File size: 3,794 Bytes
6169d5c 80b7bab 3923a48 80b7bab 3923a48 80b7bab 3923a48 80b7bab 3923a48 80b7bab 3923a48 80b7bab 3923a48 80b7bab 3923a48 80b7bab 3923a48 80b7bab 9baa5df 3923a48 6169d5c |
1 2 3 4 5 6 7 8 9 10 11 12 13 14 15 16 17 18 19 20 21 22 23 24 25 26 27 28 29 30 31 32 33 34 35 36 37 38 39 40 41 42 43 44 45 46 47 48 49 50 51 52 53 54 55 56 57 58 59 60 61 62 63 64 65 66 67 68 69 70 71 72 73 74 75 76 77 78 79 80 81 82 83 84 85 86 87 88 89 90 91 92 93 94 95 96 97 98 99 |
---
license: apache-2.0
---
# LimaRP Persona-Scenario Generator (v5, Alpaca)
A previously unpublished LoRA adapter for [Yarn-Llama-2-7B-64k](https://huggingface.co./NousResearch/Yarn-Llama-2-7b-64k) made
for internal use. Its primary purpose is generating Persona and Scenario (summary) from LimaRP `yaml` source data.
To some extent it can work with different text types, however.
## Prompt format
```
### Input:
{Your text here}
### Response:
Charactername's Persona: {output goes here}
```
Replace `Charactername` with the name of the character you want to infer a Persona for.
By default this LoRA looks for the placeholder names `<FIRST>` and `<SECOND>` (in this
respective order) but it can work with proper names as well.
## Example
This image shows what would happen (red box) after adding data in the format shown in the left pane.
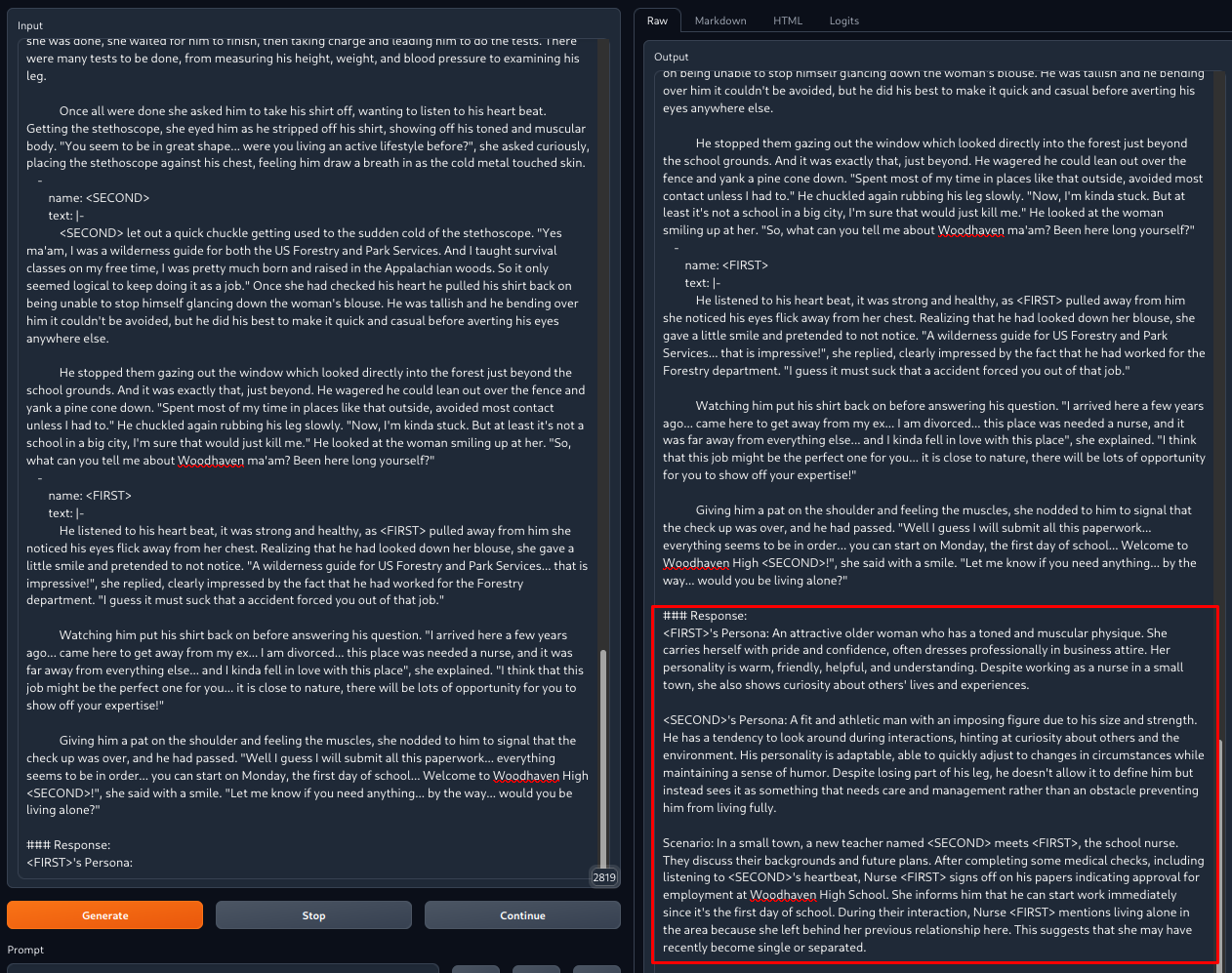
In practice the results would be double-checked and manually tweaked to diversify the
outputs and adding character quirks, peculiarities or traits that the model couldn't catch.
