Update README.md
Browse files
LICENSE
ADDED
@@ -0,0 +1,51 @@
|
|
|
|
|
|
|
|
|
|
|
|
|
|
|
|
|
|
|
|
|
|
|
|
|
|
|
|
|
|
|
|
|
|
|
|
|
|
|
|
|
|
|
|
|
|
|
|
|
|
|
|
|
|
|
|
|
|
|
|
|
|
|
|
|
|
|
|
|
|
|
|
|
|
|
|
|
|
|
|
|
|
|
|
|
|
|
|
|
|
|
|
|
|
|
|
|
|
|
|
|
|
|
|
|
1 |
+
Qwen LICENSE AGREEMENT
|
2 |
+
|
3 |
+
Qwen LICENSE AGREEMENT Release Date: September 19, 2024
|
4 |
+
|
5 |
+
By clicking to agree or by using or distributing any portion or element of the Qwen Materials, you will be deemed to have recognized and accepted the content of this Agreement, which is effective immediately.
|
6 |
+
|
7 |
+
1. Definitions
|
8 |
+
a. This Qwen LICENSE AGREEMENT (this "Agreement") shall mean the terms and conditions for use, reproduction, distribution and modification of the Materials as defined by this Agreement.
|
9 |
+
b. "We" (or "Us") shall mean Alibaba Cloud.
|
10 |
+
c. "You" (or "Your") shall mean a natural person or legal entity exercising the rights granted by this Agreement and/or using the Materials for any purpose and in any field of use.
|
11 |
+
d. "Third Parties" shall mean individuals or legal entities that are not under common control with us or you.
|
12 |
+
e. "Qwen" shall mean the large language models, and software and algorithms, consisting of trained model weights, parameters (including optimizer states), machine-learning model code, inference-enabling code, training-enabling code, fine-tuning enabling code and other elements of the foregoing distributed by us.
|
13 |
+
f. "Materials" shall mean, collectively, Alibaba Cloud's proprietary Qwen and Documentation (and any portion thereof) made available under this Agreement.
|
14 |
+
g. "Source" form shall mean the preferred form for making modifications, including but not limited to model source code, documentation source, and configuration files.
|
15 |
+
h. "Object" form shall mean any form resulting from mechanical transformation or translation of a Source form, including but not limited to compiled object code, generated documentation, and conversions to other media types.
|
16 |
+
|
17 |
+
2. Grant of Rights
|
18 |
+
You are granted a non-exclusive, worldwide, non-transferable and royalty-free limited license under Alibaba Cloud's intellectual property or other rights owned by us embodied in the Materials to use, reproduce, distribute, copy, create derivative works of, and make modifications to the Materials.
|
19 |
+
|
20 |
+
3. Redistribution
|
21 |
+
You may distribute copies or make the Materials, or derivative works thereof, available as part of a product or service that contains any of them, with or without modifications, and in Source or Object form, provided that you meet the following conditions:
|
22 |
+
a. You shall give any other recipients of the Materials or derivative works a copy of this Agreement;
|
23 |
+
b. You shall cause any modified files to carry prominent notices stating that you changed the files;
|
24 |
+
c. You shall retain in all copies of the Materials that you distribute the following attribution notices within a "Notice" text file distributed as a part of such copies: "Qwen is licensed under the Qwen LICENSE AGREEMENT, Copyright (c) Alibaba Cloud. All Rights Reserved."; and
|
25 |
+
d. You may add your own copyright statement to your modifications and may provide additional or different license terms and conditions for use, reproduction, or distribution of your modifications, or for any such derivative works as a whole, provided your use, reproduction, and distribution of the work otherwise complies with the terms and conditions of this Agreement.
|
26 |
+
|
27 |
+
4. Restrictions
|
28 |
+
If you are commercially using the Materials, and your product or service has more than 100 million monthly active users, you shall request a license from us. You cannot exercise your rights under this Agreement without our express authorization.
|
29 |
+
|
30 |
+
5. Rules of use
|
31 |
+
a. The Materials may be subject to export controls or restrictions in China, the United States or other countries or regions. You shall comply with applicable laws and regulations in your use of the Materials.
|
32 |
+
b. If you use the Materials or any outputs or results therefrom to create, train, fine-tune, or improve an AI model that is distributed or made available, you shall prominently display “Built with Qwen” or “Improved using Qwen” in the related product documentation.
|
33 |
+
|
34 |
+
6. Intellectual Property
|
35 |
+
a. We retain ownership of all intellectual property rights in and to the Materials and derivatives made by or for us. Conditioned upon compliance with the terms and conditions of this Agreement, with respect to any derivative works and modifications of the Materials that are made by you, you are and will be the owner of such derivative works and modifications.
|
36 |
+
b. No trademark license is granted to use the trade names, trademarks, service marks, or product names of us, except as required to fulfill notice requirements under this Agreement or as required for reasonable and customary use in describing and redistributing the Materials.
|
37 |
+
c. If you commence a lawsuit or other proceedings (including a cross-claim or counterclaim in a lawsuit) against us or any entity alleging that the Materials or any output therefrom, or any part of the foregoing, infringe any intellectual property or other right owned or licensable by you, then all licenses granted to you under this Agreement shall terminate as of the date such lawsuit or other proceeding is commenced or brought.
|
38 |
+
|
39 |
+
7. Disclaimer of Warranty and Limitation of Liability
|
40 |
+
a. We are not obligated to support, update, provide training for, or develop any further version of the Qwen Materials or to grant any license thereto.
|
41 |
+
b. THE MATERIALS ARE PROVIDED "AS IS" WITHOUT ANY EXPRESS OR IMPLIED WARRANTY OF ANY KIND INCLUDING WARRANTIES OF MERCHANTABILITY, NONINFRINGEMENT, OR FITNESS FOR A PARTICULAR PURPOSE. WE MAKE NO WARRANTY AND ASSUME NO RESPONSIBILITY FOR THE SAFETY OR STABILITY OF THE MATERIALS AND ANY OUTPUT THEREFROM.
|
42 |
+
c. IN NO EVENT SHALL WE BE LIABLE TO YOU FOR ANY DAMAGES, INCLUDING, BUT NOT LIMITED TO ANY DIRECT, OR INDIRECT, SPECIAL OR CONSEQUENTIAL DAMAGES ARISING FROM YOUR USE OR INABILITY TO USE THE MATERIALS OR ANY OUTPUT OF IT, NO MATTER HOW IT’S CAUSED.
|
43 |
+
d. You will defend, indemnify and hold harmless us from and against any claim by any third party arising out of or related to your use or distribution of the Materials.
|
44 |
+
|
45 |
+
8. Survival and Termination.
|
46 |
+
a. The term of this Agreement shall commence upon your acceptance of this Agreement or access to the Materials and will continue in full force and effect until terminated in accordance with the terms and conditions herein.
|
47 |
+
b. We may terminate this Agreement if you breach any of the terms or conditions of this Agreement. Upon termination of this Agreement, you must delete and cease use of the Materials. Sections 7 and 9 shall survive the termination of this Agreement.
|
48 |
+
|
49 |
+
9. Governing Law and Jurisdiction.
|
50 |
+
a. This Agreement and any dispute arising out of or relating to it will be governed by the laws of China, without regard to conflict of law principles, and the UN Convention on Contracts for the International Sale of Goods does not apply to this Agreement.
|
51 |
+
b. The People's Courts in Hangzhou City shall have exclusive jurisdiction over any dispute arising out of this Agreement.
|
README.md
CHANGED
@@ -1,5 +1,7 @@
|
|
1 |
---
|
2 |
-
license:
|
|
|
|
|
3 |
pipeline_tag: image-text-to-text
|
4 |
library_name: transformers
|
5 |
base_model:
|
@@ -10,371 +12,173 @@ language:
|
|
10 |
- multilingual
|
11 |
tags:
|
12 |
- internvl
|
13 |
-
- vision
|
14 |
-
- ocr
|
15 |
-
- multi-image
|
16 |
-
- video
|
17 |
- custom_code
|
18 |
---
|
19 |
|
20 |
# InternVL2_5-78B
|
21 |
|
22 |
-
[\[📂 GitHub\]](https://github.com/OpenGVLab/InternVL) [\[🆕 Blog\]](https://internvl.github.io/blog/)
|
23 |
-
[\[📜 InternVL 2.5 Report\]]()
|
24 |
-
[\[📜 InternVL 1.0 Paper\]](https://arxiv.org/abs/2312.14238) [\[📜 InternVL 1.5 Report\]](https://arxiv.org/abs/2404.16821)
|
25 |
-
[\[🗨️ Chat Demo\]](https://internvl.opengvlab.com/) [\[🤗 HF Demo\]](https://huggingface.co/spaces/OpenGVLab/InternVL) [\[🚀 Quick Start\]](#quick-start) [\[📖 Documents\]](https://internvl.readthedocs.io/en/latest/)
|
26 |
|
|
|
27 |
|
28 |
-
|
|
|
|
|
29 |
|
30 |
## Introduction
|
31 |
|
32 |
-
We are excited to introduce InternVL 2.5
|
33 |
-
|
34 |
-
Through extensive evaluations on a wide range of benchmarks, including multi-discipline reasoning, document understanding, multi-image / video understanding, real-world comprehension, multimodal hallucination detection, visual grounding, multilingual capabilities, and pure language processing, InternVL 2.5 exhibits competitive performance, rivaling leading commercial models such as GPT-4o and Claude-3.5-Sonnet. Notably, our model is the first open-source MLLMs to achieve over **70%** on the **MMMU benchmark**. We hope this model contributes to the open-source community by setting new standards for developing and applying multimodal AI systems. This repository contains the instruction-tuned **InternVL2_5-78B** model.
|
35 |
-
|
36 |
-
We delve into the relationship between model scaling and performance, systematically exploring the performance trends in vision encoders, language models, dataset sizes, and test-time configurations. For more details, please refer to our [blog](), [tech report]() and [GitHub](https://github.com/OpenGVLab/InternVL).
|
37 |
-
|
38 |
-
| Model Name | Vision Part | Language Part | HF Link |
|
39 |
-
| :------------------: | :---------------------------------------------------------------------------------: | :------------------------------------------------------------------------------------------: | :--------------------------------------------------------------: |
|
40 |
-
| InternVL2_5-1B | [InternViT-300M-448px-V2_5](https://huggingface.co/OpenGVLab/InternViT-300M-448px-V2_5) | [Qwen2.5-0.5B-Instruct](https://huggingface.co/Qwen/Qwen2.5-0.5B-Instruct) | [🤗 link](https://huggingface.co/OpenGVLab/InternVL2_5-1B) |
|
41 |
-
| InternVL2_5-2B | [InternViT-300M-448px-V2_5](https://huggingface.co/OpenGVLab/InternViT-300M-448px-V2_5) | [internlm2_5-1_8b-chat](https://huggingface.co/internlm/internlm2_5-1_8b-chat) | [🤗 link](https://huggingface.co/OpenGVLab/InternVL2_5-2B) |
|
42 |
-
| InternVL2_5-4B | [InternViT-300M-448px-V2_5](https://huggingface.co/OpenGVLab/InternViT-300M-448px-V2_5) | [Qwen2.5-3B-Instruct](https://huggingface.co/Qwen/Qwen2.5-3B-Instruct) | [🤗 link](https://huggingface.co/OpenGVLab/InternVL2_5-4B) |
|
43 |
-
| InternVL2_5-8B | [InternViT-300M-448px-V2_5](https://huggingface.co/OpenGVLab/InternViT-300M-448px-V2_5) | [internlm2_5-7b-chat](https://huggingface.co/internlm/internlm2_5-7b-chat) | [🤗 link](https://huggingface.co/OpenGVLab/InternVL2_5-8B) |
|
44 |
-
| InternVL2_5-26B | [InternViT-6B-448px-V2_5](https://huggingface.co/OpenGVLab/InternViT-6B-448px-V2_5) | [internlm2_5-20b-chat](https://huggingface.co/internlm/internlm2_5-20b-chat) | [🤗 link](https://huggingface.co/OpenGVLab/InternVL2_5-26B) |
|
45 |
-
| InternVL2_5-38B | [InternViT-6B-448px-V2_5](https://huggingface.co/OpenGVLab/InternViT-6B-448px-V2_5) | [Qwen2.5-32B-Instruct](https://huggingface.co/Qwen/Qwen2.5-32B-Instruct) | [🤗 link](https://huggingface.co/OpenGVLab/InternVL2_5-38B) |
|
46 |
-
| InternVL2_5-78B | [InternViT-6B-448px-V2_5](https://huggingface.co/OpenGVLab/InternViT-6B-448px-V2_5) | [Qwen2.5-72B-Instruct](https://huggingface.co/Qwen/Qwen2.5-72B-Instruct) | [🤗 link](https://huggingface.co/OpenGVLab/InternVL2_5-78B) |
|
47 |
-
|
48 |
-
## Model Details
|
49 |
-
|
50 |
-
InternVL 2.5is a multimodal large language model series, featuring models of various sizes. For each size, we release instruction-tuned models optimized for multimodal tasks. InternVL2_5-78B consists of [InternViT-6B-448px-V2_5](https://huggingface.co/OpenGVLab/InternViT-6B-448px-V2_5), an MLP projector, and [Qwen2.5-72B-Instruct](https://huggingface.co/Qwen/Qwen2.5-72B-Instruct).
|
51 |
-
|
52 |
-
## Performance
|
53 |
-
|
54 |
-
### Image Benchmarks
|
55 |
-
|
56 |
-
|
57 |
-
| Benchmark | GPT-4V | GPT-4o-20240513 | Claude-3-Opus | Claude-3.5-Sonnet | Gemini-1.5-Pro | LLaVA-OneVision-72B | Qwen2-VL-72B | InternVL2.5-78B |
|
58 |
-
|----------------------------|-------------|-----------------|---------------|-------------------|----------------|---------------------|--------------|-----------------|
|
59 |
-
| MMMU<sub>val<sub> | 63.1 | 69.1 | - | 68.3 | 62.2 | 56.8 | 64.5 | 70.1 |
|
60 |
-
| MMMU<sub>test<sub> | - | - | - | - | - | - | - | 61.8 |
|
61 |
-
| MMMU-PRO<sub>overall<sub> | - | 51.9 | - | 51.5 | 46.9 | 31.0 | 46.2 | 48.6 |
|
62 |
-
| MathVista<sub>mini<sub> | 58.1 | 63.8 | - | 67.7 | 63.9 | 67.5 | 70.5 | 72.3 |
|
63 |
-
| MathVision<sub>mini<sub> | - | - | - | - | - | - | - | 34.9 |
|
64 |
-
| MathVision<sub>full<sub> | 24.0 | 30.4 | - | - | 19.2 | - | 25.9 | 32.2 |
|
65 |
-
| MathVerse<sub>mini<sub> | 32.8 | 50.2 | - | - | - | 39.1 | - | 51.7 |
|
66 |
-
| Olympiad Bench | 18.0 | 25.9 | - | - | - | - | - | 11.6 |
|
67 |
-
| AI2D<sub>(w / wo M)<sub> | 78.2 / 89.4 | 84.6 / 94.2 | 70.6 / 88.1 | 81.2 / 94.7 | 79.1 / 94.4 | 85.6 / - | 88.1 / - | 89.1 / 95.7 |
|
68 |
-
| ChartQA<sub>test avg.<sub> | 78.5 | 85.7 | 80.8 | 90.8 | 87.2 | 83.7 | 88.3 | 88.3 |
|
69 |
-
| TextVQA<sub>val<sub> | 78.0 | 77.4 | 67.5 | 74.1 | 78.8 | 80.5 | 85.5 | 83.4 |
|
70 |
-
| DocVQA<sub>test<sub> | 88.4 | 92.8 | 89.3 | 95.2 | 93.1 | 91.3 | 96.5 | 95.1 |
|
71 |
-
| InfoVQA<sub>test<sub> | 75.1 | 79.2 | 55.6 | 74.3 | 81.0 | 74.9 | 84.5 | 84.1 |
|
72 |
-
| OCR-Bench | 645 | 736 | 694 | 788 | 754 | 741 | 877 | 854 |
|
73 |
-
| SEED-2 Plus | 53.8 | 72.0 | 44.2 | 71.7 | - | 69.7 | - | 71.3 |
|
74 |
-
| CharXiv<sub>RQ/DQ<sub> | 37.1 / 79.9 | 47.1 / 84.5 | 30.2 / 71.6 | 60.2 / 84.3 | 43.3 / 72.0 | - | 91.3 / 94.6 | 42.4 / 82.3 |
|
75 |
-
| VCR-EN-Easy<sub>(EM / Jaccard)<sub> | 52.0 / 65.4 | 91.6 / 96.4 | 62.0 / 77.7 | 63.9 / 74.7 | 62.7 / 77.7 | - | 94.6 | 95.7 / 94.5 |
|
76 |
-
| BLINK<sub>val<sub> | 54.6 | 68.0 | - | - | - | 55.4 | - | 63.8 |
|
77 |
-
| Mantis Eval | 62.7 | - | - | - | - | 77.6 | - | 77.0 |
|
78 |
-
| MMIU | - | 55.7 | - | 53.4 | 53.4 | - | - | 55.8 |
|
79 |
-
| Muir Bench | 62.3 | 68.0 | - | - | - | 54.8 | - | 63.5 |
|
80 |
-
| MMT<sub>val<sub> | 64.3 | 65.4 | - | - | 64.5 | - | 71.8 | 70.8 |
|
81 |
-
| MIRB<sub>avg.<sub> | 53.1 | - | - | - | - | - | - | 61.1 |
|
82 |
-
| RealWorld QA | 61.4 | 75.4 | - | 60.1 | 67.5 | 71.9 | 77.8 | 78.7 |
|
83 |
-
| MME-RW<sub>EN<sub> | - | 45.2 | - | 51.6 | 38.2 | - | - | 62.9 |
|
84 |
-
| WildVision<sub>(win rate)<sub> | 71.8 | 80.6 | - | - | - | - | - | 71.4 |
|
85 |
-
| R-Bench | 65.6 | 77.7 | - | - | - | - | - | 77.2 |
|
86 |
-
| MME<sub>sum<sub> | 1926.6 | -- | 1586.8 | -- | -- | 2261.0 | 2482.7 | 2494.5 |
|
87 |
-
| MMB<sub>(EN / CN)<sub> | 81.0 / 80.2 | 83.4 / 82.1 | 63.3 / 59.2 | 82.6 / 83.5 | 73.9 / 73.8 | 85.8 / 85.3 | 86.5 / 86.6 | 88.3 / 88.5 |
|
88 |
-
| MMBv1.1<sub>EN<sub> | 80.0 | 83.1 | 60.1 | 80.9 | 74.6 | 85.0 | 85.9 | 87.4 |
|
89 |
-
| MMVet<sub>turbo<sub> | 67.5 | 69.1 | 51.7 | 70.1 | 64.0 | 60.6 | 74.0 | 72.3 |
|
90 |
-
| MMVetv2<sub>0613<sub> | 66.3 | 71.0 | 55.8 | 71.8 | 66.9 | -- | 66.9 | 65.5 |
|
91 |
-
| MMStar | 56.0 | 64.7 | 45.7 | 65.1 | 59.1 | 65.8 | 68.3 | 69.5 |
|
92 |
-
| HallBench<sub>avg.<sub> | 46.5 | 55.0 | 37.8 | 55.5 | 45.6 | 49.0 | 58.1 | 57.4 |
|
93 |
-
| MMHal<sub>score<sub> | -- | 4.00 | -- | -- | -- | -- | -- | 3.89 |
|
94 |
-
| CRPE<sub>relation<sub> | -- | 76.6 | -- | -- | -- | -- | -- | 78.8 |
|
95 |
-
| POPE<sub>avg.<sub> | -- | 86.9 | -- | -- | -- | -- | -- | 90.8 |
|
96 |
-
|
97 |
-
|
98 |
-
### Video Benchmarks
|
99 |
-
|
100 |
-
| Model Name | Video-MME (wo / w sub) | MVBench | MMBench-Video (val) | MLVU (M-Avg) | LongVideoBench (val total) | CG-Bench v1.1 (long / clue acc.) |
|
101 |
-
|---------------------------------------------|-------------|------|-------|-------|------|-------------|
|
102 |
-
| **InternVL2.5-1B** | 50.3 / 52.3 | 64.3 | 1.36 | 57.3 | 47.9 | - |
|
103 |
-
| Qwen2-VL-2B | 55.6 / 60.4 | 63.2 | - | - | - | - |
|
104 |
-
| **InternVL2.5-2B** | 51.9 / 54.1 | 68.8 | 1.44 | 61.4 | 52.0 | - |
|
105 |
-
| **InternVL2.5-4B** | 62.3 / 63.6 | 71.6 | 1.73 | 68.3 | 55.2 | - |
|
106 |
-
| VideoChat2-HD | 45.3 / 55.7 | 62.3 | 1.22 | 47.9 | - | - |
|
107 |
-
| MiniCPM-V-2.6 | 60.9 / 63.6 | - | 1.70 | - | 54.9 | - |
|
108 |
-
| LLaVA-OneVision-7B | 58.2 / - | 56.7 | - | - | - | - |
|
109 |
-
| Qwen2-VL-7B | 63.3 / 69.0 | 67.0 | 1.44 | - | 55.6 | - |
