
## Preparing the environment
**Note**: I have developed this project on Mac. It can surely be run on Windows and linux with some little changes.
1. Clone the repository, and navigate to the downloaded folder.
```
git clone https://github.com/CapstoneProjectimagecaptioning/image_captioning_transformer.git
cd image_captioning_transformer
```
2. Create (and activate) a new environment, named `captioning_env` with Python 3.7. If prompted to proceed with the install `(Proceed [y]/n)` type y.
```shell
conda create -n captioning_env python=3.7
source activate captioning_env
```
At this point your command line should look something like: `(captioning_env) <User>:image_captioning <user>$`. The `(captioning_env)` indicates that your environment has been activated, and you can proceed with further package installations.
6. Before you can experiment with the code, you'll have to make sure that you have all the libraries and dependencies required to support this project. You will mainly need Python3.7+, PyTorch and its torchvision, OpenCV, and Matplotlib. You can install dependencies using:
```
pip install -r requirements.txt
```
7. Navigate back to the repo. (Also, your source environment should still be activated at this point.)
```shell
cd image_captioning
```
8. Open the directory of notebooks, using the below command. You'll see all of the project files appear in your local environment; open the first notebook and follow the instructions.
```shell
jupyter notebook
```
9. Once you open any of the project notebooks, make sure you are in the correct `captioning_env` environment by clicking `Kernel > Change Kernel > captioning_env`.
## 4. Models and Technologies Used
### The following methods and techniques are employed in this project:
- Vision Transformers (ViTs)
- Attention mechanisms
- Language modeling
- Transfer learning
- Evaluation metrics for image captioning (e.g., BLEU, METEOR, CIDEr)
### The project is implemented in Python and utilizes the following libraries:
- PyTorch
- Transformers
- TorchVision
- NumPy
- NLTK
- Matplotlib
### Introduction
This project uses a transformer [[3]](#3) based model to generate a description
for images. This task is known as the Image Captioning task. Researchers used
many methodologies to approach this problem. One of these methodologies is the
encoder-decoder neural network [4]. The encoder transforms the source image
into a representation space; then, the decoder translates the information from
the encoded space into a natural language. The goal of the encoder-decoder is
to minimize the loss of generating a description from an image.
As shown in the survey done by MD Zakir Hossain et al. [[4]](#4), we can see that the
models that use encoder-decoder architecture mainly consist of a language model
based on LSTM [[5]](#5), which decodes the encoded image received from a CNN, see
Figure 1. The limitation of LSTM with long sequences and the success of
transformers in machine translation and other NLP tasks attracts attention to
utilizing it in machine vision. Alexey Dosovitskiy et al. introduce an image
classification model (ViT) based on a classical transformer encoder showing a
good performance [[6]](#6). Based on ViT, Wei Liu et al. present an image captioning
model (CPTR) using an encoder-decoder transformer [[1]](#1). The source image is fed
to the transformer encoder in sequence patches. Hence, one can treat the image
captioning problem as a machine translation task.

Figure 1: Encoder Decoder Architecture
### Framework
The CPTR [[1]](#1) consists of an image patcher that converts images

to a sequence of patches }),
where _N_ is number of patches, _H_, _W_, _C_ are images height, width and
number of chanel _C=3_ respectively, _P_ is patch resolution, and _E_ is image
embeddings size. Position embeddings are then added to the images patches,
which form the input to twelve layers of identical transformer encoders. The
output of the last encoder layer goes to four layers of identical transformer
decoders. The decoder also takes words with sinusoid positional embedding.
The pre-trained ViT weights initialize the CPTR encoder [[1]](#1). I omitted
the initialization and image positional embeddings, adding an image embedding
module to the image patcher using the features map extracted from the Resnet101
network [[7]](#7). The number of encoder layers is reduced to two. For
Resenet101, I deleted the last two layers and the last softmax layer used for
image classification.
Another modification takes place at the encoder side. The feedforward network
consists of two convolution layers with a RELU activation function in between.
