---
base_model: Sao10K/Llama-3.1-8B-Stheno-v3.4
quantized_by: Lewdiculous
library_name: transformers
license: cc-by-nc-4.0
inference: false
language:
- en
tags:
- roleplay
- llama3
- sillytavern
---
Quants for [**Sao10K/Llama-3.1-8B-Stheno-v3.4**](https://huggingface.co./Sao10K/Llama-3.1-8B-Stheno-v3.4).
I recommend checking their page for feedback and support.
> [!IMPORTANT]
> **Quantization process:**
> Imatrix data was generated from the FP16-GGUF and conversions directly from the BF16-GGUF.
> This hopefully avoids losses during conversion.
> To run this model, please use the [**latest version of KoboldCpp**](https://github.com/LostRuins/koboldcpp/releases/latest).
> If you noticed any issues let me know in the discussions.
> [!NOTE]
> **Presets:**
> Some compatible SillyTavern presets can be found [**here (Virt's Roleplay Presets - v1.9)**](https://huggingface.co./Virt-io/SillyTavern-Presets).
> Check [**discussions such as this one**](https://huggingface.co./Virt-io/SillyTavern-Presets/discussions/5#664d6fb87c563d4d95151baa) and [**this one**](https://www.reddit.com/r/SillyTavernAI/comments/1dff2tl/my_personal_llama3_stheno_presets/) for other presets and samplers recommendations.
> Lower temperatures are recommended by the authors, so make sure to experiment.
>
> **General usage with KoboldCpp:**
> For **8GB VRAM** GPUs, I recommend the **Q4_K_M-imat** (4.89 BPW) quant for up to 12288 context sizes without the use of `--quantkv`.
> Using `--quantkv 1` (≈Q8) or even `--quantkv 2` (≈Q4) can get you to 32K context sizes with the caveat of not being compatible with Context Shifting, only relevant if you can manage to fill up that much context.
> [**Read more about it in the release here**](https://github.com/LostRuins/koboldcpp/releases/tag/v1.67).

Click here for the original model card information.

---
Thanks to Backyard.ai for the compute to train this. :)
---
Llama-3.1-8B-Stheno-v3.4
This model has went through a multi-stage finetuning process.
```
- 1st, over a multi-turn Conversational-Instruct
- 2nd, over a Creative Writing / Roleplay along with some Creative-based Instruct Datasets.
- - Dataset consists of a mixture of Human and Claude Data.
```
Prompting Format:
```
- Use the L3 Instruct Formatting - Euryale 2.1 Preset Works Well
- Temperature + min_p as per usual, I recommend 1.4 Temp + 0.2 min_p.
- Has a different vibe to previous versions. Tinker around.
```
Changes since previous Stheno Datasets:
```
- Included Multi-turn Conversation-based Instruct Datasets to boost multi-turn coherency. # This is a seperate set, not the ones made by Kalomaze and Nopm, that are used in Magnum. They're completely different data.
- Replaced Single-Turn Instruct with Better Prompts and Answers by Claude 3.5 Sonnet and Claude 3 Opus.
- Removed c2 Samples -> Underway of re-filtering and masking to use with custom prefills. TBD
- Included 55% more Roleplaying Examples based of [Gryphe's](https://huggingface.co./datasets/Gryphe/Sonnet3.5-Charcard-Roleplay) Charcard RP Sets. Further filtered and cleaned on.
- Included 40% More Creative Writing Examples.
- Included Datasets Targeting System Prompt Adherence.
- Included Datasets targeting Reasoning / Spatial Awareness.
- Filtered for the usual errors, slop and stuff at the end. Some may have slipped through, but I removed nearly all of it.
```
Personal Opinions:
```
- Llama3.1 was more disappointing, in the Instruct Tune? It felt overbaked, atleast. Likely due to the DPO being done after their SFT Stage.
- Tuning on L3.1 base did not give good results, unlike when I tested with Nemo base. unfortunate.
- Still though, I think I did an okay job. It does feel a bit more distinctive.
- It took a lot of tinkering, like a LOT to wrangle this.
```
Below are some graphs and all for you to observe.