## Known issues
- While the scenario/summary is often remarkably accurate, personas don't show a very high accuracy and can be repetitive.
- Persona and Scenario may exhibit `gpt`-isms.
- Peculiar character quirks may not be observed by the model.
- The LoRA hasn't been extensively tested with different input formats.
- There are apparently issues with the EOS token getting generated too early. It's suggested to disable it.
## Training procedure
### Training hyperparameters
The following hyperparameters were used during training:
- learning_rate: 0.00025
- train_batch_size: 2
- eval_batch_size: 2
- seed: 42
- distributed_type: multi-GPU
- num_devices: 4
- total_train_batch_size: 8
- total_eval_batch_size: 8
- optimizer: Adam with betas=(0.9,0.999) and epsilon=1e-08
- lr_scheduler_type: cosine
- lr_scheduler_warmup_steps: 10
- num_epochs: 2
### Training results
| Training Loss | Epoch | Step | Validation Loss |
|:-------------:|:-----:|:----:|:---------------:|
| 1.992 | 0.06 | 15 | 1.8884 |
| 1.8026 | 0.12 | 30 | 1.8655 |
| 1.7713 | 0.19 | 45 | 1.8539 |
| 1.7145 | 0.25 | 60 | 1.8502 |
| 1.6686 | 0.31 | 75 | 1.8507 |
| 1.8409 | 0.37 | 90 | 1.8469 |
| 1.7741 | 0.44 | 105 | 1.8434 |
| 1.7384 | 0.5 | 120 | 1.8407 |
| 1.7562 | 0.56 | 135 | 1.8390 |
| 1.7392 | 0.62 | 150 | 1.8373 |
| 1.8735 | 0.68 | 165 | 1.8381 |
| 1.8406 | 0.75 | 180 | 1.8377 |
| 1.6602 | 0.81 | 195 | 1.8350 |
| 1.7803 | 0.87 | 210 | 1.8341 |
| 1.7212 | 0.93 | 225 | 1.8329 |
| 1.8126 | 1.0 | 240 | 1.8330 |
| 1.8776 | 1.06 | 255 | 1.8314 |
| 1.7892 | 1.12 | 270 | 1.8328 |
| 1.7029 | 1.18 | 285 | 1.8338 |
| 1.7094 | 1.24 | 300 | 1.8322 |
| 1.7921 | 1.31 | 315 | 1.8310 |
| 1.8309 | 1.37 | 330 | 1.8316 |
| 1.7373 | 1.43 | 345 | 1.8309 |
| 1.7873 | 1.49 | 360 | 1.8313 |
| 1.7151 | 1.56 | 375 | 1.8306 |
| 1.7529 | 1.62 | 390 | 1.8300 |
| 1.7516 | 1.68 | 405 | 1.8293 |
| 1.7704 | 1.74 | 420 | 1.8294 |
| 1.6351 | 1.8 | 435 | 1.8290 |
| 1.6186 | 1.87 | 450 | 1.8291 |
| 1.7086 | 1.93 | 465 | 1.8295 |
| 1.6595 | 1.99 | 480 | 1.8290 |
### Framework versions
- Transformers 4.34.0.dev0
- Pytorch 2.0.1+cu118
- Datasets 2.14.5
- Tokenizers 0.14.0 |