|
110 |
-
| **InternVL2.5-8B** | 64.2 / 66.9 | 72.0 | 1.68 | 68.9 | 60.0 | - |
|
111 |
-
| **InternVL2.5-26B** | 66.9 / 69.2 | 75.2 | 1.86 | 72.3 | 59.9 | - |
|
112 |
-
| Oryx-1.5-32B | 67.3 / 74.9 | 70.1 | 1.52 | 72.3 | - | - |
|
113 |
-
| VILA-1.5-40B | 60.1 / 61.1 | - | 1.61 | 56.7 | - | - |
|
114 |
-
| **InternVL2.5-38B** | 70.7 / 73.1 | 74.4 | 1.82 | 75.3 | 63.3 | - |
|
115 |
-
| GPT-4V/4T | 59.9 / 63.3 | 43.7 | 1.53 | 49.2 | 59.1 | - |
|
116 |
-
| GPT-4o-20240513 | 71.9 / 77.2 | - | 1.63 | 64.6 | 66.7 | - |
|
117 |
-
| GPT-4o-20240806 | - | - | 1.87 | - | - | - |
|
118 |
-
| Gemini-1.5-Pro | 75.0 / 81.3 | - | 1.30 | - | 64.0 | - |
|
119 |
-
| VideoLLaMA2-72B | 61.4 / 63.1 | 62.0 | - | - | - | - |
|
120 |
-
| LLaVA-OneVision-72B | 66.2 / 69.5 | 59.4 | - | 66.4 | 61.3 | - |
|
121 |
-
| Qwen2-VL-72B | 71.2 / 77.8 | 73.6 | 1.70 | - | - | 41.3 / 56.2 |
|
122 |
-
| InternVL2-Llama3-76B | 64.7 / 67.8 | 69.6 | 1.71 | 69.9 | 61.1 | - |
|
123 |
-
| **InternVL2.5-78B** | 72.1 / 74.0 | 76.4 | 1.97 | 75.7 | 63.6 | 42.2 / 58.5 |
|
124 |
|
125 |
-
|
|
|
|
|
|
|
|
|
|
|
|
|
|
|
|
|
|
|
|
|
|
|
|
|
|
|
|
|
|
|
|
|
|
|
|
|
|
|
|
|
|
|
|
|
|
|
|
|
|
|
|
|
|
|
|
|
|
|
|
|
|
|
|
|
|
|
|
|
|
|
|
|
|
|
|
|
|
|
|
|
|
|
|
|
|
|
|
|
|
|
|
|
|
|
|
|
|
|
|
|
|
|
|
|
|
|
|
|
|
|
|
|
|
|
|
|
|
|
|
|
|
|
|
|
|
|
|
|
|
|
|
|
|
|
|
|
126 |
|
127 |
-
|
128 |
-
<thead>
|
129 |
-
<tr>
|
130 |
-
<th rowspan="2">Model Name</th>
|
131 |
-
<th colspan="6">MMMB</th>
|
132 |
-
<th colspan="6">Multilingual MMBench</th>
|
133 |
-
<th>MTVQA</th>
|
134 |
-
</tr>
|
135 |
-
<tr>
|
136 |
-
<th>en</th>
|
137 |
-
<th>zh</th>
|
138 |
-
<th>pt</th>
|
139 |
-
<th>ar</th>
|
140 |
-
<th>tr</th>
|
141 |
-
<th>ru</th>
|
142 |
-
<th>en</th>
|
143 |
-
<th>zh</th>
|
144 |
-
<th>pt</th>
|
145 |
-
<th>ar</th>
|
146 |
-
<th>tr</th>
|
147 |
-
<th>ru</th>
|
148 |
-
<th>(avg)</th>
|
149 |
-
</tr>
|
150 |
-
</thead>
|
151 |
-
<tbody>
|
152 |
-
<tr>
|
153 |
-
<td>GPT-4V </td>
|
154 |
-
<td>75.0</td>
|
155 |
-
<td>74.2</td>
|
156 |
-
<td>71.5</td>
|
157 |
-
<td>73.5</td>
|
158 |
-
<td>69.0</td>
|
159 |
-
<td>73.1</td>
|
160 |
-
<td>77.6</td>
|
161 |
-
<td>74.4</td>
|
162 |
-
<td>72.5</td>
|
163 |
-
<td>72.3</td>
|
164 |
-
<td>70.5</td>
|
165 |
-
<td>74.8</td>
|
166 |
-
<td>22.0</td>
|
167 |
-
</tr>
|
168 |
-
<tr>
|
169 |
-
<td>GPT-4o </td>
|
170 |
-
<td>--</td>
|
171 |
-
<td>--</td>
|
172 |
-
<td>--</td>
|
173 |
-
<td>--</td>
|
174 |
-
<td>--</td>
|
175 |
-
<td>--</td>
|
176 |
-
<td>--</td>
|
177 |
-
<td>--</td>
|
178 |
-
<td>--</td>
|
179 |
-
<td>--</td>
|
180 |
-
<td>--</td>
|
181 |
-
<td>--</td>
|
182 |
-
<td>27.8</td>
|
183 |
-
</tr>
|
184 |
-
<tr>
|
185 |
-
<td>Qwen-VL-Max </td>
|
186 |
-
<td>77.2</td>
|
187 |
-
<td>75.3</td>
|
188 |
-
<td>72.2</td>
|
189 |
-
<td>70.8</td>
|
190 |
-
<td>66.0</td>
|
191 |
-
<td>74.2</td>
|
192 |
-
<td>76.8</td>
|
193 |
-
<td>77.6</td>
|
194 |
-
<td>74.6</td>
|
195 |
-
<td>75.0</td>
|
196 |
-
<td>69.1</td>
|
197 |
-
<td>75.0</td>
|
198 |
-
<td>--</td>
|
199 |
-
</tr>
|
200 |
-
<tr>
|
201 |
-
<td>Gemini-1.0-Pro </td>
|
202 |
-
<td>75.0</td>
|
203 |
-
<td>71.9</td>
|
204 |
-
<td>70.6</td>
|
205 |
-
<td>69.9</td>
|
206 |
-
<td>69.6</td>
|
207 |
-
<td>72.7</td>
|
208 |
-
<td>73.6</td>
|
209 |
-
<td>72.1</td>
|
210 |
-
<td>70.3</td>
|
211 |
-
<td>61.1</td>
|
212 |
-
<td>69.8</td>
|
213 |
-
<td>70.5</td>
|
214 |
-
<td>--</td>
|
215 |
-
</tr>
|
216 |
-
<tr>
|
217 |
-
<td>Qwen2-VL-72B </td>
|
218 |
-
<td>86.8</td>
|
219 |
-
<td>85.3</td>
|
220 |
-
<td>85.2</td>
|
221 |
-
<td>84.8</td>
|
222 |
-
<td>84.2</td>
|
223 |
-
<td>85.3</td>
|
224 |
-
<td>86.9</td>
|
225 |
-
<td>87.2</td>
|
226 |
-
<td>85.8</td>
|
227 |
-
<td>83.5</td>
|
228 |
-
<td>84.4</td>
|
229 |
-
<td>85.3</td>
|
230 |
-
<td>30.9</td>
|
231 |
-
</tr>
|
232 |
-
<tr>
|
233 |
-
<td>InternVL2-Llama3-76B </td>
|
234 |
-
<td>85.3</td>
|
235 |
-
<td>85.1</td>
|
236 |
-
<td>82.8</td>
|
237 |
-
<td>82.8</td>
|
238 |
-
<td>83.0</td>
|
239 |
-
<td>83.7</td>
|
240 |
-
<td>87.8</td>
|
241 |
-
<td>87.3</td>
|
242 |
-
<td>85.9</td>
|
243 |
-
<td>83.1</td>
|
244 |
-
<td>85.0</td>
|
245 |
-
<td>85.7</td>
|
246 |
-
<td>22.0</td>
|
247 |
-
</tr>
|
248 |
-
<tr>
|
249 |
-
<td>InternVL2.5-76B</td>
|
250 |
-
<td>86.3</td>
|
251 |
-
<td>85.6</td>
|
252 |
-
<td>85.1</td>
|
253 |
-
<td>84.8</td>
|
254 |
-
<td>83.1</td>
|
255 |
-
<td>85.4</td>
|
256 |
-
<td>90.0</td>
|
257 |
-
<td>89.7</td>
|
258 |
-
<td>87.4</td>
|
259 |
-
<td>83.3</td>
|
260 |
-
<td>84.9</td>
|
261 |
-
<td>86.3</td>
|
262 |
-
<td>31.9</td>
|
263 |
-
</tr>
|
264 |
-
</tbody>
|
265 |
-
</table>
|
266 |
|
|
|
|
|
|
|
|
|
|
|
|
|
|
|
|
|
|
|
|
|
|
|
|
|
|
|
|
|
|
|
|
|
|
|
|
|
|
|
|
|
|
|
|
|
|
|
|
|
|
|
|
|
|
|
|
|
|
|
|
|
|
|
|
|
|
|
|
|
|
|
|
|
|
|
|
|
|
|
|
|
|
|
|
|
|
|
|
|
|
|
|
|
|
|
|
|
|
|
|
|
|
|
|
|
|
|
|
|
267 |
|
268 |
### Visual Grounding
|
269 |
|
270 |
-
|
271 |
-
<thead>
|
272 |
-
<tr>
|
273 |
-
<th rowspan="2">Model Name</th>
|
274 |
-
<th colspan="3">RefCOCO</th>
|
275 |
-
<th colspan="3">RefCOCO+</th>
|
276 |
-
<th colspan="2">RefCOCOg</th>
|
277 |
-
<th rowspan="2">avg</th>
|
278 |
-
</tr>
|
279 |
-
<tr>
|
280 |
-
<th>val</th>
|
281 |
-
<th>test-A</th>
|
282 |
-
<th>test-B</th>
|
283 |
-
<th>val</th>
|
284 |
-
<th>test-A</th>
|
285 |
-
<th>test-B</th>
|
286 |
-
<th>val</th>
|
287 |
-
<th>test</th>
|
288 |
-
</tr>
|
289 |
-
</thead>
|
290 |
-
<tbody>
|
291 |
-
<tr>
|
292 |
-
<td>Grounding-DINO-L</td>
|
293 |
-
<td>90.6</td>
|
294 |
-
<td>93.2</td>
|
295 |
-
<td>88.2</td>
|
296 |
-
<td>82.8</td>
|
297 |
-
<td>89.0</td>
|
298 |
-
<td>75.9</td>
|
299 |
-
<td>86.1</td>
|
300 |
-
<td>87.0</td>
|
301 |
-
<td>86.6</td>
|
302 |
-
</tr>
|
303 |
-
<tr>
|
304 |
-
<td>UNINEXT-H</td>
|
305 |
-
<td>92.6</td>
|
306 |
-
<td>94.3</td>
|
307 |
-
<td>91.5</td>
|
308 |
-
<td>85.2</td>
|
309 |
-
<td>89.6</td>
|
310 |
-
<td>79.8</td>
|
311 |
-
<td>88.7</td>
|
312 |
-
<td>89.4</td>
|
313 |
-
<td>88.9</td>
|
314 |
-
</tr>
|
315 |
-
<tr>
|
316 |
-
<td>ONE-PEACE</td>
|
317 |
-
<td>92.6</td>
|
318 |
-
<td>94.2</td>
|
319 |
-
<td>89.3</td>
|
320 |
-
<td>88.8</td>
|
321 |
-
<td>92.2</td>
|
322 |
-
<td>83.2</td>
|
323 |
-
<td>89.2</td>
|
324 |
-
<td>89.3</td>
|
325 |
-
<td>89.8</td>
|
326 |
-
</tr>
|
327 |
-
<tr>
|
328 |
-
<td>Qwen2-VL-72B</td>
|
329 |
-
<td>93.2</td>
|
330 |
-
<td>95.3</td>
|
331 |
-
<td>90.7</td>
|
332 |
-
<td>90.1</td>
|
333 |
-
<td>93.8</td>
|
334 |
-
<td>85.6</td>
|
335 |
-
<td>89.9</td>
|
336 |
-
<td>90.4</td>
|
337 |
-
<td>91.1</td>
|
338 |
-
</tr>
|
339 |
-
<tr>
|
340 |
-
<td>InternVL2-Llama3-76B</td>
|
341 |
-
<td>92.2</td>
|
342 |
-
<td>94.8</td>
|
343 |
-
<td>88.4</td>
|
344 |
-
<td>88.8</td>
|
345 |
-
<td>93.1</td>
|
346 |
-
<td>82.8</td>
|
347 |
-
<td>89.5</td>
|
348 |
-
<td>90.3</td>
|
349 |
-
<td>90.0</td>
|
350 |
-
</tr>
|
351 |
-
<tr>
|
352 |
-
<td>InternVL2.5-78B</td>
|
353 |
-
<td>93.7</td>
|
354 |
-
<td>95.6</td>
|
355 |
-
<td>92.5</td>
|
356 |
-
<td>90.4</td>
|
357 |
-
<td>94.7</td>
|
358 |
-
<td>86.9</td>
|
359 |
-
<td>92.7</td>
|
360 |
-
<td>92.2</td>
|
361 |
-
<td>92.3</td>
|
362 |
-
</tr>
|
363 |
-
</tbody>
|
364 |
-
</table>
|
365 |
-
|
366 |
-
|
367 |
-
### Invitation to Evaluate InternVL
|
368 |
-
|
369 |
-
We welcome MLLM benchmark developers to assess our InternVL series models. If you need to add your evaluation results here, please contact me at [[email protected]](mailto:[email protected]).
|
370 |
|
371 |
-
|
|
|
|
|
|
|
|
|
|
|
|
|
372 |
|
373 |
-
|
374 |
|
375 |
-
|
376 |
|
377 |
-
|
|
|
|
|
|
|
|
|
|
|
|
|
378 |
|
379 |
### Model Loading
|
380 |
|
@@ -407,21 +211,6 @@ model = AutoModel.from_pretrained(
|
|
407 |
trust_remote_code=True).eval()
|
408 |
```
|
409 |
|
410 |
-
#### BNB 4-bit Quantization
|
411 |
-
|
412 |
-
```python
|
413 |
-
import torch
|
414 |
-
from transformers import AutoTokenizer, AutoModel
|
415 |
-
path = "OpenGVLab/InternVL2_5-78B"
|
416 |
-
model = AutoModel.from_pretrained(
|
417 |
-
path,
|
418 |
-
torch_dtype=torch.bfloat16,
|
419 |
-
load_in_4bit=True,
|
420 |
-
low_cpu_mem_usage=True,
|
421 |
-
use_flash_attn=True,
|
422 |
-
trust_remote_code=True).eval()
|
423 |
-
```
|
424 |
-
|
425 |
#### Multiple GPUs
|
426 |
|
427 |
The reason for writing the code this way is to avoid errors that occur during multi-GPU inference due to tensors not being on the same device. By ensuring that the first and last layers of the large language model (LLM) are on the same device, we prevent such errors.
|
@@ -435,7 +224,7 @@ def split_model(model_name):
|
|
435 |
device_map = {}
|
436 |
world_size = torch.cuda.device_count()
|
437 |
num_layers = {
|
438 |
-
'InternVL2_5-1B': 24, '
|
439 |
'InternVL2_5-26B': 48, 'InternVL2_5-38B': 64, 'InternVL2_5-78B': 80}[model_name]
|
440 |
# Since the first GPU will be used for ViT, treat it as half a GPU.
|
441 |
num_layers_per_gpu = math.ceil(num_layers / (world_size - 0.5))
|
@@ -471,6 +260,7 @@ model = AutoModel.from_pretrained(
|
|
471 |
### Inference with Transformers
|
472 |
|
473 |
```python
|
|
|
474 |
import numpy as np
|
475 |
import torch
|
476 |
import torchvision.transforms as T
|
@@ -553,14 +343,44 @@ def load_image(image_file, input_size=448, max_num=12):
|
|
553 |
pixel_values = torch.stack(pixel_values)
|
554 |
return pixel_values
|
555 |
|
556 |
-
|
|
|
|
|
|
|
|
|
|
|
|
|
|
|
|
|
|
|
|
|
|
|
|
|
|
|
|
|
|
|
|
|
|
|
|
|
|
|
|
|
|
|
|
|
|
|
|
|
|
|
|
|
|
|
557 |
path = 'OpenGVLab/InternVL2_5-78B'
|
|
|
558 |
model = AutoModel.from_pretrained(
|
559 |
path,
|
560 |
torch_dtype=torch.bfloat16,
|
|
|
561 |
low_cpu_mem_usage=True,
|
562 |
use_flash_attn=True,
|
563 |
-
trust_remote_code=True
|
|
|
564 |
tokenizer = AutoTokenizer.from_pretrained(path, trust_remote_code=True, use_fast=False)
|
565 |
|
566 |
# set the max number of tiles in `max_num`
|
@@ -680,13 +500,13 @@ response, history = model.chat(tokenizer, pixel_values, question, generation_con
|
|
680 |
num_patches_list=num_patches_list, history=None, return_history=True)
|
681 |
print(f'User: {question}\nAssistant: {response}')
|
682 |
|
683 |
-
question = 'Describe this video in detail.
|
684 |
response, history = model.chat(tokenizer, pixel_values, question, generation_config,
|
685 |
num_patches_list=num_patches_list, history=history, return_history=True)
|
686 |
print(f'User: {question}\nAssistant: {response}')
|
687 |
```
|
688 |
|
689 |
-
#### Streaming
|
690 |
|
691 |
Besides this method, you can also use the following code to get streamed output.
|
692 |
|
@@ -731,7 +551,7 @@ pip install lmdeploy>=0.5.3
|
|
731 |
|
732 |
LMDeploy abstracts the complex inference process of multi-modal Vision-Language Models (VLM) into an easy-to-use pipeline, similar to the Large Language Model (LLM) inference pipeline.
|
733 |
|
734 |
-
#### A 'Hello, world'
|
735 |
|
736 |
```python
|
737 |
from lmdeploy import pipeline, TurbomindEngineConfig
|
@@ -739,18 +559,18 @@ from lmdeploy.vl import load_image
|
|
739 |
|
740 |
model = 'OpenGVLab/InternVL2_5-78B'
|
741 |
image = load_image('https://raw.githubusercontent.com/open-mmlab/mmdeploy/main/tests/data/tiger.jpeg')
|
742 |
-
pipe = pipeline(model, backend_config=TurbomindEngineConfig(session_len=8192))
|
743 |
response = pipe(('describe this image', image))
|
744 |
print(response.text)
|
745 |
```
|
746 |
|
747 |
If `ImportError` occurs while executing this case, please install the required dependency packages as prompted.
|
748 |
|
749 |
-
#### Multi-images
|
750 |
|
751 |
When dealing with multiple images, you can put them all in one list. Keep in mind that multiple images will lead to a higher number of input tokens, and as a result, the size of the context window typically needs to be increased.
|
752 |
|
753 |
-
|
754 |
|
755 |
```python
|
756 |
from lmdeploy import pipeline, TurbomindEngineConfig
|
@@ -758,7 +578,7 @@ from lmdeploy.vl import load_image
|
|
758 |
from lmdeploy.vl.constants import IMAGE_TOKEN
|
759 |
|
760 |
model = 'OpenGVLab/InternVL2_5-78B'
|
761 |
-
pipe = pipeline(model, backend_config=TurbomindEngineConfig(session_len=8192))
|
762 |
|
763 |
image_urls=[
|
764 |
'https://raw.githubusercontent.com/open-mmlab/mmdeploy/main/demo/resources/human-pose.jpg',
|
@@ -771,7 +591,7 @@ response = pipe((f'Image-1: {IMAGE_TOKEN}\nImage-2: {IMAGE_TOKEN}\ndescribe thes
|
|
771 |
print(response.text)
|
772 |
```
|
773 |
|
774 |
-
#### Batch
|
775 |
|
776 |
Conducting inference with batch prompts is quite straightforward; just place them within a list structure:
|
777 |
|
@@ -780,7 +600,7 @@ from lmdeploy import pipeline, TurbomindEngineConfig
|
|
780 |
from lmdeploy.vl import load_image
|
781 |
|
782 |
model = 'OpenGVLab/InternVL2_5-78B'
|
783 |
-
pipe = pipeline(model, backend_config=TurbomindEngineConfig(session_len=8192))
|
784 |
|
785 |
image_urls=[
|
786 |
"https://raw.githubusercontent.com/open-mmlab/mmdeploy/main/demo/resources/human-pose.jpg",
|
@@ -791,7 +611,7 @@ response = pipe(prompts)
|
|
791 |
print(response)
|
792 |
```
|
793 |
|
794 |
-
#### Multi-turn
|
795 |
|
796 |
There are two ways to do the multi-turn conversations with the pipeline. One is to construct messages according to the format of OpenAI and use above introduced method, the other is to use the `pipeline.chat` interface.
|
797 |
|
@@ -800,7 +620,7 @@ from lmdeploy import pipeline, TurbomindEngineConfig, GenerationConfig
|
|
800 |
from lmdeploy.vl import load_image
|
801 |
|
802 |
model = 'OpenGVLab/InternVL2_5-78B'
|
803 |
-
pipe = pipeline(model, backend_config=TurbomindEngineConfig(session_len=8192))
|
804 |
|
805 |
image = load_image('https://raw.githubusercontent.com/open-mmlab/mmdeploy/main/demo/resources/human-pose.jpg')
|
806 |
gen_config = GenerationConfig(top_k=40, top_p=0.8, temperature=0.8)
|
@@ -815,7 +635,7 @@ print(sess.response.text)
|
|
815 |
LMDeploy's `api_server` enables models to be easily packed into services with a single command. The provided RESTful APIs are compatible with OpenAI's interfaces. Below are an example of service startup:
|
816 |
|
817 |
```shell
|
818 |
-
lmdeploy serve api_server OpenGVLab/InternVL2_5-78B --backend turbomind --server-port 23333
|
819 |
```
|
820 |
|
821 |
To use the OpenAI-style interface, you need to install OpenAI:
|
@@ -854,18 +674,18 @@ print(response)
|
|
854 |
|
855 |
## License
|
856 |
|
857 |
-
This project is released under the MIT
|
858 |
|
859 |
## Citation
|
860 |
|
861 |
If you find this project useful in your research, please consider citing:
|
862 |
|
863 |
```BibTeX
|
864 |
-
@article{
|
865 |
-
title={
|
866 |
-
author={
|
867 |
-
journal={arXiv preprint arXiv:
|
868 |
-
year={
|
869 |
}
|
870 |
@article{chen2024far,
|
871 |
title={How Far Are We to GPT-4V? Closing the Gap to Commercial Multimodal Models with Open-Source Suites},
|
@@ -873,5 +693,10 @@ If you find this project useful in your research, please consider citing:
|
|
873 |
journal={arXiv preprint arXiv:2404.16821},
|
874 |
year={2024}
|
875 |
}
|
|
|
|
|
|
|
|
|
|
|
|
|
876 |
```
|
877 |
-
|
|
|
1 |
---
|
2 |
+
license: other
|
3 |
+
license_name: qwen
|
4 |
+
license_link: https://huggingface.co/Qwen/Qwen2.5-72B-Instruct/blob/main/LICENSE
|
5 |
pipeline_tag: image-text-to-text
|
6 |
library_name: transformers
|
7 |
base_model:
|
|
|
12 |
- multilingual
|
13 |
tags:
|
14 |
- internvl
|
|
|
|
|
|
|
|
|
15 |
- custom_code
|
16 |
---
|
17 |
|
18 |
# InternVL2_5-78B
|
19 |
|
20 |
+
[\[📂 GitHub\]](https://github.com/OpenGVLab/InternVL) [\[🆕 Blog\]](https://internvl.github.io/blog/) [\[📜 InternVL 1.0\]](https://arxiv.org/abs/2312.14238) [\[📜 InternVL 1.5\]](https://arxiv.org/abs/2404.16821) [\[📜 InternVL 2.5\]](https://github.com/OpenGVLab/InternVL/blob/main/InternVL2_5_report.pdf)
|
|
|
|
|
|
|
21 |
|
22 |
+
[\[🗨️ Chat Demo\]](https://internvl.opengvlab.com/) [\[🤗 HF Demo\]](https://huggingface.co/spaces/OpenGVLab/InternVL) [\[🚀 Quick Start\]](#quick-start) [\[📖 Documents\]](https://internvl.readthedocs.io/en/latest/)
|
23 |
|
24 |
+
<div align="center">
|
25 |
+
<img width="500" alt="image" src="https://cdn-uploads.huggingface.co/production/uploads/64006c09330a45b03605bba3/zJsd2hqd3EevgXo6fNgC-.png">
|
26 |
+
</div>
|
27 |
|
28 |
## Introduction
|
29 |
|
30 |
+
We are excited to introduce **InternVL 2.5**, an advanced multimodal large language model (MLLM) series that builds upon InternVL 2.0, maintaining its core model architecture while introducing significant enhancements in training and testing strategies as well as data quality.
|
|
|
|
|
|
|
|
|
|
|
|
|
|
|
|
|
|
|
|
|
|
|
|
|
|
|
|
|
|
|
|
|
|
|
|
|
|
|
|
|
|
|
|
|
|
|
|
|
|
|
|
|
|
|
|
|
|
|
|
|
|
|
|
|
|
|
|
|
|
|
|
|
|
|
|
|
|
|
|
|
|
|
|
|
|
|
|
|
|
|
|
|
|
|
|
|
|
|
|
|
|
|
|
|
|
|
|
|
|
|
|
|
|
|
|
|
|
|
|
|
|
|
|
|
|
|
|
|
|
|
|
|
|
|
|
|
|
|
|
|
|
|
|
|
|
|
|
|
|
|
|
|
|
|
|
|
|
|
|
|
|
|
|
|
|
|
|
|
|
|
|
|
|
|
|
|
|
|
|
|
|
|
31 |
|
32 |
+

