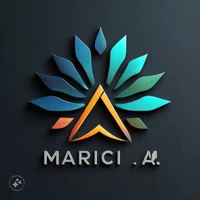
Mārīcī AI Solutions
AI & ML interests
1. Developing Ethical and Responsible AI Interest: Building models that prioritize transparency, fairness, and privacy. Ensuring our AI systems are robust, explainable, and aligned with ethical guidelines to avoid bias and unintended consequences. Why: Trust in AI is essential for widespread adoption, especially in regulated sectors like finance, healthcare, and government. Leading by example in this area helps set a positive precedent and inspires confidence in AI’s potential. 2. Expanding Retrieval-Augmented Generation (RAG) Interest: RAG remains a transformative method in making AI more intelligent by pairing language models with robust databases. I’m interested in architecting systems that improve the way data is retrieved, integrated, and used within applications to provide accurate, contextually relevant answers. Why: Many real-world applications require both the creativity of generative AI and the accuracy of fact-based retrieval. This blend supports everything from intelligent chatbots to advanced research tools, amplifying productivity and user satisfaction. 3. Scaling AI/ML Infrastructure Interest: Developing scalable and efficient infrastructure for model training and deployment. This includes using distributed systems, leveraging cloud platforms, and ensuring continuous integration and deployment pipelines for AI models. Why: As models become more complex and data requirements grow, building a resilient, scalable infrastructure is essential to maintain performance and reduce costs. This area would enable AI teams to move quickly from concept to production. 4. Personalization and User-Centric AI Interest: Implementing models that prioritize personalization based on user data and interaction history. Techniques like reinforcement learning and dynamic user modeling are particularly exciting for creating highly adaptive user experiences. Why: Personalized AI applications can enhance customer engagement and retention, particularly in industries like retail, entertainment, and education. This aligns AI development with a user-centered approach, which directly impacts user satisfaction. 5. Human-AI Collaboration and Augmentation Interest: Creating AI tools that augment human abilities rather than replace them, focusing on decision support systems, knowledge work, and creative assistance tools. Why: Building AI that collaborates with humans can help professionals in various fields (medicine, finance, engineering) make better, faster, and more informed decisions, pushing AI towards positive, supportive roles. 6. AI for Social Good and Sustainability Interest: Developing AI models to address pressing global challenges, such as climate change, healthcare accessibility, and sustainable development. Applications might include predictive modeling for climate impacts or optimizing resource management. Why: Beyond business impact, AI has the potential to address broader societal issues. Leading initiatives in this area would align business objectives with impactful, purpose-driven projects that contribute to the global good.