File size: 1,650 Bytes
1f6c952 ac4d9db 27107dd 94d59ed 56736d2 124463d 56736d2 63f8686 |
1 2 3 4 5 6 7 8 9 10 11 12 13 14 15 16 17 18 19 20 21 22 |
---
license: other
license_name: link-attribution
license_link: https://dejanmarketing.com/link-attribution/
language:
- en
metrics:
- accuracy
base_model: albert/albert-base-v2
pipeline_tag: text-classification
---
[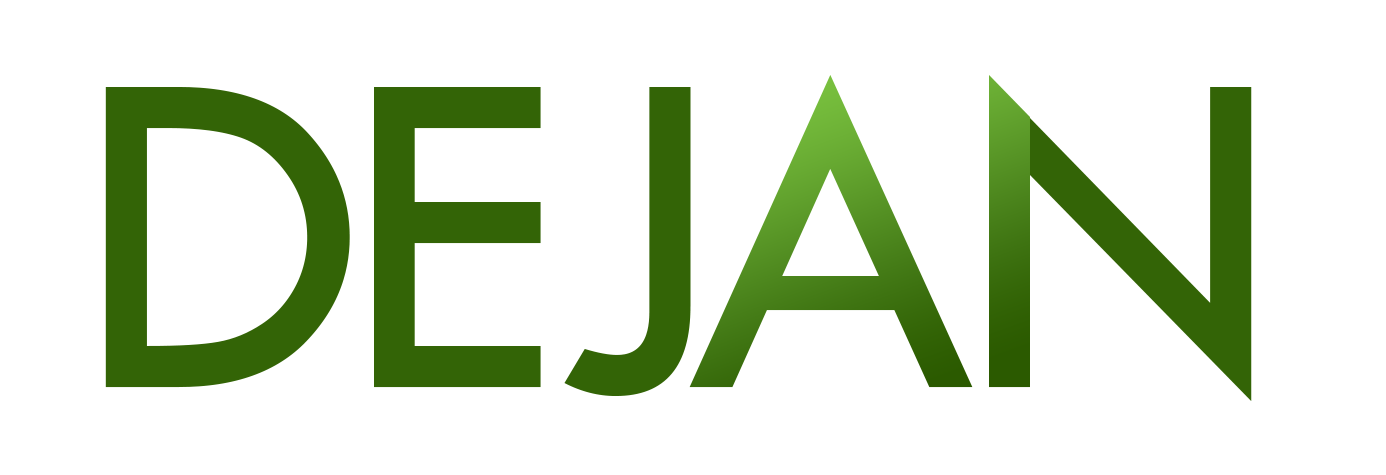](https://dejan.ai/blog/search-query-quality-classifier/)
We build on [the work](https://research.google/pubs/identifying-well-formed-natural-language-questions/) by Manaal Faruqui and Dipanjan Das from [Google AI Language](https://research.google/teams/language/) team to train a search query classifier of well-formed search queries. Our model offers a [10% improvement](https://dejan.ai/blog/search-query-quality-classifier/) over Google's classifier by utilising ALBERT architecture instead of LSTM. With accuracy of 80%, the model is production ready and has already been deployed in Dejan AI's query processing pipeline. The role of the model is to help identify query expansion candidates by flagging ambiguous queries retrieved via Google Search Console API.
## Practical Application
Most search queries are ambiguous making it difficult to classify intent and make decisions on how to optimise for them. Query expansion helps, but only only if you know which queries to expand. This is where our model comes in. Take it for a spin here and try proper questions vs raw keyword queries and [experience the model in action](https://dejan.ai/tools/query-expansion-classifier/).
# Engage Our Team
Interested in using this in an automated pipeline for bulk query classification?
Please [book an appointment](https://dejan.ai/call/) to discuss your needs. |