|
33 |
+
|
34 |
+
## InternVL 2.5 Family
|
35 |
+
|
36 |
+
In the following table, we provide an overview of the InternVL 2.5 series.
|
37 |
+
|
38 |
+
| Model Name | Vision Part | Language Part | HF Link |
|
39 |
+
| :-------------: | :-------------------------------------------------------------------------------------: | :----------------------------------------------------------------------------: | :---------------------------------------------------------: |
|
40 |
+
| InternVL2_5-1B | [InternViT-300M-448px-V2_5](https://huggingface.co/OpenGVLab/InternViT-300M-448px-V2_5) | [Qwen2.5-0.5B-Instruct](https://huggingface.co/Qwen/Qwen2.5-0.5B-Instruct) | [🤗 link](https://huggingface.co/OpenGVLab/InternVL2_5-1B) |
|
41 |
+
| InternVL2_5-2B | [InternViT-300M-448px-V2_5](https://huggingface.co/OpenGVLab/InternViT-300M-448px-V2_5) | [internlm2_5-1_8b-chat](https://huggingface.co/internlm/internlm2_5-1_8b-chat) | [🤗 link](https://huggingface.co/OpenGVLab/InternVL2_5-2B) |
|
42 |
+
| InternVL2_5-4B | [InternViT-300M-448px-V2_5](https://huggingface.co/OpenGVLab/InternViT-300M-448px-V2_5) | [Qwen2.5-3B-Instruct](https://huggingface.co/Qwen/Qwen2.5-3B-Instruct) | [🤗 link](https://huggingface.co/OpenGVLab/InternVL2_5-4B) |
|
43 |
+
| InternVL2_5-8B | [InternViT-300M-448px-V2_5](https://huggingface.co/OpenGVLab/InternViT-300M-448px-V2_5) | [internlm2_5-7b-chat](https://huggingface.co/internlm/internlm2_5-7b-chat) | [🤗 link](https://huggingface.co/OpenGVLab/InternVL2_5-8B) |
|
44 |
+
| InternVL2_5-26B | [InternViT-6B-448px-V2_5](https://huggingface.co/OpenGVLab/InternViT-6B-448px-V2_5) | [internlm2_5-20b-chat](https://huggingface.co/internlm/internlm2_5-20b-chat) | [🤗 link](https://huggingface.co/OpenGVLab/InternVL2_5-26B) |
|
45 |
+
| InternVL2_5-38B | [InternViT-6B-448px-V2_5](https://huggingface.co/OpenGVLab/InternViT-6B-448px-V2_5) | [Qwen2.5-32B-Instruct](https://huggingface.co/Qwen/Qwen2.5-32B-Instruct) | [🤗 link](https://huggingface.co/OpenGVLab/InternVL2_5-38B) |
|
46 |
+
| InternVL2_5-78B | [InternViT-6B-448px-V2_5](https://huggingface.co/OpenGVLab/InternViT-6B-448px-V2_5) | [Qwen2.5-72B-Instruct](https://huggingface.co/Qwen/Qwen2.5-72B-Instruct) | [🤗 link](https://huggingface.co/OpenGVLab/InternVL2_5-78B) |
|
47 |
+
|
48 |
+
## Model Architecture
|
49 |
+
|
50 |
+
As shown in the following figure, InternVL 2.5 retains the same model architecture as its predecessors, InternVL 1.5 and 2.0, following the "ViT-MLP-LLM" paradigm. In this new version, we integrate a newly incrementally pre-trained InternViT with various pre-trained LLMs, including InternLM 2.5 and Qwen 2.5, using a randomly initialized MLP projector.
|
51 |
+
|
52 |
+