---
`Turn Distribution # 1 Turn is considered as 1 combined Human/GPT pair in a ShareGPT format. 4 Turns means 1 System Row + 8 Human/GPT rows in total.`
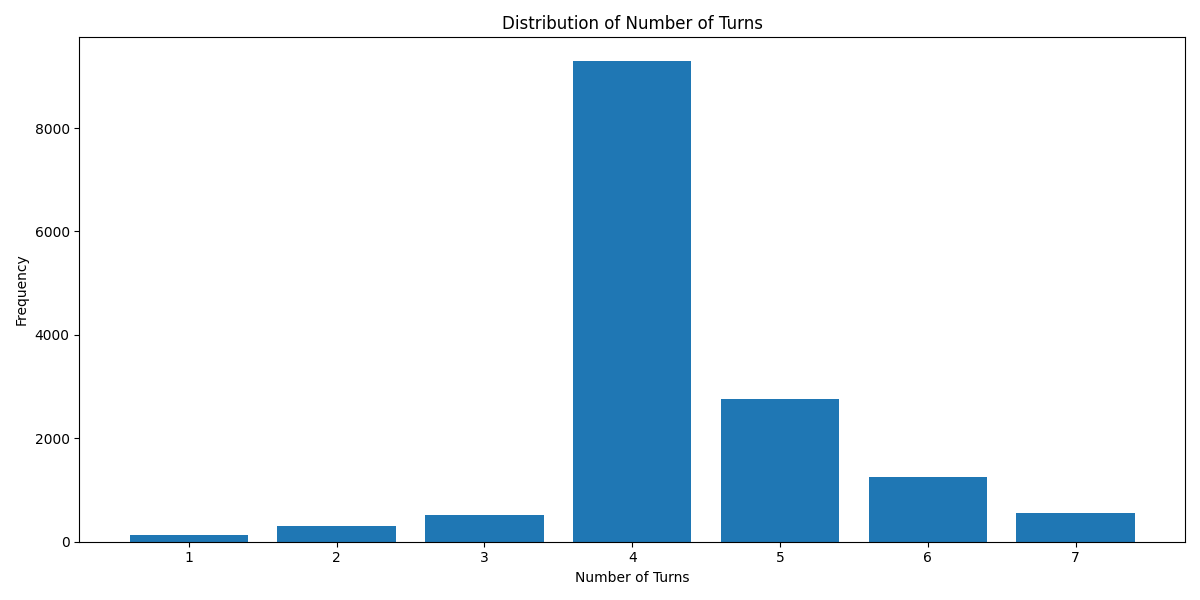
`Token Count Histogram # Based on the Llama 3 Tokenizer`
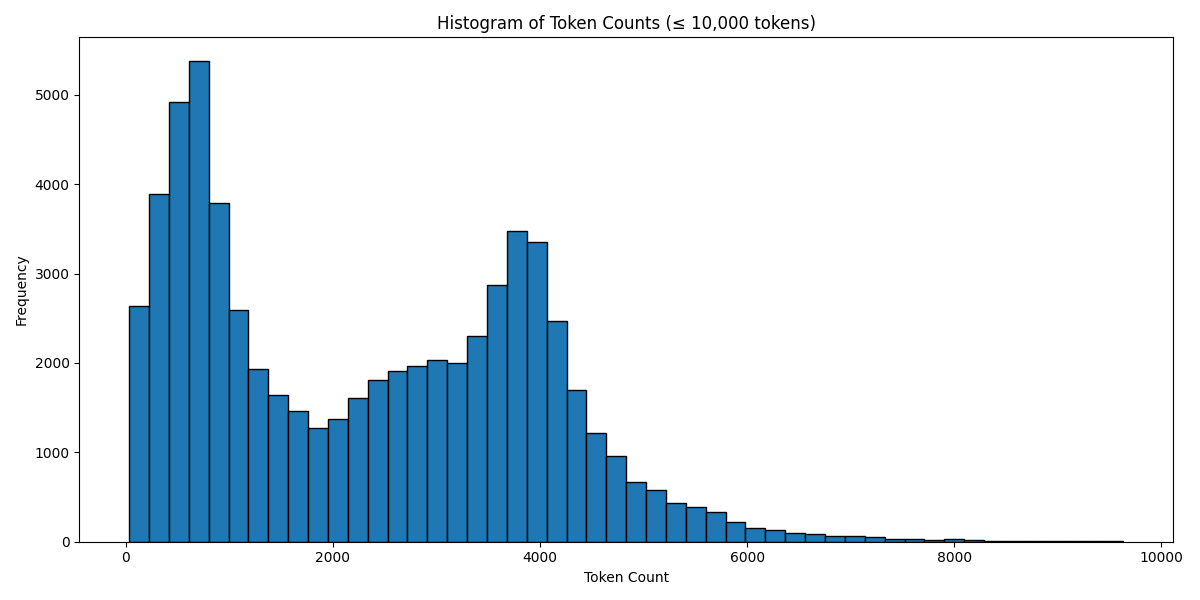
---
Have a good one.
```
Source Image: https://www.pixiv.net/en/artworks/91689070