The encoder side deals solely with the image part, where it is beneficial to
exploit the relative position of the features we have. Refer to Figure 2 for
the model architecture.

Figure 2: Model Architecture
### Training
The transformer decoder output goes to one fully connected layer, which
provides –-given the previous token–- a probability distribution
(, *k*
is vocabulary size) for each token in the sequence.
I trained the model using cross-entropy loss given the target ground truth
() where _T_ is the
length of the sequence. Also, I add the doubly stochastic attention
regularization [[8]](#8) to the cross-entropy loss to penalize high weights in
the encoder-decoder attention. This term encourages the summation of attention
weights across the sequence to be approximatively equal to one. By doing so,
the model will not concentrate on specific parts in the image when generating a
caption. Instead, it will look all over the image, leading to a richer and more
descriptive text [[8]](#8).
The loss function is defined as:
\right)\%20+\%20\sum_{l=1}^{L}{\frac{1}{L}\left(\sum_{d=1}^{D}\sum_{i=1}^{P^2}\left(1-\sum_{c=1}^{T}\alpha_{cidl}\right)^2\right)}})
where _D_ is the number of heads and _L_ is the number of layers.
I used Adam optimizer, with a batch size of thirty-two. The reader can find the
model sizes in the configuration file `code/config.json`. Evaluation metrics
used are Bleu [[9]](#9), METEOR [[10]](#10), and Gleu [[11]](#11).
I trained the model for one hundred epochs, with stopping criteria if the
tracked evaluation metric (bleu-4) does not improve for twenty successive
epochs. Also, the learning rate is reduced by 0.25% if the tracked evaluation
metric (bleu-4) does not improve for ten consecutive epochs. The evaluation of
the model against the validation split takes place every two epochs.
The pre-trained Glove embeddings [[12]](#12) initialize the word embedding
weights. The words embeddings are frozen for ten epochs. The Resnet101 network
is tuned from the beginning.
### Inference
A beam search of size five is used to generate a caption for the images in the
test split. The generation starts by feeding the image and the "start of
sentence" special tokens. Then at each iteration, five tokens with the highest
scores are chosen. The generation iteration stops when the "end of sentence" is
generated or the max length limit is reached.
## 5. Steps for Code Explanation
### 1. Data Loading and Preprocessing
- Load Annotations: The code first loads image-caption pairs from the COCO 2017 dataset. It uses JSON files containing images and corresponding captions (captions_train2017.json).
- Pairing Images and Captions: The code then creates a list (img_cap_pairs) that pairs image filenames with their respective captions.
- Dataframe for Captions: It organizes the data in a pandas DataFrame for easier manipulation, including creating a path to each image file.
- Sampling Data: 70,000 image-caption pairs are randomly sampled, making the dataset manageable without needing all data.
### 2. Text Preprocessing
- The code preprocesses captions to prepare them for the model. It lowercases the text, removes punctuation, replaces multiple spaces with single spaces, and adds [start] and [end] tokens, marking the beginning and end of each caption.
### 3. Tokenization
- Vocabulary Setup: A tokenizer (TextVectorization) is created with a vocabulary size of 15,000 words and a maximum token length of 40. It tokenizes captions, transforming them into sequences of integers.
- Saving Vocabulary: The vocabulary is saved to a file so that it can be reused later without retraining.
- Mapping Words to Indexes: word2idx and idx2word are mappings that convert words to indices and vice versa.
### 4. Dataset Preparation
- Image-Caption Mapping: Using a dictionary, each image is mapped to its list of captions. Then, the images are shuffled, and a train-validation split is made (80% for training, 20% for validation).
- Creating TensorFlow Datasets: Using the load_data function, images are resized, preprocessed, and tokenized captions are created as tensors. These tensors are batched for training and validation, improving memory efficiency and allowing parallel processing.