|
53 |
+
|
54 |
+
As in the previous version, we applied a pixel unshuffle operation, reducing the number of visual tokens to one-quarter of the original. Besides, we adopted a similar dynamic resolution strategy as InternVL 1.5, dividing images into tiles of 448×448 pixels. The key difference, starting from InternVL 2.0, is that we additionally introduced support for multi-image and video data.
|
55 |
+
|
56 |
+
## Training Strategy
|
57 |
+
|
58 |
+
### Dynamic High-Resolution for Multimodal Data
|
59 |
+
|
60 |
+
In InternVL 2.0 and 2.5, we extend the dynamic high-resolution training approach, enhancing its capabilities to handle multi-image and video datasets.
|
61 |
+
|
62 |
+

|
63 |
+
|
64 |
+
- For single-image datasets, the total number of tiles `n_max` are allocated to a single image for maximum resolution. Visual tokens are enclosed in `<img>` and `</img>` tags.
|
65 |
+
|
66 |
+
- For multi-image datasets, the total number of tiles `n_max` are distributed across all images in a sample. Each image is labeled with auxiliary tags like `Image-1` and enclosed in `<img>` and `</img>` tags.
|
67 |
+
|
68 |
+
- For videos, each frame is resized to 448×448. Frames are labeled with tags like `Frame-1` and enclosed in `<img>` and `</img>` tags, similar to images.
|
69 |
+
|
70 |
+
### Single Model Training Pipeline
|
71 |
+
|
72 |
+
The training pipeline for a single model in InternVL 2.5 is structured across three stages, designed to enhance the model's visual perception and multimodal capabilities.
|
73 |
+
|
74 |
+

|
75 |
+
|
76 |
+
- **Stage 1: MLP Warmup.** In this stage, only the MLP projector is trained while the vision encoder and language model are frozen. A dynamic high-resolution training strategy is applied for better performance, despite increased cost. This phase ensures robust cross-modal alignment and prepares the model for stable multimodal training.
|
77 |
+
|
78 |
+
- **Stage 1.5: ViT Incremental Learning (Optional).** This stage allows incremental training of the vision encoder and MLP projector using the same data as Stage 1. It enhances the encoder’s ability to handle rare domains like multilingual OCR and mathematical charts. Once trained, the encoder can be reused across LLMs without retraining, making this stage optional unless new domains are introduced.
|
79 |
+
|
80 |
+
- **Stage 2: Full Model Instruction Tuning.** The entire model is trained on high-quality multimodal instruction datasets. Strict data quality controls are enforced to prevent degradation of the LLM, as noisy data can cause issues like repetitive or incorrect outputs. After this stage, the training process is complete.
|
81 |
+
|
82 |
+
### Progressive Scaling Strategy
|
83 |
+
|
84 |
+
We introduce a progressive scaling strategy to align the vision encoder with LLMs efficiently. This approach trains with smaller LLMs first (e.g., 20B) to optimize foundational visual capabilities and cross-modal alignment before transferring the vision encoder to larger LLMs (e.g., 72B) without retraining. This reuse skips intermediate stages for larger models.
|
85 |
+
|
86 |
+