### 5. Data Augmentation
- Basic image augmentations (RandomFlip, RandomRotation, and RandomContrast) are applied to training images to help the model generalize better by learning from slightly altered versions of each image.
### 6. Model Architecture
#### CNN Encoder:
- An InceptionV3 model (pre-trained on ImageNet) is used to process images and extract features, which serve as input to the transformer.
#### Transformer Encoder Layer:
- A TransformerEncoderLayer with multi-head self-attention and normalization layers learns the relationships between image features.
#### Embeddings Layer:
- This layer adds positional embeddings, allowing the model to capture the order of words in captions.
#### Transformer Decoder Layer:
- The TransformerDecoderLayer generates captions. It includes multi-head attention, feedforward neural networks, and dropout to prevent overfitting. Masking ensures that tokens don’t “see” future tokens when predicting the next word.
### 7. Image Captioning Model Class
- The ImageCaptioningModel class wraps the encoder, decoder, and CNN encoder into a unified model for training and inference.
- Loss and Accuracy Calculation: Custom functions track model performance by calculating the loss and accuracy using the tokenized captions and generated predictions.
### 8. Training
- Loss Function: Sparse categorical cross-entropy is used to calculate the difference between predicted and actual tokens, excluding padding tokens.
- Early Stopping: Monitors validation loss to stop training if performance on the validation set stops improving.
- Model Compilation and Training: The model is compiled, optimized, and trained over multiple epochs with early stopping.
### 9. Evaluation and Caption Generation
- The generate_caption function generates a caption for a new image by feeding it through the model. The function iteratively predicts tokens, appending each token to the generated sequence until the [end] token appears.
### 10. Saving the Model
- The model weights are saved to a file (Image_Captioning_Model) to reload the model for future use without retraining.
## 6. Results and Analysis
### Deployed in Hugging Face Spaces and share image captioning service using Gradio
The Hugging Face Space Image Captioning GenAI serves as a user-friendly deployment of an image captioning model, designed to generate descriptive captions for uploaded images. The deployment leverages the Hugging Face Spaces infrastructure, which is ideal for hosting machine learning applications with interactive interfaces.
### Key Features of the Deployment:
- *Web-Based Interaction*: The Space offers an intuitive graphical interface for users to upload images and receive real-time AI-generated captions.
- *Scalability*: Built on Hugging Face’s robust hosting environment, the application ensures smooth operation, accommodating multiple users simultaneously.
- *Efficient Framework*: Likely powered by Gradio, the interface integrates seamlessly with the underlying Transformer-based model, enabling fast inference and visually engaging outputs.
- *Accessibility*: Users do not need any technical knowledge or setup to use the tool—everything is available in-browser.
[Gradio](http://pytorch.org/docs/master/optim.html#torch.optim.Optimizer) is a package that allows users to create simple web apps with just a few lines of code. It is essentially used for the same purpose as Streamlight and Flask but is much simpler to utilize. Many types of web interface tools can be selected including sketchpad, text boxes, file upload buttons, webcam, etc. Using these tools to receive various types of data as input, machine learning tasks such as classification and regression can easily be demoed.
You can deploy an interactive version of the image captioning service on your browser by running the following command. Please don't forget to set the `cocoapi_dir` and encoder/decoder model paths to the correct values.
```shell
python gradio_main.py
```
Access the service URL: https://huggingface.co./spaces/premanthcharan/Image_Captioining_GenAI

- A Web- Interface developed using Gradio platform and deployed into HuggingFace Spaces for user interaction

- Caption Generated: a red double decker bus driving down a street
### Model Training
Figure 3 and Figure 4 show the loss and bleu-4 scores during the training and
validation phases. These figures show that the model starts to overfit early
around epoch eight. The bleu-4 score and loss value unimproved after epoch 20.