|
87 |
+
|
88 |
+
Compared to Qwen2-VL's 1.4 trillion tokens, InternVL2.5-78B uses only 120 billion tokens—less than one-tenth. This strategy minimizes redundancy, maximizes pre-trained component reuse, and enables efficient training for complex vision-language tasks.
|
89 |
+
|
90 |
+
### Training Enhancements
|
91 |
+
|
92 |
+
To improve real-world adaptability and performance, we introduce two key techniques:
|
93 |
+
|
94 |
+
- **Random JPEG Compression**: Random JPEG compression with quality levels between 75 and 100 is applied as a data augmentation technique. This simulates image degradation from internet sources, enhancing the model's robustness to noisy images.
|
95 |
+
|
96 |
+
- **Loss Reweighting**: To balance the NTP loss across responses of different lengths, we use a reweighting strategy called **square averaging**. This method balances contributions from responses of varying lengths, mitigating biases toward longer or shorter responses.
|
97 |
+
|
98 |
+
### Data Organization
|
99 |
+
|
100 |
+
#### Dataset Configuration
|
101 |
|
102 |
+
In InternVL 2.0 and 2.5, the organization of the training data is controlled by several key parameters to optimize the balance and distribution of datasets during training.
|
|
|
|
|
|
|
|
|
|
|
|
|
|
|
|
|
|
|
|
|
|
|
|
|
|
|
|
|
|
|
|
|
|
|
|
|
|
|
|
|
|
|
|
|
|
|
|
|
|
|
|
|
|
|
|
|
|
|
|
|
|
|
|
|
|
|
|
|
|
|
|
|
|
|
|
|
|
|
|
|
|
|
|
|
|
|
|
|
|
|
|
|
|
|
|
|
|
|
|
|
|
|
|
|
|
|
|
|
|
|
|
|
|
|
|
|
|
|
|
|
|
|
|
|
|
|
|
|
|
|
|
|
|
|
|
|
|
|
|
|
|
|
|
|
|
|
|
|
|
|
|
|
|
|
|
|
|
|
|
|
|
|
|
|
|
|
|
|
|
|
|
|
|
|
|
|
|
|
|
|
|
|
|
|
|
|
|
|
|
|
|
|
|
|
|
|
|
|
|
|
|
|
|
|
|
|
|
|
|
|
|
|
|
|
|
|
|
|
|
|
|
|
|
|
|
|
|
|
|
|
|
|
|
|
|
|
|
|
|
|
|
|
|
|
|
|
|
|
|
|
|
|
|
|
|
|
|
|
|
|
|
|
|
|
|
|
|
|
|
|
|
|
|
|
|
|
103 |
|
104 |
+

|
105 |
+
|
106 |
+
- **Data Augmentation:** JPEG compression is applied conditionally: enabled for image datasets to enhance robustness and disabled for video datasets to maintain consistent frame quality.
|
107 |
+
|
108 |
+
- **Maximum Tile Number:** The parameter `n_max` controls the maximum tiles per dataset. For example, higher values (24–36) are used for multi-image or high-resolution data, lower values (6–12) for standard images, and 1 for videos.
|
109 |
+
|
110 |
+
- **Repeat Factor:** The repeat factor `r` adjusts dataset sampling frequency. Values below 1 reduce a dataset's weight, while values above 1 increase it. This ensures balanced training across tasks and prevents overfitting or underfitting.
|
111 |
+
|
112 |
+
#### Data Filtering Pipeline
|
113 |
+
|
114 |
+
During development, we found that LLMs are highly sensitive to data noise, with even small anomalies—like outliers or repetitive data—causing abnormal behavior during inference. Repetitive generation, especially in long-form or CoT reasoning tasks, proved particularly harmful.
|
115 |
+
|
116 |
+

|
117 |
+
|
118 |
+
To address this challenge and support future research, we designed an efficient data filtering pipeline to remove low-quality samples.
|
119 |
+
|
120 |
+

|
121 |
+
|
122 |
+
The pipeline includes two modules, for **pure-text data**, three key strategies are used:
|
123 |
+
|
124 |
+
1. **LLM-Based Quality Scoring**: Each sample is scored (0–10) using a pre-trained LLM with domain-specific prompts. Samples scoring below a threshold (e.g., 7) are removed to ensure high-quality data.
|
125 |
+
2. **Repetition Detection**: Repetitive samples are flagged using LLM-based prompts and manually reviewed. Samples scoring below a stricter threshold (e.g., 3) are excluded to avoid repetitive patterns.
|
126 |
+
3. **Heuristic Rule-Based Filtering**: Anomalies like abnormal sentence lengths or duplicate lines are detected using rules. Flagged samples undergo manual verification to ensure accuracy before removal.
|
127 |
+
|
128 |
+
For **multimodal data**, two strategies are used:
|
129 |
+
|
130 |
+
1. **Repetition Detection**: Repetitive samples in non-academic datasets are flagged and manually reviewed to prevent pattern loops. High-quality datasets are exempt from this process.
|
131 |
+
2. **Heuristic Rule-Based Filtering**: Similar rules are applied to detect visual anomalies, with flagged data verified manually to maintain integrity.
|
132 |
+
|
133 |
+
#### Training Data
|
134 |
+
|
135 |
+
As shown in the following figure, from InternVL 1.5 to 2.0 and then to 2.5, the fine-tuning data mixture has undergone iterative improvements in scale, quality, and diversity. For more information about the training data, please refer to our technical report.
|
136 |
+
|
137 |
+

|
138 |
+
|
139 |
+
## Evaluation on Multimodal Capability
|
140 |
+
|
141 |
+
### Multimodal Reasoning and Mathematics
|
142 |
+
|
143 |
+

|
144 |
+
|
145 |
+

|
146 |
+
|
147 |
+
### OCR, Chart, and Document Understanding
|
148 |
+
|
149 |
+

|
150 |
+
|
151 |
+
### Multi-Image & Real-World Comprehension
|
152 |
+
|
153 |
+

|
154 |
+
|
155 |
+
### Comprehensive Multimodal & Hallucination Evaluation
|
156 |
+
|
157 |
+

|
158 |
|
159 |
### Visual Grounding
|
160 |
|
161 |
+

|
|
|
|
|
|
|
|
|
|
|
|
|
|
|
|
|
|
|
|
|
|
|
|
|
|
|
|
|
|
|
|
|
|
|
|
|
|
|
|
|
|
|
|
|
|
|
|
|
|
|
|
|
|
|
|
|
|
|
|
|
|
|
|
|
|
|
|
|
|
|
|
|
|
|
|
|
|
|
|
|
|
|
|
|
|
|
|
|
|
|
|
|
|
|
|
|
|
|
|
|
|
|
|
|
|
|
|
|
|
|
|
|
|
|
|
|
|
|
|
|
|
|
|
|
|
|
|
|
|
|
|
|
|
|
|
|
|
|
|
|
|
|
|
|
|
|
|
|
|
|
|
|
|
|
|
|
|
|
|
|
|
|
|
|
|
|
|
|
|
|
|
|
|
|
|
|
|
|
|
|
|
|
|
|
|
|
|
|
|
|
|
|
|
|
|
|
|
|
162 |
|
163 |
+
### Multimodal Multilingual Understanding
|
164 |
+
|
165 |
+