The reason for overfitting may be due to the following reasons:
1. Not enough training data:
- The CPTR's encoder is initialized by the pre-trained ViT model [[1]](#1). In
the ViT paper, the model performs relatively well when trained on a
large dataset like ImageNet, which has 21 million Images [[6]](#6). In our
case, the model weights are randomly initialized, and we have less than
18.5 K images.
- Typically the dataset split configuration is 113,287, 5,000, and 5,000
images for training, validation, and test based on Karpathy et al.'s work
[[13]](#13). My split has way fewer images in the training dataset and is
based on the 80%, 20%, 20% configuration.
2. The image features learned from Resenet101 are patched to an N patches of
size _P x P_. Such configuration may not be the best design as these
features do not have to represent an image that could be transformed into a
sequence of subgrids. Flatten the Resnet101's features may be a better
design.
3. The pre-trained Resent101 has been tuned from the beginning, unlike the
word embedding layer. The gradient updates during early training stages
where the model does not learn yet may distort the image features of the
Resent101.
4. Unsuitable hyperparameters

### Inference Output
#### Generated Text Length
Figure 5 shows the generated caption's lengths distribution. The Figure
indicates that the model tends to generate shorter captions. The distribution
of the training caption's lengths (left) explains that behavior; the
distribution of the lengths is positively skewed. More specifically, the
maximum caption length generated by the model (21 tokens) accounts for 98.66%
of the lengths in the training set. See “code/experiment.ipynb Section 1.3”.

Figure 5: Generated caption's lengths distribution
## 7. Evaluation Metrics
The table below shows the mean and standard deviation of the performance
metrics across the test dataset. The bleu4 has the highest variation,
suggesting that the performance varies across the dataset. This high variation
is expected as the model training needs improvement, as discussed above. Also,
the distribution of the bleu4 scores over the test set shows that 83.3% of the
scores are less than 0.5. See “code/experiment.ipynb Section 1.4”.
| | bleu1 | bleu2 | bleu3 | bleu4 | gleu | meteor |
| :--- | :----: |:----: |:----: |:----: |:----: |:----: |
|mean ± std | 0.7180 ± 0.17 | 0.5116 ± 0.226 | 0.3791 ± 0.227 | 0.2918 ± 0.215 | 0.2814 ± 0.174 | 0.4975 ± 0.193
### Attention Visualisation
I will examine the last layer of the transformer encoder-decoder attention. The weights are averaged across its heads. Section 1.5 in the notebook "code/experiment.ipynb" shows that the weights contain outliers. I considered weights that far from 99.95% percentile and higher as outliers. The outlier's values are capped to the 99.95% percentile.
Fourteen samples were randomly selected from the test split to be examined. The sample image is superimposed with the attention weights for each generated token. The output is saved in either GIF format (one image for all generated tokens) or png format (one image for each token). All superimposed images are saved under "images/tests". The reader can examine the selected fourteen superimposed images under section 2.0 from the experiments notebook. You need to rerun all cells under Section 2.0. The samples are categorized as follows:
Category 1. two samples that have the highest bleu4= 1.0
Category 2. four samples that have the lowest bleu4 scores
Category 3. two samples that have the low value of bleu4 [up to 0.5]
Category 4. two samples that have bleu4 score= (0.5 - 0.7]
Category 5. two samples that have bleu4 score=(0.7 - 0.8]
Category 6. two samples that have bleu4 score= (0.8 - 1.0)
## 8. References
<a id="1">[1]</a> Liu, W., Chen, S., Guo, L., Zhu, X., & Liu, J. (2021). CPTR:
Full transformer network for image captioning. arXiv preprint
[arXiv:2101.10804](https://arxiv.org/abs/2101.10804).
<a id="2">[2]</a> Lin, T. Y., Maire, M., Belongie, S., Hays, J., Perona, P.,
Ramanan, D., ... & Zitnick, C. L. (2014, September). Microsoft coco: Common
objects in context. In European conference on computer vision (pp. 740-755).
Springer, Cham.
<a id="3">[3]</a> A. Vaswani et al., 'Attention is all you need', Advances in neural
information processing systems, vol. 30, 2017.