|
166 |
+
|
167 |
+
### Video Understanding
|
168 |
+
|
169 |
+

|
170 |
|
171 |
+
## Evaluation on Language Capability
|
172 |
|
173 |
+
Training InternVL 2.0 models led to a decline in pure language capabilities. InternVL 2.5 addresses this by collecting more high-quality open-source data and filtering out low-quality data, achieving better preservation of pure language performance.
|
174 |
|
175 |
+
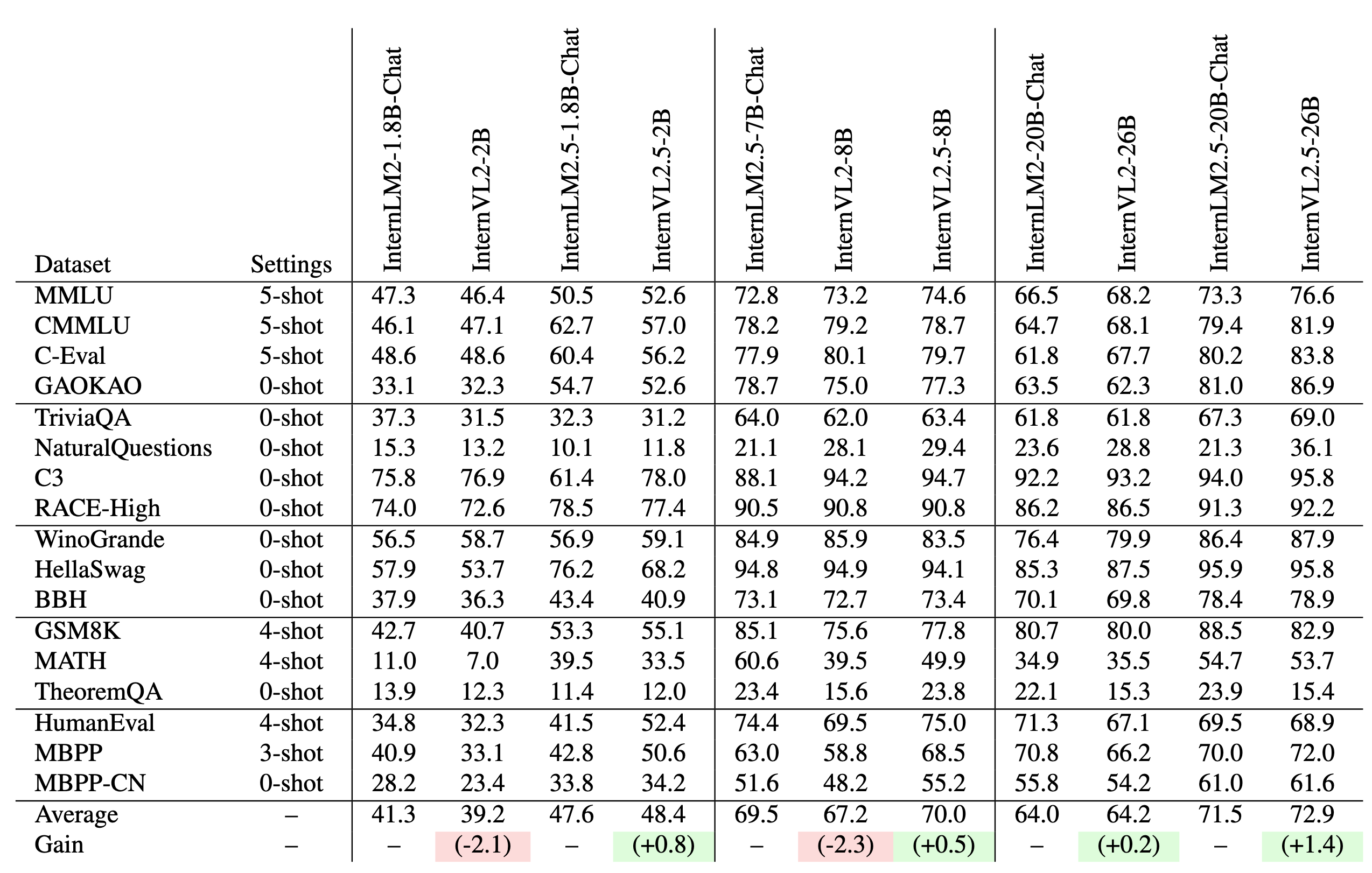
|
176 |
+
|
177 |
+
## Quick Start
|
178 |
+
|
179 |
+
We provide an example code to run `InternVL2_5-78B` using `transformers`.
|
180 |
+
|
181 |
+
> Please use transformers>=4.37.2 to ensure the model works normally.
|
182 |
|
183 |
### Model Loading
|
184 |
|
|
|
211 |
trust_remote_code=True).eval()
|
212 |
```
|
213 |
|
|
|
|
|
|
|
|
|
|
|
|
|
|
|
|
|
|
|
|
|
|
|
|
|
|
|
|
|
|
|
214 |
#### Multiple GPUs
|
215 |
|
216 |
The reason for writing the code this way is to avoid errors that occur during multi-GPU inference due to tensors not being on the same device. By ensuring that the first and last layers of the large language model (LLM) are on the same device, we prevent such errors.
|
|
|
224 |
device_map = {}
|
225 |
world_size = torch.cuda.device_count()
|
226 |
num_layers = {
|
227 |
+
'InternVL2_5-1B': 24, 'InternVL2_5-2B': 24, 'InternVL2_5-4B': 36, 'InternVL2_5-8B': 32,
|
228 |
'InternVL2_5-26B': 48, 'InternVL2_5-38B': 64, 'InternVL2_5-78B': 80}[model_name]
|
229 |
# Since the first GPU will be used for ViT, treat it as half a GPU.
|
230 |
num_layers_per_gpu = math.ceil(num_layers / (world_size - 0.5))
|
|
|
260 |
### Inference with Transformers
|
261 |
|
262 |
```python
|
263 |
+
import math
|
264 |
import numpy as np
|
265 |
import torch
|
266 |
import torchvision.transforms as T
|
|
|
343 |
pixel_values = torch.stack(pixel_values)
|
344 |
return pixel_values
|
345 |
|
346 |
+
def split_model(model_name):
|
347 |
+
device_map = {}
|
348 |
+
world_size = torch.cuda.device_count()
|
349 |
+
num_layers = {
|
350 |
+
'InternVL2_5-1B': 24, 'InternVL2_5-2B': 24, 'InternVL2_5-4B': 36, 'InternVL2_5-8B': 32,
|
351 |
+
'InternVL2_5-26B': 48, 'InternVL2_5-38B': 64, 'InternVL2_5-78B': 80}[model_name]
|
352 |
+
# Since the first GPU will be used for ViT, treat it as half a GPU.
|
353 |
+
num_layers_per_gpu = math.ceil(num_layers / (world_size - 0.5))
|
354 |
+
num_layers_per_gpu = [num_layers_per_gpu] * world_size
|
355 |
+
num_layers_per_gpu[0] = math.ceil(num_layers_per_gpu[0] * 0.5)
|
356 |
+
layer_cnt = 0
|
357 |
+
for i, num_layer in enumerate(num_layers_per_gpu):
|
358 |
+
for j in range(num_layer):
|
359 |
+
device_map[f'language_model.model.layers.{layer_cnt}'] = i
|
360 |
+
layer_cnt += 1
|
361 |
+
device_map['vision_model'] = 0
|
362 |
+
device_map['mlp1'] = 0
|
363 |
+
device_map['language_model.model.tok_embeddings'] = 0
|
364 |
+
device_map['language_model.model.embed_tokens'] = 0
|
365 |
+
device_map['language_model.output'] = 0
|
366 |
+
device_map['language_model.model.norm'] = 0
|
367 |
+
device_map['language_model.lm_head'] = 0
|
368 |
+
device_map[f'language_model.model.layers.{num_layers - 1}'] = 0
|
369 |
+
|
370 |
+
return device_map
|
371 |
+
|
372 |
+
# If you set `load_in_8bit=True`, you will need two 80GB GPUs.
|
373 |
+
# If you set `load_in_8bit=False`, you will need at least three 80GB GPUs.
|
374 |
path = 'OpenGVLab/InternVL2_5-78B'
|
375 |
+
device_map = split_model('InternVL2_5-78B')
|
376 |
model = AutoModel.from_pretrained(
|
377 |
path,
|
378 |
torch_dtype=torch.bfloat16,
|
379 |
+
load_in_8bit=True,
|
380 |
low_cpu_mem_usage=True,
|
381 |
use_flash_attn=True,
|
382 |
+
trust_remote_code=True,
|
383 |
+
device_map=device_map).eval()
|
384 |
tokenizer = AutoTokenizer.from_pretrained(path, trust_remote_code=True, use_fast=False)
|
385 |
|
386 |
# set the max number of tiles in `max_num`
|
|
|
500 |
num_patches_list=num_patches_list, history=None, return_history=True)
|
501 |
print(f'User: {question}\nAssistant: {response}')
|
502 |
|
503 |
+
question = 'Describe this video in detail.'
|
504 |
response, history = model.chat(tokenizer, pixel_values, question, generation_config,
|
505 |
num_patches_list=num_patches_list, history=history, return_history=True)
|
506 |
print(f'User: {question}\nAssistant: {response}')
|
507 |
```
|
508 |
|
509 |
+
#### Streaming Output
|
510 |
|
511 |
Besides this method, you can also use the following code to get streamed output.
|
512 |
|
|
|
551 |
|
552 |
LMDeploy abstracts the complex inference process of multi-modal Vision-Language Models (VLM) into an easy-to-use pipeline, similar to the Large Language Model (LLM) inference pipeline.
|
553 |
|
554 |
+
#### A 'Hello, world' Example
|
555 |
|
556 |
```python
|
557 |
from lmdeploy import pipeline, TurbomindEngineConfig
|
|
|
559 |
|
560 |
model = 'OpenGVLab/InternVL2_5-78B'
|
561 |
image = load_image('https://raw.githubusercontent.com/open-mmlab/mmdeploy/main/tests/data/tiger.jpeg')
|
562 |
+
pipe = pipeline(model, backend_config=TurbomindEngineConfig(session_len=8192, tp=4))
|
563 |
response = pipe(('describe this image', image))
|
564 |
print(response.text)
|
565 |
```
|
566 |
|
567 |
If `ImportError` occurs while executing this case, please install the required dependency packages as prompted.
|
568 |
|
569 |
+
#### Multi-images Inference
|
570 |
|
571 |
When dealing with multiple images, you can put them all in one list. Keep in mind that multiple images will lead to a higher number of input tokens, and as a result, the size of the context window typically needs to be increased.
|
572 |
|
573 |
+
question = 'Describe this video in detail.'
|
574 |
|
575 |
```python
|
576 |
from lmdeploy import pipeline, TurbomindEngineConfig
|
|
|
578 |
from lmdeploy.vl.constants import IMAGE_TOKEN
|
579 |
|
580 |
model = 'OpenGVLab/InternVL2_5-78B'
|
581 |
+
pipe = pipeline(model, backend_config=TurbomindEngineConfig(session_len=8192, tp=4))
|
582 |
|
583 |
image_urls=[
|
584 |
'https://raw.githubusercontent.com/open-mmlab/mmdeploy/main/demo/resources/human-pose.jpg',
|
|
|
591 |
print(response.text)
|
592 |
```
|
593 |
|
594 |
+
#### Batch Prompts Inference
|
595 |
|
596 |
Conducting inference with batch prompts is quite straightforward; just place them within a list structure:
|
597 |
|
|
|
600 |
from lmdeploy.vl import load_image
|
601 |
|
602 |
model = 'OpenGVLab/InternVL2_5-78B'
|
603 |
+
pipe = pipeline(model, backend_config=TurbomindEngineConfig(session_len=8192, tp=4))
|
604 |
|
605 |
image_urls=[
|
606 |
"https://raw.githubusercontent.com/open-mmlab/mmdeploy/main/demo/resources/human-pose.jpg",
|
|
|
611 |
print(response)
|
612 |
```
|
613 |
|
614 |
+
#### Multi-turn Conversation
|
615 |
|
616 |
There are two ways to do the multi-turn conversations with the pipeline. One is to construct messages according to the format of OpenAI and use above introduced method, the other is to use the `pipeline.chat` interface.
|
617 |
|
|
|
620 |
from lmdeploy.vl import load_image
|
621 |
|
622 |
model = 'OpenGVLab/InternVL2_5-78B'
|
623 |
+
pipe = pipeline(model, backend_config=TurbomindEngineConfig(session_len=8192, tp=4))
|
624 |
|
625 |
image = load_image('https://raw.githubusercontent.com/open-mmlab/mmdeploy/main/demo/resources/human-pose.jpg')
|
626 |
gen_config = GenerationConfig(top_k=40, top_p=0.8, temperature=0.8)
|
|
|
635 |
LMDeploy's `api_server` enables models to be easily packed into services with a single command. The provided RESTful APIs are compatible with OpenAI's interfaces. Below are an example of service startup:
|
636 |
|
637 |
```shell
|
638 |
+
lmdeploy serve api_server OpenGVLab/InternVL2_5-78B --backend turbomind --server-port 23333 --tp 4
|
639 |
```
|
640 |
|
641 |
To use the OpenAI-style interface, you need to install OpenAI:
|
|
|
674 |
|
675 |
## License
|
676 |
|
677 |
+
This project is released under the MIT License. This project uses the pre-trained Qwen2.5-72B-Instruct as a component, which is licensed under the Qwen License.
|
678 |
|
679 |
## Citation
|
680 |
|
681 |
If you find this project useful in your research, please consider citing:
|
682 |
|
683 |
```BibTeX
|
684 |
+
@article{gao2024mini,
|
685 |
+
title={Mini-internvl: A flexible-transfer pocket multimodal model with 5\% parameters and 90\% performance},
|
686 |
+
author={Gao, Zhangwei and Chen, Zhe and Cui, Erfei and Ren, Yiming and Wang, Weiyun and Zhu, Jinguo and Tian, Hao and Ye, Shenglong and He, Junjun and Zhu, Xizhou and others},
|
687 |
+
journal={arXiv preprint arXiv:2410.16261},
|
688 |
+
year={2024}
|
689 |
}
|
690 |
@article{chen2024far,
|
691 |
title={How Far Are We to GPT-4V? Closing the Gap to Commercial Multimodal Models with Open-Source Suites},
|
|
|
693 |
journal={arXiv preprint arXiv:2404.16821},
|
694 |
year={2024}
|
695 |
}
|
696 |
+
@article{chen2023internvl,
|
697 |
+
title={InternVL: Scaling up Vision Foundation Models and Aligning for Generic Visual-Linguistic Tasks},
|
698 |
+
author={Chen, Zhe and Wu, Jiannan and Wang, Wenhai and Su, Weijie and Chen, Guo and Xing, Sen and Zhong, Muyan and Zhang, Qinglong and Zhu, Xizhou and Lu, Lewei and Li, Bin and Luo, Ping and Lu, Tong and Qiao, Yu and Dai, Jifeng},
|
699 |
+
journal={arXiv preprint arXiv:2312.14238},
|
700 |
+
year={2023}
|
701 |
+
}
|
702 |
```
|
|