<a id="4">[4]</a> M. Z. Hossain, F. Sohel, M. F. Shiratuddin, and H. Laga, 'A Comprehensive
Survey of Deep Learning for Image Captioning', arXiv:1810.04020 [cs, stat],
Oct. 2018, Accessed: Mar. 03, 2022. [Online]. Available:
http://arxiv.org/abs/1810.04020.
<a id="5">[5]</a> S. Hochreiter and J. Schmidhuber, ‘Long short-term memory’, Neural
computation, vol. 9, no. 8, pp. 1735–1780, 1997.
<a id="6">[6]</a> A. Dosovitskiy et al., 'An image is worth 16x16 words: Transformers for
image recognition at scale', arXiv preprint arXiv:2010.11929, 2020.
<a id="7">[7]</a> K. He, X. Zhang, S. Ren, and J. Sun, 'Deep Residual Learning for Image
Recognition', arXiv:1512.03385 [cs], Oct. 2015, Accessed: Mar. 06, 2022.
[Online]. Available: http://arxiv.org/abs/1512.03385.
<a id="8">[8]</a> K. Xu et al., 'Show, Attend and Tell: Neural Image Caption Generation with
Visual Attention', arXiv:1502.03044 [cs], Apr. 2016, Accessed: Mar. 07, 2022.
[Online]. Available: http://arxiv.org/abs/1502.03044.
<a id="9">[9]</a> K. Papineni, S. Roukos, T. Ward, and W.-J. Zhu, 'Bleu: a method for
automatic evaluation of machine translation', in Proceedings of the 40th annual
meeting of the Association for Computational Linguistics, 2002, pp. 311–318.
<a id="10">[10]</a> S. Banerjee and A. Lavie, 'METEOR: An automatic metric for MT evaluation
with improved correlation with human judgments', in Proceedings of the acl
workshop on intrinsic and extrinsic evaluation measures for machine translation
and/or summarization, 2005, pp. 65–72.
<a id="11">[11]</a> A. Mutton, M. Dras, S. Wan, and R. Dale, 'GLEU: Automatic evaluation of
sentence-level fluency', in Proceedings of the 45th Annual Meeting of the
Association of Computational Linguistics, 2007, pp. 344–351.
<a id="12">[12]</a> J. Pennington, R. Socher, and C. D. Manning, 'Glove: Global vectors for
word representation', in Proceedings of the 2014 conference on empirical
methods in natural language processing (EMNLP), 2014, pp. 1532–1543.
<a id="13">[13]</a> A. Karpathy and L. Fei-Fei, 'Deep visual-semantic alignments for
generating image descriptions', in Proceedings of the IEEE conference on
computer vision and pattern recognition, 2015, pp. 3128–3137.
<a id="13">[14]</a> Oriol Vinyals, Alexander Toshev, Samy Bengio, Dumitru Erhan; Proceedings of the IEEE Conference on Computer Vision and Pattern Recognition (CVPR), 2015, pp. 3156-3164.
<a id="13">[15]</a> Hugging Face Spaces Forum about image captioning model.
https://huggingface.co./docs/transformers/main/en/tasks/image_captioning
<a id="13">[16]</a> QuickStart Guide to GitHub pages
https://docs.github.com/en/pages/quickstart
<a id="13">[17]</a> Microsoft COCO: Common Objects in Context (cs.CV). arXiv:1405.0312 [cs.CV]
https://doi.org/10.48550/arXiv.1405.0312
<a id="13">[18]</a> Show, Attend and Tell: Neural Image Caption Generation with Visual Attention arXiv:1502.03044v3 [cs.LG] 19 Apr 2016 https://doi.org/10.48550/arXiv.1502.03044
<a id="13">[19]</a> Deep Residual Learning for Image Recognition arXiv:1512.03385v1 [cs.CV] 10 Dec 2015
<a id="13">[20]</a> Gradio Quickstart Guide https://www.gradio.app/guides/quickstart
|